UofT PEY Co-op
Whether you’re a new applicant to engineering/computer science at the University of Toronto (UofT) or someone who’s going through their 1st, 2nd, or even 3rd or 4th years, you’ve probably been curious at one point or another about what 12-16 month job positions are actually posted on the much acclaimed PEY Co-op job board.
Well, as a computer engineering student at UofT who’s just finished their third year (and consequently have been able to access the portal for the entire past two semesters), I thought it would be interesting to do my own data collection on the topic, along with some analysis on recurring patterns (e.g. locations, international opportunities, etc.), and share both my findings and the raw data (thousands of jobs posted across several months) so that future PEY Co-op students can get a better idea of what to expect from the portal.
SELECT * FROM JobPostings LIMIT 5;
id | postingDate | title | company | companyDivision | companyWebsite | location | locationType | numPositions | salary | startDate | endDate | function | applicationDeadline |
---|---|---|---|---|---|---|---|---|---|---|---|---|---|
43541 | 2023-09-17 | Business Insights Analyst | Air Canada | Main Division | www.aircanada.com | Brampton | Hybrid: 3 days on-site | 1 | $0.00 hourly for 40.0 hours per week | 09/01/2024 | 08/31/2025 | Operations | Nov 15, 2023 11:59 PM |
43902 | 2023-09-17 | Full Stack Engineer, Business Systems | ecobee | Main Office | www.ecobee.com | Toronto, ON | Remote or Hybrid | 2 | $0.00 hourly for 0.0 hours per week | 05/06/2024 | 04/25/2025 | Engineering | Oct 6, 2023 11:59 PM |
44010 | 2023-09-17 | Software Engineering Intern | GGY a Moodys Analytics Company | AXIS | moodysanalytics.com | Toronto | On-Site | 1 | Salary Not Available, 40.0 hours per week | 05/06/2024 | 09/08/2025 | Information Technology (IT) | Sep 29, 2023 11:59 PM |
44045 | 2023-09-17 | Compute Kernel Development Engineering Intern | Untether AI | Main Office | untether.ai | Toronto | On-Site | 1 | Salary Not Available, 40.0 hours per week | 05/06/2024 | 04/25/2025 | Engineering | Oct 8, 2023 11:59 PM |
Scraping job posting data
The UofT PEY Co-op job board itself is located behind a login portal at https://www.uoftengcareerportal.ca/notLoggedIn. To get in, you need to:
- be a student at UofT (to have valid login credentials);
- be enrolled in the PEY Co-op program; and
- be registered to start your 12-16 month internship sometime between May and September following your current year.
All of which means that unless you’re a keen student in your second year who’s opted in to get access and do your PEY early, you’re either in your third year or you don’t have access to the job portal. As an engineering student who’s just finished their third year (at the time of writing), I’ve had privileged access for 8 months and counting, and I’ve been able to save data on every single job posted on the portal since the very first day I’ve had access (I came prepared).
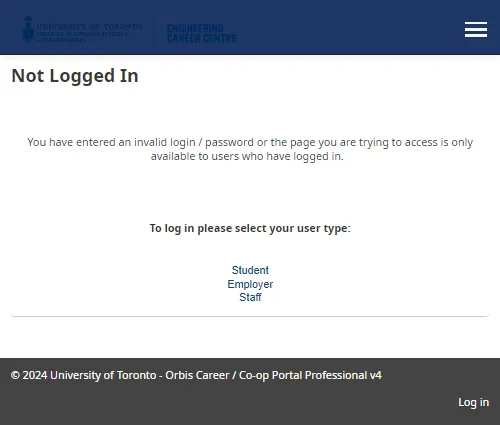
The PEY job board login portal at uoftengcareerportal.ca/notLoggedIn
What you can expect from the job board
The landing page for the PEY Co-op job board has the same look as the one for all the cross-institutional job boards at UofT’s Career Learning Network (CLNx) and uses the same organization of elements and processes for browsing, searching for, and applying to job postings, so if you’re a UofT student who’s used CLNx in the past (e.g. for applying to work study positions) then you already know what it’s like to experience using the PEY job board (from what I’ve seen on Reddit, I’m pretty sure WaterlooWorks uses the same frontend as well).
Sample job postings
Your average PEY job postings look like the below.
Note: The design on the left was used for a couple years, until the most recent redesign in late 2023 (which took place halfway through my fall semester of third year). If you’re an upcoming PEY Co-op student, job postings should look like the image on the right for you (Edit 2024-11-14: the job board has been redesigned again, and now looks unlike either image; check out my post on 2024-2025 UofT Co-op job postings to see what they look like now).
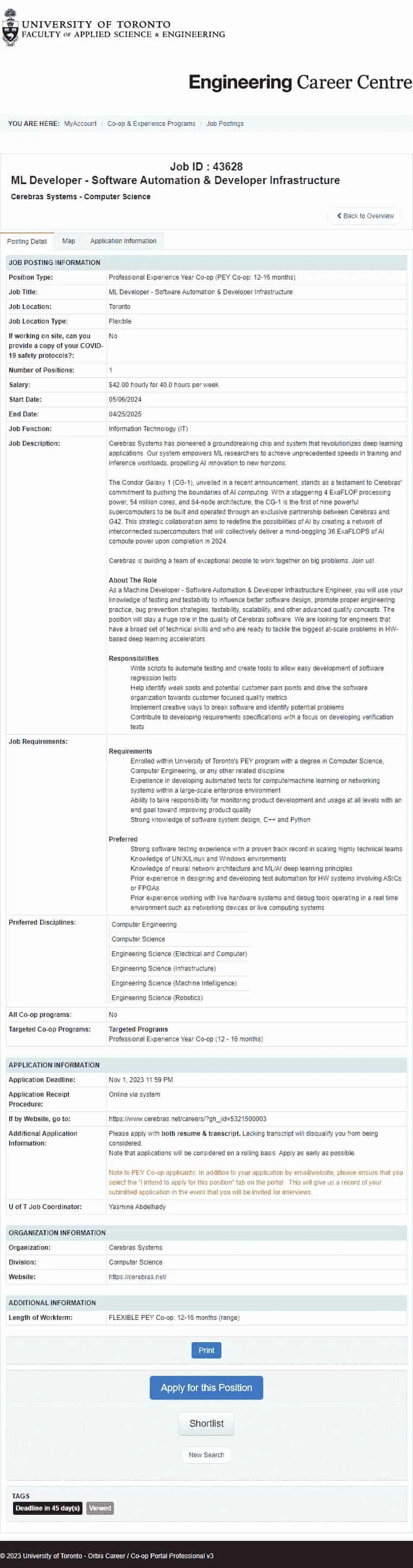
Old design for PEY job postings. One of the earliest jobs to be posted on the job board for my cohort (2T5) in 2023. Shown: ML Developer - Software Automation & Developer Infrastructure @ Cerebras
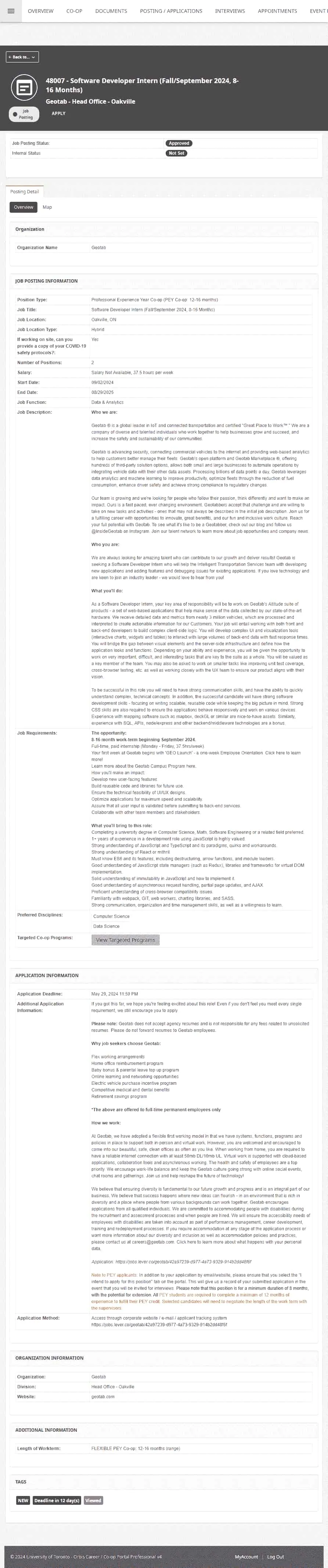
Current design for PEY job postings. One of the most recent jobs posted at the time of writing. Shown: Software Developer Intern @ Geotab
Nothing much to look at, just some basic tables with information about the job and the company, which thankfully are simple to parse.
Getting the posting date for jobs
One thing that’s been important to me since the very start of this project is making sure that the timestamps of job postings are available to view. As someone who took a few months before I landed a position that I was really satisfied with, there were times where I felt a bit apprehensive at turning down offers for roles that I thought were fine but didn’t feel excited about. I had no data beyond anecdotes from upper years about what’s posted over the course of the fall and winter semesters, and so I couldn’t really predict whether that dream role I had in mind was just a couple days from being posted (or whether jobs would start drying up so I should stick with whatever I had at that point in time), which is why I hope that at least one of the things this project of mine can provide is some reassurance to future PEY students about what jobs are posted and when.
One thing to keep in mind: every year is different, and just because some company posted some number of jobs at some point in time doesn’t mean that they’ll do it again next year. Of course, it also doesn’t mean that they won’t do it again, so make sure your takeaways from the data are taken with a grain of salt.
There’s just one problem: there’s absolutely no data indicating when a job was posted.
Well, except for one thing: the New Posting Since Last Login
button on the landing page.
Whenever you login to the portal, that New Posting Since Last Login
button gets updated with links to all of the jobs posted since your last login, so if you were to check the job board every single day and save the data for all of the job postings shown there each time you login, well, then you’ve successfully attributed a posting data for every single job.
Which is why that’s exactly what I’ve done for the 263 days that I’ve had access to the portal (from 2023-09-17 to 2024-06-06).
How I’ve been saving posting dates for jobs
It’s all thanks to Gildas Lormeau’s SingleFile web extension, which allows for saving a complete web page into a single HTML file (unlike Chrome1). In addition (and rather importantly), the SingleFile extension allows for saving pages for all tabs in the current window (this is important for making the whole archival process a not-headache). While SingleFile is also usable as a CLI, the tricky navigation for the PEY job board website means that manually navigating to pages & then saving them using the extension is a lot easier.
By Ctrl-clicking on every single job posting shown behind New Posting Since Last Login
(so that every new posting opens on a new tab) and then using the SingleFile extension to save the page each tab in one go (using the Save all tabs
option under the SingleFile extension.), I’m able to condense the whole process of saving new postings for the day to just 1-2 minutes. Put each day’s postings into a timestamped folder (made faster thanks to a handy AutoHotKey script that’s always a keyboard shortcut away), which itself goes into a big folder on my local computer of all PEY job postings collected thus far, and I’ve got myself data on almost 2k job postings just waiting to be analyzed for some insights.
📁PEY POSTINGS ARCHIVE/├── 📁2023-09-17_20-14-10/│ ├── ...Job Postings (9_17_2023 8_13_11 PM).html│ ├── ...Job Postings (9_17_2023 8_13_12 PM).html│ └── ...├── 📁2023-09-18_00-51-40/│ ├── Job ID 43541...(9_18_2023 12_51_48 AM).html│ ├── Job ID 43554...(9_18_2023 12_52_08 AM).html│ └── ...├── 📁2023-09-18_16-08-36/├── ...└── 📁2024-05-17_16-25-26/
A snapshot of my local directory structure with HTML pages for saved job postings. You might notice that the naming convention for HTML files are slightly different across the folders shown. That’s because my file naming system changed a few times over the course of my two semesters (due to the job board redesign and my reconfiguring of options for SingleFile).
Why not write a script to automate saving postings?
Is it possible to automate the whole process of saving data for job postings?
Technically, yes, it’s absolutely feasible, but given how easy it is manually save data for job postings in a minute or two for every couple hundred of jobs (with the assistance of a few scripts to make the CTRL-clicking a lot faster), it’s just not worth the time to make the routine task more efficient (I’d be spending more time than I’d save, as any XKCD reader can relate to).
Storing job postings in a database
HTML is fine for temporary storage purposes2, but I need something that will allow me to view and analyze the data in a fast, efficient, and easy-to-use manner.
Enter the underrated gem of database technologies: SQLite.
Now, I’ve never used SQLite before (just PostgreSQL, with a bit of Python on the side via psycopg2
), but thanks to the sqlite3
documentation for Python I’m right-at-home as someone who’s used psycopg2
in the past (and of course Python is ideal for the scale of data I’m working with here, just a couple thousand HTML files).
But before I can start inputting all the data into a SQLite database, I need to figure out how to extract the key information (e.g. job title, location, description, company etc.) first.
Extracting data from HTML
If it were just a single page, I could use something like Microsoft Edge’s Smart Copy or the Table Capture extension and call it a day, but extracting data from >20k pages is a whole different ballgame.
The HTML code for each job posting page doesn’t have the best formatting…
<!DOCTYPE html> <html lang=en dir=ltr style><!-- Page saved with SingleFile url: https://ecc-uoft-coop-csm.symplicity.com/students/app/jobs/detail/17860b208317feac1449f4953038c9da saved date: Mon Sep 16 2024 22:38:07 GMT-0400 (Eastern Daylight Time)--><!--TODO LANG--><meta charset=utf-8>
<meta name=viewport content="width=device-width,initial-scale=1,minimum-scale=1"><title>ML Developer – Software Automation & Dev Infra Intern</title><meta name=apple-itunes-app content="app-id=1362056979"><meta name=google-play-app content="app-id=com.symplicity.csmandroid.es"><style>/*! * Bootstrap v4.1.1 (https://getbootstrap.com/) * Copyright 2011-2018 The Bootstrap Authors * Copyright 2011-2018 Twitter, Inc. * Licensed under MIT (https://github.com/twbs/bootstrap/blob/master/LICENSE) */:root{--blue:#007bff;--indigo:#6610f2;--purple:#6f42c1;--pink:#e83e8c;--red:#dc3545;--orange:#fd7e14;--yellow:#ffc107;--green:#28a745;--teal:#20c997;--cyan:#17a2b8;--white:#fff;--gray:#6c757d;--gray-dark:#343a40;--primary:#007bff;--secondary:#6c757d;--success:#28a745;--info:#17a2b8;--warning:#ffc107;--danger:#dc3545;--light:#f8f9fa;--dark:#343a40;--breakpoint-xs:0;--breakpoint-sm:576px;--breakpoint-md:768px;--breakpoint-lg:992px;--breakpoint-xl:1200px;--font-family-sans-serif:-apple-system,BlinkMacSystemFont,"Segoe UI",Roboto,"Helvetica Neue",Arial,sans-serif,"Apple Color Emoji","Segoe UI Emoji","Segoe UI Symbol";--font-family-monospace:SFMono-Regular,Menlo,Monaco,Consolas,"Liberation Mono","Courier New",monospace}*,::after,::before{box-sizing:border-box}html{line-height:1.15;-webkit-text-size-adjust:100%;-ms-text-size-adjust:100%;-ms-overflow-style:scrollbar;-webkit-tap-highlight-color:transparent}@-ms-viewport{width:device-width}hr{overflow:visible}h1,h3{margin-top:0}p{margin-top:0}ul{margin-top:0;margin-bottom:1rem}a{-webkit-text-decoration-skip:objects}a:hover{color:#0056b3;text-decoration:underline}a:not([href]):not([tabindex]){color:inherit;text-decoration:none}a:not([href]):not([tabindex]):focus,a:not([href]):not([tabindex]):hover{color:inherit;text-decoration:none}a:not([href]):not([tabindex]):focus{outline:0}img{border-style:none}label{display:inline-block;margin-bottom:.5rem}button:focus{outline:1px dotted;outline:5px auto -webkit-focus-ring-color}button,input{font-family:inherit}input{overflow:visible}button{-webkit-appearance:button}[type=search]{outline-offset:-2px;-webkit-appearance:none}[type=search]::-webkit-search-cancel-button,[type=search]::-webkit-search-decoration{-webkit-appearance:none}::-webkit-file-upload-button{font:inherit;-webkit-appearance:button}h1,h3{margin-bottom:.5rem;color:inherit}hr{margin-top:1rem;margin-bottom:1rem;border:0;border-top:1px solid rgba(0,0,0,.1)}.container{width:100%}@media (min-width:576px){.container{max-width:540px}}@media (min-width:768px){.container{max-width:720px}}@media (min-width:992px){.container{max-width:960px}}@media (min-width:1200px){.container{max-width:1140px}}.row{display:-ms-flexbox;display:flex;-ms-flex-wrap:wrap;flex-wrap:wrap}.col-12{position:relative;min-height:1px}.col{-ms-flex-preferred-size:0;flex-basis:0;-ms-flex-positive:1;flex-grow:1}.col-12{-ms-flex:0 0 100%;flex:0 0 100%;max-width:100%}@media (min-width:576px){}@media (min-width:768px){}@media (min-width:992px){}@media (min-width:1200px){}@media (max-width:575.98px){}@media (max-width:767.98px){}@media (max-width:991.98px){}@media (max-width:1199.98px){}@media screen and (prefers-reduced-motion:reduce){}@media (min-width:576px){}.btn{vertical-align:middle;-webkit-user-select:none;-moz-user-select:none;-ms-user-select:none;user-select:none;border:1px solid transparent;transition:color .15s ease-in-out,background-color .15s ease-in-out,border-color .15s ease-in-out,box-shadow .15s ease-in-out}@media screen and (prefers-reduced-motion:reduce){.btn{transition:none}}.btn:focus,.btn:hover{text-decoration:none}.btn.focus,.btn:focus{outline:0;box-shadow:0 0 0 .2rem rgba(0,123,255,.25)}.btn.disabled,.btn:disabled{opacity:.65}.btn:not(:disabled):not(.disabled){cursor:pointer}.btn:not(:disabled):not(.disabled).active,.btn:not(:disabled):not(.disabled):active{background-image:none}@media screen and (prefers-reduced-motion:reduce){}@media screen and (prefers-reduced-motion:reduce){}@media (max-width:575.98px){}@media (min-width:576px){}@media (max-width:767.98px){}@media (min-width:768px){}@media (max-width:991.98px){}@media (min-width:992px){}@media (max-width:1199.98px){}@media (min-width:1200px){}@media (min-width:576px){}@media (min-width:576px){}@media (min-width:576px){}@media (min-width:576px){}@-webkit-keyframes progress-bar-stripes{from{background-position:1rem 0}to{background-position:0 0}}@keyframes progress-bar-stripes{from{background-position:1rem 0}to{background-position:0 0}}@media screen and (prefers-reduced-motion:reduce){}@media screen and (prefers-reduced-motion:reduce){}@media (min-width:576px){}@media (min-width:992px){}@media screen and (prefers-reduced-motion:reduce){}@supports ((-webkit-transform-style:preserve-3d) or (transform-style:preserve-3d)){.carousel-item-next.carousel-item-left,.carousel-item-prev.carousel-item-right{-webkit-transform:translate3d(0,0,0);transform:translate3d(0,0,0)}}@supports ((-webkit-transform-style:preserve-3d) or (transform-style:preserve-3d)){.active.carousel-item-right,.carousel-item-next{-webkit-transform:translate3d(100%,0,0);transform:translate3d(100%,0,0)}}@supports ((-webkit-transform-style:preserve-3d) or (transform-style:preserve-3d)){.active.carousel-item-left,.carousel-item-prev{-webkit-transform:translate3d(-100%,0,0);transform:translate3d(-100%,0,0)}}@supports ((-webkit-transform-style:preserve-3d) or (transform-style:preserve-3d)){.carousel-fade .active.carousel-item-left,.carousel-fade .active.carousel-item-prev,.carousel-fade .carousel-item-next,.carousel-fade .carousel-item-prev,.carousel-fade .carousel-item.active{-webkit-transform:translate3d(0,0,0);transform:translate3d(0,0,0)}}@media (min-width:576px){}@media (min-width:768px){}@media (min-width:992px){}@media (min-width:1200px){}.flex-shrink-0{-ms-flex-negative:0!important;flex-shrink:0!important}.align-self-start{-ms-flex-item-align:start!important;align-self:flex-start!important}.align-self-end{-ms-flex-item-align:end!important;align-self:flex-end!important}@media (min-width:576px){}@media (min-width:768px){}@media (min-width:992px){}@media (min-width:1200px){}@media (min-width:576px){}@media (min-width:768px){}@media (min-width:992px){}@media (min-width:1200px){}@supports ((position:-webkit-sticky) or (position:sticky)){.sticky-top{position:-webkit-sticky;position:sticky;top:0;z-index:1020}}.sr-only{white-space:nowrap}.sr-only-focusable:active,.sr-only-focusable:focus{position:static;width:auto;height:auto;overflow:visible;clip:auto;white-space:normal}@media (min-width:576px){}@media (min-width:768px){}@media (min-width:992px){}@media (min-width:1200px){}.text-center{text-align:center!important}@media (min-width:576px){}@media (min-width:768px){}@media (min-width:992px){}@media (min-width:1200px){}.text-capitalize{text-transform:capitalize!important}.font-weight-light{font-weight:300!important}</style>63 collapsed lines
<style>@font-face{font-family:"Roboto";font-style:italic;font-weight:100;src:url(data:font/woff2;base64,d09GMgABAAAAADnsAA4AAAAAdEwAADmTAAEAAAAAAAAAAAAAAAAAAAAAAAAAAAAAGoEQG6NaHJB8BmAAg0oRDAqBlnD9BwuFWgABNgIkA4swBCAFgyYHIBuSYwPucFdDbzoXjGMreBwIbotEUckJF/xlAjeGaH3AExtiRQynVZWflo42xR04ysTekpfb5qp5ZxYb4mNn4DgfNZfoae2ft3rmzO79SEFUwKSIQCXChS2xyncBQEWa8O0Znl/n+9euit7puFOVJyJGT/VkzTCMsUixSptVWWJRVv43p5Xe9n0F2gFKO3WiJQRJ343d57YpZ4Dj+C/1as/eh3BvDDdiyXLrhUKWVXbv+yGTAHgYYoZBlAS0jiiuVL4OUbRzgwN4B/T/W/vZXZv3Rlb8wH7EpP1QcUnUL0zIhEprRJoZZbNMNbe/f6vnfZTN+ufMmGMKlEQYj3pXI82O9l67Oj1o7x70uHoSGO70KOlh74wQOiLIzFoDsQwYE0WE2YfmFJLUaeaPXA4yDKLcmVOC/6/7Uv/UATp2fYCacAjGez79qoeTrStA20IBiwdlNJgKUIB3nv/+YvqO9QW/petW0d6Jwiagj83STk5NQAVUJUbBo7+c/n3HrDW1krEbMmY86uKZinP/qjAS5QkKq5bN3ldPE9WQV352dp2kFvJs/2dFSg6jT0icRUmcBmqrMKslO5/sQzreIHLm9v+fO7G9p32fRYIEGyRIkEHcbd//XXSzQAQ8qVjGv8sfQ7XdbLp/PD8ggvZdh4AhAuB5mr8kAxBzASBZXxVSqEKKVUiZCqmgqFVqHo1GreAmHAKz6jzIlQiCsid6oJgnBjRMBa7k5+PCZWnNMASjkTSRZtJy8yDAuVQYQDzwwXZuQODZWcmxFnp+Mjcm9JLg1PgDQAAcryVe/NzkeIAB1LUDAQYY52XCgi0nCOD07WdDCJ9b70uN6Jd5ZY+6RLo/1O2vs3YNeZ938Y7TF3C/A/3NgHGvZTv5GTDPN5Hy0z4O9IFR0mdc5ieIzPGr53Zk3aYnNqtUtmu+waF1uWv3nxsH/2Sf97sDS/ngfpbuvza6/2KMudZ2FBc9pJYqOOTvJ83fyPO8y+uqlYO73iHOFPd0Xmbdbtv9DGQhH9OHgtqreZFPCEb3HUor3viVSSHo7rxKU950f2ZSl0XAxchdneVFu4eRWipzfCEDFys7kg36QzYymwiMrurc4k2f+IAd9ly9sG/VdDr4L8d4PlarPod3s3u311HJxcfTpVywcjadnu9IwFR9stHr+Erf1lp1cvsiThf1DtrZ5eTSt1kUKMdnlU5Kt/PDWJkimKVB5bkGNE3XJDTdzwPP38xaniZeQ4Jd2u1yM3aGpItVYL6tk1t3Mrq4lVeZt9e/u2239wD90EZ7uBhkoT4C5wkhC0MdcJKT9yEo0MBDiwEqc5b4WaMQu1aYMzciPBTj04rzE0BCZSut2wAZQ/9HyRWfKfvhD/cAqIBvUBAKBAUD8YDg4CAcCA0RIoKwkEPBDTNMEeELn8gIRF5UFKMoJ8pRlxt22AqjGT1FsYyt8tjHUU2cI+rjekBD3OOrMf4R7Qn6gI6EJEJnohKtH0x0AlCgDDoKxcxhRe+IIi2MvDBK8lIXhi2MpkRaYmnLQ0cGXWn0JNEXx0Ay8xbNkjWIbQtx5gLOVQQ34dyFeIrnLZKPGH5S+YsRIEGxMNXC1LwF6dSFqVsPit64oOvT3zlgYF7IoLuslumqW1BuX8A89twevvAajw2+As4bH6Ft+gzyww9wP/2B8NcuWAFQACYwCAZggUNwAIUH4gHw4CE8IIYAEQB+pWc8IRGADDnIoQQh9D3YkqFNtE7gIngAJkIRQQkrAh7RQEUs0BEPVCQCHamAIhNQ5AKKcsCiEoioBixqgYh68ERjD0lO7+SEs2gqcNEKTLR3qBMddaOrXvQWKwIeu4DiEfB4BTzeAUtAEBO4h2dswnaCJ6HBSFhgwt2l4QlfIghaooKc6CcIYBI5hBfeEiQQwQEjpeKXQSGDENlC0jiwlBFHpZhkDogGRWcSAlgE4IMMHtJAsWAuHRmsxbArBJ8ggBd626G95GESniJufqEJhBdBeBEcgq6UggDsKcUd9yA5BAILfoXqesqw5TMMjSAKLvBAKcZJKaYUAtAKSyCkIrIHH3zg4YEXBDBQwDpryUhCXmAiHelOmcgoG9mZRjBAwIGAF5bDwhbw8ICHBzw8Z4hYl+CPTaBjG1AcAhHHPdy5A1dMSkGAOIPio6DHdw99BqEVgVWwCMCDADzwQTongrK/D7xuY27k/MT14O7c6i3e7I1t41Zv2c1ubhkUeW7No7k09VM5QdNnvD3TyIxno63TR+Lw4UplI2fDD8ZnH1bga46h52xy6rMGlJFIV/aWuWenci4Npi8VHr2nDx9z9rzHLKwMesOu6hngjDns0faITPD82bw0lSDpEFBw8GgkSZMhS44yFeo0sHFo0aFLnwFD1mzYcdycWj925cGLD1/+ArfisA3/yRVXXXPdDTfdctsda+6657EnnnrmuQ1btn3y2Q8//bLjtz8BUCAYhIJ4IBxEDjN8EYhghCIckbAiGvFIRirSkYls5KIclahGLZrRinZ0ohu9WMU2DnGMc1zjEa94xyf+CUpowsJNeCIShcoOiyMWJyyuXcCz6/nC+dn0q2mn6XfTn2YRdutLpQtKLbsDVpy4JHB7jaXUH2njhZde1etWROrYFTKBRO4SVL0GDK0hNggwXN/8xC87ftefViqldgKfL0m8qPF9BY8lrFjHNrmrsWnLtk/1uRWcFBlyZTchBIUMd5+AzhiVZb9lFazZch/BaGo83UqP9OnPJrZs+zR/phW8CyFHmEik+shAruwWID09nZstW7Zs2bJly5atx1sgvv0aEbZfRL0Q+3rctU2/59HrvPwQ83dxPSW3ryrsPmV9VkWfMdi3IRCQGMPz6A+CERvoKMcXHgMD0evt++b59nlxx6LltozCtlfWtSq6H45WT9PpTfp9w0ZtJUCTNt05aLap0FNxpyGtrI1XdPvjsh7v6lYx+2Px7f/BY4899tiaVzsSsbuF8UJU2L0ZHIeq9VnmyM1Ezeck24PS8YsWOvN+wrI/sArWbLlyv+/RG7z7PZ9+yz9Scxu9zTSAoX61PVxz3Q033epv+om33nnvg499sz/Ysu2Tz376Zcdvf/rfvv75X7uLArtvCyLk/KFIPcG1aHuUWD8pcScRqUlkKjkq1KKxCYdo7aIDvdjdRxB6wro5nA+Bsektvv0epLCfgiP0BuP+Jri3wYn3Eq8mvpMXIbRmIdBBp4tKH9OG18ii7msXwIwmbbo9FD3cRGywBHQc8mG0er2CFP0Dz5DleEkD0oA84JsMfb8nmbOA3wNc3N4BD3YxDpk9ytFuPaVHG3uoj3e0H/BEF1vh96egplMK7BTPw+CBbW7zHC/6kk8EXgPNSAcBfJZ3Jm8eZw8NjUsE1WHxCXGg7P+PWgzkxkZFBIP0Sor2D4mNT4sDfrEJobHA41c2GTglNmybVBFsm5mz7i9QIuElOMEgPEe6VLomUsSMovcRnBiPB0bYVt9YDVV9hcckeHDJmGZBDAiB3D95GA9YJ2VLkeqU084465wlI9G4Y3MOmzXviAWLjjrmONSTAwc5C+olwIpdCJ1wRwYfLS7weceTXZhVNOte2sDFiRAxjgkBX4Goj79sxeiIwDcuSxADEy8+/GZVGF3gtUu74GL0YxDDGLmeRExj5nSEmBltFy44IHQ0PkZNxUKIaeTC8ckn3XFy6HXE62TdphCZGGRvikqFQBUZSJCnzeUOVA/VSq3oCiqPUiix4vuh6JYvTuuETK9pMTWm3PgxXvWl7uvaDosXrtOwGEKDOjDQ/3ysB3WhRio3yQmKQ8wiEAzYsmzWmB7NKkVCEMJEhg0SwDRHAUyLL2AafQXTBIIIQ2mmNdMlqjZvMNFOayiNciQJIvhQfxTVhkxddLoR3EWrA7CAvwadYcJrIDLnd/cwlxRebwUk4ai/onHyL2IKbrQSmBM+u39jhesKfeOr36KmfoqK+jVNUf1wK6N4vYdnHB45q4GbReOycorkSjJQWg6xRb7IiYzaEqmTbb+W3QrewuYV6j0SvEEsfxuUqJgcBV3R1gigWd9YMUwiLB+hcJ9RFEhhEejdhfgZNi9Ql5cXCQHXPBdBI18PGGTx7tcAO+hyH6U6Uj1Dlcco8wTvytp5hCWeFoO73YOrReEcO6YKQuTVOoqr+3Os7Bcbm7sgizR3kGyttWxXsFZszQU3EOcqoo1rTvpz3j/AbSlCUqYBg0MX8ZeYX3TmrDHZsifI8Y96pS8J/nK5fxehgCJKSbl3ksoKHNA5XOVqYkB8s+cQJSjApEfCNAck6tKk+xMCRalt5By9LwciJFMJbFCjltsHsQcvM78hgfX+Uwdyw3rm/JHwF1N2i79D5PnKI5AbPV4e8hhHSwAB/e+B/8Dz9o4GLNBHAOruaxoLC/xbASMuBwepiBwpfTwjSFTjc41grz4TJp226roN23Yh1biOE73aD/rRQHA4nAcufiMY6xzWeazLWQIsEZYYS4qlztJhmbFSWROiYmLU/7t1lsKbsEi/35QzzrvhjU/R7LJtMOIyFh9LaLfVWNqYuLE/csj1OSJ9FnA+YAPaJzpfiC7qiObVqAZVK2W3qCufg3KlShTa6/2zWKE0/tDT+fbNmAMBHuuN8kDKo+0sb9B1/Jp88kJXPPoVee0VKfbCS6+8tqFEqTfeeue9Dz4qs+9X52dZuQp//fPfLgTAewMBCAwGrHddBJCXAIxfAhq46IvAdZ8IwDnvATal3yLG2z0PQYKDvLCawAz4KKpYAzDhag3IsB0KEdgKVReob5VcgGqBBCyijKguZt80EIhVEtyEpopdvXs3eiPN2vBiNr1ecZJREGBVZoir5k1zMnj/eG8JFvynCoFHoYRK6bpSkYrQELlJpNkHI4q8fJ7QFNqrJFehB3glH0p/WAfBhGIWk4xIulJkMQVVbIIlPw4iDoQP3wNalJom1ZXIGlWAFUvMF4lJdaL1VFF4EcdiAhMFeGqi0RWwa5hboWmEG4a53/lMoJxBTmw8ZSo2w1wTyWB1i8SoBnPJlGoUaioOVMAyBzT7aIzyPCxKHxmqWSyiBFB6kZkhjCTGSySlJsUfD1eobCWk6dlzWn5ltqdV9oBYePgzlryy4cDH8PKr5IKHTFogt3YXf04vcnlbB14yd1Pm8FWJ3nnjaSRZ9X36eIrWIjJhjACbQqyFNmZJGqG1EIBpikyb6XRKjLh1gDhFbq2LxZK9c3xz2ns8caRjI3hkRwb+2PtuuZluojStp9aynkhv+YxFpoUEtUcwJcMJzj6qPZlERhYEhsVponSPaEBnmdigpZmXl6YLKv0Dc3ez/d+rWhXnV+Rvy/yvXRq7ZtRjbrM2MvRgY/z2dsrCK0NK3B6vpdtJb7twBQKIjcZjIXr53ywxkHWzLnmfy1xX6Ocbyj20FbAjvMn6zETXQwqtdiWmhXv6iCXfyvcbOrdJvQh6LqM/vPVgmSPOwNtWJQwrJIKg4qgri8mfSBZVyVz1d1KpIGd/12XBfqflOYJF+d/D8aIIMYX2H+dBIVAvD611FBbvEFTGhDYyaoWL9AqtN9ylaL12eok8lLoFLbLuCHD6+HusQ6sFRdQmLrE42Ons4uRYNM6s0ijNZEM+Zdc9rdLCSPbkelH0u9nOduIU7Sk4F2Azn/KPOFcRvqaFZYgXu5nQ80sswcvqG7e8gDb/e4W99KerMx0Zc5+x6KUq1hmVXSDswK5KxKg0xBJvm/VLhU6MvW9TRSqH6MkcPRnOk5lgUahvagVN2UYiuGO2KKKwlPztDahBIZX9PsdYbV1VUiYs0G0tKHBsvrIlc+3dTKMekJsei9teWwHz+wLGwvnEQt2tcEL+2m6N/M4vbLblng5gxTEr77ALFEIUSKs5y83ZGqep05jHGkh5F1LyRchE2toCH/JiLJahx8ppDt3a0GColkq1i1u9sLNm1kCnqLxoEcug0rGIUgJPvHanDk2GXTifRuUQdBuPbewUkQXtJxlqyg+nQ8SaodnZ2kp80yhqsz0zMLsH2rOsTTL0IYgY9jmmsb28308QModtqT8fqBQWOQ1lNA0tNgxb8Xgp0GCVP9RaxaifiCU6NtkiX5yorXkAkg2aBtnROFXUN/3ICk44Qmctq7YTbttnsJlwlN4gKRwsQVSVZq540QQWzvuTAQsBm9M+/Ri24E4zKDJuMbmO7AbHtFBAZTnepKNYi8p6tOUYASe65pKHnM+v7byzSw73nAQQsLPVHddedIfP2Z1BllDfjA+BbtQRKAMCuzQEwgjNZB7mH/h6ePJ+kW2tES4sc222Ywb+frI+cWvrBhPCUYU7qX60tPDCDFCjDZMoFKMUYIxh6PVgdiq2FUoE9EVGjg4E+T4+qdhf97rddkw9tVAxWctI+ZBieI2c9V3miJ6jzX0J1t4erkSd4gFwuqxg7ecHzNc1z+PBmGgCuPeUbG5tel9rSk6HJsehZTermK+nRXw9/2IJIpL9TAXK88v8rX+iGbOn66mqexbz5oAqzoQx8mQ/qfzkEBBzVzzM0WKfGsxYq7qica0TtVCIP9ojZGuCUkjPYiJlbAZCcgAd+Lj01erhz9jZV5Ack/ITmctR7CMYdzjZcsPy7U7vBP0j9wrBMmjWSeGUHFuYjLgdpWImqjskRO3bxPjLyVsjPd/mIl9dazG75pQcPpJffqrrvrPhGmsPBcwnwAqpSuMDYjCzniolXLiOhqIr1CO5ZISyS6ORWqX9n0lIRuLe5mcm1ZJhwTw9h2ha4glMCY3xHFxIoUM7MfPSZN0JBxeL5X+36/xHxF4JNsV41Hgrd7UcS+w9CB3l3BB4ZR5aPel8xZKwO5TGGCUZYkfu8gZqifgcXCNgsIQM1TeFphPDqnGyNBTEqXp56UAm3jncsFsefO0/wuOfDt15QoyUSG/X9GuNp9mCXy2lgKR7P/n8n0AeSwAh33QlgHgB7d+M9YI+4TBx3Wh419xabtX92IJA8CajuU/4i5Of3qQm6RNtregLQX0dj2J/ZuO/SK+H1WIqe+VxoElIe317owptnpq8Y2LqtvGJWyfHt8ZKKExRxIC+gAQtFmbdfVqOMhpaKtwwvtPkSuGk4hmkTH8cphnkOXlE86z+E8ZkvP32K77zg/4St4IPdi2WatGJU7VGBCArhB2hnu4Z9zmivIbbtelFRFOANl0Z1OebqgvIuFoIorCMlsuVuBrpPV/iVSFnsoTkPMxX3pfye/3P/CiReJ8whRed/sfq+cGBW5mdPuFo8xQVhELb6Z65fQty7A71tRkl0ysEQ2EGzqCUsw4qktcXGqR0zHSFG2kLDYE2IXpjV6SB7ArVznpAMK5T1Ew7jZYRqOgIClxDkvOmNUIJWP/I0A/lDh7edOz7QaOy9f33MR3T8e9EpOnSeAQNFkn3/gjXzFFqsLmO4GzJmLiN+dKWpv7uxHtZmoE86yHcqWAIGtUpSUMbxtVUA2+mm9rFGptOXzH4wI5oo5amlHQKL/o5bxq59za4LGBDHvoweYifAS5XNTbkubW1iK1RKT+dW+793IRfg9FptNaBQTVD+CfVfPWrvuZ/mDnmiP2vA3XCqDP31i2U6plDwwB2+pdr/KnwotC6IKHAfFaASB+sSqdJlOhfKuNUneL4OFmedMZEAOfWDQ4XHAtVj5GuvckccQQ4Vm09MF3yhOcx3grA4D09kjTgZAinIiuiZiluV9xY3+mcbL4pq06l7WloFbnuWr0Si1+n3NrEuBMB/8oivA+1cMRukyvGNB+g+WgIU/SfzkkTOY6/fEwW1582+LxjzrIEG1Lc/++lrTa57XJ7PHG8oOjvIqlnfb83mWWAnF6Gc/0AEk0pMk/fxmW87IdD/uWQPxzM13Nwbns4tJU8cWMG72FJcSdG0Aygl8aq8agpkenVmhNNy81lVHPAZHtAbbtWdTsylLyAE+Uq1Pdoy6sLbOjRquv5xgULOGqocF3bpBNX/eluBADNfq89E4NRKR98V4+k7rnEaNs4fwgvckxzbNQ4se8l71E2Y6zzelvLswm+SqK7em5eIDXcTK07RfAEs3Pj3DhR5LhhrFM8q695T/6sHV+4PgG1Qi+ngaj5SmLWNCUHa8wsnTiUkpA3TsnvpZfOHu6+ibbwcrPwqSlnJBAsY2wx1u6u5n41lcxogl0ux7kmKWuKkouz5yueHE1MzpqmZGNNGMVTs+1ZsT5oC093c//KcmYMwT5WF23l6WIRUFXOTMPd7dV0S+gFQqbiHD/55GhckOvSaFJE8r79lAR63fXW8ZxU3pIeqbuaMt/aDu6/a5Q99fTc/SM3r/IOE8PqlloTte/dXlnIZu2rjCyZJnoRfS3qup3hdCZPknPRRM5UHl8+yScy3bNo/CqgDbuobqsr/eQf1gQKFp5Xshxustobxdat+Sdho9DLbMcE98xc/zJX5BZqKxYRdW8/G2SsXTmzl8qrYd46NjSK+JBlET8tw7vCm74/MAKtPvhxIGT6xzq72LrErFLLZl9M1Up6xXE94qwR3aQPOJy8lXrb4wfH/cfpsLMePzUB7T8lOoORxyzl0POEDgLc3ktpF8ELZPzagi9Hj70hrwdO0pI7k6yeIGvRgcqCajIk16upncmWz1FVPDEqgupSJCt6czi+u64nb+6Hmgq/IgHtxpPDvlkaH3H4m5IyQXaTvxHwc0jIqKm2rPsHCKDU3r26cemi4AH+ps4TXW1v7u7p5+w5ivk2MXvqLv8h/tmr68nZCCBIg3lP7ozz1/E39Z9o63p7itXlJDKDezIzdfYR/yQpcWhmn2KBeNEpWpHALO7FsZwzMf7LhAwOvXvpzsjo25MC3fzdy5OX/YFq4dJX7nezarZp9UGgx5ZAGAP1wU1PrPgQtwT8+z0xN+NW2GZZGDALZU+k2Vyn5LGe/EScnFx6iD7CQQ/0He0oP98dqkRLo6d2FaWBOWh/KtB9vcwVKI51NDV099bKd2rJGs0zu08toVdUfzifl1Byosx1m1bObNYtHD2D0KtGjYgm0/Opueb3+jNSQRa4ciBJklJBzuusBuaStRrDtVoayGwr7j5gHGZASz8wWyyfJ1uyTM0TnN3zcDX/TJjnHC2VUXj81H7FeLnoBUqiwDj6wVzSdJjDLM8ShxJo03nAN2oMc1pwv0DrqRPjE7ATaFoYX+XM6frO98cE6vgrJuZa2rZmgM7AVbRt5tt9pQ1Hr/KM8jRUcDamKqGssQjXS/lQvNQDA9/iHAm4QhofJdYvpbKedEy6iJoKGcNTLU7U+UXthW6O6tez98KzYE1dx0H//gMelR+ygznPszKBgamtfJqc/RU5rjxgD/QMemR/yArm3MzOAn71IgeK8/MSI0M87uHD8T4ZXL/Cs4NiNZqiPS/O72/quMHfRYkvPQ9W5dsnK/0g2GeJ/cvSIZEeFJqP4na9vhoYvg8q6etJ275SaxClerzB6BQZ5mjlbVl2foe9yRePiMSczOPD1J085jFE0e7fj1xFnivnIHrnJwcd97R6XF8RHms0R1yD3xs6wnKIP3hV2dwbqYlYKkm/cVG97w7Cytr1UzLs/oxaWckfHYckqPzR3Zo8oPX58OzbWT2V4UNGgFI6rf32bRW2uBh4k9amE+5Bd1cEJxj53RN5wvmMuvO0AqEj/PevUK8lt5Uu/Doq3MNfszgzXsPWX97qE2oTaDg0eAphCywKT+reU/q1nn8iI+xlDXDA27HOqbAc4z/efP2DZ52NPrO0frxko+ogXWRa4gbW2AwqcrsxHJ+8TLine87901e8D1pLy3uIKUe4ElwF+kSOrMhKjo4AlHs2cdjM8FOskzJBsIcaT4kQGXm+PAQD+mK3VlI6pdIC7njF+KVJxyYtAQPYlTs5lzmklekYrmZc2m1gPt1l5ULwG139jMY8gjngbaXfiMwu7EF3ta9+fAo/yZ64fHzqrqGp93+EJQx26eaX+dotGztLm9Gp8DfAlpNzsyT3Joc0P81JHS1JG2WDhQ8fNWBoPs3BLr2WfIiBLZw6/FboOAfviDUhRzcnZg9++MA6AX79xa77iz80hIdmhnt5ONAVfKgTvb3HBLSzb3MJpJVpTSCAnGZwA9XdA52Oehzimqe6uaccY947YXgnkgc8NOTMPa7GemiShqfZcu6uxBVXDlCmFS2nxTyHV0N2eDvR86xzx5Sx3Z3Fy+kxL2AVwLX0CF8QWTiiiBsUXnoDu3b5w9xjA3slothlrAkwuXp5e65ia98EQXRS6gHeCuyER4U7/Loyfwm691rkzJ4zgk+eJrKTTyFOsg4Ijxy+eAReAVQ/sdaQBS9JfkK+HlxUrLMS969ziC8jlhfZs/j3MfFs0vz0qWKwHO15kpbF13TnQTMLHyes+XFoYvjHReEu4aGVC6cLgSFlheZzIDM0+kCsWRPJhxzd1LfhWVS4muCzRMri8A2s3Dk0iG6UyGJY3ad5zaZ2K3w1O32Zr5Ec3zqy6Vf66s8HgXPNoYMPr1y+hnr5TGEX4DifDSdJZnP0Ohzi6gBn9jS2aU3qmSrhYdX5LM/nzGrs4Yds7OBDkdNLX46eE/gpdhmbcgls1uDd5LzkuMJ6yoxDxtTvM9qgwp+7vWcuRI/nTjTvm8htYXqwnuTrQ3pYjXPMRqsO0eVj+jwr0zpA6jBVpcvc63L5C6O449bHTRPeZ68YAztfGXFXUyAvXuEvwQlU5B0sNgeW7IG0rRiLNUefMJ0CyAxISdk8pVraPeYyaJ6LNICfn9GibShrAZ67Bd/8AuM+oWraPQKia2FJFfrda+qj93TBEpuTxgF76N+19uMMwRbMkxHnGr6RUgbK+0dpeXxtrIOPEZBQN72kqa2q/GG9YJqzYOWfQz3Dh44xO0KMUaZmxZhNVin0ucQtXxF8uQhHNfLmU0KK9oF1WuJEsKyfyDiH3Ao4KZU2kRcSUqOaIswuwLOUK/HG6u//EBG6uGh0rMbRXH/f0ve90mle27wpIznZ/U8x8m/ApGjtjX6xIo54x+fT/S2Li9RBbAAh0Ju/p/HPjYK9g8du44Y4+Lqe2YqTOxoCMLjVdPcyiJcWHKiGzhekJ48lSUYmVYdQV3TC0uc8Nz26LtLsknud81mj41QXsoP8C8G1PONY79/0wp7avPyVsQR5cJFWdLzB5S+tMom3oevfvdKcoaOfCSeUaqnjBFe8O6N2arwAHINaWnSbJhB3FDq+bOgcfaCAt8RaUtJaa0AdDgGfC1/kaxdoPHiwADJ8/nz7cyTU0MjM569jpDNxdDiICfOJSBtJFQ8TX+HgNOiST8DvuauJC4XhQwe7nt2SPC3caumzGpoW9rOujZ8vu1FReH2vcbLne1p5V93egvMH4+QBGW7gVPSwOi23Jh+bibQluGTMczLmceaQKSIqOSEalEN/byjUZ8yV4rxAEQc3pMNhuI6VtUi7o4P/Ns3femg0X6qp6bkMF2qpo2cTENMSPy/NsBQ9XG57wlx9HG33iKYr51pLlAkoKgTKbiVQZudcx5rqoYPSIyJBFfR3XbYh/aDT26upvOv0nvu16WX1JfBsy9SXlYV5dYXYdJ/z2BnHcWbK8JaUGh8D/GGLniWtdI/YkNIPNeCoyte6/+fL6n3xB4JDz6BSYabEKL7Wtp0XlWUJYyFhp5DpcBP9GFr95FhZ99+HmscUO6gVjPqJsZLuf080FxTbwDot5WCotGe4JYcs+YAjccYicDU1G6oEeTN4TlaslpW875JO8d5hJo5lZQ3QrVD4AOKlFQEksZA4FiMdKX6GberCibS08loNSYtsgNxt3NvbkJtxsnmvHFAwoxshyl6vSNVkL8f1jyLZZ6YpSwWZUr7gP0b8ONshfs9rG8GTlOynLc2DC6OEag4uqbu6pOBkGjVEuGfNpFmO/NZNaIla8Lqjb/T8AKGYjUjoKWiogJ3fCwTYAarim+GfME18HJipvt0zIScM9aHhOGqKWlK8ezZ9SVUc/Bedsgi+GJS1t7EUl4NyILimjnHSp7GmhoiYjPToxnc9EvlFvzRxQzqg3FPU9bmu+DdtNomuqwVU1YlPReAbHFQKJPQ67J3XHyOROW0OuliNsOh5lq9JloMu0Z0G93zaH5uBJbKSlBHt98xv84dKe3DGoMq1WdT1hr7c8rZ11f5EP0iqw0WGUhrUJG0cNEccUtEG39+mqcewm+7Vpz8A8eDaQqxUbi82AWWJtZX6XuXM7J1FY4zTkHVVvIXi77WSLKNrqlQmH/N2iL2QRt7Ts2Q6xyPtxOMlv2HNE2hXluyAP4FDXj3FAZ3l4hFqlqw1afg9Q0uGcxzCTi1B8gePGdyIfPucjU68THYfNgFph3OQ/FTlhLGN9PCEY4wzkQ2ttL1iL7TiLaKAPXWbv6pvOA1KCfLnhrs4+gUVlvFlBwnU9w6mQYmhfvHxDtaegVBhGW8GOLFwQsnI9CeNC6K1N3qkSzkSzY/ne4kyzzVlnnfgR59Ah9MskIaBVTfe06TXNaXX20h9VwgdHEJt2+HK9GOZRU8EasjhcOR410UQ1aFoKnDaO/gJ4I5wkwNlJGdOKdtcSbusqM869/Zzf9/wbd4pNo9ZNSGgKCay+uJNkQkl/XAuMD7TeXXqRdxZTzO3y7NaV+fYAaRI/tFU59Ki1rNDTacu9a1HH3Mxd3x41vrxuaTjkm+k349N1R+bNxDL4a/zI97urRlWujTriRshnHj/baYOu/80EZggDxmKFeOCw/Nkt3PSc7XM2RzzrPRsue3sdC7lJUFv99otDBWQCAXbnIJtbPEFTuF3DjAOPkQJn6hoEUjTCGwnRfJ3k4+fvf74rcCKs8DEqasLLe8KpOMFHU7yF18x8RD4a3p4gVlC4g7V1ArejtpznhRR0bXunnP+4jvBZQ4p1myiOyBmcLOWlUU16yO57I9W9WyxdHZhRfFJ52PHwoyGKMYUq33B+dJtivWI1Tghb4b5hmTFtjWgltZKGDN4z0lKODF4Z2YZEqpM3nNsCSMG7wwwF4vgbzw0XBJdVkpIwQcKVYkOL18oLSs4SK5WDw/E2oQ5uyWOzQhW8RegHFEh0WleILmujDeHbaSNa6n/ulFUeSnu96Uu8gVCS8OXVwVVN5OQoWveZ3d127+qPjipj/Fo/fQ+7KO2v1V9KFI/4/7aiXI8mDwtqJNSWAXFpKcQ/UiR89l18HGCWY40ysjBwgRE7/NjuGkyIqbysxLi2yjZbG2iL48XW8am0SufNduDQO4ZJVi5uwF2cDVHXflKGAzorCzv/1fZhyD1MZ49PFWCtvtASIOpXh8pas0SX1twzY3fNooqmwUqH/1LMsK3+v0ivRT9/VrnMzdOIAcDN3hdCJXmkhLp+3otUlXp7VhjiLhHx1SdGEXwsnDxhi5onvOCykKIzqKzLxBHgJpkhLSbDCDfuUMI7Knz90usJwaQAvdXBwQl1VnwFdoYcPQiLNgZFc1Whhr60daaWUCiSbLFfb/lVY6cNOl+iNf1bJzZN3muPCjeT47pSAjwJjuRnBXP2/gXhjC9OIyo4aL4yMx0YhApoiTdwlD45yXAeGViGgQrdSVakqx9fdx98ryZdmx60EBGdEJWNimAltpvYMdnHoY1IgSN5m5U5mLC2RiXuCCfwBJ/kgPBLswjGWYNhBi7pl6hbgDqsk5cIc4MWOamVycOvrgoNZPcycEN6aiLX6p68jOsLkAkMNxGgyz5AAR5ocMfbMBFr4ve1IReDJBWpsGpJfXzBCF1I6bTX8lgPjtmoTg1F+fSFVaDBGGwKuuntIA8eqZ8Bpjez8m5/tc+VC4IByTdl0tLzjBqKJECXadOlBTtO8moI0fwtZ8+uyCPdub6uyX29SrCxRV4XML9nZN7BxX/CQDyEQrXMqc2+2NmYvAmKQLvnR0akn98mFWlKTZ096xKz1X+XmpK/cPO7BuGMRT/ilPIV2jYDfBIa8zPZMzUnDH9lTvkHKHN1fYZHrX/PKaLHmwAkouVglHN/NHt0TLtsar7GpVLF0uBMm0hM750yBQMXI5sQN1FeUlvsc4sKuF6u7izNgZOVZOmYOqBehgmAZNIidtzSl3ZLzoVT1jyLuG42bDtBi38Z/u7If3OMHgFGSnpzqGbRnHH1rV11eGSt+hpgG++drZW6Gn49SeCM4KzfM9u+GvbHwn9fY+OzzrhPgWdFuoUbJteGoWDOA5eIlx06eKd1qzOfnJKvIRb7JywVdQpo7ThVpNCnDm95PNivWe30u8ncuUyJvlrXcxUvIlJTm+F5V6sWYqMf0D/HvsskmdKsTdUAM1M7RkClix6/tEisXRFmEX8l9zStpEDjFL+PtTiBQLJG2tkiw5Mi+Y23mkVyuUIN32bGi5u7YSK7Uued+wtHW+k5uOcCG5E118K10luKFdcZV/3vsWBwqruKkOWRtLTaLa7vj9scrObv5weV1xhNvir6f0Do+VzCQQrtDkxsS4faM0LNt4kKCBC+BsUdvE68zlzhU4v6XvFH+Gwp5OnfZWcGHKN5/8WOr/PSsy85d77yDu/fD0hGPNVoFUg4nB6btXaULwsM4eZO1af3f9WQO49MIFNXQq9dMvjctURir6i4DtYWsIhKYCTNP6p+GJ+1g2Daq7SdwXZC5E+ykkHjUDx+cpU8BAqcEJ3XLyWmGVehoa8dbvTJGhZjuqhMwym7GO1T6QSzpGIBPGvGdayATN0mtyzKWpfyeDt46SUJfYeyq3Lw28A+e80e7AIPfmOugyzxFkqCyhRrN1ewhzBFlWdKxObtJy2nNopZZnIaIKKiSSGaz6xDroOl1OR/6cigDUCRrCTZx8B6SGHjqtnyX32A39O100d3RE6TXQhKVwnOPI4k/cNjuSA61BzSU5H7TOo5sIKuc1hEHaso231KLOHkmEg1vPxcUH2Imx48kpLxpW8+hd8JaRAHBTueKjRLyjjcl79c4FSMtddzK79xyu94/cZXJwVJb6paI7+S1OyT3hi+lih8ivVZwoRDfuFavBzTUBZ4zePMWhYWjtrTEBsygDSfDjeNinYxMIpksecxyw+1NLEI9hIkGBhy7Yx6+ZIhqDETFxjgcbKaap7dIiF3GrtvHSUWLYm0T4q1Eb2TcyqtIaV+W/ADPuu2YQzBATubttb1A0gXHKZFBKN9ebkk736KcbwrQpkjgvWnLZviTEMvtwDwm1hrlIpUSnMImokmxwhNAII9Pwznp9pSiSHHC48CtTmKRGpfAWMQg49/+MjKCapxoJDj4hWER6mlvZ+aBN7gnm4p7mFvQfaAozIP8owfZZM9Uu0BECpTz7JFfOZX6MBufeLgFDxIU23hENgx3KxgRpB8GHUn+/rCs9kZcSJRB18PS/YRQmjJTdcCL/AOrWasWKthrJSeOdRIJIVKRZ+Rwqtuhl8POOo6KlFYJFm8vqAitM5+3OaPnymPud3Vs2AD5/m0NMR56dazk8HMwuB/peb8lz5k7L7sUDPFhxcPtoAFUE5ujdTNHOgDOhgF18VI+vELKEEx+vVXZmP448hO0VBTS2MPL7aH3k4R3Zk/MIPzBGJFyYz1jknfF12HG/yPEkzV6GHT5aAc3RuB0eH+wewKjrPjreGv/3gdNE2RfoebiBIaqyiuxgYl7nofr3+8hlYum706bPOfU1rKd8ym4KR1pb63kpo72Vqk7x2vkagn66PkZKv3UWfS+OXbA4jEAgg1/Pu0KAMYY/BOLvWP9jA+GRUuEE5LVtgcPRozqffDnP1Kum5QuNjM1OGkKdbsF1YZae6CB8/5Okaah9a0aYhxg/eH5Dx/x90629tYp3YZQ3Ry23A4JP0mRe8Ls431Y1AzP643MMhZV4CzoqhSXvryFGU1L76zLCMjFA2ZO/h6whCihOpYSTf0FhfLuSnA8RpwsHYTvidOYXWkJiK+NT9lBhqylB7TGrBADmrW+wu9B1YWObbxB7Mi8aa+TpYmmd5GTTjjMe9zEHFt+/ftLdffHFWBsHW9JwuHWshizCMESF4MvNTVSYpmLZvQa/NOKALH9Cfvl6QmXCPZJ0U7BPQ4E+yp/q7KdlZBNf46V7Am4f5O3kW+gnWhHk6u5Z4AJ6U0NSaqOxOchw1ZXIgKSApEuvrVUV0lzj39c48Kd+8Ce2410XamOhMQdi5edpZFfix7ESscMaTHjYAy7AgG/nZG0hz7R5QfUQDfv31sjVhEhz1/zF9xCK8aCbgys0CYPS9ls2o1tJIyyRw7UFGzoXhtJGM86qnUXQhKKlR3TLvuOzE7g/ALVD/ZGBHVT/PkabopCGLQv6HRR2+wWN3OBr9uFVHrgDuSJSBhXtYwWhLdzQEgLmrR8b+SB/0EjYSRTV4dlzvnfHwkSkCOPO6oTb8v+pWPdjBXwO77xkFHuJgxwPPe55RELX7fyDc7RAQ7BExMaNmqDcHwZ20m2Tu2AWGh49IGW3yHNPlBXlRPiofk467KCDwX2AhoTMA7Fparyq9GD8KUGaMW5AcvMgsmjH8vMu0fN1lXWBjGkKJw/GjPbM49UmHJB3Oyov8NGUHSQayrPRblh3PA3hLbyTF1/pp1h+QpxnJ6/rIfnQ3Sy0uCqQe4ikrbpKnDXZeF7NxQ1wTehcdMYu03aKySBtTC7Wg7Y796KhDv5uWrdKA+djMEyBPS0de58C4wSLiONo+YL8+wrLMHPFmnVzsXVq3S3Fcp/l1E/2qh6oSf0jPO7neiNuVi09TY0NB7FGrC5Kj8Ob65UL/R56WkrxO0+meA2dxbl9pqN748hxQ87R053Um9qNVyr9DIThz3n4MkPTCQnOZspRIimOKy17hRVWqO+VXVUGSGSAneG5yTW05QhZNl+j0nnLWXizmseuPaS4HLhI94Wjauwkz7+Q4kgwKPltAijdghcvpBCtLYyK7KlY9MHmTiv7t/gfdfbPhWX3xkf2ApXiDRt9whfQ7Qo1r+ndG7jaAq4CKni6pOgKic7ycHJJPrdXKdlHBC8luS52oSJp7KBnJdjyUwkqRBx8ISymx1E/rfQfS50mDj9Y+iFAVsTsTrOpr/EaxdFunMgD7buvYgTX1VGezO3GNtO6fKHj4nzwUuD0paXdMzzFdVIhA+65lkYp5pwonK/0S+SHo/3QhoZZhSy2Uf0Fbvn/cEDxk+luDAqSBAkzAwAu+hr+xI3A5hHaELeiXx5T8MsORZtqiXmqUaGRDzhFPIanQbgcrCzWCMpMMIspCK48HqIBXtAsvSDmEP/RGjohZSLnDO8XLEc6WTJWocpPTavHPVJerSKUzxkxttzbpggCod0/U5yRLIxLOyg3ek2/iILL65RHCP6FJ0xJ1+dDTJfsvtZAnLz5xomqX5YJRAJ27BrvMd3yabf0kN1wmUHCugiJdwVH6gpcG6GYAtFpVu/ENmG7/UEjA6epvMnR75unv/wy4pH+Xcsn36UCQSsnbq2HqznKqJsbnOWkXqH+vQ9aAdUu3GRm4eW9fi3/7RO3eu9Nmx80tHzSo9eAdfLLOVH3rb1SHKn/IbgA8tn9PjygJo63v3KTWUdOXblEPjFoD4B33TZdv3pXgQDxF/PXcHRv1fSU369kfZ/2WSSsrWfYtfNumXzrMWrkr/Dt35A83x2TkD9qYzyJQ192NIBtICoOeuhPab70j2n6uig+zWLtl3tH++Z8RZANZIR8BCoH8dgZY4xMA/GMA/HfckA+wHrsTQEZ/3Gc/d8iusrEsNoBf1UZH241kA1khjACHga2qY/SHGX9Q39tt2pyxCORVtN+4Ffv105keZphZB9r9cY4/IH3vv/xRLOeYef56jw3Gndfb8B3XXWbuC9YUQ9yFvMx41/5l7Yr8T/4gX1aXJA/eflDf25U3t76Syc8Wb2930opixtm1nyvTzjP3TyEwL8nOVGB88BZ49+GXbrvgke3v/OXkXGjAEJjXZsAjXZ8Vgito0/6fK0VrWtBhEGIULBp5Ld92gWuDvdnxo7e7+01gPz+TeHlcXsz6j9NHznHPpdeJ8239dQbYH78bGQTWrx6EHgbp211Tgj1XhI5rFRL3DYDfuzJEsQ9KMhE/uL05wFa2t9bp9W2H4JrG0CyMrwOP5sga4+Eoxw6dUSnHV0q0mCNrNbm6RHC274rwL8+wxrL7bY60ju3mkw87/pP9t5zKsUWdpnJspMtBEscxbQtTtVOSRlGO7fTqlMQIki8C5dhpXTNIr+78cqcZofeAhe4arew8W8sxrRX0zopAnYecVinuh0jiZvIyA3E9kUaCIDlh0bWi/Yp2dleihx0279/6ZSOxmxNJLdU/NnDrgUKWwv7HdhgGnhRc5mHDY2eehM+gC1Ebk37xM0Bpf9iSHYxegjMujvLWVCK45XA2Tl45+PZ0iuVo3PVP1qdVp79/z8Ct+8pjlqxpFWQnDrs5zykcG9GfQ+b4gB+I3LPHdsKIJ/gsD1gBeV/jWaPAOqEJIBWYv7peOXYU5AwVJbS6zoy1u6xpg2Qp/U2pk0o2x5p9qwOmncPvVAgwbnrpMednEJD0/oHhPwGAr/6ZMgDgZ3E5+31vrXXhUwoYHjAAAfaXHHgATn//772/Zxc+AegLbgfKS2arMNX843O/95EfcPCyrFGisbbrtpO1TDdu1oanxBHeOWMlu8I5ZW9fak8mmSM/od2Du3gESiSCtIg2USSVxJ9cIHKbNiYGxadPLxp4VoAUvyecGevwbsimJrK4i7wXRfES4V1pXFsOnxWgfeXxGWSFP/j51E8+hQPe28yEl2s+W0YdriP7m+dR/RRq0tnFUVUTRneKM1i529U/XJP5VnNYY8dl7kWix0T8NOjapkW0xDHKmv78zTS+7/xKe+SqKlui/WUaZpBdjn0rOKJkt11wp/a0cmTsdn/vTY6BfnNzg+LIl9AxeZ6Wut4blJV7lUx23OxKBmkmQ0HCLkxp9/RjylBUhffOHYeZO1RTjVwT2ZqsSG4+8maSazPT3uIo30KkTWF/Jgz8qz34xxWpumWZ+e1FWfeP6dOYO07kdQbujf89C12fiVRXu13kWWsbHgzM/P7yPubConb/HRunxvhUGc2tG2+WF1Y8j7KmN19DqpnVLs5vd6OXwW8BRAgNM/FpijkHnP3AwPEQyKPIrQrPge8C+UjVqfS3LgSz65uaGFnB71AU+2BtO5PPXeCxbycbqsuchpsgSoVmZn/pKzleulkt+1np5oqhCn02s04Apzvxm3MIso+IB5BK/gZpEGniTYDLBs4eDmklCXwU0kGukJTwWFnzzEnn2Lcwd9k8p2xm+74f4D279DhwWvM5uAWzFQJUgPFaHbviqAKr4LGmgE+wWSGIvCLGm3FZDWCyAgCAGeCPqNk0o+4XGAcA7BXYxm6BbwDAPoFpsAR2AHCDnQP3rMZcGIdTghMDDrC/2CFkiPlYDYFUlWhEbgXXwoDlh9lYYdEY2OiqqH6iCv0lVRIDSKORc26w8ywDnCScBQDAKUIbZwingNXR7FpQ8zEfdp6wS3qe/bKDM4VTwU4VzgWrWYYs60+nmsFAQF6iCZMnJMhNbPoNnYSDg4oHAE/zQhsFYboyCgbvzCg4CV3bRIxCkpI+CkWMGyc+LvACqSWcSIeylOEqCZZ4eOiIc1s3QbII3FhRQi/jxkvBleJnx5opcw5czV+RGiUqXCQIeUiqq8hN5H7/8aylCvbiHock86ihE49VSlWp06SrEpWumn7NrC6sq1Kr3WcxfcOJsiSLiLnZIHpUFkuecFdjcce1Fi+UEje+kNjxLt0iRcX8mIGn2/sWRgkc6G9ARwtzAwA=)format("woff2");unicode-range:U+0000-00FF,U+0131,U+0152-0153,U+02BB-02BC,U+02C6,U+02DA,U+02DC,U+0304,U+0308,U+0329,U+2000-206F,U+2074,U+20AC,U+2122,U+2191,U+2193,U+2212,U+2215,U+FEFF,U+FFFD}@font-face{font-family:"Roboto";font-style:italic;font-weight:300;src:url(data:font/woff2;base64,d09GMgABAAAAADtcAA4AAAAAc+QAADsBAAEAAAAAAAAAAAAAAAAAAAAAAAAAAAAAGoEQG6NaHJB8BmAAg0oRDAqBlgT9bAuFWgABNgIkA4swBCAFgywHIBv9YyVsm0a32wFz+n7vQVSwbRr1oLe6IkwSWBT1MHIKUPz/hwR6iGV7inkgQyQudTmbt91xrK5oWZYhlp0iMv5NOR7IIOUAdVxxDOnj/oiSK8yB4wR/7u3GNskGDigTeNfwhVZn5Ki/wv0N3D0Dx/moufDE1/X+qYsOpUEkdQDN64grAttkmR3+dw3xc/u7d9tYNCklZSEZoqRUSeaoHBmSEhE2zsImFAQRvliNEmLht5LFvNJRJ/ApiOezvTw7Mzv7HkW1eIKt8XgsjvhWXHVorER2YRAG2frfa9S2bgiRJLaHTRfNEhpcI5RCxaO+Wep/n858XztjDOHBpxKYZndkS4kMlFOALXsaX2sXTYD9ftERdAgrrXwK3wUIgHndUluK1LubJtqG3Eea1BCVmXmEirXNPo8gFlNs9/+v+Wnf29nszizPYrYIDo9ndHWVgOrNfUNv3rzA5KeB+UTzMVnGJAUARULVfSgup0QsCVxlna5VKwtuT4WqkK66ENZJ2k6wtLaicbtaZ4InF8DqA1D18e3Fy/Pg0unmsaMiNMrESsb+zCFR1bAVdddTaIOC57+uT33HtgUbF8dtamnu/mM/oMZUaCpOUwEKxn5CFIuWzv4xt2lHW+gy3dlzkm+0/aG1pKPoxkYYDVE2ma4wFb7ipaiz03YL4BDEV+KwuL8KgzZ4AXxexJs30Vu0HyfSWCOq5vZVPxVh9rgaGgmNSJAQghTi7r77/602M3MAzrQJ0v2qLmPDQp9tESmTbVifEsGQlYOPVHovYydInC/I7G8VsliFLFUhK1TIaoK6VuUzsVEjxDHHYDwwBfKsxEApVjtAiVckOEqFvCwzgWW2xtwSkp6NuA7X44Z1g0BGqtsJF3ulWxB9o/+51fA7h/fX8Ie132MaOIDdNce4tz4PRNASuHE/k8k0CxnjO8NB+ieXFXEijqE5ba2LWXJ0ySGpb6uq5dx9GPcmr+3WMxwIvgL6pQJ2/F8+JCe9InMLLrn5C4AeHCR9hAfxP3jq8GVznJ82q+77cyIfKrbC2ZXbsvRH/cxf6ir82m9mfGY/SunPeulPbZbl4u14trvUsAic9Tcxc1fyIq+zXGg5sw/a+VgJRrOYB/WePUxvzuRdurGwczUv8z9aW/AaK4oXNmvkA+3IUupy7T05nqqcBciOmYpMzDk4iaGbs00kTODBLIcQNta3Wcm+RyN0aUPzV138LfbeiyuTVW/ZnPnFIQXvzksfovB975E/wB6Q/VxaRIVZplNuvmKARhqTFUP+vzhcdZaeW0vno2f1Dv5JMzJz3ocFw57xHhQ/VB2z6GQEJVdqSvLfq8RbHJ9JfeGXaMzdPC+VVQC4qOTElbWFpGuLgFit+J2EgltZyul6wV8dJ65uBPSxI6ire4Gcu21D+AJ4HIgJht3IJuM3HAUaSHTsxGbG3AaWIM4mS3C0hyRXZHhmWd58ySnLW3TotVX//0pmfaTsm99cAnjBKwpR4B+DWAiIDyEo6QGCU1KFZAxxMr9YWNZiwkuUkFGqnMwKFeRVqi6/dn0lDRurbNpUdfPmalq2V9uxo5YuXbR2662tbz89MBHxgxWsFsCihFLYBQYQbAGzHYwSwtTBaILRhk4HMl1I9BCkD78dMBhAsRMms8zHnCXIOkOOnCDO4OwBcQFyg8qj8PnuxAFsPhD5QlMApgJM5TeoTTshHTqxdEUW0K0HS28JzKMFXCcLmXMLwe13Yp56AfPSMpIVKyheeYfPex9B33yDfPcbzh/rdgADYhjCAAtHOMBYxAJKQALQE5EI1IkuUjICPkXMVNHST9iMg3/jH4iS7QExe06s3CGUR4S84ssnQn7xFRQsLFhUsJSw0sKVEVZWuHIilZ/w3CxSRYSvJEplEaucA9Wq1KpRr351EGog2EqojVBbYZ1E7zxRCdvIA5G6S7CHiD3P5NXreiP+vmL2+0CDiaPwIBzCJcZfiDVms2qDNMtWgLYVnsUmUwYKlRLisiENTI0AkWjIRIlgomLcE+xmq2zSBFk2Ipvg6ISDwrfq+IckIpFCX/vw8UeYGGFiD2Jm30DAXrLccR+eQhAZdULY0EtBaz4isggXJEhYEdwoEZLggD9k/jBCJ48IEVQkwnAwBNgG64EC8FNioUJDCxdukSJbLCKCEAiFTGHIBRUJFQkVCWPh6r7aUE989QUbCdd4omy7oJQQo4EL3ILtJIH2E98WVOEPjVRkokhEkYhgPNHB5OPKBYRxCUIEZj6F+Ef4O/ir+LP4E/gDuFpcBa4YfwKXiduJY6I19ATNoGpUhvyRJ7Z60CBgJqwZ42JxmCftKgQmXDFjJx6zgKk4gEEnjgFVI08ZiyMbD1dsXY/K0MxY8iGC+1DkyQ408zTwBDRaoLqHqwgAthDCw5rvmgGnV3IGlcEF4sIhoKDit8kWW20jT5kKdRo0adGhR5+BnXaxZMWG/dr078yVO09efPitBKzCRv/vs+b854Z5N91y2x133XPfU888t+CFFWs++N9H33z3w0+//D7XVKent68/Y+as2bLnyJk7T74CBQsVLlK0VOkyZStVrlK1WvU69Rs1jtTT5q3atG3XsUv3Hj179e6LzYYUe1IcSHFeLrdV80LxfUM/0k+/6vc90Xgo3ZJVH5FqYpbz8jWZ0t7RxUuLlmq5Jckp1G0Cj7lCbF169a8hKzREzp98xw8//arfrXRlHyB7m8GdGi8sTObBgmWs4aLSe2s++L8+tnBBYdEaTIgjYKJs0wgwwma+VRbBkjWXEYBfZUuH0indevIeaz74f/lIC+92zBycCY4JI1qDN6DOzCW81KxZs2bNmjVr1qx9vAbSL8voZNSXTl/oesPt2ipvuOYB93Zw+XfRGZKZOXnpVpxxpRnTl9twZCEbLVDpmbcC0HW5sU4vDQYRpw+m7zvNK1Oiz1mZ2SovtoqzT2l6UKgfHr28Z5B5K6WVKG269Bd/a1dBDxVihrFxdyzRz7udp49PS2h4KJEy7z311FNP3bU0E87qPUZvdBanK4FT/3k9zgwzQtj5uU1xpXR+EB0CzPKMed6yCJasOXPZds0Kj7zhmVU+kcrL6IqQXvT3ubj6zw3zbrrVX+WZVa+98da7/j5vrfngfx9998NPv/zuf9bf+Otfre8V0NWCA+nbYozE1tJxLZk8K9lGLpuNbE3yVKhFYxEtorOKHnbE5jr8CVTwsITyQzDaZo1X3oAsznOILLrCKF8E5ANEqVzh/jN7nn81V6TQaEiAPjYDQlYIlRB2iWCXaE61CZUuodIfQQQqpISzoJzD3ZBrqJ8H5S/jzzvj7tXgjtQr9YZ6e03AF1fykHc6W9RRxSwOm/ZjN1FpMFqpM/t+1MKp5d5/CBogCZ1N3C5UuNq9FvJDSHB3MRjcmH9mEnxtli9v28b9peIR87wr/u+jZpN5Ox/XuGcpovfA2+N/5317bm9cf6n/cHjpntVPuKmtbs5JJJe6WMA7a5qEttMVLoVbJ3oXzsTN/bFlCR5cB6q/qiu0jIvKEwfU87eTOGj90xt6InNdukRJRowaM+6yK2249n0756QTTht2xlnnnHcB4eZbxJbnfOBMOBBykxiwYQdfBv6i5gJozQS+WS3MNW72rDhpUcZETbmrLj1hss0viqr3J0aQEGEiNrSqEgR4ZqWvadfKoHbWrjK0vJ4yKVMbbVywzTP9AP3g2XAPg2rIOBHNLVjmZMeb39mxL5FCh8bdF8bHxnQwRKRCZKEEJCnYbnEf3F6qkzQR4z+uozAyI/IjUflnV+MKXO5r99JY8v2tl3qmu3tfB8dbwaF3t3izmyj/17t6VNM1WJmVUP420qhG1hhYM+GEAzrVKxMGR5wQJnIkYOqL18Jbg0+t1uePdSBchnpZt6ynbYdd7ffUmKp/x5MjSQT7B9HtEaW6lu2qcjs4Ql9Az4iZrn2GFus8LCav+UaQS7H6U/J3/jqcXBcbnYfiW476Gd8zundf/SrZ6ntJVz/2M6y+pdNWNr9jh1kN3bpFT5udTRwlKVv18mi8la5oCVdrJXjng9ny4Ay/0PuJ6g2/V7zDVZ++44oTtVKpWZXmVybM6N2b3T2/EyjYTIpo51XSm4KXcmXxTTdIfSiXHdOcxvRCDjpuqye7enZgtSDjqZRntjNT90Tbumcse5d5T5mOjzNFlFj1QOLsYYiz4VzHmxYiQu4ILN9d7p9hF+NdKszzmeNZ/C+MP+b9B3iOIjxlGoi06KP/CvOXADOWhFizJcb+T3pTL3J8FPl/FlGgSInXxcrkYJUo2J8MKbga/EFWZsuuJAJg2UGuhQEwe4wxiOC5S17uTn44OyweYmNsTK2GewfZQndbHCV48Du3OiDFqkb7WmjmUvP1NVoEjAJoB1AcgOSrYwmC/r3A/wCMvFsbFAf3AeAabh4pUtBE2LLckMsXFScXJu/KEJ6c/d5rWVig2xFHjbrqhhUfrMNMOffFvtqP+smC1toMttQznNSjUo9LPQ0JGgw48JAim02aPUKfNfj/+vpD1gHJuDju6W3ImCnzXvmfDXbaI9j6KQhQjtxFAv4+td+yRD6XSWYcIDMQgHpG7wQ+XHsYfjhd89/avzV/K/4mLjQsVN2/gZKFooXChbyF7IU3C1ELQQsaz/8+L3nWAo+DUwQDgc6EHCN3dJjMUmwOyTfMM+vJAfnXy1fgpUVLlq0oVOSVVa+98dY7xfYenF9hUKLUH/AX/APrEFhTEMGQHqPrGAAyHgDzCUAByJYLKPx6BmSYBwRcj/Bw0xx3wWORQsNU2DD7T1paOrwIqy0GIbN3BZsFzkXStVQWgEX54cZhyC1ahB4UWMyzZIVEsh6G6rpxYDUvwchqotWCMbYVwC+cIDws9qA2DjD+/a6iDL4zl8n7wqP0ZaT1O5ZmTR1kFxh6lcc3w3KaeeI+LQzw1H5Rullla+5h7yiRchj2TCe9rFfpa981CISBnfXjelDmMlqlAUK0iP2wlkHu53IeDbnvJaywGcP3m1UdA99kPitlFajWqcDdMTljaJ45pamXri+DZX0/TTcJbiOVQcI47ZMkdVe2mCAnMeMeeXuMMLvgjimACadyNiwHkYfug9gD51LTvxDK58cEnrw4E+Q9FlwQDM+f8Mtu25nN/CAOuKi+8T5+JKsD4JybNUPQUZFA27KKeWrExjfjmK1lRooiAhvr2JBRXz/ei8gYIuAY1wuaSEVWrFVYTTK9kVQF88Az2+/RuleLEu5yx1xD1LKM3Fegh5Rh7a6I2xvefdeQicQotscIqDMkvywmuVxWU0VxJeb02p0guUvapu5Wq+NlzdHUMSR1rrnoNFS1TBVH93JeGp5z7WHcBLPJ+PztiS+nQLUpEKz518huese2VidZleVQm5BjFL1bjWsLIFVuYKnIPetSJdmVUTd5tCBq/BfR8DF1XUcOepV7nxYkcMDNs2BYEPL9AuWdYk8VXh0zgxEIm1BZMKutLhUCZWtbn97JdbVx0x+aW5AQpMgl0ayt4SNYJMKE6ulb8AeYJzqAW2f7oGS1Coz2Tt0Mb2bpEa5nifxDdJiFNDmetlkO8yEKuWNfxYLv5rxCELPnB5uFczz6Wd+PY22sd9Ju8Y+8YL+mUMqWNzmriWOm0TbOiph7c3lm6XdnxU55Y5oZJuZw6Dz/r3R8Rck7INiH44UBSi3IjbDbqyXAq9cikq3XJQr2skfM0/nw9JK+iBO2NKOczfyYEiWr2UH4Ra1N7bzoRUveK1BRStRpEwGtJTOd3qmpsQpimh/eDYZX3Vrwi6/y0Cv5iB2xxWHyvR71sUMZ4NE+8I80JOvbNPg+iA88TN1aGIRSW4l9GuNsNdFQSpMl12XClpRrCg3rojtu8NatRSFSF7miSBuILysAzlTKuZLd7cCM18s2fBzKll3r4PhLp9X67ZtZYdEAuAL3SaNb+4ZKZ0MADbxWQuA6MaB1jyfKgc1bAxhgR+38vuKfPE3PvUuAR/fAYhd6i9jbpj3wXRuXqMjwmpUEQqSs5GabwHSY5Uy6SbsQ4C7YB71JoYKFczqbQ4GbRFV2LgCwzaMLdF0G+6xHJOIkJnqaEkTzvPIidHFxJx+cxp3hXMVO63uCtGCJ8X2bjm33MQTO5JpLJpMCj/XzmgTaHWgvmd+UaaTGjaBm3bfRxijASHp5Pyr6CUY+QIJQyD5jGFvtdpkozeUCS2UBXa74WA/+xPgWIEXB9pNVLH/ebT/TPhpLaIz+ouIfMWJxS9APyIFfewcP7oEM5eSY7nDRpomTfqpAQy5Gqx4RGAlpb17mBcQgZ2fUnCQcjrZOULiJIJfwk+R6keE+xYLvp4MfVMNbye8g+SIcOQmWdI+DI1YzBuQkHtnqgssh9CiUhD2OwGcze7PD8lNGv7zOZ/GB0T1g9DeN6F2gVkA+ug2A7r+RUeYf3IKDDnbDlSYEuAFhTRf8msryAAs3w5JvnBZbrOtEizVb5rShjEOGInVWoQuU55dB0FlxCfZvtPpzOk/QhfH/g1CQyuiNn1Rl3omugBg5E0qZiH0u91PtPH964ssOoJR7+cfAMNjZ1VFfEQ0fgWPoABVZWXbdXiBaKG5wciShTpNmTgrTaDoHniKsJrBmYCIWoWQ/Xy/AoznKEm8yBn3uxrJgKOCjmOF6AUaZ1chVf5Mtz5TVSDEZeXVdiP6N9ba7KyuTrhPn0Q+NLYr96ETItOYkICgwajmMbkF/JdWk1nDdJPrGuzElRWCMSXrndAMj/e2oFs5LLdeTAXdX1MdYgC4A456r5MvgpuGspMA24ZoDwqOk5sya7XPrINIc97Tyw9MaBK5c66Z/6QsPHL948oTRXbYarf0il3oHvNPBxCiQPueHlBO0H/y98OcgMqVybKjC6VWTxKNM9gI+wCJLFHwEtO+oqUL//OmJ8WLnKMkj5uQc/ToH4E8u1UDZiF2XrXXuYj1zVT1g6A+9K3TpYvfMBkDslKKbb7IhfFjhICfcaXOro5otajf3ISwAoDJNGWAIjbrVoReNEr6sk6UqzBo1Y8XhFEokNzsg5fqdRAgvO4OIb7tARLONevR8Hx2dyYIvmKw4zI0FbHGsSiBpyHU+JWNK1hp3BmhqVAUN7RQYP5DIMcl8oTUQt+UCeEx4yov5L5FyhXLaYeETcJOz9wNssWEMuA7A1KdpjNOVbgQaTHLAikiKkZJ3bEOsS4gSdLpIgOfLW1FyWbGfI4xYYohXN6RCKBxctYTQYdG0vfULXS7AGyyhrldstUzR9tPb/OtjwOpf1mlNtLGBYi2uB6Cg0mcuGEmksIafM0v828Cb1Zi84j36E8dsABUcqCYM+Am4+q9zdHmu2bDyJERRV9LKlrsUfQkp7utPZLY1K5zxy1DZ2kEpb2pCp8JHhUg2ldrfBdGZdSsxMrDaWE0SimLorWvQVq04r1bsnD6WXfSgDYcdSJ5Zga1R9NIphLxAV+QC6HspFcLILd+in14fRXJyJKCP2Wy4thYvXOGtEc5Ymi1196MZpB8O+kvNMyYxs/R3eTi54fiSGZIxGQUZ9DADA3BmCgTi5kq7oIaa2rnr41+M5ozzWjmfDS7OrLiDBASc5QC7OfdF18V/Ix8APMXqIJLYcFNYhDLHCjo/mQe3GZlriHtdCLJc6Bn6NZq953E0/Iczaal67v4gzYoOgcPk2mF/cB/jiv7RPrJgR/kmGmSHA9AB62xMqOLAYQflHeT5qL+Wv5Sf9Zp/3at7uT5jbp0AeN4ECvEGeD5Wvhn3AyVlsQdBnxLog8Cn+hgKTixTuCGCtWuj1A0ZprgJvAiEGmJM1BytJgLmqkw0nKwnAsBwy/aaltDalu9Mb0L7WTrLT7K+K7ShS6GhK7S+6ynTb6397DeGN19tS2jqN4WOswpAwzGGHJhQK5tUHmdcxxdAHXgxy2PKTIfKzLQINb1ijHBpzTnnsrEiONxGy2J217i6Pve60JPMuEIdeDU3TJe6FCJ1KZHck2A5QuamUFsaj1fAcni1A1CXmugFvKF0lICKDxJzCMb0Ct6RVBQHyw4Rs/0hwdcxwRo6wvhkWgCfLZ+vU5IldIDcdEqIRKICPe/o0UyYhIoOEHMItvSCE0dSUALce5iY7QcJPg5JNtAeJiXRgvjsCP628XbQGSalk4NJLy00tPdEmioD0SvicoZOzeR0StO1S40wGzW18yVRB+43NHC0ZmPrJM5GSf1sPty0VOhfNb7/IToJn9ykHiJGdcy9cD6MHWk2uDOE6RwqZ5QwU1s7CPUyVG5g98m8DH9yDJ+Vb5CNH8wvBoJF0TvlvawvhQAlQoCAY1fU29LCTTgIjQSrJgZkbKbqTXZsHSNdI+MOUYjRYM1QL0V708s0AduLuX/fabuqCx+AXbiV+J0hzjLWbfGt1ykbkQ/FEYjyv1WKVPJXytiRGGYRZ5tJD3dLGNjirt+i8lPB1ghkLg7xxx51fpHq/GKQP3bA4WYqYDKNtRIKBAuEK5MEq6QugPXxbVbwbaBsLiMlpXJTlq1gBt8DamaClpBiPJjhT8J6ki1fonJCpLyYkjJlxwMcD/H+4F9Cc6LxFqixg6bClHMTEHtA6MHNYXJft2+gp+J9+PalN0VgDYTrH+TfKaux04B+Kt1MvLVGIPn3KWHQkUL3T465Os3uZmQW9OWicjRzdkN7AhC+TPs+Dk+hq/eYA/wTi8v66otA9OSGBNh0q4NeRCsobstFpXCkT6Q0EQieIL84D0+gqSeMI8TIxmOFGtGbY06SUhgjrLeT6BxaWKGd5FKK2o+VowY0NUSrpeRUNdWRDLMAUK+5+4QV/NgwNmVXbAOfsfrJv8VqQY8RCWkYOIHVt8evTLFifArech2a4Qk4jmNN8j/DM68jHpp+diIeVpR35qImNHqfcZzWUn65GDWj/Aigf/oiJUzqPc5sq41gE+8JSoB96dYPqbnEEIcRQ7O9NWNoADf7jH6c6CU1YZDU2W9iGJoMn5RJBFM51ATNy/nJAdAXtoYGnqHGM7uL58Hu5Eh1wch8tUkraUZINzAWMCYnN/Jy1cNVIs/wJdDPi726gS6gx/fpx8nZB8dqNTyU3Q7yhVJPEhZH0GF07Q6tL4CW23mwELXAe2foZfT89t4S2ITuDxHULShJZb0pqBReOkwroCSXtaXAQjRxAGh96rH2nQwLQ/GwL8/qESWPXl3y6W5hLjyMJl7h57FYybM7OIVZ23GqFM7Wr0hDUmoiHl0v00+jpakeLh7JRv6wMyJsnBJLDlbjJYWC2+Wx2wVTX2Yy/bTyMoQLwU51AwV2uIInbjs7fDtQN27MUhJI/pLO9NeuyRTKB0411Gi/g5P7HXzVQn2ocaFVESgBdhZvSEsAwgOvpwZQMzo0Re9hnb9wIM433Feye8xW+p8ZHGVLiQOpi8BmXsAFxgXjPRyimt0cMS8sPAefST7SlPV2uhLawCTt3GIDPhuchb7H5XDU1eevSTiPP8dlf6o4gX1ADQL/vuP58ecytnxs3g8G0UFHIcdG97twBGwYbDS6+bzjrIRTzNEZ7Aom1FWjOXMvO/z+2BcNnJ9xjBHmjTtUtzk2j++iI+rAPVGXXgM6N5uzHzQVqWryklkNvLRaoE5CgsCScZfXAuZxt68wDpPjqw6kb+dsTDxLTKFfElh8/vECx9kHdN4bYNZSsyuailEQZydog5dbaLkifzRJSgCYv7iqtqD9bwF/Ga1QiYuYLclG9Jjk9HlNSls3dgO9XkdJKji2f6wdTeKEVLZRJaaQB0y2uFYTHoIdxb8+r3XBjpciHRz8fiRGRWiYlaqotDdeAks2p9U/R6Dld3n/PzVDLC1VqGvyQjsCBuzz9RzHHyxOwuldRbPDPvnJNYAdlw/Ucvenz1HMm+1zoGY92M07aukrEHwQm4G/WZRFnCPZVmRASdU7CsNNoQ/L+IlUQtPBgy3oOk5UeStJuI1kCE2xyXMr3RDgSvONUjJYnK8gMCPtnNwDpNemx1fKcT8TPP3uGQP6X3kZ5M/rykL5N4UuoCsLrLF0ek3B/mJUhqYuifYA8vz7WQ80pDxiInH1Zrhm39VKyUWZMnCXc57F0Vz3mQEkzXjsBkypCBvovaKYbG6y0p6vvuyl9VdRdKqEXkAYd9OeBVr9QsaIGDn4xdvyRRow5L+O1tikZWRPtuMvVVAZGjsxQcAVYdOZsWu4fZgLxVGoRWbuojqtWeS2YTYwTLeeao6IwqZwG7Yq/JGdBQEzvMVudAcnZLAZB7Y5hDoofLs8BNfxT5eYo/zXpV+99gkLBEefH2O3Muo6D9WiGAA0VdTur3ru/rheCtgVgqmsmHf4GuRIthasl5i+tIvV1Y9dRx9YxFc2PI0bDlt1svdkA6MM22vNnEjsGk5EYfs/mVnvyaHVXnQbJ6y7hQwmfdrCfZz7SA+BR29fssfoNRdvtyt/D6XlJ17lgNZHp5jVzPKOgy3IDQC9vGihC8/dRkwfiKUKvCUm1hxb8y3CcrGZuOAr5PQMWt3g8SbUARfqGbnU0Nrq05qvLE/NUarJKTW8zz7lWBl4FhtzmZyTTm8aO2GPltqAvZn5Iv20eTr4A7DEDjMCjgM8ct1D2WVd0gKqwGYzvJ8zy8h1j9PIBU8EDgzMd+P34z9OSh0jX3kHhE9KPttq+qdMeRMvmd3AywBNhYR7rnckChVjiIXazDnHF6Kmsrlyy7xk4pdUcpF22cb+xGRiCC8dbJW1ehfSbH478nGZVtp6v8nzVcBUGXBrki0QqZfnr/WRSPeXF1wsKgWNnBA25zcz0GhiSZ4ddoXhA7aEis6ydr+bZYaY8LudNQFUF14M/1XFWEB2zTWdp02ZIaT/D9H13RyQNqPv1EjtuufZey8aPA/2ZAV7Aqk7q8HHhIvBDTNBopWVw5hXLCyBhw4Qc/gi8ts/ujY+AawMSkxKURJKhkOpgoHJ/JW/TxyGg+jUOLPVYyOf66Z+sSd7otF0nBFn69oIGF7OpaQQ3WLigUGviAzElEdw4bDbQ95Gui6OOQy4cYFlw7fxrWj/U+wKI4ILCb5Yb8pxLnqEMl44vhb3rwgLxuqx6Xdg3j4ZnSqTSI6X6P430YvyseOldp9pzXqUMLvJguZh9wRsAF25jx+IAzCzqCURZaBLTX6CWBvWXXoE+GwUOlhOmMnJwvxgxx5Fl2hfLnMYxIpWVRycxzeiQy/RqER4GuAzhtaohNsejWKw/ZG6Hq5/xf0LY0KxJtzMJ/H74BF/HrxU4f6JWiFNa2igL+/Lx1LhYI71Iq2Uz+L9FnqUwdWKpEDoh7XYGxqb6Vj4TPuDoH95uLlx9WOJQIlVuXcW5JFPPhhiBRwVqBXpHDtaBWssWAKB2erB+XnMRHbOb1bAT/LJB0Cu3t4f63JRdJNri6UEb2HvLboxH5ODQqC3io+qjrulWWjrAKpDV89v6N6QVdU/g29CBxbQOP5Egc/LoUVzvDfWl3IiCQWhoyE7g/b8EPUvjONgzbjpr4C/rqDtv9uoDVXeFDiTPMpNHnfXYB7n4cLg6VzTvJxlLnMYcMHJVModvRQXHZyhAzMUM29wcnOgAKF+PerSBf7Gg32FqNuInxYYIz2E5O5Rl4aldZgfzOAoW5rGlBoC5ZJdxbubUpjDIIXc919G/L2GLv7ZpKhCcDKVOQxSgWCSnvSxmqknKB42RrMCLIMnYhJhC1Y/QT0qxB1LSb7sacrPO42lwdneDWkZ11NSrovzvyIkwMk66eiclRTKhB4QnNCTPWsedDEiEvoD3f90WG1mtbfhVvfuxYrgxX2u32gNbIl1peA27jT2EA/vbU2hcndMF8UHYZHwRHziDWaWFGus9Ust1oVbBRvugM8C3rDfSs0+aAuXafYoRqy54sRtfBM6+BCbokfoiwyV/p3M/GqG98W60k6noDB4KEIvxJ240avMzx+rxk2/lJkBvlIyEKd8BecLOxyUnWVq45hmj1JCNpV1Xcc3oUPP0GWJiFRA8MH6uKe5KBAdCtjp7ckv6VUYHoS14a79FrsBFNTvcuISLpVtjMh55doGymTZw3p+g0FBUPY9eLiBUXrG4LDaO3vqeULxmwM9sBNODhFquHj/wrRomNAWQXKj5x5Rz5Q1FnqxhzrKlzc/2E/KjYLHHHxq8L5cEFqanApT90cBCUF7ZYGHzICHKTclooOwBSM+XED3eBj020fdy52/zAoYUxEAv/fm9rzEN2MN9wRGEk9xE864mBK8/IKcsRTc9VSJAGIQM5UyoZcEmg6IL9ZkywroBdAF9LjA5YfLZm+Rk7qhhJP6+JCtoUJBejmSS3qhfImelKgNix6hfBp6sQAsFvqeqr8gIipV+GIqfqp2ToFOLQRNx8Q/lxVtM121qOlNC4KOHZSA4f0E/1nrRpqAfBaoDR5kBe9g+KjKJoewQteLNXhc06BWtsY+KPlyjw9/bSeZErpM3PRF+pumtxuHZb+u5dWk/5e9NeKYtIvSrLBD8mWWJXX8kBHYSwthroyEgL2myH2b2sZV2XtTdhfMYgjWO2wllqLkcX6eJU4bN9rUqO99yz8ksbTHm7+ug4/MeUuSQZIrmp5uocA2/w+79fhILuJi8SEeLtYmNsewrascyG7nnc2DiVisv6+HlaktdIJJKQJJYPh4LAW3IUM4OYRJZ0qNLKENVSNg0T4FnSyV4saLVT260MmWf5m6fcWfNZEUwjrL4oV6vrnZKbDtUar8kwqRxv/w9XEAZRS0JqIDuMdkoTXMvrOQB6oL5ajCx9wC7kAWZ4YV6gXitkk8kxMcZnFOym0Q/e/3p1OoD526xzyYTk1PaOeiLGzojOCAHL8diwN2/V8w2noLBcOz1qaWJzssvIbbHE6ITkkuDpwqPOLtmH/gUuUU8ocndpuaThyx9Jk46HJObFr0Xgcvb7+Po/anRIrX5pO++5q8grFC9Hlfz3d2OTPH9d3p0jSUhz4UHfwF2E+f3azCryky3L1kr3sz3Dx1TDJ0TNwZbm6y190Z7urrawUA0ohBKJWYiMgF0ykFX1OAcc8Z5tX1RW0Q4xwxvrh/2a0AuwFfrzAnuZBed+BUO6zD3a9kpJHcOOmZ8j0a9pIwin+ZefWba3kw8zQxtnRwya0Am0Z3X7PHuLTqlpP7UCe8UUZPExyctHlj36lTfmC2r0D+9WaR81Tv2DxLznIaa18CxZ3imVbYpgqY+KQTiKnZkkzT2UPSpxF/mySAzCwwveXHbjo5WI4qUf1jWh+fLf+F2ccNsATtGyTvC2Q9GUPhsKafWUwKoOb69/tjk4RtK6oqCoIP+AWn4GXk+Xq0UmCGUVmycCe9AKvC6Dl3vgWrmpAFJ14G+s1NoBNQbKLcV2hEZO5NsNfERXQGCU+U+4HqET+lwMhcTmoy3gsXfy67uwRUqu2iRUZh1jDGhG3NwdOjjmZlwySU2khOU9Gle5Pcte3jfcMkDmSByBwHPgsrMwOglZefoirWzQ4XAnk3Rf5b9vGeHkc8KH55n5/QKKuxUvT5HWZsJab5QxkhIXifX2gK7sOoWXd/hCozWmmlxS8fZBZg+zBGzs1PYcrX1LTcA4km5p9BIa4knRBLKmizdtpGDKCRrIHkxkATfry5NcFKy8YGHfU6BBxh0h6iJX/jJdAJgKbmLm+Gvxdg1I+j8Pa2YOgF0ytRwERubsJ+H0UfPx9LV30TH1+8ibep107DAF+wxcQgkxmSYXo6xvR0LSOkxvR0LEngsyIjWBHUNivQ7BMMowNITjkWpjsdQJA705rDSNxfkgxjYW4KwZ+QUJ+7/HGyHwjWO9m4YxxNbx87UyNjEGzCMuQAemhvShxdKUl6OyT3mORvxxfiIo7mZsJkWJWJOBwlF3tbpwgHgjE1l4dzAEDsG2gEFMFYAISC9cLS8PbKxNfDhDQ0likRTTRBvNV3lGhL6vHr6BjOItWHxChvdaDlyecEbsOFv4IzQvdU8M8XAeMuDxyfZW+U2nSDX0ZJSuUGKBA0qtiA34sLOBBZjQE7PyzBKI1oF8riKGSBuebwhLr3pkwf8zAWkGuYLEZ1Y9Q6UiC7ZWK8Cs3eviPkeqIbs3dmgjtOifEo8oB+qLicnkb2JEV7FLtjQbBwHzUJbDtGj3Sq3V9nUlnEqKNyoyuiYDTq2CualQREDi5ODqIedHiO0cYaHRkqTbGzFS4fs1sGo0bZUv9oM+dAmBQQUyvcIVPYbGbGs2GF9JhL7WCF6Nj7HLKTrvlBuK0GmGdao5k+rlY0n1JvE5dSb/FoF0uxyJYIsFPgOEr0yemM4z0MbsOeA3/zvvsmHWaJ6nmYHzxubLS7eH/s6DvlKFIyictMENwndXdEj97eCfdokIb1s1SD3ntnnUT/4cR0tpHFTunkJbkLu6j0LbjZVOMKsIc5LUTdHLwjEJvkaSirgHTsYojnGXISJadsZDm0fBfQMUlh3OWp1EfFq4GOpzzmPkZR8/4SBLpTqAIcsamHT/tx5ahngJyzU9I55qyYhWeaTlRdrWGKPbH+/WgvcuiV11YMDLMvgknwSCE1LFI3pr5qVyre0FDW1bdFbo8DycknyQUl41r6hNrB7gX+NHghUt7XIXw1NB+rg32DxL2UPnjmxj9AcaflBdVEonTIyxSKTaDw138/eRzHhZ3xAn7995+iU+gsEJmhBz2xJdvwWVEyqmu52AHUk7rZvbFwZy7HFZ5y3G2lofABVE+XUxKJvvHhu9RbcrCrw7pnOTTvSrp/ejTQxQuW3EfiGFdFveS7v2m8QxlKR6ez3R5Tcmg1xW8e5hciN9hlrrkbvc84+blsi92TzGjOmIlK8EOLqpJbqVvszt/a6FzrEQA70L1nAmdZLY0PS1EDbg4n/phoAowSCq8LJF7/bs7eKKWyICujJLWZV/Tr/h2taAafgS1JDtHISzGiKwiUzcZn4mew2cqdfKc6sQDgtS2yOwiUXE9LI8zjr1RaEM9JHMR6rQOkgsR3b7MD6dh0Yzx1bifWaMKRMhEx3GgFz6KfRuC7gDfsW509rBRB7e6UWK/yY/P4NrT/ORzf5blRlpDbnNvEM78Yn3o6VSVv9JwcNuKufwTHN7kbRABHBSi8ZxvXo/kOT6Zgl7K8grCjyWtoD5+xtVlhn33lGtic+lDHH7u7BYOwfTTal8/JDO4oJDHl3iluIrsSXejVfQfBllN2JehKrUzqnqKnFRlwGH8OCU/QQ14yAojWtKzSpmh0Hl+fLu9YU/4OZcCpalmuU+FqWTEqRMMVjt8Z1SxJqKT7+DjWVHUqC+Wj/w6EqTHbGUUVLfEoFV2pSRS0hf252Myo1pEoO1ZF4TkgnKRHTaoTPjQ8XAodTwgEgY/OMClTIIuaVA/UJr5SIkHuqfGT0YBG+2sJGJOR0ImbaG8dGgGtoX1SopN1SKidncEuJRVL0+BdWoaKytaWQPvuHC0oLsF5o1yAqZakkrHBfXeM5pfAdYKbbxoq3NzkLZsGBE1Xgw4JFwPGCmgCLME4sFHjFn/GcjaTYEuwSKPcpltnY3tdkRVmErzHlTQEVOdfSa3vDhD1o3wJiLjym2qGpYqmC+/lMqMkRgFdw3K3dnjGhkzhomRWvOQVIPbKWCuUK5ohUsZlpUidAxL/G2vFFgqXb6jnCtVsnLArttYJSxctEetO2dAvdQb0r+KdIkIdbDx9HXF4h7BARxsvPxvwQG4yy28hje2VkQAYt3nPs/j7WfaUThQA7TTzf8vXhyYqQNVYvUGa4kZ2ZBT2txRj/jBDGkRwJSJgI7hd44ETaVUbBQz3c+J9F5iep3B8mjR9+U8wBGZLCkeGyYTC89i3RY03Ym04pkeDRF8zMP269XaVlPWA+YClp4i7/cHFgz5eIhZttzvsblva3m7iM80GBr1DiuwQRZBEPvngGDPgGCNV9NDtc30wFdbpSYZwNRNgGqgpYiewUr8xA75iXXWY3qFeck0k/yN9Tn4uM2mXKFIPkr9gdpFg9+jZVugO3fnPbGPiHyTtMkcZw48b+zgE55Vxkb9QrO/O6S6YDBv1N4aka8e1dtE2zYj6fsi+fcC4AF/koTZYMqdkFwoNjJ6q37OGfbRCc1DC/b7ELhLsGT/TCJ1yxFpkmfgHqYyGqlopd5MvzQz4PfGkwKjYSf/zjXcvw5Ubhv9/1HuobbnZq9gqd7CxobqrDGZfZ9dt183R8PPW9zRU8rK55jlzaMbqJA6HA/Kdd2ePFLsXejHkCoUp6Vj6MK2JmsI+evBMPvbTQ6U307LYBwaG/fUEWfmJOGcYkUNLZR8hIUszl3knjKGMaxosn90g/HfhGjuT4ZYhdT5c+nweMnwkkngbsixyA4FrVULhJNMzwmrRTNY5OmcvPhKfdbAxE0XA4nQYFAwwI2tTI+AAI4L5XPGW9q6WwB7aqwFZZ0mye7U49BHx8kqEHMitwMfgUw71JKNUWLqflEMwFZlD72anInjBei/tCS4OSbbWkTF4O7yVtTdovP/sfvKTC3cY7sYgxI6QUm8WbuQliYs4kp0N07HyRJwfae9BM442isKH96bHwci9CXVzNp5Wu+1jnQlGdm7mlg4xTiqHzO3Nd5kF2vqZ25gamQXZgkVXN7cETno1PhafwRtMgekw/Qilwpx948fyfw7kPaJ0fMC/mwEGi2p8/tZxVnZRXLw93touAJC+BlAtPDzM5NP2bLOF4l4mDKqZl6fZtjTXbbaYtI8vKfvOgwBgbBapbhaZrbZs9ZodEgQ4XN4xVsixxOaT4Jup4smHWIgyRXKfC3hpOwFlsX8CIB05Va02WzRpuLCeQHYN6sDj74P9pZ33kzr4hOBAmQN+cBKnmzPYXwzSX9ULfUQb74qmpazJeZxo70y/CdXWHl5iwMd53d2CRv/7/EPC4R/YfG8IQNLhpAMj346AsjoKkI49vgCt1Xnt7vX5jf008T2qXjHul2Q1uiDR/OIcrlkuyEW5JJflCq5+ECj4L3hahXO0ATDJ2oS7PF8qSY53U9amcX+xcij9tzisL3HK+jAqssTc2HqjdlpllCG2tGfXrXzHtmGs9MooDutZnPJ6d6wvUIxjeReHquJU2nbHZpBqbuEF9b9Y5ZU54rA3xalSGUalXw5urDOH7jBW3NKPwxaNU7Vpd2yp0CCy3i1NmP3FPAEQh5Ubp5QPI41IbixAkbjy4cUcrrQcgwmxvA6T84CVj5TW2M9Rfhj74ksInyuTftROyWHGqJxwrBaRzqMxLc6I8s72mVL2Sn4RDfn0MMI98VhN1w5TirJuVNOnnYaSh1t7jVtmpWLlSvNm2ObcNImurAI2idxfqLRDMSVCB00TqtSr3bfZjMVJMwFqwW41eNfgiWjbK6boJjNrAL9RqZRpxCaRI8lOuwfx1V0A8kbhkmW/KkboahZoalo5vvS4LLMO/VGsHY0rL1TVLfr82lCIaYAG7aZKtPi49lFApjMC29tDVQNbshNHTGDTbOucpTrTVjc29LfM83LPoJEkuK1dq5FpiVEorBQyula+KMrMmTj6kS/Q6jzg+XOhob9lpZdvhpkQZmlvXYZwsHVbHdmrP84D0guZReeP9LdsZgVnfuBG3fYiDfHAZup2Gz6+eCBEDLQMXnx0PkFioGPlJD7cjgL6tnZ6n5fXFKuaF0A+5E8KZF8ceDpHq4i73E97xPa3sOVWaoM1YqjGB3tWo0Ce0IbNGofM3Zol7CxuheU0iIXlCxWANbGxyEJlDzpZFNpj1lSGpb91TgvOoMa8RcXqrOgwV4h41WoXOhYc8GphvogTAqDevrb5ZBg1HNn5xbza46+Zb6Jlxd109aBMdGqLtXBEN00t8kt0tATw7mE9rHTL8fwpN+iNIh9p957PL+e6v3UOImcFsl4/7MviBSm/M0yL+GLHSvlasK0LsBzfgiMbpM//VS4+dcvtqf8ZoEi+VcTo5sj5UH6jK0L9Cl0dJPUuucXzDPD59pB5wLdtuG/dVuxLD/LzMY/2LYd6aKqXgKpQ9uWn6twE9MjFr3csu/sCtMy39VOniG7pZ3tIQ5f0yr444ub8o9q6dGGgppye2mcMX5XFymR2mZV/weGu6nsKgvNqLazL08nqKO3ps3zMKmX06AIyATT7izI/92mK7dDsPp3jgOt6698k1TErb6Gq3jdWvrkEmcd4Xu4ploA7exshpJ1LZBggfwEYbr6zhwDYT1CbGf3wW0hqXs9YylxCBiGZ00Znbl5t0Vs/pLpyjBOAL31r2pQ368wFbvgexZD9bePh34+rlvZUc2/azI/xmh1HxnE51y8gQ/c3NruCFLNb9hcZbhaPGbbf/BLS9gVKgMvmvzDJq8EF3Ib736Evp+Wh8KwMI2C4/73bhzlwdq93F9CEM3f+8nZlnldr9rEOA2ypWxwDzM19gyVg024R+oB5dJem7Oi7i9u3Qomku6TimqxKpttvc8J5t3oMCCEXma9ZW6GHSt6iy0+S9gHpWt1GRPL6irwAFTK0U+4cBkka5l4H40C6diGrk/2PRRZzSma4PG6MccUSvBv9Dsktdo9lHQX3SrATbZWDKT3o8TM9TptnU2taZ8aRRsGbop2MfG5p1hbGZ2ZdNyCGpymSVEnc/70fCTtvQVmXLlcY6TNbjzYv38+1Lu5JyWc6tq8hn4mk6wJxcar2dOQzTVZx4XYVwzUUwZumHSKfOWLtIAfO9vXOCB86JJLVgczwfmyz57JTVpfVkWxMTDPn2Tz5eTWBoOy89WtfZGvNbLKn1eXLEO1fenaWR35fU5bX8/t2xT42Qw2YPqSEizoVz+haVPHNbVzO9Hgq299WSuiiJmOq3ZRRf96GsSgkNMUih17kVR3Lz1ZRI1CuVrYVP36cTAcFJ8chRf50Gj0/uY2yfPzX+/3Nbis2Z+0t8vE8m6x3rHgmpn1PqZdXl30yY5svY9xaU6dv+7Tv8qZBwNe9xcUD9tse5XNL3jwiQnj0jCu3NarbwX4/+lmTZyqetxY6B9Om2xDBFxlT6sn8J5Mhv7zjD+KGRwDAvZ9ZLxIA78vpx1/6D3cf1XokEUYGDAAC6cYfKiCDc5QTem0gZx3TyfjVKFgTP04v5RNaberOVFIZNzb7qpGZZ1DeRrpATNekcLlImEg2VZwjbUyxliitjWlb2RHIRVVsxrpYDOfjCHHq4qmuq8MkqjJHBqPduIgbBnVXtSO3FKtZzPG5znILyNwbWU4SjDNu4a5sl/TSYoLGnuh8uCLp+DVusIpst5I6N6tPS9J8t3v6KM4RJ7ecjLlt8FoH43Mtt+eYoRnKWq2g2wImayVxy5TPCkpxG/mJU7Np3CgOieR4chkqLbtd9rhjjw2lmqJfN867dV/ZwfXW1rEte4KYjFuWx5drD1JaPyh1Cbe86/A1KX4vqVvzmV3nc0A7fdut9GqM7N/ypG5P3BrL7zHlO2n5uvejuozUr3Mwdy2rK8A/wk5xK831VSdizBRcJiKXp+/YT19xm0n0A5x+gNu6Ho133q3zSuuTY/U+p/uBN6vEzNpHch/0bK+av5j6VmuWb7H0jSjWtrJt+llMo21XK5GpFD+JbMabwYTmdU3Qva243ppMa/4sL/+im6l+oQhzwH+dwP8iwH/ywP80wL8v1joAsg8QAwA+v4MXHxsq0tmK1F7+6Wlv59pfb1umqyv0bxwt24Nfe6DZZfm/H4gYN5Zzx0dciqOjKW5MZV/cU48ApK/Du+/nSyhSsAxDI/6Ot2I5ZgIm7VwH+E+3H2jAEbgNN+FhnPmd0+FxF/zSqadnOfTHWpaw4s0uB9qPbUCZ0tMTahdid1nRIbTWmMnJQCoaTwWdyOyE4w6hssbMVjgiQQDmnB41gbFcCAewVMi2WOgGsEyIRhJyADUWDvWphDLMJrFhUGB5LZAEhUtFSCmbw6suF2ke+Z6eYClxFmRwiXpDvUfKnhy2DHW37G1j4ZOAjWQrANhEtoEt9L3uV0tyz/wMF5NsJxtiTXPMR1vKpsimsi1SJcEk/Z+ayHcQZLxqQtzhEY57oP5Kz0gIcRmAgVaVJuQ33wyZudcsZXUS3b3DPpS6bUBp49zD0hMA70p6t4yjR1lKCZQEiDssyNl5CCWxEnCCKOGC5h3HSBQi8aHYsGTCjB1nZhSpUaLCSaxA4SSmaPrhOMOYpUSAFXM9OIGFm34Mqf7JVanTpq9hlWJtu91LFUs9LKgsWR8zya44aRJ0c5R2VCnmLPaqRgnZsaUYQZSYUeKoqFMvT9QgAwjBuPVbC6YEaeULiRDsAAAAAA==)format("woff2");unicode-range:U+0000-00FF,U+0131,U+0152-0153,U+02BB-02BC,U+02C6,U+02DA,U+02DC,U+0304,U+0308,U+0329,U+2000-206F,U+2074,U+20AC,U+2122,U+2191,U+2193,U+2212,U+2215,U+FEFF,U+FFFD}@font-face{font-family:"Roboto";font-style:italic;font-weight:400;src:url(data:font/woff2;base64,d09GMgABAAAAADq8AA4AAAAActQAADphAAEAAAAAAAAAAAAAAAAAAAAAAAAAAAAAGoEQG6NaHJB8BmAAg0oRDAqBlBT7ewuFWgABNgIkA4swBCAFgwoHIBvqYkUH4q4K3lwoYMd8cDsEqdK8HUUlJ0zxf53AjSHmG2gXJ8QRUYwLJaOwfjMKbMHI5TlRBEqxPHT24qe//nLn3YHSgivdXtrm+zNwnI+ay/ORf/98a3dXn/s+jGjAiGD2w4HScWpGADKG9Dt1PL+25v230Sy75IJIWIDQkoJ0S5eAVElkSVooIqAIRoKVZ55i39l1NWe6DlBWsu9UieiyEQTynyWBJZBAeEHY9PJEIGNDSCF4fhJnMR7EYMvcB8rYKcNqj3MvXYOXF6C2AwA1J6bcD4D7bOrL7YzIITR8AGiRZnfWuovPLCcKsE6axm59RZOwoSjDrydUdm8FH8z5vzUr25W+DPBfrLgFlBFuyR+qE5J8dVWnp6sqHejObGghycxC5zgzi9zHoE4tCHNyMwtI7pAUsrtT987IE/qsYlQrnDseHpdOX16ZX507j8SYWMnYnzWFrIatqEujEMYAz3/7pfbd4LwTFQcqk7CM01Fm/iy+xb8bACjhP1WuqkoSqE2FIlKyqkJXKFVfi9bU5jM3Bd4CqisaWyHPyQDvT4FAxdiLqyzPf38jLZDAQ8z0vLrthgs7HtgC/pfTwttJfH2Jv8VZrJobFeNvvlHzdK6jUIoUyUoQkSAhc5z/a7NcNaDiE2C9z8stY6uS76J6KBvofUdB4NjkM4XyKxlDLAVCsv4AKQSkGJAyQCoJUK32VDRpg+2yB+G0Bcj5SEFZnvVQLBMH9QJwLj8ZF8vS5t4ITRNqRi2odfsQcCJ1xkh3v6eDBwielpUcf+JnJUfE4eeHpCZMhgRYXTM97xnJCUADkoPU72MaJ2ViIJYLEjj61mNHmoSt9kx9+3F459UZWvrlYfTTXN90/z+K2bXrgfsZ9KeTYvCLMVPNuHWyBlWseA709k7R90frHWRm98Wz3VJ3iOHXeyfmgT/jhjrb8prfy9f9UJP3c7uR8XXFdM0f5Wv+oGu2XLCd77Tz71Zwu/8Xs+OzMFEijPO29i29PErukTzO3blrtzOe/XmVUcJ4vRM2bWyd+x/hjl34UXBV6N48Sb+3vTV7U58DwOm43ZGTY3b3EepVLvrbDm4MXvQQ5svNKGNgF3RRQ0upSWoYsaX7cmpftbnuj7t4Xu0XPYT3des538LznX6TLi2FwVN0el4JgUWqi1izf7G+9Ra9tvXIIwzX8efIycHfaAdU7R+t6s117Wr3qQjV2exRkYu1PbGgeiZ6idvFyHUoDOfU6i695pNsLCF1fz5n1guS1xNyr4bP0nnrr7aLt5eDvuts7cUdFFF+AE4ChAsEXhpbGewYyVra6PQY47FkTZItIM0heBlXHmR4gTzfYAX+Aq1QFbxar0FrDH9NqTrvPTVf/OQZQAV+oSAKBIeG6BASJmJCWDiIA+HhRlhExCImKhKREB1BlMVEJSpyohYtuVmXdQqjm/WKYh17FXGMs9q4xlVD3OOuMZ7x05SABOhMcIJ1JTRRuhOTWFsRYsVXhVXhtAhhpFgKSIQAFKuBoAwEVRCnBYR1QNAFDj1g0Ac6AxBlCHzrQYgRMBkDl2UwlTVbiH0w4soN5g4kHoB5ArIRWHyAzBdo/IEnAGgCga0YCDVAqN0Q0q2HmF59hPUvV8SArQ0MGqTjSIs4p2AxF1xFce2pSbhvCeGRp+ieeYbpuVeoXnsP+eIL7KufSH75CyuAArQQiAAiGGFAoSM64LAQCzhhIzawBpqiRwgM5HAxboQxdkQcxIhVPuKvX4AZqUihRToyhCMLhiOHUSKPUaOAUbICo2YVhrIGQ1HCUNQwIuoYKRoYEU2MFC2MHm0HuTakAZ3omLrCmNHDaNHXAIMYgGEMYX3WmzZgOA4YiheG443h+GBEAjFOghysgikMAkZPGCaacIyWCM0QmUgzCoyfGIyb2CBshCQUEMcEcVyQwpNS9UsTViElS5gWtgZAFIFMFRjUgEkdxGgBoi1ECok8sDEISOBiEXqhsGKksh5F2Y40DkkqJxKc1FnHb+mMnpy5T2r9iJMiTmq7lPPOQ4jXwq77B7mEEAbWQNnZr0W98R6tikiJCZ1wii6UFKMkAT8Z9SOUnOKRIIGFThwJgYLYYWtlViLPaVmd1c24JmtGxSjuVRENgiE4GSVMBmOhY6FjoT+TYvtNJGM3ocZ+guI0IcX5DnPZYaZYRZGQnlF8NRGJ3x3qEsRPdlExCNAJ0EkQSs4CYOR+EvDKTWzj+gOuO3f9Tt+Bm7xt27Q1W3aTm1vGxZ1v5t6cnYapmuDpO15sGY3IjI5OH0nDl0vqGzfPzGzyKXI0rW9D3+Q80zC+biSFSXvLrPenas72DW9QvB6YvratFHc9k1FZQk5uV02AaBtDtEfHdQhyu195dqog6UgomFj4VlptDUVK1KjTom2dUEfPwNDIeIOtnYPz3Dzb3cvb1y8gaCbiLPwbj5wPMeVSWx/TFvvc9/Hb+8fnV6r7GUBBBKIgOmIibsQiEUGkIp1lkYls5KKQlVmV1VkTxShFLerRiGZ0oxf9GMQw62MT+zjFOa5xj1e84xPfBCQ4YQlPRCITlRg8DmQ5G5cnu3fNxu7hh+lrY9/gOz/kZ1rsIC11aWmeOZGNi3CzoOGNDKqt04dHPOaJPNXioMU6CZ2DjNstPPoZZDiL2GGjcX/tK3zjOz/kp5a6aLbg+FYh3jT5vX2bNdhga/bGk1pe84a3vJP3WphVrEFJHOaQRsHFfDKbCFM81i2zWdhij+dZCL7aYr2yPjHAVnsNb3jLu/J7tLCXxs0yZGxVa2tASRxugfT1RGoAAAAAwBsAeDZA/FrAwehHTkNUveQ2a9cqr77jXaLLT7epp+X2scIeUNbHVPZRQ30NCZsQUyxerQnBkRFBuMoanNGQ+lb3/pb49a5N+wG5HVTYjsq6TmVvxaTXswzaYNRnnh1aAnTRx7AcHLkKSyuCFqEJ12c8w9ZngoJmlFjrJZHMQSAQiGv4gEWNHiR44yjs/gSqk/vsOkvc9uL5YiuboLp/o0eEZZOsW2OzsMUedzyf7NUin1b5tixgWe3lrL/FDMKwv9CEi1ziMle46p836QX/8T8veeVft+YNb3nHe77yje/84Kf/1arf/JG/swr+vihUxkGrhFqksnJNlHyTpVBYYatkaw6UUEfTtAemg9druQxYbw4+CyaS8KYcqQ+EYNfAr1VIYT+AsfsZ034R0iZMoTd5V/PzOyL8DmAzIMIQjxExz7xBCi+xFxCLLvoY+jAiidBXjMKDVsNUCHp9V8GEft7pfjLe6qA66B8sm4x6l8dayoL/3dw83qZ7upliiwc4OwRUH2jqBQ91dmx6uJu99P8hyHfkIBbxJTR0jLMhT/BSb/xHCC8AlWTjZybrcG7wS2c+7WmbkqDm6QmJm6Dsv4+aAXKfHxMVAul7NbZ86PMT0jaB//MTw+LB659CMri8WLbtXk6XWQAxlxSoLBUjGvXOyUUtXZBhOZ3oTYgpx/XhsbfTz7YaroaKjFlYcNY2LYIZk4asP0EELwyHZEuR6rAjjjrmuBM6pL1nU/aZNG3GfgfMOmgO5SKLIVvJu5Ew4H1FRCwDBsKYMeDj5qAnGlS2iF5+vxk5SDGNGYGpJB3uetIpXVYGS16VFFFixEmQ1MIyIpIldLboTIxinA0xsbyfmMfChrTz6GyLN7SdeWbuWaexDCSyTCw4y3y8ZF3seRPxuiiHlEa7ujraTQQImicBFXk6LPag+mhUasVWcHnV2pIviY9EO35g2iZ0ek+rqT2VxpfxpM/2QNd1+HruugyrIT14g4b+5FXdqcUardwkJzhOsYggNHjjpEnb9GlRJRqJNDFcjJCB0JK1CK0+IDT5iNAMIUX0WMmvFFF5LGx1YrNtEZanJ1tBhgTeB1HWw2dQEyknenoVBH4DakWL+DlBoltJ6wy3GCKX20Co6IRfRfvJb4dDyEqboJb9lAHyFbOl6+c+/Cia4WtRD9+OMwxf0kZXvP+G3o1XKyvzqKncrlwcpSrtHdec+lbpUiprwpuy6slbszWHK3zC7jfC/1Z4Tr594VNMn+uEYdGPCOguPxswMVG1hDARPynWWkWWoPEunCfrPKJVHr9LKKwlVBs8aoE1i/8ag+9ix/CQhvvU/MtnYejusbaxpvGsl3OvKVz7zpkiFeVwi8rV7RBXjmPtW1pQtNp1K9durNlfESd921K4TMEFcisXU+jHvP8CN1FBpkYbjQ5DHEaMibBkS4w9R1Kcf9Kb+1khgFT6s8haKlTz2UiNbKQehfXJsdzTiYMgz6qdoooCTOut0PQGwbPMWpdGUFRPh1Cq54Sk/TxEdG3SunuII1yJaUAG6+2nBXLZoW3+UORrqbE9QreAZwH3Ay4H3OtBztaAQP9+4A945qGrQRZ6D6DutCbIkoX/EZr87FOiGyBlpv1DmSDj6Z5pbPkG7DLuiNMueeatv0gj7mO+T/edvjcIY0zHCpcTsifIniR7tqxAVkZWXnaVrJasgayFbKrsrht5ed6fv//H+ccYyaKt5XY7asFlz71blW5DQ9+zZCVkpc9ZU1ZfyQnuL+Ep9QaZXgdOBjbQvknsod+Z4+Vv+mv6Gr+aL+XXSv33OaiglBIKyef/h/GEoU0dU6+1Tt1rCgIe7LLq7qoe6Ex5tauPL8lLFzrv3hfkT69IsUcee+KpZ0qUeu6F//zvpVfKlH9xfohVqPTLb3/8RQCvDwKEQEP0X6cB8nzA+AXQ4NTvgIu+Ak54MdhwwyLH1QVHyDDkJQ6IELgTkSEzWFhaAPzEiCJEzLW/GmpaJAuK+fmRhEM/af5k9caAkLFXsYUiNd/U9t/Qhto4ZUYeJLLG5h3L2YvY6HbrRG/sSTD+8t5U/UGYIPhlOce7bCcuLJdaIw0sStL7i8flLF+nK7klDeiNf8GcirXX3tPdEokqupq8XXO0L6bqSusMjAZufOVN4WrmJStcYMBNzaUyMzzNpgOTu8TFCn93fZkm8CvkueRLp1LaLUhifUAjRYtsTKfcnRP78eHdBeH9blwhptxlFyw5AKHBVKI1HoOBnzjbM96wAVi18MHjvHrdySYdOsYshxgfomNplX+hA8cVwYcyCMaXX3x+43lm6pltiKFAKQOBOgGnYBoYuU8uQIO1BHEYjK1PfJrqlL1nRnKOuDddS9YtccpaOspxmjJap5wX0YjrIdfvvemVbxPMYp5THCoPodyDWlTgMWtCaSdTfMBzgRRoweKw44kvTD/FxKp32fNCCjNWDAQY+c5LVrb5j5pqKUrbgeeqba6Sfhl53dbsWi2yJCM8HKAZVUMDVDJacpQ1EGTjljq+uK14G+C7z+0z25j2CgSHTy2hpfPSrUuaq+K10wVjVSuGcgWQIT6C3UWu5usBJcnHsdRYQVTyq1xZSE/fi8VkSHVDF0dL0ncwyLNgnIveiaIC5Y1XrkV2taCrRscUlv4iBHaRbFnuTyUpd17mJs9H0hT/A6cSg+S5WwzTWtL31CErriar3jzfsEqhlNoxFU3bXB0CRr+i/xohNd3grHvXVQ7RcRaSIneHMI0c52MUc3mcOyXGQhZpIKjsmuGTq41ammrrFe+kTVShzdgj3wl8m9zFprEt2UFWFtLldlfaebmi3FIrM9W0x210zz/eGjz4Lm2S5A0I1uB0bhBlOHEUbRsMhsPJj4x8kpIsyjRa2bBrKWjrz9Im3XohqBZ7qVXo6bISq+Ql+9yWlXxYoKJ0U94UHAuuaD6pRrvTebIVfBNvmt9PXS8NslzYCJGMiV+kI8wpIjKWDK2VAlfJ8JRU8WQNRu2QPF4TUmtGEK+H9ebGi8e7FrIO2RPcEGSJNZukVyzcDEAL80oZy8FNFiHqpRVpCxpYOQl5Bi90ZlezTG/OCfs2wenny7zWPy03Apks3SS9lFJLRBkD8ZWWvGRjgDbeKHGiKYz1WG05EVm+jmWKZGuKv7M13a1cKnhyPxyYhOoBrO65OIToKHI3bWDYTk8CMVJWciUmmIqzfBBVOgYV3AeH4NYRSlg413UHo3taQbX9SkKa7d02AtmEnukcvFlga8o8A7Yz1xOVTcZb24/yG5GF+zUlMhrs8W2zVjiCajHCGJi3Vc8rW3D61M4Op23eu7BEy/wfTDXzepPpD231KQowroupVKLo+nczNnMcEsSqLQaIo3mhVubHEiShFSBa2OM6hQdH2OXSjeeZC89sw7+Q2Fp9R/O1TxOWYKvVZC7IcrOVxlzGsf0g6Qg5O69aYT9bXj/9m8Fh/ECbwNqWJbppRZgZmj5ihftyFUqzEtYzZYCnmS+UEmwcop42JemdkIL0RRnRM7ao+BXJtye8HgN6ocSjxqt1ji65KYeupxSVCs9sd2LJ93EOLKIhJo/8sRhheGG3mwx2XUcF2Qf6WamVr5Rxcj+UxqAyiOVwSVNf1/xpDUhSGxeoCEUxPqxoLtggCIwqazbo1MVdRNudGgBB8kEGSau6tFdLpiTXOrvbKcKQQ1jaXv+VQkq7sNBUSwETz60khIZlV7lCEKRUylJkjNquGZg2d5FZ4avQESqySmCAiq4kSdsyORJzxEVc7LBm9UwwRo6qmjSybkvGljmtVHASKUs0yBnWPohGMBaIUCwRhofllWUar+Cvrq4mgYJaGtHoYD7WrH94pdd7R7+JCv3PFULCbq9W6qBbw9hKxxdtCsiS73T9ItnmoqaBYlVbmPFgIqr+8zvmtju2Rytbmem5sHskYQZrtf1YSPRm3ZESbZLcAakNctP7xLBBPWWWRDvpsdt7t8Glvdw/iCcq7/sXFKXOREHs6L6L2fo5/Zcnj7nnh23WitxdzXPZ8+XGZ6ntobzWPygnKs1GXYzg9KFFOrNdOMWEp/5JxkBC2wtZijEzzWgDUoi+G8KbxuE4WvDf33iemX32zdUUP0Br2lNeq/ozrkBEPIoyXTlVO35Dv+h/ZSNaBqXRgXiZFIP82IAjsBNhoZ6LgvIEj5fV2DhNjB3CT8HbnxADGEJGR/kJp0QYMdk6fhwXeDFblqzjDcgAHZvoGNMR3UGQW/S4RHufkvAFjvcvZIX7fWczgm8k6hFe0QZ+sV4rextUu7x1tNeyc4WdC331rdIyhEzh9hiz1DoRvD084cQjeAfTcnw6zxNsI1z4wpK1JOfUhbRMP9+/tfMytKqyCzGPuPbk/V2ux6OgQTNeumP9N1Uk2lNtDpqY6m5OeLvYsaQwV2rFXglTndUm1jt1BKYehM7beCdPLxrI4cj1URD4iW/jpiWjNVFXGcV7TFzGrqSbXHjezPT7tgdPHnyS4N1Nbrn7rydchxLW1RjU+XPPHniC88bE4ohCrBYvs/M1mU9+FY6sy11sT7sqA1DaWUYZ2DYG5RJrUZK1Kk5aK5MqKcLZnZdUyZ5EDqlyYr4pyUwRDgQYwsTJishVjlKryo5zYvq5KCScBql/KYGA9R5MrAiyyO1hLHLnITWGXCYbb6RZXUIyq70DzeruKik8TiW1uF6bvvL14XRNO7BjuPACk+eZKbI2oyCDIWagDtel0DHCuc3sGe6SWr2MHBM9F7ebA0SJncAcIjZnKb+IwRtBkMbRDzHhb9XK8Yqzv5s5RPB0Yn9gk09hBU06bkSCghOrk1DJUrhqSisIEQC9kubwJXtQEYLLDbmrsawyTtMQpu6xi/AaJ17P+f7zOy7NdNvLOhyc66+AjtSt4f6r7nPPWhEdVQSO8Wg0i4+NwLDHgzb4hgvlSSBPnMQR/kCmChdZpr9lZjzeilFABQrAoN41zGgRPSwl6sXpeX9lTlXtYADeDhKtCW5+m8D5SzntTYNOJTh/nrYfjnf+aO1saOl8Jl7xd8vwN/EqXnt/Y0f/T1SazJOm+W/IvJVgvk/7am/Skv9REPIDNIXz6Z4xW34F261vzmSfYAzdvDkhtupO0+r7zdIN7zhH9lGbInvCiCTUVMRMoHn65wjH16abdFJCWTueXJnnyx1ulD86JD72H2N2mlGU2p2G8tGOEpC9cToT122jFpAdOKVT23NxJmrcSc0LYtXP7DLLJ7lYBL43R+HhDBeaI8XeNMIMWaCICIbLHXIOrt1BzSObsWqmdm/BGahpO7WAbM6q2jtulE9yNgs2RZYoNKK6ixPFrskKhf2scmZ9CrXWFYkOtQKrLZzZi42b7ZUcrIupSczhfxaHcBnas4OaT9t5rq4p5Fuub5lY11bBn86dPZ/O2iSNl1/G8+jpw20OpURrCmdsfDKHV8SJy6ul4ntUd73w3R2Rsc6M6CBXdwv3xEwQj6iyjy5w+LcJSuxkn01OY+1+J0FOT+rDzCwK+nkp1iDcd23iaMrEKeoKFMT0Ankb+Y/7J0Gp21Eq2QslG6g8RddJu87OqiYmOnYRNl54PxHM9AQJfFfEx7xeNEnTr81yo2ocr8/At4m3ZWOR3H3xzSGgNMSuktld6Xxuxvncx9OubbfNAE0+yi6lTqpGsm2vSJnCY/goc1fQdheGrsBvFPIrCN8JNTIBzdxhHYrKEPzD34/3/6K+RrY0a4UPajpsjXukWTzzhfI/YUezlv9f24apxnQvZWeCGen3EUo1adctuedKcuwUsCHRDlNKyLsurVgC/xl2+Pmicpk9UsdlYaZftEaCHhK8bZtwHWtTYlUELsJ7BnjVk8Aqrbr4KKGEmMLXr3LGuEfOXfPzvzZ/BARYMwNKpqpY6ayUxJJYtBn1VQinTAJ3H/fhPJrBl++xp2iR1dtzdENXh+yipgidlHi7iA/i+w+oE1NATsyo2Iy34O2dzAJGemFJAa4CWNf430lB2wkD1xkD1xKRdE38/LT6tYWJwnNn4M69A1dvCnYYJNZuMtmCptAxlvAl3gNxoct4HN24S57ZTsnIKo5CW/DuQ0Kd7LKs3WnYBiWp5YLh9KRQj8IClnrr+F95+TRORkPZDreYRfx7IjJnyFvxpaeco9Ro2UmL1LZKA/36YLQ9RcWHYoZc3DQ6Q5xNIZO06xC3i+6j2BrsBdYjxhppxqc11kl8E2+fB7MSI1p8xe40vYB1vlPUWM7RZS/O4Hl8d4mzm5HbebBE33Gd3RA1gH2A/PgwHsfnbgsXuzCTMto24xy0Z4Cdz0zJrE5AeXhPF0VBjhEeXRmME1FnEyuFERVbGoziUVcDaCUX2DqOOLiiZDSYZ3eXWciqL397p6gYj+NTH6nniVipPtO0kmx1sopQ98qbmPfixjdfPJawLoBqQ7jgrtixMJxD2r3IbosXztuzLhgoW7R/yR68nS3ZuO5KtsJ5MFZQVZIaUA7foCTVrwzqV+s8Cdnpn9mSDXooV2ERTDpYyUF74oJcrHIThLKYAZ5pvjgCFSVxvfcBb/jT6Qk0hg+cYw9zpw+OZUWFeIu1fd3A6Xl/+SxHde7h0naweyfijeK9WQ7lO+uIFJQ4zeymD7Q73Z0oRFVESUSJCt1UilQWTDSRpz0ZbMq+GOFn1duIezjr96enTDHq1CapBw1bYRve7ipIrfJ6ehgkRztNrqJbfccFXtETC8QxQmysyfT8UlHQvam9RqQdqUQsyb/lE6Pku2FwFemouNwr0LuS63Q654xG2sQIjzwxu0HyQeYoGArdmNgBD+4vsCeFL3+KCVkZvJ+WwDm57NnjhW2+zDVQsb+Rk8NO21SchpW7OLBldyFnMysmPi6PtAzAVviy4pIF9RG5jDiTn/iG3IBdGY7cDFXrhGwK6Tx+C5THcyyNR+JonvRLfsVP0TbyRvL+uls1uJ10ultrlwsPQhZMwt5EPbFjdBtlo9ZsLdTKY4WnHYDrq6jKT+X3Mwo4XXt2N2MwIraG++BFqZ5i0Q1I9R5yuRYOhsnFLolF874S/ySb8HzvCtYTR20ixRImUR1xY0vWG1o7Jd2mdXo1t3sPcRa/e8G9epBRWd+cj+cov6RWc3nVhP2prTcb0VVSTryhlY1UN0D2fOrAQuqA6tx8/ObTr/whIM1ZuTnQezqeCsYnqvQF/qcL+Ai+8oB5Yo6RkViXiLLx9q28Alg+eP2w+2P5Hcg5+fTkh+FqafcVV4DX0yfV4xvr9BDIe04IfRpUPDn4Ve/Gwjak2ypaVDVwLbb6pASvFS8D2TOyJbEsi9hDFuEnY0siyyNxDlz4VcT5LWnfyF3kTNOwrctSPHtSFvF1/FmG9glHa5oXiYYH7BniPm4nn2y91YxL8dX2MlXh54QJ+UT7/QY8T/omWPOXnwc++qH6tp4IT2Hp4xvuKeHLa95+NO41h84TndwKoezSji3YG0BvwuHW5aqk3x9shewq4FJR8hdyNznPxL9XItGzP+sCriEuFW6OvFXCne7G9DDaHZ1LWWi/0Wh8J3Gy0UJZVAixn/JDXPGPSD7scnCJcAjshT4NT+DtU94pRsXEpSbtpUjWIvzHh4ZTHdwtnNzC2hIsD6BzJUO0f852l3GHQgnvHT2zcfZdeDlRTFxMjFsk580DvbS6qRBVoP3F7M38lrMOLdp2yyULRf4yCvWTX0JqiQp4lJy2QMmfA1phX4ccnisEpxu8UxxR3hycbnZKqtcS3MgTjOLbco/MWU9wHXE1O+xfbi2j+M4sI+Uut6NydzVuJ1+eEPQziu+BEaz4tOe6GnlilJc2cQoOHcV7zHdKaX3sp2oDfcR4Udz8/jGFkYmd1CcqOEwxZRlp2U7qyon9oDhUvG3tC507btevIZ1pnRdmz2wXL0PojTe3H6651u0vMhekKOJedh7+65GX6iELOteHZ36S6r0j2QZrRl7Mcr0qDoq3J/KtDyQB8/PEMH9GZQgYXwqS+BsPJIm3z3G9KuZB9uQs88HegZu5gzf74EdbtqAtG+Sn7tSdX3kBpuOl17RUjuI6NDlBLaHvlDj6dB6YnvTgwKRA5I/qAoUcp4EWWNh0ziUebcNzJzmDG8VJDmI3uNeqvVCXl67Dqn8rYOd0HDOUZu8dDhvcJPgjtfQzW7KJEziwbkXeGHcGpuONCutncTcev086LhQ37k40RnXF4m04ddzlpuHwMI7BfaWu7+B5YTDqThUP3AGciJKxMwExRBk6WLHxE7vDlNdd//l5RTXejs/d3b4NUMzmomBcQxz8R+yYYhA2p6SmlYObDH+4ln6pOJeYwsFborSnuaMwwkvZUj2HW/HeR5TDStOiRF1cbxTqoWQNe340bO7FscRgmccH+MA/gx/LCS/ZcQd66EtlRcQoPnSDNkOYUEwYbieEm0ouFON+XN4ctdE85GYC4l9IJEZ71bvZsEEoL2kUMhjmO8ck6kaFt0gOhvlFBw0iLUeuaNt3FJiayg4Win0oUfeQobUT3GXEJ+pI53OziDkcVKmQOcq0UJC+KPrtHHkTWgzT94pMCGkYwAV4sl26mh9UXDyBw4mWSNfjOEMwwyfqonti8FacOujxzrC5h9yE579yr4I4f6a26wBuR03neQfSTk6nL7jYcqubiRGcsZcWRprmzsA0PJ5injSYWlV15aw6Z2cAnkUBvSAZbsC6PsfvGO4vQaamwkLNNlcKJTtZn+ZkpDi176+cV+XMLR2bg8EPtfkbUpR9ETJwW0LilqCX7857xp+Gx1PcUZgGCQtDsczy+qM4EtX5CFzb917EW3HnFf5E+umptEV3X155HxGKBvLFIrMvzmQssBL8M0LQNhzWS2sWn2aeNACJcEPJisb+WdyBG88FEhgksicY77N/dYlTOTpW7/+L0yrK623987q2Fpeh4/U+X1ktnLF25pumB9YJFHN6WmZ1OOFDtAdGHeGkUv2cmJlZ1eG4mjixJDYDGzQl+MOV1HNFOcQcDssOpk9zLe8MAT+ztP4gSE+A3zTJvSmhKxr34M0dvpT19b24jJi/BStanGdwUL7sprHKB28qBBfm7MTtaHqJcoScY8mrOn8o4qI1yZ1oSBiIQh04rTWAb1rViiuIuX9gLW71oO0O66G5o4i+3naDIC+5rRvLOcH8Ck5ipcqczmtX6iFK3ZeZUTSEzh/ErfsAucREbEQByFztFd2GFbBFyVdiVq27k7yZHJ7RuuCUROxED07irinwS9kUEYrqvWD5DRMV8imJupNJA4qiFEcnuRh2m4mweRyK62IWbeo9IlF3RIUMF2Lzi07gLtxyg3cg5eB06iF7RZK1vas5cYwcM0grFnHzT/SdMPZLIszoiLyIQRXbwmASjLeCaLPBnMx1g2Yq1pQI02ymehiMkxRWSeBVTfB2z8rKf/cup0qemmxWb75/WPkvewcGuRJ8xcU1K96UUq4QJtZcodYnnjjXQy3QJIc5pXAI/msLFrSZSTSsdeeuk+qQFq+G+/G92s1veDulLrrYcsubqEINt+jL/5d5bOAWGC5IvWa5liH3VnZhb0CkA71W2UDmA7KS4faVasMku4n77HATpPy4ZaXElVmSuXTcP8mC1aTvIPMZWRH7W/TXJnVr17/h7RBccrURKmuichpu05e/lrqoGRITBY7XGOzB47eqUR6RnThrbWZWTiiPpnYTQluPX67B6UScv7eb+foN9tGbhCNgcucIo0XksOCzs1pvEB5XEdqxdPkMvCgMRj3JguCdIFJ6eb5/mcqLadWX6csa5tg1zARlRuaV2ZJ0kbVL02sfFi1vOtu6IzaxOBi3k0/9ZXN0ojalB/ZvkrrG79gYeZaQ7nkp3W2GktZI3xNcGBD09Mj8kjgn+vkIeQwfuUydOAiUmJCscLwJdw4JFSz/oSPoAWOThIOlh/BpXJ7XUx5f31e6zIPuxWv1lo1KTe2pyd/cPJk1jedQRfru9oTGXU3LvZjewvUukqEZya11+RqPkjmbbS/1ZcfhVHwzr+G+UDY72eZCb2YcTsP/5NXfBfliQU/rnYeXvonmGkgPG4rmGOiaHtIzXSeaoy09jGVzSc8uLQKNTRsSn+HvFEbx4kzx5xkwf79f+PDVoMhI1jb2+PJz99Et/P4p4/QUkD2M8wotNoIbal7nF0qzYDrEJoh4O698n8x/yd5/JTI0hjVCjSvdla6arVaDLuKHT5inpqh5xa05uAzNpHKiGS5B8Rx3KxlWyktKp9aoqmgbO8xuvQRyMZEY1xJtYtgEJqYrAnvEh7277nzp4faBtezepXcHhdQOFu2FZ+HmyW6bHW3BDbj/H/YoPYQ/f/FOD6rFXYPk5joge1iE+KBAlN8g/YfNozg4+ZgT2ynyh69Kil7ElEPoIHb4dyaXf0j0/F+0RprHDg+mM3xXip5+HBZw9hjei6RO1PqKnBA7+zhk48IcMGawuP1sg7TD2NbPMzkmIxsHk5Jnc8YSoFRJnOLkQSR5qT/vZjDi9+QXoWxc0kLPWeerz8qzyLL3NZeqC9hIRG1ItwsA3b0ZM6rUFOmB1ZB2xeb6dyPB4SZf0UWxc09Dfc8cwZMTcdcXUKzCLCq4ez4tjziIbe+uU/GOs0sK/jmb0isH/uq+MZ87n6DtWkVzdwWoIrUUUDYrmge5qDgo04JZDC8ZNW6VB59i7UkxVbMwx80FdWCNwi0q+0yMvIka0LMUqxWrqwXOyf04YWAgEgWj/BYUjhP7uiNQCCpqyI10WW+joePhvDFI10lbx8sDFB97hYi3BZv0Dpv0pku0pZn0DFFFPiqLtyvD1i4rfkqoYqg70zbR8p0pONvd7wRG6njLFpSBqvJIEdTsttwPJxpyQDQ10hFMkf/6CJfLmknS/j2M8L7MvDyiNo0UTElvN01TwgWkhKmCEpSOWnLi2zVNdQxMfDeEsyJqSF4AUl/gucbKfpB8YhCZSHdXpM80EjPk4B7aPrkZ5kmDQV5yQuVWVO5s531jimt5B0R8qe+uEg//8BfZtc2336Y2iIDQqQkYvgHrTYTf/9aEP+iYiQGMWRVI41IiYnxzK4HjRshC4UyrKOYNlRJ42dUV5XFXV7wsLEkUVtw4VYMHTrI6KFbckcuLrbgWDyywWimW3LGrC3b76UHWyQ7IiUjczImiuVAjbOOtkStKTGGHgfU2ZrzbQHRYsG96MiuZFRKQ4o9CUEmScMA+4I1+PDuBd+FDZzgjnJnDu1MifD0kWj5vUBtfej+uwjl45fIixCWBj2qwNCn4heXKiUFBe5/xTWNBm64Vb9xJLnMq6Aqsds12Ijlv2Ux2zHQML8pwJjv/AVKcs53BUWQEpzrndLXPP4/ZQXoKwerK6cTeA0vB+cQFXJBVNNx26a96Cj2Lvc1zbsm4zzRRtf8PipNxWxtW4xDVTZom/eQrf5Q7otlojR0Zjspa6bb6m/Fh0q+Vq9miTSAzPaG54jVcgpePOHOMwpqTr2MbxUBHb0boxkT0fKAcQPWxDm4+O6OgNg3DtWnmk43CpWkXZmMyUR8e38tqpgVu/ci0L1sX19isn6RMH3p+cBfeizbbGHTE2CWhiKpQhme0TmxT6/r0QDvdYBNfvcAsuqt9nAuOJsrbRBrAGkQy0QF/TY9t+/m4EW3bRa+h9//ed3sJmJ7MKM9MHxyF6mM5npM8obafe2ZRPBr0knPYuu0Yzkfjebb3uJXCaYdp5vWs2ILcKPBBPYa2qtoVlRnGE62WqM/GVE/p9VnIHEli+tJsfbwM963bRGzv0e5lBJWFMJwj/ED/KX9ilUYyy1d8tIf+sKSQmMMPeLxFdn3lh/vlxbgTJyTg6ZiON80GcRfzIjz3aln23NVtLb9cqB//bFE3bzupHp84y9vGqdpyMJHIJ03fkTkCJo+8z8ruPyOuowHVSV+H4Ls1f+FR+X0SzsPqJy6RyFcpoqUY6i9uKqScEqN68rr7KG/La5E38pf/+UVUcz4th3Je2m+5AmWd5p4WerGm79nZ+Pc9+DLPi0fYylgs7BMrrCYW78ocZAXChuMS/JFq+tmCHGIWh+cE06dFdQemYnSKKqZwL971mJhnzRqR3BsTu6NQP05s96Gsr+shVRDzd+DxzHG40xAlC2tvCVtAO77TGyws0qeqpCTsbfsB2aKrPP2D/C98qZ4wH7xoBRL2RCWLLdIQxw3ajV5+kL2mqkx3pDjSi2rbYPUTp0x0KEUpdO+9W0Qu2pNne5dbzsuayg2ix6UWBBE7yfUJ6/PNTRZMk1EOmk9SDtxx6yO5BM3VenwTalmuQFqr1WOzCZuTUpPrwoly0vG7kgdFSV71yUPxuJp09JnYlKVcezgx3KnT9hvsU4NZTpG+IPhkwApvEh05NNeOXK8ua3RWqlK4ygqvAB2Ld5wmlLRtz1gHwRVlxwJ3XSbyLcjzcUtO8UTeBTk+HsmbQzyMApVVnaxcA3RNlVSdbcDg6X1mQlZpwMqL0/fVPQiZ+CwKc1NutT+Sehhjgga8lieBiMid2oWVF4AXCc9BZ8UAyE7dFu0ojqXTTRlW3ZyYQqI6iHChFG6VZlqDtss3Tu/S3n+7l/beAoEYP8w2sVpQKl46JZyocB8418Xs45zWJF4pVjTJy1R4CCRBqF1yvXiVcMw0J0j+LmCVKLuUNkHbsh1TEt2r3kW0RXJTp2Qzmj5eb2HgFzkgNcnLNTreW5Lsk7TJ1y0yxhMYUnuL8h4W8X1LOkA4bFojkems2ydKwCUx5B9r8v4qA81Hy5JpthQrZkZp6WYikMiRIw1slk5HS/j1uuWf94Fozi6JinHR3BEjloHgOfJpXiY3FKMQigbQ1TMqx7JFc1PFK9LAfIR3PEvDssGq3idAItai5krN5gAJ79aFOucFH6eFerZLJhiFVisL2pTBj6G1c1SibgTYYYKZjLDkwN3ICpXIW4or9yZpJqAnWO4xy+sfibqb2UKPGjY7A/qfIwpEDcMyEjiBwslLJ3KR6QPftvMlR/+ZHUHxaL9iEF/o6a3pU8EHh5spNnbtSD9ytRMSbf+OfFJjuAG8zGJ2uOTEs8UpZI0qVpqLq3Ylayagx0huieV1S6LuHwMaoOJw4uqQGW5Te9NLT2cFRDjWIlO1O929xi91k1AkN66EEyux78bR7cgTJYkeXu1ISmhd+PhS159Hco/YgUuSdQ9Ul4JpmZvhx0tvHoQTl0zevTe4rWu7yq/MrmC0rbWhvwrln+M1K+tv0Q7yN/Q1UfVzOON7dsdZu30kEgmU+t7fIGqE7c0af+tbzizEBfuYHaws3viu/RUOEWXqMxu7gLd9xz4tdZwTSpQQ0if4kxScH04Uk6UXedPw+Oy4n5qhFC1WGLVsW9fraziOlXj45/Dh/y7ryweAZWla3TXxYheDHT/NNhWU4GRyznhXEUpFDVtIoc1AC09App6uVHM/E8sJ8/9A4cRyVhHzN+H50NUxAsWmNZHjyFl7+n7ixp2MamvRc7TX1083+qP1LhoUK/2gfC8v/2hDWxiYODkxfrJpUqzQF5LtKEnlNnEGOI2IHtySS3DziPp0UgilsNvGVwdH49jB3AyUgirSTxwyMdXRMfbYEOZK7vC/oqeJaqWth7qOjWGgb6qqpuN6OLbBYYNvXFYtOYmcvXusEOWjkglGvaboFXh4XZ2VX94VsHGrC15vl7d2rVOUipGHH8XUz8AeGBEFTHe/ULOVyWYG6hbEMg83PtM1MMJKRjLfbI2ZObHM2/fnPrj1byGAeYSRhqfREY2VEscF7Xngk9w5J2ibS8j/6XX5vZ0fN/663MF/wf3hJ4BVwPsCQAUe1OMUWC2UWaAAl6WAkR9/3BFk8PdPB6NscEdREEDu7gMnosPuGBIG2hVmiKNaWwXPmArZEnWbxJRaVNN7BksCuNMwRHQDjb9UZ7CCnXfA4YLJMVAxbkc+s3Ct3iN9oTf6pJq+k7v2ZV2GV+DayS7LQXjTGAx3RsroLmgKdexqznA/D3CWBzH3SwHBf+FZlwZQh+oz0Y+eVoTfx7VW9h1aJCY6UpqWj2m97weNQcjgf2y0FLEh+THF6piV43mXYh/TjRkV82OU1ltYig2CSmrRkKbJSOs7fym2V6XpFuuk5eG5qvQoTRsiaX0EtfGqfNZviY2H9K2ew6NDaVrepPU+LcVGVUZ6HCot6cNdQE0cR6C3kT3vcanxZaSm6V8jjbHBO0xZpw1j2fbAUdrCLtjqwULTFlWtWjxbvLNpOu2ads7na/upDc+jdHNZfa7ce+MM3eEe3PUYodGIp7R9oFk+NrhAH2wMPnoCN1vRrgA1ZBAwFftErWb7izbKGlBq1SU2dllZqVoybQTUY5qIaNDFYtIWNM/H6mHKzRj+0oHPKILXtHnq+vEqaaP0bA0wbPQrBnGt8RkkGat93laBhBUq9ONfC68yyVIgFtuaFKiyGfowvLcYDsbwW7JINQ11U9GCiwJGsph2BQysaLnfgLkHxftapGHgpyFXKR9eN/dtdQ+0hGILw9aFkJUitlYKrhT11oshW35MBdSgx0vlhgbn3s0njQSrZsrVR0PlvnWWtqpaetbC4dg23dHliIGOg1laeC93oERaMUw+NnRHCPVtEdAH6tT3X17lA0TISN+tfVOyO8nIiPxcMwMOrz1pFMCgGb9yc+00MQBxSLik5kxwNiA9tcyZyNmdoRzT8jUbZS+ZI5BmPdBLN7+q0CrH1oEhK/ayZXW8JlrBoI13QmvZfyjo4FsgNQ82UepyxmNeTLmhr7nwobO5sBpVL5FcxgrdoHG+xiS6Yqc0GX/kBkRCK86v5XGXtiOfmkx5kDdGpg5a5eUBWtJFa0q40Tb1B/XQJu/m/Edx4nx9jysM3hothUvfkV9f9PN8r63OYjQk6AXD6XWBflg7ajxCBroZsuq52ABmayv4DUUIHJ3/jVuE2htz8WH/DNzUVg2vVQ/876d2rfhon3Wzj5CO6TWnzYJ16zVuqvAa+jp74x36mKQ7ebrnxkb1to68rqJ380PNlMY03vhaS2yKbgB3bGvdXRM2uvvZTWgYFf1pHWrpuHFc5agegvsbR1XfvQMGrzqrPmz+qOmXN2f7qO//76DYFufDmmTa8uqsxdj+3LP2rAFVkPcg26Xs0izyfpJj+RAiZrIH1BtenYP/bYbY7SOvXMvzm987TVJ3s/2+zjAE9bHXjJroYBsbF0BRNS9hov83/ITovWII1qsOcjJaOjZVj85QCFD+wrPOg+xBZ5rtt21g3YpSrZbn0gOoXeZcYle59XL0l7Vwuml/VrvZPFs3lq3OwwdIfUcpBDJiKWa+fn+pfnzb/1b3M3factLnr5fOyru8eUFsqAJrgR+SWTAIg2TqbzmvdGijTaW2uN8PG1m1Ms8eSLN4m4MK4XQD9x9D92OvBjWPPo3alrLUcecOCsCdlLral0SdT73/ulZ26NWjSjqvKaRal3Ox9jLaSL6nbcj3/jJ/S8Dh1Qcz/JafkA2BbE9ez1tAvwurtAF8GRzuctBRANbOxSEVwgX8+0sulRf7RgmP0YcGlSWWhw/AncH2mbHIuO25j/m759+/1TdBqxzNpXUtY312iOVi3L49ibCUhwXUT3G9WOxSOVgK+SXZF6LInTxnK8TZ39uIPJH3//fV41uQ2/vb5EXToKVyo9ocEltU5GXXBL8tmTLg+iwHsU00E4QIFML3ztaLxR65Z95L4qBuWgu0SxqKkZW9ZE1IbmljyZb0s2WRJ3lGWbu1qKX/LB/L5U9sWGdktXoU6kak/UG7ZoPhu/nbXEoMXFm+t80fkRsT9nv/pi1cBle3N0WT0Qcf1MkO11UH08Fvy2VCdn9aAjd703xgsd5An/0mUx4SW26mpRSrz4bx+L8dzPr2n1zd9ZVBlO7x2OXNU/r6eli99+/QtN2yt8/MuqQp9JR5OeqWM0n79U8Mg/WUg4oEMP9wj1q3b3drIigwtAZO6GsqBe7+uNbcXnR5rXNRcG4ZCvrOxlVnhniGDz5JaP0/aJJHAPjKnx2qAPw0br/8Ef994wSHoxQEOgIg4PD8nQVwwrmJ/ol/FNAnbPfk+bNNuFT75+6HjhPAfJRarCvcKpLdmjVX9xkk3I6x16ZxKPUTKq6SOKQh9a1KcohaN426e2TR8B1xtCGTTBK9mD6pJS8pZ3e651drDkHnEgzmlJEDARZL0DP7gOESl7rkSqtSbB4edZe1muIHzRvKDaOeX0V3ZLvM8JYVLKPeHj5sYl1hUCNW107bFk9U/TC8vXKinudq99lWe/KyJft/6jtSPkxhbDjPqhR2kstlTqsiLC8Bq4plX6tYjnmpl7eTysXyvKKWJ3HP72SFG7Z/yJTb6ENmsKyIvt0UtmOH5cUA1dHMvFz5zfMS1gMF83G2ZiYjNiM81csbcqym5vjfjsmb4LXhVjX5eE14tYx5nuV9zweNjTubgtBZ5MTczae8FG4rhMwOX8c7L0rNyvGI/3gkYWS5UYktzA05g2skX3qvH/l3Njo6xwmtWbObEExtfH1zJtde+26tijUrmGwN0KsxMls7frXxr0wWlUi/ivOwPlM9JPE4iLHOn7bhvSU+Bgvhfe6D4+eC49+B45MAjv8B/v+F+QvyHpABAOtKY5ukzrTbnXaVaU1HL5oZl7SOmbT0mIFlSH8EChqBiNmYt/1IWEVyraf+ryw7y4t3JUvdduxuAhzdNnwaBRUnyzTf0lZiyKAG80wHHN9oa4AwKmlkkPx71u0VKfa/6YC/dPSFajEgdEbb3lxQ9nttIFzSR1baKuxJOwakrD02PFGKqoyPpJyKOZABDUgpe2xc+SkkCMCc0zufCU+XggOYKsU2WcoNYJoUWpEUB5TGxCl9DkLpZpFYMEpgek2QBIXLAaGWDodvVV4cPsHpCRaOsyCdW/QL6jdbSdXxZG65cpeNiU8CFpKlAFCns8v03CI4D5XcN1a4mGQ51YKoNJe8saQsiiwqyyKHJJikpX089bkxBPJ8DcR4IUM2uYlbOhIJQ0UH8ESnhf4Iy0FE+Hf3xwRahiT1J1slgVOufg/OqS54ttSvdxIDamqGqypE0uZhCY/o3ETJonDjxQjrB0+QIkJKOQe2zFly4n6WV6FJlTo3iUIflEou3LZYIQbca6NkHnPPJpBtaq9Biy5DCalfXddkKNl5HufVcnPcJFmSkVHBDEuyQZY1gUeNZQS5CcKoVm7ao/ioW6tMkWhA6pann+9aOFUY9CfQscJtAAAA)format("woff2");unicode-range:U+0000-00FF,U+0131,U+0152-0153,U+02BB-02BC,U+02C6,U+02DA,U+02DC,U+0304,U+0308,U+0329,U+2000-206F,U+2074,U+20AC,U+2122,U+2191,U+2193,U+2212,U+2215,U+FEFF,U+FFFD}@font-face{font-family:"Roboto";font-style:italic;font-weight:500;src:url(data:font/woff2;base64,d09GMgABAAAAADscAA4AAAAAcxQAADrDAAEAAAAAAAAAAAAAAAAAAAAAAAAAAAAAGoEQG6QcHJB8BmAAg0oRDAqBk2z7SguFWgABNgIkA4swBCAFgzIHIBsjYyXTbR7K7QDxWefs84QbQw8bBwBlP2okQtg4oD34s8n/f02gItf1l+4AhEM4opTuLiva6UI5lJJDKw1dk9iIckKuNrfiHyw+PhPa5o374lbiEkssDPz21F6PC/MVBmilZhqZ0O4R21ubeZm2bFN+4SicjISx4BqtTonOwLaRP8nJy8PjHORLmh4T63NMEmibmh4LxQ4QJKBCaP/P83P7c999q4CNSIkSJCRHfqI2KkeWZImEpIkKoo1iNH5tVAzMLzY2FtiEfgVteN5v78N+J5PMo7iqcYqFw4jSJcojFEIiPE6W/ss9Q3Cuq5UX8dUDCBqytvIWZ8Qtki/r/s1p0N2bvwuSU4ILKXQxzv+zsCutEdwtFg6ZhWnEuw6KxlnYKspLoXIMP4Bwli43+AObezedAvhU2kcqKJnTblOMMgS5BP9dCyCAyG2X6xjYNIihWR8xAuK/AP5BxRMdCFagcDzGuRz//6eldL6u3rTv9nY7s+zOvIDJ5yyyUyY+IajxOV9a7WrfPLemtKbEvU+KRqkFlUoDWO+sdBQcbpygsAQGMBT4p79eNZmDoWPXA/Tcdpe2vdb/mwIF22JBqhgt1ioe+P9v7087m9p0HegS4UBInJt/Bu7/l9JSixscK+qtKEqzMrU6XIpUEVrn/6Y1O3N1QmnqcKVKTkikRc38yeamJNzsLuGxyfUaessdRSNbcaCQpRfVqkIhLA6HFFjTVKkWITUOCFmqHHhk4a1QGRR1v1p/8zFrbq10SJAQ7ENEcmv346fw7/nRv5P87sey6qkaVSMq6qosY6vSu/4M6MmQCQMuDpQQuLIcLN9YlxPEQxyk4s5IHSMNjDQx0tqGF1nyS6zQCdvrAMIllyHXSwVKs8oBSh41DzJiMCDtRo5V6EwBQrfCrDSrTMfnQ0AXZpyQ6z7ato/yp62zx8XIlzsPHBv5rnL+5CFIwIXrhGOfnz2JDoQNSLO/KXctx0TUgUjQ6c0roUpRtn3Mj5GMurR62xQf+dV7MjQZ/JD3U03jGa375Va+ZoqWvpHJtCRLu63Slt08etRDbPDZTfQTyU7ruyc/UvRKnXG2IZM8fcMD1Q9Y/lm875Oa4pQ66fB9/ZzKv4jl/zdNArvDpOcY0nPQ/f8Q8vg3eQnidd4nPOxONmLy/ozkofhqj5KVcxnLZjJkcymv8gmnKX9PJkp6mlHPIXZkdE/NXB/UksjsB3rh53AGBsDbHcJrH/znUxjs+oTdVXIHnLxJ28dxotzVyPJWIx9pfC+5R6R0hdz3EYv7NdbL7oeoPB0/8yuyY9PrKSLkkEjYa4Z/pvBSZxAV0B43M7zv+kE7Ez17KJ6PAERj3sXieU/r2i+obN0U5svpi7Eb2XZtS25gtIdaxJOk644dkPEM5Xm3u4wbUTCOgReK17la0Xdc7vUnv5PRHBGPPiVfuTEbRLJLtF/Ls9movVmCriJ8CbIT063/jTSHJQYRJ7I8eFEiRlW+o5ogodSFqyVq1BYjjo62Ud8GWxjo+b+J6z4zNe2XsAJUAEc0FVEhgY4YkMgKgWTuFUjhl5EqHyJd8YZlKDeSadxYlWkL1Vat1Nl0UO/VR4vfAEuCBlkeMkR72GgrYsdakzDB2qTp1mVuUJZuhKzwyCAzhWQyUPkMjsIFqfQlGEkwUYGFBCsJNnKJZLKVwU459go4yOMoi5N8HiONFzHEZ0SCBMNCJIWKhYlEyCaVIkq6GGXFShcnR4OExRKW/Iass568DTaSsSmMQpt1k7FFoR6F/rvIG3SHu7tcCMNeio+vvMbwhltgeWsMzbjPkGnTsG9+If02A9atAwr0BCKAiBEGlIEYgLMRG7g5iAPsc74x8ghQ8oNfJjiFj4gDIzgIFoJV5Qp61epkaoTANYNaK2i1g1onaPUCNQjUMFDTIJoFeW4QzYNsEYyWDyjSrKXWrXebGKyioLdd0a6d9u116LB7E7hvoOGBRwYuDWJccMc/Yut1YC4EY3LITQn61DWZNm1PJwQzgz/rEw5CvoInCihURT5UJkEvVsmmDANEZpci4mQyRRazyks5EUvuhTqecGCijCJ82PAmlSebLyhHLJ1vSTUhwaVdOoKfDB1hlHv1lfJR01PTz971tQiIrzLueYCiIAgm7CP1+zg5Ez5TIoL+AQOZytVJ5a0kCMqUD1652qGIImwYKEBCQIVYOOvWRbnQ69cvNwUXnH32JiJ0EBgELtPKlA82DNgwYMN4I4vXUCoJWp9A/YMc8Cg5rKqDVXlBISHfUIqKIazoR7QKRFCOpjBRhoEyDBThvXOh3PUfBBdv5A1O71e98pdO+blf98PcmxtzRaPiSnNmaid4zEZ92PjOuX62nPXH8Oj0q35K9X4P9Mbu6vpxP/IG1xtUct689EFGbn6gtq/D585ZnzFGoSs5dueKvj9wrg/bNJHdNYLJuEcfzvrvoa99X21GKdfUu0/nhn95VISUtUwpf++x8UH6P7QUiYqFTUCXPgOzGTJlxoIlK9ZE7Nhz5OQfYhK+AgQJFiJcpCjRYsXbYKPNul036IabbrntjrvuGXLfA8Oeee6Fl96YMOmTz6Z9890PP/3SF4oIREUMxEL88hUrV6larXqNmrXr1qvf4OyGTZud27xNUdt27Tv07tN/wKAhw0eWjho7YfKUqdOmz6RKCa/wDnH4RMgIi4gnGsuSGMcEJvEJn/EtvseP+Bm/EpNz9WhAQ5qlefoy/jCZlG290ohG9dpcW9jKSaLgL9m1aW1ZPQuR4GAIORsp+jbf58f8nF9rtuZDkhCKZC6ajI34kI3/yp9SvMo7MYfF+BDGEsaZYJJP4XNnYfQwwDD4ZlS1iyoqPhYv5hByIcsr73gHxPgQVhIJLJH3NuC78c3Q7eO6T+xM8il87iwcVfFLDXXXAwM3JPhm6/Wz3WSCCSaYYIIJJphg4tUTkOi8xsWMVz7vceOm16okH4TnoUgpj38vJ/tVZ1BdNmvKOa05a2vuInHwuGALz0eJuCJCZNZf6QsdGQPKr+sXndtykq06ueripil7taYbiyg32GWcY255s3eWMjbYYt9IsHRU8k6lsovn4HkZZZ8xVxhOcU7JZ6wUa26GGWaYYZ4zmvB0z/OESFx1z4P469dZ9RwP/GeCbPox3TgziRkRIY8845WPvANifAgh7MXheUOaD6LyTmzwJXrZ9EzYAj1xMM5ucJNb3OZOfJtn3vGeD3xkLI7nowkm+cRnvvGdH/zkV/wdH/zhb5iZVzDzrlDBMx+Lp6FS1YxzaeVZaUt0XK/uBglDzDCPlvlQ1lGUd2WHg/tSEggrpWykxd+EIMmE6HyA1OU5jBNvuOSrxEzCtHNA5MOj008igtBx2BGyp8eRvDdusEGvlqIy+bLBFvuYTFip1ZhKjxugS8o3IYgyUAkZgIAuBuoqg7+HsAVbaMsbFqJV2UNDTAeHijkj2GXEfQb4jposl3DeAX5zTrCPxPyR5KkN8oX8jk4661IJ62LHCSlTdaFanRVWJJN0/l1vNScfFtdeXg40/Xup46A6memJUMqQ9cZJyS3JgZjkJWdD+PMNhRD4xfUdQsn3SdyhMVqoCWxgQ3fOU4pEiUtJA6l8xBrDkjz+dpwOl+7O6qnllVauxYZrdlolgRNVCPwPIoRjOq1SkWJn9DvrnPMuNBnLb3bYIf864qhjjjvhpD7UjwxDUJGdCOzeb4wIqQEZYYcdwOP6IKsopqySigKz47nI5FKulJFC8qcOuNiUqWOLb6VCjjwFipQaWY1Q2OWbXXG1HMup/iln4POUW7lD2tpZTetdmTi1czzh3dlcy0QmdQak7JTuk0sGOgCTnNkWVjV7+/3sb8MIOis+UKEL8D9TNpo7xZM1CRM+c0ZrFM/EeXquneOn8ySdyON5LI9hT/doX+vNvbRTOqoD27NVW7bp6G+N1eO6UturugorofzLvZSLDhMG/GunjVZpk4GkSh4fM1GAsKrmIHT4H2GFLwgrIWSSK2qCmhCyUdRGyso6S0Z8awod6hTJYicHA8+uWSobStQMmMa3nxvRk0IVGUUlsKQATFpvJ4HnWVu/Pcst/vgzidSJ+CJnJbHiGpaiNNq79dMzt755Ztb3yQ5a0+Ld5EU+tBhWCC9qiAgN0xC8JtCPmCRX1PzLPXsLQ8/AmvD0tphE1XzXYBmSM8n6AB28hVbxnUtklvN9wN6ztaUMm943uxfySA8rIhmpx1LNoUeDsm5D4q5ghVew8EYOqiTYqkhIiJESZxJmS4wIplNIVHG2nfUCczEMUzyD9KxdjqfwQkSYjrDwLISEqQgqD+goSM/IegjjNY8irPHbqeUeAMyGPu5BtzZU81lDyOXiQwK3oI1BaNIN4f0Z+FuBqYxRmLJEZ80elyMnQh7E5PnwoyLgL8E8mo5YthmqW3mOBjbWxCZa2dSi3WYQuHiOxS67VPOgj/LjXyaoQMWBTkMKGFl1Vb4aQcmkFjKcyd+okqwh+lfo+HqIH1zq6isKqO9ZC5BG8puqD6RdSZM8dSWaQ/WtzKjfysZPdm6EQPy58BccfK1t0IAeBJQl20uDBvw7jub3gyEm09E/8h7OKGSBjKPGZnvt0++Sm96YNINmV0if6kv9uJ8ehDFmYO0PCY03Nd7WeE9DWUNdQ0tDT8NCw07DvVhc3DtaS0v278xMkxmwRsN0v9F+Z112y1ufQi14eZj/roaihuqqzTVsK+knjumMMsx56jkHdAM6gIiK3ZPUXG2O9khXtL2LW/RFR5at/4GWNGZh6lLjg0lmeb95fsdfswcdhoBdhvV16jtcLJf15pfsV9e57ukL9r9Xr8ErI0a99sZCjd56570PPhrTpPnF+3fWotVvf/w1g8BdhQAh0BExoycgHQfkz4EAPZ4HQ38EnY+CDgazapJHlx2hwFCR4IaYxjbtmIgRKRa6EBHjRYY4g/UgzV0CODqXGyH8EXnupJEjMihhwIoCTU5szExkE0nNYZKWCT4ZIlJpTWf3z0265ggyDfv7vL9Hsl4CTiHYJBnw9B9tzIZJFgMViCzI9mExNV7WZOslIFt+qf68VjMtzUYEJ4LJo9V1DPqucumojYKSwGozN+a21tXoc9DRYcvTmDP3/lQX0jGaAWMNu61jrIGPtRx8OhkRY6tBEs0JhWWQwbeMf2yMcfr9NHomyEGbjGj8GirTkSUQqLh8rb2UuDiFCu0VD7QAGul5shhLJ8v2O+kKyBhSL4xwPuX69D2NKD+dbeQSYJF23LunzIx+67jlAGJR+WF2QV8ZAolhiB+KQPRESMJk5VC7nLXKknNEAo3ppXvrtDVqYz42qDUikLUktZHGsYXpUl3YS41B37x94lUKeedkNzvqY+jGzYplqFDE/d/elUhjlMuS2jRA6SnBSMW4T8uv2N4ra/kkOZqz0NVQOsej/0kuRvVRhhLtfHhpgXK3QvpGkTFDHFxf5CmqhCKoyo0lLPczsY9eAKEHOl1S4JK6qWjCSGfGRgksKiniKBz9Lb2iXfNgkV0WgVMI9L0z0oR54I20TYyPURMbG4NusUV2+LNanmXvN9pPa2RGUxY5+FdacdBPM8wRi9SPn4jjPOYCJ8wQ9aK9TAkzzHlSv8G4wt5GtABXmKWq0d9+PTr++pl/z8ypxzkaZx07bjUgV32jFoTd1DfuRTz+3ExmgbSHttdM74yDRQ8oqbs0IXa228YW3EmMPEbj0T5Dcyu1MKXEF3naqWP2HdgOGmwbVwsvguaWtUp7pEZ/mlVGxb550biRfjoe8vZggHpopCGpwd6dV0cd/b0gzZ3h7gZ6p5YnHIW/vJSPwrnAY9GCaX9ScMUaB1sDk0EFez01B/SoGEcI1MTRaCHXDCXYeQEiZ+Sd8AMyvTtDRQwehlMfXmCmGTkX5HGMx1HS0o9gxdHk+jRBSKn5W/t+Jgo9yi+FWKKTiPKx8CUdVSoHMYchaGLwhygl/u6WV7Wye8Et9lMI4xTaEWLpUaZta7ZYbLEm/twAHcEln4AeGG0aUSOCPUx93NQwC0tU+iX7l7gTSdq75bvUIIAO3mhPnidm28Qyb1o+ruaUooCPiiBIOilXtOhn9VJgj5ZyinKKCtPl+lO8C1am7bMKkLLad4ob/u5N3mTda077zYafRhC3/WoelxDnaCkypAI99iPLPsB4lKk8dgANMQFbxmicoSzyR+5Pn+0uCEmzG5bXPgPsP7tqyrg3SXWLhRzYUwoR57OVOao6L1GrnSOqSmkHklvQE2agozY9iOlpZJw0ZESNOna/jDL8R1fE+GP1EDH34xVIrds2qKyd3WCtYCBBzskTEn8AN2sFQqp3PMmUGKDIJEzUU/9eM6aRQYURxDAVEgLIFC8XqZNwqrU6qeh70SMOfwy7NqEYTsPaJo+CGhFYiNouqwzAvxZmkzvMOcYbRN01LkOQgPAJhFFkEcJYjuTG+QD7EAbRFUXGexNm7Q9gUUOk9/Yud/cD0nlyNairi/oOHxIOiN4NGlMm2re6FC9XJQwqrl7uNiebYhEdWh9GIJ1OUo/d2JHe2IwmjtoI/foElGteY1IkDT8NoXpxWrQFcjFKzRyN41dVCkIh6dE8qkodcAsUjIgsAQtsXIJayJFC0FH0K5jmLWL8Rk6pJgsWYc2WKtFzlEqGZ+ppm52rBPmgfsKxbK0btVc47n6QmTEN1LgNx0g22+lUhA48Bj4y/lTDlBmRsnK8FfIgFW47xz3HFvNqbvh1gR7WvpOTR2JSBCxShliwxmAToTlv0kXe/a2w2BzjMPMwL3prOVAv/tHnHu2m6rhKx737jce1fgHHQ51ZT3G1mcvMwkhV82xfjW7k+L0R9eC2miPNI2s1dMnQJOT9B9dY9lSG76J4aP1Ujwi0LlIXuF6a8fg9Fhwk/RXBAk16vpqUVQKoDtck9+vV966CI4VZmrNKSefun7uqFOcoz0Zsx9WhqOn7O4wFegXVanOvk6PE0ewfL3V1sb3tFyssTrmCUw9Z/EX3gwpDKisHVJgL2K8BRzpJgVlm6qoAOB+/U9K2/FtU0jjDZ6JnTpii1tjtVKh3qDGsjVQVp1ish5yCW75XTVI5ldwTLR/oqhOtOmthMh4LvcBrZvB61LYfh0mZSomslbBc25JYLPFqqSQxofG03J7WkitB0y6n0ivHGuJA1lyAEpYCSTmj/NIJRCet1/WNSUBXRdhC+IU9SJdtv02re1w4p2eruCyw2o276REprbFvuDHpFxyK/+UUKVsykRmKlkS8fmID3Up8Z5Dj0WcVuw+QsdRHlXJVnPkb+s82fnmHiN4291z9e8aIOfaD1rLQHWfdk4whWjtPoRstCCkRr00N8Skk8ybKbHeykUDBtCdVInB9lLOzHDpey/mfQDfrxN45oxTimkRwx4lliMCm8oDC7FUQqP7Bnn0gvFcgvzcvPuioDWXR9mvQppfd/XPqJXrxDqG7pE1GyzLlO+6Tuyu9n6DlWBE7J2KQWafNn2O2UYLy2kRwaVUNkjIpluzpBudKcAJHKG3QXaclTq+fj14VB2+Vu/rJSqGI4OtLE+VHD1pqllRGxoAFk8qjXWrf6tZYCY3ZtB4pU6jDxRUl2QqktuOlS4HF/7NPFld1hWzBSEJkr9xK263Ot687l+38enpi7proXcnMoNYZCRkRiQy09xXJFwzXz+ZYhSOZOwjv7/iVWjfTBSwxo5z3LkJAII8Sqgl6JAx9nMJz5P4cUfLwhZdPsVzwTvejMwakfdHbg+3JKeh+tm7far7b2dVmHRoe5ZvW7PKP3YzB7EXo6nWHrrSH4ewfn9Hv6WsJ0Wwvj1TUt+EhEsapE1/SwTXYVBf7pk2IzA8PHP/ngQd7+v7hU6QkAqdwbqJE+47F3Xd5DMH4UqhAeJbcNQCU3gGcGgMUmkw9snSSVTbSjSViW1AwBmF7rzMG8E/1Dlt7J5/2Oj9xoUTSnhjgCYol2dFlbcGsDnbh+47Qsebu8jW7t10/U6n+lSt6qVIjXbRpVzD3VgrKLi6r1DzJEV1Rqf7YVLCLfvLu34GX4U893Tkw/0Oil3W7kHOOuWvk4SHhzkcbDO6uVC/5yDgzRq8JXRxOoBA3jx1Odw6Y9zZ17Fgd9yZz79i9Ptmt59frLO5Q6v1IPzMG1JrUpkQUg/IKQWPqUj3O30UTUFy44uPb6jAPle2i8amObPHRXdr8PBu/9oMR4Uwk3ZVqY+PffU4aQ3M89acOF+yi8SiunLQTO+swB5VvoySTNszs43s1eSTC1ivxXGgwy4nhRtrbuid+joih2XPflvlbf0kv8webWsXhi4sKJA4E/SwrxV0jd/dgNFEcFLCc9CMDM9aXBJUHDLkFVwlzN0CBt2nv5h+EQ9yBkkv4JHo9fFQNDmUW5P/z5cShfDorNL2MBtnMQJiiMpMchZ6s2EgvbxuH5AxQlCsLqdwQiIdhgY9lgr/oe452ri8NY7FtEM6gjWST8HCblI7Mo/203Sie5Quet3TSL/dMmujVYM62C3tEqWSPLb/9o/EQXftfhc0ZoPDltoK//2Z5+hx0ryTCmKAwaOXfyS1KrNK4LUjFgdFdTZLe3XxJ71uf3mL9u4Ueq94CzasmoGSDMl3n7JBS3c5vYEmfue+lGfgUO7r4Huz2l9ib+fie6/YXg2v1gMUz42V4JpiCzP8mRkBMd9SDmM1VMB4m+/DCN2IEJHQHvT/mIqE5vWE5m2Qm9XITlUUW9qmdMbZgpbFebaU+ZDvnQN0Yu2dxXoXOtX9O64Dzx1T60O47e/ip0BBpjRTjcekKHv8jMBqXDo2VthLT+M5d9kH+6ZFXT3sWNYMy9qqFknXzmTHMiNj8GBSM0gs46DGgFzcfe5xUA+fxi8fsY9TIxu019l3uzGUz6AW9z0O4Ei6kZZzEjI+A49OKk4gEXNbMwrHwhUXziDIAmy2yfZoLB0TwcRt4krD20Nbrf0xXtmfmJxXAiXtnHv/UuD0XG5NqyzyOVlgy92RuaWvdx0fwo5eU4yPU1JTCKIQnSnZwM1mczK4M9DFlzndwuHhcsH62H+ndCRhqWLATU1Fjjc9TVh0re8nYaG0z0YWvj3PP0bNmDdik7Ozw9jkagDIjTT2ZUUxhbEcEitf5NSxi8+O7wTtM7eBFNYjpqrf31RfugduASO3heGmkyO8ALZY9O+vNPXwOjz5jH5b5xK+WWNlvpqA4k6wnl3ApMZQsPSQclFNISa6PRbGoeCkrgYlNrYxB8aigNf4nXRpaEoyCkHABE0MPDisIRCgkrIPpc3cR45bLreaG1YiImkuDnrOa2XlLP480NGEq0ZUff4taS8ap1FqTtja7y6tfFT8So+RQUyd2GEYSiRFdEZiLa79xd1ADpPNgboHcfYPnY8Wac9Yfi+f8hX8EbE/tdY/sAg+tNU94XGv2SP+JGalEc06USDX6CU67FbkxB67tGYmdJ0Mr8nIQeiJiEt/nI/AP/r/9DO7D64OcXdy+U1sqUmPDBFm7zfgLFTTYWvp7W8s/E54gSZBzQTSxwLV+XRtBQqzD9Fpq3Ub/l/sakA9KEcFMU09wcvUdXkZi8bIwWZk9ONXuqr1ddftnvJ3YvvyVRgqVOk3rymDdatsI21CXRDkzPez1edDaWmkDuYOfbuifFZjbe4XoJ/S6y60hl/Gd2pw3F9b7kPWZBLk0S9W59tPZujqy76f2KNjcDtHs9mIe1u29J9Pc9/3z1BUhBX3eUO8Z+Ahj1ziHZC5/ypLOCt9FpXFWdZ5/2rvc/xIBJVuruPEcrLQoHcuefAWVa4o4EYoTV6kAIOl+ovM4gPsBtxEX5pd8oq6mkNxaztrobugkbuDfdNq7KXJdeVsp0UDe/qL7TpAih6Fh5wyR4iIIbab/HKDPu9jbCkepQyVO2v0Kbmpr163he84TPaOsFOV8cIn8qfNUqVSGSM/r3NqIgaN5G8Kvf7vW+oZXD7da+/Qmj3YPcJDNFMVlfFPRws/HYkmvLRDD6YB07bhDKBee1NT+T19LZfxD6dGgBW3KHcSP8fSX8GQC5OAVdRd567v+B3mxhB9lYOlgC+rBxCgrQx3Nfh3QNp3XVJrX9I031PstM7Y0I/YbGE3zhnqnwfpI3zRz5I/6XdXvt/B/+P57xqUp4Iz240ic0yhkgXnh5aO+fcczKl7ftXHqz7c5T6nQWBOcr9Zrr5f/doWg+7qX4xOlS41y5y33ToeO1cbpprj8y/mSE7Um8I6pmaF1UpUqUAwV6GLPL6CCYorCNwgXZEteqyubpq2ncu1cO2VjwvcueYUfYNBkTVOqRDZtbKz04DpOo6JcJiVh7hg9IYrg4YWO8rnybxhHG2434C7yAUfrK1SpBqs6+erBKP7Ovy5z2erHr/ifskTT7mY+i4PPbcojjwLY9frdvLyvdeZLAOvBPURos6Yo1XYO69XIkTvqHuBlxNW6ip/UddiX4cvyc8zvGqNnUnDmo4z4KGKR8imDY0/BW4zTEyKJJurDGf1focNMO9HM8pf6NRj/NcLfYC7edqPD7pqUK7Pv1wSq29vEpXCJOeV5WBbA8lyNXO6AR6DaQ//TD+hLaZyuUz9SFxMi4nJ+3hVcOwXUgsL5RQQV1RVwwgT8094S5SdBR54yOxnsJUd/JneiHHhXUnWLXDgNtKrWxlrEQHWZEDA6epxTNDrN6Rk9on06CYKnepneg5ovJIJX+BH+PQODugohN6aZITdlMmjrKnEaHlyjmKtAuQeBTpsXkhQPUHonZWi9VOBzMEsR4flLa1vfGDVUx2DhtndU3d73mHRRobrcBEOLrSz2Hp2njpdkbwuTNfeavbNe4YPCmb4D6ru0twUxf8TraBQtogDbq2Pa62pGB05dsTynfRrU9oDZJbc28Z5FbFabyhaUXloCzIu9LwQrxg+A5bl0mSqWn8oGy++araDp3vP72t1N93dsuX8avpyp0pzhgG7K7SP3Tf7BymOqqdy1OAuN9VJEtA6FxbE1SEkcHiSIryScETmQDR9D1OQFnXdDSlAfXr7Ob/D6SZMa6qBn+yRI4H1V50w+NG1KYfrTYBJfcC5U1OgRMzcW1BBDGNWkS3vEbwET80yYtdtwFe4bJs+yPprgpJWhKAdHLPHrNxLlkQyi4rRcl6wPvN0bipLSZLyeAzNr4YGbiflEBlpcJJ3mrtZSXV/092PrMtyLL744/Cw2qiQMZ+DaL5RhKhvc1DS2ZzLO1s8nmjC6NN3oHX8E3OPlzW/ZhUvQ0Cj1tHHQOy8qJ6pdisQ4siXwlgm3CYtw8zf4IVgsea456SNruYFaTxX1ZXMLsYGv6ep+5KTvogsrNmShMlLYWjbfLWE4C33e0kcJ5s2BZU40004aBMmMy03dswbreBSl1lsX9qL5ABm90+PGPjAvluvFxdzP8mp+RcFDTb1bTDnf1oj6cVCNDv4BsCxV1e4a/LpHKUCnkx095y+Nq+/CFNzSpMLlO1eXb8SlaOQl/YzRJ8dgZYnM6KpQIo2MqgsdtqAvxAyisV+xCygVXWhasgeXo6Ih2SnKsfGSkxIdaEU9kYyiG+gU6tvA3zVgj7MG7N5eE5708jvX7iuxNpWI2+v8vEHV0o5dvEaudfLfTvTRk6/Rf0tPoFQELepQ1bTzkhxs7+Et2oODoyecGWwstfNTechxRkNGcFiEj+n9Qft4BNg6GrnloLzKXLOwsfUExqA0qYJjx65zeAMuuyU7ST01XnLGx1KluIEowD49upxbEyV9Eg2VX1oKCiinF8q8ZR22A8rF9jo1bR1HcCmRf0d2inrSKU4b9wYtrSXSEKaJTsE3Hw5vDdWNZfj9knacgdaXxfzgdPE619I+LF6B09Ha0tjv7I6PRWRMSo2UYBFN54St0uG1qe0JuBT7d1miOUyaNzgHK2l0p9DONNUQbTisKIF4x0c+fggyeVVNuzEWVqcFXiDK2B+dSG9CGLUkCqXi8JqQV3OYrZhG1J0GN3WN3dn0szUfMEMnRlXrJQxBf3/GE3+XF6/DFWjwFfW0CWqC9CLSotoikBOBd78cMW5BqsMkouagSiMwdot5ZFoJQS2sG53Sp+sBKFTmrlhObN4IOo1Uk7RcPAcjqINk/t8zB1EnunEalX8AJAkLlSD3fL+lnCAVOze15XDqGImt7rodWEL0oRdX0YZRQOHJ0nCILPYGLSsXY5kT6oMTqbEGGhRnMbH9He+0WOhchdg9bFFK5r5Zg13GMrA3q7L+OI5BGcl2A4rM4r7xkjOWWxhVhWQVJayBnsx08M/sPThhrZWM/2qG2p1jp9i9AyeZIIMXE2pNZotxI7EjA1RLuxcGd8OGD8HfB38T5aqNPyh978xnEIUdB18wvsOx2JAnVAPhVOPM4xzkLqPR1xhGEDx1nUQElo/+N7A5p7f5XJhah+ga1rpgY9gDrqdtMK98L9ttcNrXGlrRyFQ9uMJQf6p+X+SelK6VM+ehJa36SGPpeLy39fFuOX+1p4Qn0V5vCy5wevjPzvRA2uNj/+z6pvlF6/zpqDwxJ1fiq/YUe+2uFZ1oabDK/SCzw+C0nxW0qompdnCVofZY/Z7IOykVfLcKFfddeLsSeyCeuKjIVXtzk+Tm/klOz6Uny7EnSvTJK3DTNnaPyeChYH/HdUYP8Vu90dxU7FuCncUiU7FPd7uvBKb2RiBBusDzGXAWD13apHrg/YDp6yxlcYHtPU6DQHd0SvOs5v4XF4wfNWgUXERrhxOj84NxDqWN8SEruhrGw+S6VRMD4nYQW6/+1r7ijiK1DQ7qjFVrraxW/8Wrabv0MKMGD+KNh7QjE0DGBaUF40DEb+QQVF+abLkEjs6ZR2r78AJuqljdULZ2zQJlH4avbIG/Cqa4cG3nwpyl+4sO4jEkKti1snzdziWOYxotemv1G3JXrW40SyJzcW5X1pRnYCK+UL70Ia9U63dpTdmlkvClikV3QTs5KC1OsHJ1WLl0u27rTv3O7cfmTFvMMXoSe25i38lH7/utq39BwKEfUrlP36qi0HDlRcPUC/DQPM85fDkjIZ3dSmcu3TMZuZy4j36+JAc+UbLml5ch5vpEtpeQcdy53TRAS7NU+IVz7GJafDa7kdM7+/wrNIQ/jNAvfgTKvKLKDERCrsZeZkpSuVROfojhk6L2WUxrjRI50bDDwj/8WjuNAjnhvBsILw5aI13nHvVwdxHB3RLcvMrKrexcNUtt9v2/BmCu4G7uwyo+vqML5+PGB5ztjCjZyfNPNqAiXL4T56wGUuoVGYjQiL1E9buKCimRRLgQLVSj4wsK6v8B2YdGscPjo6Uyc7Lrv6CfHMMOj46XJuqrdojl7z9k5y4j+tGmgaVS4aLc2uvUqMtn8DDWu7AsGnLOeKmhg3KyyitxGCKfrN6ZDj0iaarEh6Bj/bQ9HIXc3uo6xEKZHQyuVZAtdKmkzkPqqN/qHURgHKmpLGDzuXTAGJKp3rEXFN6WWx5OiL0xgA1eQauhYv38+1dK5hOVeMfWtTnauzVrYtTub1uNXf4KPVZ0LM/SYxXdOxiDpWRhI0mkUlp8vI1lktWh5SxL1OEuAKy4OCO4C85trgUxinLt2L03kkgHsH3N3qBUXQXclAMIv3VnLopAqV0oBpM2bZmHMEjU0ZoVbeUw92SAf0igpYPpiZAQMGpI9pu14uuQfNkhGTVrRWrPvEsV/rJVv2ALdq4NUUjzVQwLZsEy9tfawTwPHQFG+f7VdYiP8uvJaJLXUepvX4WLQ0BuFS7knK8Njg4Ts8NjkIHPCaBnbKuoRkKirJQMN3QID9KlGUBqCXxfXRviorL6nF7AkZnoRLANnh1QRqJBJRzU7E1ugOo9O0wOzUdBav1CohQHVtEbBUz3M3KdG5aeswglBIl8z4/xkY9BVkoj3iFG/gquyF4ylH9pBHhDvbDhoqOLX/c5P3+RuZ/vwW4/MQxgw2EnH65E9sHS7RSJxS0UFemiIDZtjhj8WXskyeq0iX4XrlAB6GxbXoYbLjG7KLbcxtuDXTgLt1xgrqXYMnBpO7dF5R+lhbnP80BuKL6YhaUhaZHuGR7IHcWXMCMhq4NJCd8dFx4kLsvm4Jme4ng/5IXIKXy/cf4A5FIfHsFr/7F3cgfO786OjQsTZO41sxdL2rvZicpTg3n5kG7k/Xw8xACW2vuf1lKhdanWcO5s+6sLJXXpaaEVoFWa5fzTdUPbD+dM55rNn/9A/vwn4x8QLqyEsL1LOg/Ducl5B9BDwl9W8ex8y/XTaBoLkvKWHrrwxaacI+C3O669YmOwtkZTMlTgpX/5H0u/WrKZfDhj8INS0Xke6UGx07ts+DAe9ZKP5Lf+4JeDplzvIZ1rMAzf33PP8a6Zf/74CyyNxnlDvWsfh75+gRagzhYukROXV5WJAX2cdS9I5eLM9GW8HU+fYleabQ2PX6Nim5pvjGnussQpyO39NnAKdyCis9vZNJfsyJJIpkPRXIyHZH4jHS86HGMfYIFKozu7R3vgQIJaL0gDnpsFlL5gS+c9W3/jNWhwP62AUUf2vzmDoOKwuarFpYQfooewHcZkZRIrux4HV6JrpHTB2ubjeJ5yiaM0JMyq5ohK1xciH1Ri6ahnusNGdMnx2OaxwtZU/Ys7IYvIi2W5LfXzEl16G0/kNxmnjQRm2yotBrswYdBaZzf5AUVf+ZZWweuWFoKPlxbEP2HVsnObJ583LsTpOC4Sl8W3Pm92Yh6bF+XdYxp0btiqMOlKhSOTtmIVtoLko44jvAIOI6MnkaCRDTcVu4CLq+cDncsPHXc5+knaz/n5iEwlfre7/b2WTNn6BJyrC/R2MPeRxNvrBW6zlEti7/j5rVv/DMeiEK2wZWuh/XZeA3VBSTZYUFOBPretRL4oSD1q5enjv1VSR1uZE/+iimuxEhvskgydtQGfePUsg2Uh89vLiY7Taj2K3uB8XlFjq4h+pqGaaMXhxQnEO7mTm99mzmZVben0z+V/Z9KR4EsXx+AUHF4T+sqE2dJPaV5neq2Q6Qz2F/h+sAE/W5/B09h82EJl5xBbvb1ZjM7Jep2v/y7SXnXz69/uBUr+BJsFTbEnc5xRLbr5UG3eZB/di4KkUWqbIWSWxuZS/HbRSkRCI7FmHtsODeLz+Apb6b5cTh47JWtjOlFOlsc5b3J1PO1ciWhoKNrMdVvvKG5F80sivvM6xkgJR+nZHiqbaLwobJIJU5P9fIhGImp7VRqt+72DYGGFVWarT7Y3DRbsC2oDdlDLcoWh0wNbHZF8o7MBhgKNebZOLth+nZAZk6Su3bvmLEZzZFoAb1tw1MKa2OCK4lBpQ3VsRElRVkjEXFujI95esVG8veZib3DoeMkqrV0Up3g2y+HIWX9CP2UVTb6grjMefeiIFAg9CINUkC2+ffiWyR8QlIKGi8kF0Op5xmkfjWOmr8vCqvyqApYWsZngblKT9wBrmLKWeLcnBrjZmIrdu9t9PUD5qD7dP2uxCl+15oKAo/sM+EIo9c9sUxFuarogJ9r2EJRmlwSktigLDcqvyLK17oOyY0VA7ialQuOxK6rDasTKG8883ywo2qKY9w10VW1EjTL/Cmz8RUmurkqIQoSSOGWZ5OrC+Ki8omjQZW2rW/V4IfZuPASyybueNAi9Gg87VTQ1gDS33N9GKr3MHyxdeb50eDUzoiI/gwhCZE2ZGxXKZFRG3GlN+S5Y2wOKi1vkBTsVs+pcqPZy71ESylQ7dD1OKwQVovl9xi3hilmB8oIocBud3pdw0J7nWp0bp5J0inW+ojlWBbf8VJPvySLfk638kHpwDM6x3XLBFgQwFps2zxosBU6g1vTv2+exH6zSdZQ/dXm+OfEu0r/KdrkPsw6dY9xtCpsg5zFsp1yzeTaxZXHcYE7ggzM5d/x8b5c1wElRGn53/iRKQLZpbs5SmXo9di44NuxQxZF7V/ah/jcdD/wE3eHvV/qRKyrWdZQ3vVxhRkGXrDeOMy40pUt3V34UaS0cojj68OperBphxWrOEcf15GHSgO3J28RAMueVlirl9Th3yKWRtzm6PJoXxAm9rz54VXMUXJqC7T/ffLkPizedP33+f2TjqIdqktRu7+xYvqkN8f+TLdxrusAyNMY+yNkE5Xs16trua5JDpBQJhhvfrRrr6t1lURs8FKsBpx9gFvNyZFv7DzRjhYgPvptyc2Sa+w6ofpLPCyNohPZhrV5jQiRBo2qfh9e3K///BOREyaDSiVZpGFNrQLFTDnw8gbqe7Db/ogA29Yz2l8rFdmDdyXRFCSipAVMw79iGOsRAFXUYcwCctF/0enmXp8Q5u3iCjpuO0tqEIqIpyjQuwD02rWIRJmP2kU31SIQKexlZVITi3J/nV+h7dvhtcDPczZCGW3j6hSYagu0bj24cPFbTrVyMhWpvA+sID32MIyQfE/bXNCI+Ki4lIgnOGv8wW0gyjts+vwLRUE7R8Ekvk0PmPjZx6UEmB/0tDlR57tLb42wWG35Nb89nOGjtJHLJqWzDZIrw+LYFSIgzDtDz3OSu4OFHzuwCNh1lh7jtOdfBstPXZYb5WTqHZAYawYDRuJDhG53kpstxFtlsUfdOUZEPiU111+a7eJs1GHnFjN2AodvrwL1R9WCH4S/T4vEtmgud4JG/6Jha+0hOxZXWbW9cNOx3bjjNMZDsewagB7K/AGggC0uwJ+hTJa5gkhuowJepN7eN3bANOYDQ3M7g7Yiytz4cAl+iLOY7kCbQa/1Hib5gB3wAenAI3InKgX+xpEXVwVG8HSA7AltauP234nGesOcl+A46F7e3Qlqasyk2ZBuQu4fmRs6NG5HnEN4rpzIxq34RbjvzQJbBUUW9zhw2XXSUjtFxOkEn0ZdTQfk/MDRVVZZWYNF6uZ/EA2RAnmCwO3fcEQqdEFpL0LfWZfD/4nYEkM1Ts9CuerAcZaE46AcVaGLml8424a3IkWbrTIqpeJL6s+ATjFvdZ562lkBp67KZov6smV1q7hW7YMHuiJxQawmhNLwMyTst6cQRYa22oKLToLUEPR1eBi/dADrq2IxpeOx2h0MAabgEuT2LCssk4/72SBT3pVirC5bDKFiqV69hoWIDrCcxTvrigkXZzMrEomH39h+2xGiix5sMYhWRxFEn2KEXpyG26KUQOPsh6lU6HcJiD958lSJwhKTw8KXq2xCPkkC9xj6FPmKRpfpD/oF81AtsjkUJ2mfqkJUu6rB1/AuzrASy0EB4HspXc21gpMFr4aPWJm1jP9zCYbLBkh/RQIcT390Ok8hmW26PQur0wbjGAWOtC8OvkKs2dTgeEDWwCar1W0fR0ZkW9pk7sQtE97ujeLWf1cVx7CIDHNkdbUmyrrsvuIEKLGhAlY3Pde60zKBBnWJZzQSd5HPA6sG1m4HG7g6KFnIYBx0kDqg6+zG3AVPFfjUdwJxdfRe54bzoN7u7Ha4gJ+W53QV1jD8WF+siv9ZpZmacdeLQNepngnifLPBNQ5CUhNViC+yjEMSRLZ8BICm2qO0spEgKAjlyE/D92FGK7Az7jpa8Q7Fh06wdLqvlx1Z02oLlAaAARVF6sF5ZCFSbarBeKAwIo6AEfUU+6tNhGICaq4FkDb5jnFC98FwKTPV0bp75RgZCGZM35wH4cywFkK8IUNMA0vymBpg0Fr6aGl8EHwpYHwb0msXpDTyqznV0alAHPWTOkkDVYEcCLIJTFD0UQpbSeZVaego1WbcxWaNLwwPAybfpTHVqhEnSLIl4E0yRPAU6tm7nM+w2truDTtTBN33wfejWyNCLFCWuHd/e7kWKhv4ap/cdFAe3wqIMdwGV719Q8ECnQT9fGVpE5K/+sy8+Nf/XrQnN2uf4nppQYm1n39mFkKZk7AlQT9k9DgZXdr/Oz/fg9v9vujzJy/1qfedMz5mrQTZ25Kc6Nhqn4CpbPYB3XoC5+X/2F4aUXtfje1Bbt/QM5RnNdL3efvO77ZCUfVp5g36zp3R21zHBVB39Zfts7yU9xdjYvBsO5Tj7D/bYK+G1H6enY0cLpLdkpeeNq+l7hHUziMgeTLyxPPyyBq87YDP4xstIMW/UIfUR1RzKZfo805MvR8hpDhOs1uY5NcfMalPax+JIp/Fn6YP61svIvLxdzZc1ZJOwJIbovFnTc0E2bYJuUG/fVyvvztML1Ecj6vW/vyjT3CmuxpTBpx8dT/SIqokJZp8PQ1ABMJx3EG5mH5C+3x/MzE3/a/tz7pd7CWeZMnGn2Obdii4ARU8ieJBkVXMv+KjNEjnyeBRZVDbMg1L3uhyaD1Heny8Xshy05WVOWJWD8mOmyeol5CxZuvzVnrG2jhZMuNCzyueD+aC6NXhcPkzfYNW8H2ybZxQhM5XXHvS9lbfol9s00ww6Xn654sW7m38RyKV7u161DFM2FvvXMdBxCahsnfXfF3rOiZzdInD3+V2do32er33+Atw1gNqovTq8SzocPu5Mz7NK+7eX1tPFpxOootd3QtEIedaZ7YU87T4ZhHcer7GXbR4wKVNm8ocW8FPKt29NZtofYLC9GX3WgUF5GMnTbvYK5FnmTzBuH+Y2Qp52s72Qp90fO7w+RCPk6U5uL4jNLPbuuX1eYy/H6LJ3CguUlXuZXt6N7V7EAGXK9orK2ylClynT/7iAGaV7xdL8GFMpiy1vwn+vmpcPK//yybdnZ3G9cHpS/cszwqda+U+LeD3vRWZuC5abBUf6/LECn0EyA8uFl2P/p3XBMJnncpH4vOCuAtbdQHxzGTCtdLWbVhb/zFA/X723QnPN5Seh5Oy3KOihyTN5DZicEDpPu/r2MtWnhQMTaP2zylpRa7F/XbI+9HPrQH31ZSMFlO6UMY0868j2UkkpIO0cs54dKiP+TvKa6C3JW4ujaJRXIUJ3WR77jtWzk4/P4U+60hsAePkXmRkAfFLBv/xc3Qx1Ln+jCIGBAEig4/GHDdB5ocFqk9YE9ITvNOn46ZTScf/53dM8kk0v1T2X2U8R1z3tyAJMryjLu19GX8qqUy59YjlWf1q7k3VbMTuqWeCRCeBJzMJ6mIGjcDJuwva3e2krMapXWXt+M58kSSNkem7puL+Yr2V6ZpL+qkhdVfqTE6SfNijYvF2ym7ZnNVK4TNSQo7lD0drFMs2ioe2XMq9u25ygM2etXyXRy4N195lj8yUX5qLuOZrXbZjJ422vvH+VJ5r7aUtFt7k2jrQmA3+UiWYe+7Fk39fET36+jRQnU2BW/Q67KJD5f0/uf/gTjVNK2E5GLk8h+x7AHxao9ib2E0VyXkrZG8rO55w4t3OE0d92D7P6Q+MBZc2ai27FvJlUvrMu01uNXmYzr28Zm6d5eordvuriTq0z5wbxhKntHe5yIeKP0bGg0u+F76eX3u2uTHeGzyPz297GZs7crYUvTsf89wJR73PiUuHgNdN5buXOKyzNY0x7U8jNAfrzH5W7Gq8s4TnlHCeP4dRksIW0Xg0CXxxwbY3wqjtFEDv8rAY8A80+0HwG/v8TNJ+C/x/iDEgPgpQBoO4K6y8T6uu0/XVCW11Lz6HCscOB1f0r3X3Wxm1k0ZliOyE/Tlte9nspU8R/xoxPlvWzlM9sEX+nbTpAp/lirCYjWHKQgpWv6LOFOSvyaEMvFqCZAG2eAvNppZOquH2KxK7Z7/jSLEdr1ISdFXpuQ6o/XbvMKHrsT8pmXeu2wiFM1aNZpiRamkrF401qNlvW0XkOYUoezbIoEukoFF+rc9S7QsLbkt5C6Kakte5KaguhW5LO0kjaCCmje5Py1monvaUaUb1IAd2u7kf5aTdrZbIjbTVeQ4udzLA3M5qd9rLWYhn27pEcXU8Hm5XNOgGwdK24anTv9f3DpCpBdSRUQ6oCVZBqoN4GR0Wz3WyFVVOuh5xlX56polQTVlOqCmstP8v537QwoxBIxy0jLxwFAuX1DtKXOkmDocIAsN9EOY0ouzdNYLsyjRnYvJicptBTCbU11LlDAUd4WPEa89kxbS4rJhLlj55sWJjKRJ5C6SVbpmR1fq4iqYqu4kvMjQd/ITwYM2fCTLA8SZHilIufVCnuUiKHmM1ElIePFHqm8V4uDf2zc1mwYS9gdtkGt1TjksbkUHlSnbvFkq9CIevp8VfNauOgBi/Pqw1mS50vliuZibuoZG8HK9eLBPVBMdZLTV5kAhNfiax0XAYA)format("woff2");unicode-range:U+0000-00FF,U+0131,U+0152-0153,U+02BB-02BC,U+02C6,U+02DA,U+02DC,U+0304,U+0308,U+0329,U+2000-206F,U+2074,U+20AC,U+2122,U+2191,U+2193,U+2212,U+2215,U+FEFF,U+FFFD}@font-face{font-family:"Roboto";font-style:italic;font-weight:700;src:url(data:font/woff2;base64,d09GMgABAAAAADroAA4AAAAAc2wAADqPAAEAAAAAAAAAAAAAAAAAAAAAAAAAAAAAGoEQG6QcHJB8BmAAg0oRDAqBlFD6bguFWgABNgIkA4swBCAFgyYHIBu7YkUjYtg4ABBPGzQbYcPGAUBpBkVRSFLx/y1BsyGSXOWJda5yZ7lzASVjpewoesdnNJqyiZUG8wpbP7d3biyUfwc0sFvrNCE5lfDAp69u0aO7RsQat/i5LLsF3Rk4zkfN5fkn/3q+tatO3dvvj4hMQg/MSAgihZMBDCH1+Xl+bn/uu2+RDBg1QkRaCSUFQTIHPaJColKhDUQb85t8AzHrh5mEVQycc8GVhdsb75FActX/p/2caIgjNGjWSKJJpZl+aPR5gvYa7mkihunNvty/zRMCTVxI4ehrP2PUlEma4JBs/1vOCl8lFdidEjDMzKaUvvQFRbtcwC6eprP7PQr+xb7CeTPOb+e0IQSHTUHSx2aTY29ocADvgPzzcvtb3L4LBAOFtsTfQRUuE9crvBkA8K+hLkkxyheGJx47z/37aSn972TlpFs3ZV2ZlXqXdMDq8E3GyAAGoK/3teXrr66sXNZat9OdZ6x13T233XU/F/lSakEOywRdIS69pVVkmFJRJgQHB6BQlBildBZAYQL/1D177c0BOnY9oHV7Rc1cXHkLGDZhIDEWRvwF8MO//fKV2jpPbnKJJsgwHQewpdCla++81M6Cglpl+xcF88A//1fT2m0DW3YMLNDM5tyaN9tiFdDHTkD/D41Dx0OOgtokLKNcUuAKhMWK+L7v9oX1abs9LrciYQhukBCCBLHX9PjfR3knDapGfLeMqT3U/s950aAhaLcVCAIXgMcTbauxhNgLgxS8GlKhIVUaUqshDRStScuB6NQL22E3wrSzkEuRh7Ioq6DYJxkapYGL8nPjYwV6XUfoOqELuqFn7RBwDn2WyNve3s0XBI8pyErRPk/Iik3uc4zMSZsKCdhdMzzlcVlpQAeUgxz3FY1zrcFELC8kOBTj7iEFskrodrZgIf3bZRCuGX7c/XVPNiYhPjXYTfKWLoOq7mJJm7G3Ry4VeL7Da4NqEq0dhzgYvnDK7bkgk3ztFGt4hJvrkWw5Qd/4WFcw0x/z+MYmUsspuuUkLy7ZvFzZMWCD4C6zbJZG+gbCMQ/SU/MIGfE1BXvSDXtuJl24DeIkMoeK4/B8hnilBZ9q+GC/kPCK2bKkDXQGOvG6ybgl6Pia2G+8jysDR1gveGGRE5Hk8jhmFtS0iHYJDtfs+4n3xpT+FXSXYDebD6n1lgcMkYCzd7qrU/ULaitDTDDpEtNrXl20Lnhs4w+2NoynIgmIoqs+MaibD7IdUuXGZFxtt5c0dxc7EXmN+Y51k/ju+0TLe9ayJBmbYTxQONuRTTfiziHfX6hbvTEZIV7+3tVR5Vrz81cKooX6VBCcS4QnQWJhao3fSMsYYjBlSYI9R3KcUYHbqMibLyX+qggelwgRRlXjqGHIBpo2/U+6Lpmn54uf/AKoQBQqokJE6IgBkWEhFkSHi7gQE17ExUY60uIjG1kJEWSpxOhER1H0YqA4RjFSEZOsUhnHuKqPezy1xDve2uMTHx3xi0hnQhOqLxGJ0J+oxBtIYpKsR0iyjzjii0+cSruw8Xek0pCwVIKuMgwkGEkwkctUppUymCnFXEmrFGMhi6U89iONI2eI64h4E8J8JPmK+YkEyBZUir8bfUcJodKFyVEloVlCyzdkwCBpQ4aJG1kj36j1xG0ofx6L7yKjtMtuoLp5J9z3WB+feI7hBX+B5aV3aN6bh3zxBfbVT6RfFmAFUIAeAhFABCMMKAzEABw2YgM3HMQB9kQPRsQIUMILXsSDE/5EzBjJTfKzACvykUePQpSIR5nAWRzUqAQtS4Ia1aBFPVA0A0U7UPSCiH6QWR5EVgQZg2DEcKLE5mDEmKDEJFgxDXpWXhLNYqZ5zF2VVTcnAsctUPwDJzBwgoJIWHATPrEDdmLuBCPRIZWYoCf24sYl7hZPSCYxeEkacBAyUJXBUgZPeRKVj98iqFO5EC5xmohoSaErk54s+kozEDEsP5BwYCJAFh5sxBaVA9I4KsVZOreS4YQEl7bqSL4zMoxy1760+CGDPDLIL3mXbhEgnsXdchclhCCYsDfq63tSZsyjRxGyGDAQr9RGqXQqCSTLjB9i5QYPWWRhw0AGEgIqxM5ZLWood3o0opGoGU21onWNInQQGAQuM4RlZtgwYMOADeMg43xDLi5Bi2ugeAQZz4m17WBVOqKQkAdKsAU/oom2BZEsJ6gwEcBAAANZxMzNcCHnc8HztsBN6EtX2KQ3OuXH+rTe1K26UufiNtVklZew9Eup2PjGvDQ3zMGpPVXHk3GPwjtjagyPdaPyZAM3YV6I0Sl9439R1H6DMz7VzRvOgA9xkHV0UlfniuzUvFTssRSmLyGECY58MwerMz+NO8iMgX6N3L1XVwygSjRBv/hAnVX/nLJx1vqqkDwkKhY2SWo0aNKiTY8+A4aMGDNlxpwFS6s5c+HGkzchH/4CBRMJFW6o4UZb3yWXXXHVNdfdcNMtt91x130PPPTIYy/MmDXXfF/62re+96OfC7SMbDnLuXJ+LJ5IIlOodAaLw+XxBUKZXKHU6Q1Gk9mOKw+evPnwFyhIsFARosWIFSdeYgocA04B54BrwCeKXyAgARGWFuB9YCYwG5gD5oGvKN9QvqP8QPmJGm4Y0oK4w/xh4bB8aium5jbssgCTbtjKsCdhT8OehT0PD228arxmvPsYBS8hiYRGCG0gtIkQ4oKDwSdkOFlfId8g3yE/ID+h5aOVIyQXfIFWEBE3HA8ybPW6UIrHMcwJcAZX8IMWeA8zMAtzZF4dDC74EJITE1t3oICKh4WHg8+aBMdoToAzuIKfH0lSS8gMxdPDR4H1/H3BZwRmYY7Mq4N1Od4UULkL+DyEnNhwofUTCBAgQIAAAQIESMcEIiqMixlSmuHmUVvoEsu/J4EBVR641M4U51JhQy2dBlobBZA4xFhj848TiUsTvkpcIAGfjsyY7T0NUT6pzSluQYUGtU402MRimptZPIu8Xog6EToYYEpcW8oedXs1udgEPv0Q8/CMvFOpMnY8ns7hHd7hHd7hE0IU/hmVEgJxVcyjA8JZF+rY4z0h4eumVi3dKpjis++DYxynBc7gCj7g17Z/EUGxgqOFLt5S+CNDbAA26ZerdQWuwjW4Djf0l314Ba/hDbyFd/r7ODMwC3MwD1/hG3yHH/BT/4X1G/6QhSoDFuiBPtzcsBSkQuPaqfwxK4Aqdxvcdwghh1KvZ9fq/fRGmPkJ/xp/zyTxtn2E4BIhioVU+IRxirAuLbIYbEnnAl8u8vVEJCvBYYbPnDoL0l54iyXqhutilCodE1gJ5no0fmJ5/ECgrTIpwbSNJ8Ki3xD1nEqgtEHaIG9oMwvtVg+2V4aQ2wh9tfvthdZ97O7m6dZX9+7W/tq9Pd2H3lfo2mHfJZUdHMQuH0vHwDy64lmQdi0fADeDckrsmyp7P47lU2c95jGpGdD82LT0VKj9/6VKoPiYGB8JebWU1HbUMS03FUKO6dEp4P/DywKvZ9Apl+ckYSdrigtqktgSBjpq55QglUmIZDGVmF3AEuPPrnG26W1LNqU9cbEJGy7YolsESwqQ9JdP8Md0XKFsOSb97YSTTjnNA8NN22+fvQ446C+HHHbEUdSLDIZkhcS1gAlvKcKnCAyEcePAJxyFHtGo6CaDkuNKuchYx4ZAVJDNvaec4WWUsOmO5EmRJkOWHFdTxBdsor1zzscillkdK8nHiW3sZGg6Fy/l3DX2o+aoehyEM49l20rCZ6PaGSp72Q1zmZN5RQXYEXa3i6IhaC4HJBRYJ/G+rWHLK6eSKqL8a1mplOycmffmhXlo9s6oGTgdpuHUHl/Gs3FhjI7WETOCh9dwGApDYtDRn7zLfzmXzSlOViLiEbsIQocZU/baYli3RglICqTxMEMBQneWqdbjg9bp47RdEDJSbblkOV+SYGbVJGV6I04XU6hSIkvioywbUWZWEXknpnwJLPwG1IAemWMpISgnLWIkIG6oxV4gZsbhlxk+9lvhcqT3Ej7lPFYo3Vk9syL14YetCF9NP3w79TB8KSeeBf4bxjD8p8o0vkMawu6lqHTTQbvqMe/gLtqmGWZM/bFZsVW3jg9w+cssb1DlJSrTVzpJnOcpwdxWRgSYLL6YMNLED2WJJvZbqmXUKRN03g/3ESOeYGBPP0oUrNsUEUeiFTZmyK4QujM9qOD274HhEcu5jx4PCPpr6tzDkYAhHb/hInyGVLznPT2FtKXhH3T6vya6+1zn7SXQQoNbqK3eXnXtxMG8cyi4xhIus9ivlNj3gf8DrkUHhR5DdMbMcU9BT/nsz8/SXLmT5/mt4FMRVd6H0VZxc5mq1FGbuhpST9MHfQjsz9uxs6aTDHn+itx5RBcVmFZR5XAAJGyb3mcjKLqHV9DuwgMUZAkgwp16Ng5xh6ttHEMB673LAORyTdH8lrgBoJKQVyhPAHeByx8qd7iHpyMsBv37wh/w+L1/gDL0IUDdck1Qpgz/I5j8wipQ1sgcbHpvVigkeG83jlKjdtjpb9OuemHWArpBfMaxMT3+G/cmwhgz8JLLQ8pnyudSvrAu6qpu6q5e6aO+KOco7yirmOR/YeH3WwDCoAzr29rlhLOueWlulQuvDGdfSOd1+bpLfVhVQH95z6Tdeim17hcw2NY1+NVs1Jz//8E5MJ3TMc2T/e/Rdr/5ejWqVSj1RqIZfv5++Ib3bY/9EHBPwG3h7tILFjoWH+fsZ1e45N4Z+5urVOWJp5557oVqNV565bU33nqnVt3Z+wSr1+CX3/5YQOBNQYAQ6IhuOB8gR8D4IdDgvO8Dl3oOwNljwaZ0XcS4uv4IBYaCRANEPUa0xWnMNqaagUxcUwREvI63LC2YBGPMrUcQCmVy3l12FwZAsVrCNrIUvw51Rwt07BCFYqxoEySq4WCJze0gXjdbmIxF+Pt7J/VJyOsTjKIBNwkimGyIsiSmhUcmuj17zHWiK7KLNaAb/tH8ijVrcHQLkQQVu0y8qUXs2RQ60DoD85dtXX3KsWDtqiHkYGDALhemLCiHKv8c3cSNGBpwXYakBj7es0+ywaks6SxIoj4g94y1JC2TX9ZOi31dnZkErGNnXBZQyxbbYMkKEFrjbzDRGsdgUKO94oYWQK2FB4+xpC7oS+bQqaTyX8goIYneiOrUC7emOvm2oCgLjLteeweDQFYkMn1ZsiCsiAXKy5C0FSCQQ6u9scAhmIDMiBK8hwab4GDN+w95cA5AYCA3dV57pscouPkYI26brXAFN8O7d1yplwY0Z6Je86/arq1KbhvSMSOaYi8nnC2uMsoxaGU+W2bTY+1JB87T5y6va+TpRLBATngy+T/q47qDsYZQC8hozeOEcWLQ+z0Y//2YGpjG0jb1XBVq1lBklVWxiqM4qhr0bsPGC3ksxOxz3gkXAmtBMvU+HU8eZ4bffrDjY1oywVqXc9rHMRcUzeG918xM4wKzCbEJzHlR2rpDesjI4WHySOCpGQcrAg1fco0huDdKxXmkKEuTFybJDOQty+HeF1T3MpHPD0Dwzn9bXTGJk+4jTh5H81nDW2FUfp1z3wyUKcrjDBm2/i0GczPKTsYuM0uCyR3R1ToOeYIcOIH2lUDpueYRV6fCB9wNaLNzx/aRLE3jTL0xffdu3IMsbnNpvwSOuOeGT7bQV7ae6oVGRUNMlNRLTvsJVpBW/+/xGMCC1KN2IhziBZPHR/rgpOp7Ew/tlm4zxs++OjggZRVFPXUrmpWUwtv4bzFrga83RnOHKoccACiR+dZ4ohtLk6QGFpUwj6GUS5keTuKrvJDytgDTNAMdigyqq2oOmYkjRQ5wmhpbAIsQFRbOquSLnKheSKKoQ62d1XoqdcLtgn0nH9vRo55q6qEDec/mqjjJRL8+pP6s6mfCoAJ39KGT04zH/yZkrUMn2BFuOjwJnRtlqMIGaTBb7w6nk1ljR2tysdlJhrtinjjozc7dzKk+WAgaJ+cdxszBDQfFQNFy4IENQ5j5mCGTylS+wrOUuWNAELvOWjmiKVrwKNOP6AKvl9IjSe3o0TOq1lLdWzTprJNVSb/ZvANnkO7iWSCRSXgvbFL8eCNRkiArOvKmuDONM2RqzcJKJW1e7EhqXL2y8td3o2w96yX3sksNj2gcfPna2l/IjU0wckV97K3P8Y2204H12sdNz9gUffaxchHlWColuFUJ4uOSKBPY7oo2KkVmrjv/UhjHBGvFwWLqNuwB5Gt++dq5fnAQXV29sREkCaS+HL2t4buCXAFIEFnCj7XXmGbp4xiMl7EViiHpookGcMRBZVSyajdiAdOosRZYtiB9K1DmpjO4/SqPFNNn4dCXvWijS8k+kWk9o5JPAARMsnVpRq7Yg2s0Qermi4vDA8wsYty0RhL1TsKa33PkxuYTlntN4IPohpBx04+8Rr14DN9c4aV2hBaU4MnhuxaSjaMyJGnkDVzJ6Vfpj05Sqa+Qg5rW177xrk8jJICVMFTy3g0vqo8ua6GpMVPtTtoZaZSYnRf8Mjc7shtH8zezVnVmo7dacU4MDL7KI6r2milMY7eBM/DJtLYw5sgD4yZN7SCX+FByuppzIC0ub1mssMHKG133mtaGj+eIntO/ffUATZoWtyI5ZExkpsg7sRUM8Yl7aWcyJd8XUR4dqG7Aky77Lu8Y7sEZ1D9iRIFyFSuzHkRA0XfBYGmjTeNbEXALsh6VTt81E7Zi3FKd04nmNai5rMR9JRihQjkbWZIZiUSSc1CCVDkUpn2hFvcI0b1OPO7Af8zfpQF8t1XaYPa5dffOrdAEsu/Z9eXFWQ2Ve7S9zLhuJLiWwCUq7XntjyZin94zwBOC8fbAjWt2WYf7XePEIEwxS10pjoyC2SrY0uh6Y4gonOu+5J+QiPM4k6hoCY14qRU0XumaA68/7y6XL5mV4ipuloOhlc2/6sY8FsiQ6TwfvYQxIBvEwFHsyoQbyx6vpfPYFsrqT4cAlPV8SEnucvFZ+Uszv/GlVcpolaOKbITxWoLqPGNjXrEG3cctJO8GvPCxTLMkMHJEvBThS7d9btFBz+YUzauxKxLA5nMGU1DJjq6v+3fRh3iilpyuw6xYoxS7yfGYstXtBCRyHE3HyhYv9HHlhX6axO0aVtu6VYVa605yjY8rPDmqSu218fEtp7t/RULxwqM/aM8TsrzA3V+DvlRXi2wiQkzETjpy7uvyyCdeOg3SnILDxKoZ8/KvVla5rC9uhndzs/RHqZxU0JtUqaEmqGm1O6O2hpSOPjoU8mtdoFF2iZwYuyW1B9Sj9e78VwPlmlIs7Jc0eRhYsh/MBeo3EEnRYB293hqYKiTj+rVy59mU01x9Yv5LT4kaJr0Ym8y9Fphn3WmAHhjEjtZ9BDxbc+aRghk4sK7sfcKYjYOeO+iwWFQyESxKdq5it6+j4EfyjQ3C7/6dVw1XQDljAKbaSEsshz3V7CDg6BcpXLH6SyS6xt+G3z5JSf2G3/Hbz2mtD7VmP2qJPr5mNCZY/kFuESDbwIgoAzOQdIbymAyL23PVK1mPJe9z11CpTBXXqV2F2tW2pOqllmjX0JAmdWnmo+ldPqBEgZppazUY99A2s5NHFmr99smqsayLT/16WFGzIYMOlJXCoEJIwbW1VQAbu6jJqLbi2VEodQo1w7pLiIp8hFKSwC/TKjtbbyH6BgapJtYRqotpin7UIfWyfm/pW29gnlp6vm5y829y+JfcIjiX0yIn6zIn+o0bUcgUyagbB1J6NKan48qSFPD7cJcxppJdWEAQIFBhG1sanxvHETTgd8geXm9Y/sFrdlHg7XyQAARESJw4So6kHXz02ucu57kZuTsvOg0sdHkgTvy1LJVl7al310Y/GV4bMyD9fuY/oWd04EIYpFQEAnH7I1iMTH1p9Is+7g3CK7dwBzL/NbtoNMBfD3LXkkU7mPSvKVpt18fGWPncrd5/QJmYwrEhQKGJV9rnzbLyn67HKLQehWLImHxLfzqvs7hGB1kHBNui+VAhsgG2VmSHHAIDQOZ2XFTjen/VaZW+qMZRP9UzENW3r6XvSG2p/ylu/xoh/0Bp58+2kUOtI/sZOTLpdcWds5HydwbUfmwemebb70P+9b4fHtUw7K1jL4lsll1q4D5ibn/736Sk5u0hrf/XLhqeJScFwHrbS3ig5Bimd5tl5F6/qZk2sXnmzje3T0qqTw+qn90td+gH5bQckGsCCwKRL8qPAuXb0zW4fyutjOLGqT86Vo0r0OAYpZK0YbYc2s4qd9R1jMUeTpMM9yR9J/FID/dGKVsDVt3RzXWoGg+M08pJG2b7sa11uAyNbqZVHkYOBi7zHc6OuxmeScsdkLeH0yTvc6Ov8bOYeiFoljMOXmmJtNQIMiihRtCyMvcfj0gnOvHRI5QmxrF/WjNdjsr55PMaZiXkdh4c/6Zr7LMvaRIfR+8fnST9iQKL285liR7cZKbQL4YmmWyiHqPl5b28yIUe6m1qvfy6KAhk/UIDqg74q1+FAle9GJcV39P0Rv3IYGul02iA7D8xYtl7N99P3fQM5TEKY/qCyXm9IX96kIPCETRE9k+OWvbmrt7yySsIHaRI7WOrRINs1fQSke9WpTLt6BOewSty5U44Bo6KjcaXqN6X6s6DZVV6YTpkqMNajsPaCB0y3LaWA0op3V5OOauTxENVb84o/KVJQMkPVY1XanBWywaF+dsga1GIrQ0S+eCaIBG4FT9YvlnnITyTPIYvfyXugzPdWZOnr7XI4BF5DF/5QtwDF7qzFnf5UgVDGqlxtol6nm68arm3WKIDeaGNeoFt7GwYzfaHDQI26RuZtPRL+HUruHhB+Tr5bLg7/jA7kF9UQISj8gZOuhzQ6jrufyhshgv48f/sg2KXnzxsq9diBSEQRAfWQnxjJjOc4eUdsRHnhLCd5RB9t8Kji+gynr3PPMi5+iM3Ujd4ByWRWtV19mtSG3EKfX6Ojsoir7A4XxSJmrJYIezoiMQoYgxgZZTqqOarUWMzgbGZjeSUbvaEhm5X43IURemCvqunHyqozemFmwWaFBy9RBf7h9XW/vxtUQtRCOfS0k9BvhQODIpxQ9FE+Vp2GSszpCoMJaIgT7D4fEJqJsJffG18ceE4zkUbylzvM2tYrZ3v3lc2EK340rpQhtggM0g3/9ykn9c/TijbNZgd5EaiUO9MF3AmCmJXbmcE0t2FweA0taBrt2Ciqzp9SPX1S7AnjTi33ub7mvnspMYxT2u8/RdPo7mHzL8489yizhzGUWpR1d8PYquIY/j+PzJd5kw/n0xvFIwqC9hRTD9hmg8SofL80Ht0b/sIO+SBUlOYQXQvmzgH5I7SM+CHQ2FmE8qH8RKvR6xKzuhu3GwRopmG9uHbHzmXsAPVnumnX3xsZ4iU1LzYFPF7Im9xPhaV8tY4G6Fw12xH5AhZCWYHaEFUT69w8IqmntNh6kemar419U41UAUriTexWm9jcwbPp4cdWeIXdOn684MqvTGdTzNYclyO0ysC9x7p/eAXxkxiRMQRDigyVMxLgNjHmQ/O42P45lX2DtbWA+vKEkW+kpU+psgmSERhxPeXsFJAqD2yDfYGl1Gp7aROQ0lbC5GPiidpA5T+Pvs3B1qQEyrXCTavsY9hJviSJeTAct5DFXI0+vPd8kFiI6He/lKuOoV6hH5D2JJzRoYYRRP28kPlfu/OIYmuoe/8jYqPqt9q1tSf+5dQJYXBbG3KFpF7UFbdjtNBzJEYQpgaL2tbev+Ndwk5/lj1XzC+vuxXz1Jb/bEJMXG/iaV7lMK9FUFK7PbEGfgFvy+yDnHuzcX6K4ZvoqZzLqs/W+gvsKt/ASkdSZxgTpBbTDCevTWN0lBzNCdQdvd1uAvgWvGC17v9lWTxCNFG3C4tnKMNUgutUjfLZwnXZ1/FjzGdy55bAo2pZdm4hzzxUPnOoms4nqxKLo4gasjRerkyJ47Feugk3pR2vOd20/wZwTFLVw9XauKY48yuSwVw4ZOGuPYiYlPV7koMFlH5urY17VpzB+Yua5/2h5ZVwfpgQfjoxgvVcNLfPcc6/43iYnXIPWpZ0jGiGx6XV8zT+xltXtVTqpLt48RTjL6Jv5QDZkFydhI+RLnlxV3N2FZ8tAx14wQX/R9M9XvLoEctOd0oJV3y9Gpx7sax7mrgDpEzaTB8Lg4Ic9+ceEPXvy+zG4nL+OFbyk114F4eIfyJggLxZFht9/ce+04J65Ve0T1+4N/SnB6ZbIEHRM8lac21TdsCzbEJlqHi4k+KteBy1R2HW+pUnmmcZxu6hV6yKZ0UQ7eCnM9FZtfFzRtNXi76MrPrsiqESrYT9yrLvzBGmBtF2RflJJpGiE7i/+qSb/Qhxu7w7PNi3DqepAwtjLEn668cfJByx5Ojw5jInMzHg9TLxKJ30AVBMP3W9RvecSi8G2K37RZ+sR8/oryBAsWrEUVhWBLA/FH4+TNnB/98rUf/ItYS7hd6oZFuye+DB16HVRIdxP9VZV/pI6Q/w5+XZZN9yYN6KGsiy2AROxyq+TMh/OX7+TF8IJ9yL/CXaudc8uCZymD6vygb9a7hxnMiglIj8XcAg8gafl6D5Qk3zx4elVrQcZSWtA7dwPgDuqhKRkWkxqJo1BXGEUqkbFpdtdxVRb1DgkYv7zzyK3ot0U/MF1lmmCXgWlXgvPuCkxtCwZs8PsFRPa7WOF1v1/5QDr5+E0zbE4vnXGU+4z7iSXnyG1430+CkGtPgVLyX5PodlCI81CZdw7Q9CaHJSpvsvJZ9nljBi50wB5c941KfGEsX357QIG9ekLl5QYMSO6GEbpGy1eR30M4+5v8xQvqhzlW7yZ9hP2NU3qqetgJNrNLb4qVxeiJgQTtMWeJpqyFAwYfTSGvOWHnSOPvXfqT9QU35CGhL/d0oVmvQqPJgTPLl/BgwxiYkJad1+MDKG75Pad406k+ZJlj8aeTLmdnRO5c33rkJLx7naTzOBY1nF27AgxUqCHLPyRYU9OEWdGQ/pZo6ITg2U4U4Vl46HlM8aySyY1sJgNrPPTGN/sJXb/DGhBRP5KBMvJ8uwMGpKkciYQDbBZeH514B6xUCxYWf1WfJbTiwRjMH8TaDfIpmZmEPHkR771FOKS6ypPvqMndWE8XYO8d9p1pyEb5K0TWAezfdUXygmDMdqJnlB+7E5hFTxHMGvqfOFNB0DO9TDuI7L9AxWoBrtCs+T9Fexk3BLoxdsHjKsxeH5CRqyfM2wy+x7DXNozgG1sYLT+F83eAQmbH6n9MFQiea6xLG7ioijwzIcdupnpRDxhH1m4CUbIH/6pNnWG0a3KE+2nxrJ76G72jLPRMrXIsTUkvCUB/OLiwfWSV6HkdsLHJHa2L1SqqDAmz73K3Ak5EdN6h6cwA4yXI7nk/tQSdFErKPfi5xgSR3tjs34HrBrxUfRDBVVPzBNL1A7MC+a1QTCGDxpRTuGv18htPRxRBrr6btoXktOBUPFMqni1nl5nbiIXT4Bf3QMgWVMI8MFyILB8b6nNcOzcbJRM8+iW6qE8hLniptGUOjuPeBxInMrYKsHQ7KjORsIhP7xdKrCAHwbwzQUWBNmSn8kEA2ogDr+VBvZKXoL7JHj8M8QWmTGVs1V3r/nfNbkS3y5kk/F1hXI4UOtl2eQAJZB/jbIftAbyVkc42fNbmzqzLTeJXyo85YYpNkZZX0z7VlCUago8A7CPKg4GsuXVjZcRhHo6oAaZfWLSdwN9F7hnVENmtCPmuvoRg1IYPIERapl1wT4Ibby4npRBLhF1/7W8DabwYKfHOZsoreQ3gd0fGv5InM3YLsvQ567NgsIg0HJNX+HEqmPnDqzzxa0ZXWiB+cddLI1PI5bkaXmyO/c3rlWCbmZrtQuF+aO6rAXV/IczjcN8sDleDeT2CtIuBvraOdLyskBnFwRtSCgGf0HwaJlJwqC9ruB5RJxUWWlHAU4ZLpiAqxZ7LXcdWYAhxL1G3E18rHcWCVTvZP4Nn/pxDzJyOvZ3a5KZyrKxLJjPt5/mcZe9z2Yd8U75PqEbk4kWjYIdnPiAPdqqV9ar04IKf4dBA/SnSQq4pd16tdArn6Yp6lS4KlbQLpj8h15Im/0EH0+DgakgHU2IRsIqzHuZ51ymsalDt8PUqIACKutO+eTwGxD81eQKMMhPvXI4+Y1bDks50uY7/qzf0iReEB7zl7iuQ9F1l/1IjqtrLaQ8z6VG/26TBgND2/dg8xgEb+F9+Xc1SQN6m/jBEdQ1Riv4TaPzg5/dLgfIzBLX3l/WZcVreZElhYN8JuOYUcs3eem8w3vaOQZnTeFsMtl4D17YmVRd2i/+SUqr1nvFevNbqGleDygOGQmUb3fdvWgzVr9AL5/P8jG/DqBIssFn+pKmS9+Y00XyUqTwa3/oc0X7suOgQH4ocM3fLd1ohvl7/kvISRUcyUuHKIpnh70XMzu8B43bFxK+WZRc8WnzgWbrdidT89UumtKXV9jiFc4OzgvZjcAaFT75HnF/k71FF/9VB6NNsIaHfUqHNOfFz+oosKI0v6T9rDDIUbgukVEbHJ4GogT8kVTQ0kxyA3tMY6M3dE2dwrjON7DbEOn3reS7ihQpu2bnNL32i2D2wrfUv/OLdscY02shMFWT8O80E2yCpAZIfmQ4VAuemOEgO5zgzE7L11ZbO8/uup5W83qg0t4l+W/fxd9dwi3YdTuk+rF3XfQZvoiN1bQ4khq4f4G1mpKuRuWLSa1ymW4issw9pzFO1ZF/TByIsj5PcPa77tFdyTuyr2/iyexDce4uMyQO5sJuxRTCI3WPB0meZbWHUi8UD5EXwWVxasqyoY6is2PSQ5InenfSRrsKsipXUiewIfQnWZW7sKh7c0WhyWHJO5UD2Y1ttdqb8hi5tqO702NwFn4uv5zTfF16A/Kc6szY7HWfjWmoaroLqkdOVyq4NnrxLKO6Uls2SVdsroS6npS2kr7dTkZ2kp7vp07LwayHPo++T/0DfKM7PP/an6/AccIl9TY9Pq/HXC0pgbaTm127+GDxDP0cIEn5bDwamixNQqV46tZOSAaYTSU/ujp+hdjIlTKeEZjPWsMcrl61wb9C/+8AhPyxLBkTEiFEc0CrmOUjmhEYdCtHzD21yDjHlhMmvGjT1kK7TDTCRD+Kmj5sAxEkq6umVibxdDvptLhbXQ4Y6xfl1WZHo5SZm4OGdG+jiCnYQfo+fAlnW4C2/6j72RmSrx15U7/agdj4yhzrWIPTGMQ1Bxq8I3uoBwcg62IrZQlQ4fL5EajlN6srUbjWKVTec1ZHfx7zQVHm5ei9dj7a2ntVs38tY2yD64zUxtJQ5i+an2YP4J/rFp9/6Ek5X/4UNYMN0mgqTjhGOgMCOxMD8GZR0q3pKIDloQLu5EPhbE7WBTU3eVVqE1qK2HUWwYbMZq86i0F1nK13v5ELGWVbEXweRx3pQOTlTctgwyr2v9WFsmpUbiFz0c68WDWH3jeU3Lfy02vTL06qb7CBeghVxXR2ZqWQfZqtvqU6LZ7DXylkQX+Eoymb5pWrbWuKMuG7yJeKvNW0sCiWYAszO0EcHmEeDajaOcLdvSUDxqHEGJKHvTeBpKQC397SkhBo76Bj7O9V7LnfQM/D1hWfau1WpzlqbJ30yT7dXm7I38v1LGSCfVN06wvV8k1+PBCfM4nr1SNVIm/SKilUysK0OVaF05SiMbNua4G1f4eCKQepbp7e2k32R9S9Xr6FWgJm4qqEB1xGAOEUXk9lt1rQxmobTJyjaiHA3XoIxTQPUON3Re3ijmmUqGgWAzOFoYfYdFdmZxqRQhdaSKqMYByWVkP3yj2SWGFKFQVz3/wwKe/X8gE0TTv0G8+C1+XuK2FvWhKQydB/bt+wXzrFCYX+d3g8PsxWv2Pn31PUt/y+y/y/aRtK1pJE94LJKqot0CrP5zYUqTGorjGVnloOp3pguvv8DopliLbbs23Y3b8Pg0c60He/O1K8Z/LVniZuOU4UqkZnCjmTHArcog3CCSdkKgropW4LPHz8nWtDSRl9pm4GOHHFBUgIS/HGJP0u9dwIfR3YvMCeboeE/7Gj8P6ZpA4wyF/3JV217/nwokjUL8Urfx/91Eq3Mnnmm9tdONeqX19r5hQ6DDSZEWctIA2YoI04+m+/rnjUTGXQciVn402TvwwUhkCKH8flRkn95xAU5+bHipblqgeHvaU3v3frQFF3tGFZ+Hg8/yXy7RdNRyK+/7tJp0pDla/lSLIYfIa79VXkmUNbUazmoEkJ3kxcfeE6DyeUKXOQGvgTLPnWY2911FaUOvYIWSQOz2xOmPrvvOQOaGPF4iJyw6PQKDoYA15a7wr9TnW5Sd+Pw55qDOIq+gnqGYEs2I/G7DDD59L3nlEhpDGaauTwKNs5FTqivbrkw7vKp3wGLxskUevs2Kjg4lq7yfeBOxubxccNDnfyBTmrf2vMaJsFno00lGUzdK7JrbjC5aLeOExxD2KNHqkqw4bUTmrzP4IhmZ0Ju+D/+Nr3Blbsuur2Qkx5fEIy/UsdRx8aoTtTnmj25G5HuqHW+EKKLKh2nbbW9p/GTzNorvy8w0HwlqLpjbS/v325rz9/gslnUIekKWExeK4x+wKthdNW//Ka5H5TjZmdLkV3yj0KJsNNDLpk8r+f4Dw67Sv9PNyxUubYnWskhH3aOcclaKf70vEUO0b1Dqly4B6yfmLzWevwoXIOsgkb0NEvngquAgW1skEra+ebJVYKwsuX6A/NTQi4IhUCm6bSf03M6soZ+UownpPn2/vzZV8Qcf+bjuV/xOXiVtSpO3xKAzWv6nSinqezxJ6EVGSNw9kYy6NsmPMF3B2kbA31FPOVdaSPTh0PTIP/JSGqMKyaoZed14YDAf6z9Vycfeid4ni1EkUdsvuxYMOt1pXwDJo+BymucP6/HH/mxx/qiOtrZkhj3FCV2VSNX+0LwqWFfzjY5tTZtThLw7sYbF2jcXxAlBfedPye/WW0a3CaMl5q8BN2W5J1W9o399xUloj7uh3fq1Z3AV/qvY+z6vQXZDDjUhrCQE11PHhauP25rfsihCSXvdjOzH1t7FzehcU+h3sV4BGRPlne6Gkom27RLr+KQNdW6cFkc0jUj3r7JeWUa4r5e3imZbL3GyXQ2LPpmxnonkTj28vX14/IOm+vz+fNmiQ6xbXmDBfyd1lyxs29X6DMtYiY0CiEmpB1cVigIKc4TIr7IwzL8gPU0Yf3SpnqNVefB/mnoutmBh9IWaW7ouUkE51nTxCVdCNmyMQc2uHghHgpk0c7EJN0ImDHhTF2/DfwZLEEh+ARdbo5+g6vuBqHsaytxc40e5LlYfwNqbO7KIbgMmyrOLRJ5W18OdlJBVe29aoRA3EEyr1Hjr2Do2innL9T6SrFryEsRvzrb6xHXJpSsfeizdtfgemOoPLAxvk81ZPPlKqtXsY3O7Jq/wLvkajUevlCbV3zcNN3sljApyNZ6+EkyofQTZj8+jUF5RHI2SUFsWg8SSbSkJQ0RyVS0Y/FxfshluVZHONRdBcsnArUrKntpseLQUQtO+a/7hMooiV9Otc1jC3KAI8EEpi1ifqhTScD+8a0r+INW7CwnWbpIq2iRY2wdOVF+xrygaNSiaffBX9kOSOELTDQRr7aSKHMCu+79+Bwe0ImV1clmYYq9e8qHkvjDF0vY9JS67ml12VUskt4CFja+j5lsncGRkxw2p3eysUr0o9uAWEqJhFQ+pVXMNejkouixMwq/lf7WbV5pWzkffVcmDWY+KH8siNM+P68H2OK9VK3yu+3CsELndHy+dJsR3UI0FPKkv8ie+9pi+MsdeXdqHIiL5F3vHGRJcuc5ioR4+S24wnt4Gpg+MqHpJW71v0c1EIVUB/JCWf2HMr/4LlxcIWOILedZ+M4UzJElSXpwJrQ/ZX+4JnmxnbqA/MNzlb/bGFfseQOtIF3ke5Ys8t0fFh2kTlu/PcWMpD16XzgfrWqH5x6svjsPpq1Zz82b/mjiri2pdyjf39rSPNKLSixJdS1eWGYaHmAdb6Yrczgdf2HbBZR9JkqA9XN8cK9+mg31vekvXZtXi9h3Mdt5Gsf49u5txzZOYyMZw+8RG9oSvW194kS5EHCHdL3dVDkd4EAkU6UF4c/tV/j8IlGzDQWnMT2H4nPywJ6dnw4MLaOzyWmLpXVlYWlHY+U5hvQ2YNK60Q2moogLl4NJDw1WoEG2oIWNPIa3L7d42Vjnpfy3PzAHVjRqcrZQMcr33ihhn6/D4giaUhUuOD5WhZrRuN619hcRl/syra4ftq/00ValOnjruNpmcQNjSsb3j2d6UtUhxLBVqHIm0KqcoC5yB0neV1qNaYl0+EUUU97n5mqFIlLihpLoAdeW8P2B7R1PbQbso+j9NbSddnTSGravaulgvP2X1QajqzSZrnLV87VbYo1RU2oizcdnxrWWoHHVso3aZ8m4sPHhCCNibLv9Ybsr1EFAdHHQd3eJSaoB+u4YqDIixRTr1Lp2V8j55ylTPoAR7CdNqK2GlvFD07xO4dmJi3OB29/2te+Gf5nXrb8s0f70tg6dV7C4d/OqdvBHozLuXf2v6PGNLzu7LdRxh++rbAFcl0Tl2EpqnAQ2HIo+KdxFGxdhPvLEEoCo2L21zKnOvZh/vsc788ciil+GDo08fN4uu72M9hnvsHd9C2a8fnAbAP2B0DLCCjv+xANze9l+D29esrrOvy8U93mpsxdDBG4ovqmL4vOHhhLkSO6jO5AYTzuxuMgdlS/P9wDr5oPyXfEg+LB8pO5ozqKC24CfsCgWQWkkTgZTUSYKINxDqoDZuoh1yQsZUZC76FtkG78/vQAgZ3jsJSTL0dtSPeiQNvQAdXFiwtcZo4milrFVzMiGCBxFOgnuSTqTdwsXIXCiMbFvICCct9Nor1EoqkoFmh/xjiMwlqL3b4Cd2y2KmSWmxvVIqaJAjc9HTyDb4J5wEf2osLCMk+3PtoBEAc40ZElQCeg+9O2wXwkl+wLzuC/cgXHaUbMCZUJ18gPkg+1oD4DjaFI4kzWZs6ntcMU9Erv6xw4/UF6gDlBtGoT4Llo8let0Hh+Zj5s3HPJuPRSpIhGEPwqMFTWyJjaREhXJRGEOzmUgj5xdkKUcqFQHQudKxUI1MvCnNFmbRf54LaXPqXliu14rIICehMN4yTjXEaP4Be9yEyy0gkNxRft4B76I2BjlkAbJk19FeD0NJFXryObJRLZXxgLwq5VF5a9hFOgu/MH9uR5BAdNkOuci8u+9nHKB+oFss7gGO3F6zS9nTDRZAPZqflotxpHkH0YHalV7yLVBlT72WBh7jVg9lCTJ83UPGbo4Dfu4Bppzzy3yAdJDq3UzlnKfC7pcdcIFx6LHpo/O6B48F4YAqEdC50ovjgZA7LjwUbIEBX+WDLFv4IQMbLHcfMn1lXgXwIMS0shuuB1HgFrkbVFroO4rxQjfj1P5B8tDmeIKTfIAltT1NaQBkkC05zj0BHyhsKMI9Pl8jS5/lol/rtAWuWGhFOEiUcI1ZL3X92QpMOVB5euGra5Yjw52yAqBZh8tiUdytSiA9DTUwVJ4pr8dUeshzLN0z02i6Pq2LXnPVcrBd3pm9viJJICSUIguDK8cWB/nJUFGtX0v55hqz7Mb0yV8Dts1kt44CBaA8u900t6B5oF4H5zBHvcHsSod7sRqDZ/74Yp+Pb/TWf5gHFM7NPNZhF6HcLKPGPQy7SZOLchOAqXwCRQwcLv7F8mj9gHfxfp8TuEYnz7cK7/XtOi8r3Col8+wc7C8qng1zwCmwLr622wvWi9YeLWB9Yk3+72U96o8L8K7hW/umlYThu15PK0dKdtZuKgbn7EzfAG7QyQK6trgxMD6sPWpH/aRRjosb69J13SVrNE4qZafq0Thm1t+ax7q5YkG+/3MdtjG0/Wsd5dY0musEg7Bs0Trbhafbn4W6JTmQPwYyvkHzgWuf4Pwc4+NZg6A+vxs30q+RlEzC390Nh39t5xx+fO0CTrpLYZ0jBepz+8okm03KownGnUB0mU8J32o34/dsfVxSYL1jNxTcTI12Y8gmMVTmgG9Haz8vyCYUvR+sJ+Rz+ld5ugG6sDQM1ISl18JwMcpd9oqDLT3pzlflS79ZpDaAJItkEsFZd110e/0Z6rwC4TWMf64PeWkb5Vz/Cuo6a8G1mya0gWSCkFCcdbl1l3kzeM4jdUKUSm1bJyxMGCMS0GEVdktLm4ZLBTf9B+wmpE0/D2WWFP86XJzhj9jrXRbG6txbb0Aq9VsFVudH+RXhN9AZ/WuYppu2oYpJF/qBYKVVqT8Fdb5WFjoE+3G3wWQdb86GgN0fadNeTr6XWbl+2Qa/CST7cdcfNiBjU4qZy4EXzH8zjP/C+f+47uPeNmeHuNrO20+U7fGrXFi3rUyvCZzTu/O0vMJoH51iohbl1Elb1JRT/aASJnsSdi3ZycRZC+Hkv2odKjkwPrjldWob7bwm+r/t9NL1RvCpvkbrrGWnSnTKF/bRaQ6t5Wtj7NcE8zAazzLL9tFMvV//aVvjq/T5VSgLlihmRjCyk+E2rwUBdc81Ta4ZhOsscVA3XRNsm6YwQKxuvU5w5OnmV/U8NqOvOqmjhK3zIpqt9m7Z8fUmYIPpw/nQJbg5Hp9ejjwJ/5+je6qnR2lHXf8qA1wRFdY+vZsmpo2hx8dYMyhXXEuawLlPWPq7blwJtca2k991iKWmi+KWeEfn0GxasRH0rq9q+Ns2uboOYZNeVE713FjTGKeX52rqt5/aau4J03K0p5ad20u/pQOsd+9WQkBdd3125dSprAkaKH3KzDb1MyLZSmeU/lCKlH6o5BOqZ9uQoKNsXOmC5/m+X7jYqr/T5Q4A+OyfxK8LAN+L8Mc/3Of22cZDNoyBAAjYjyc2wNn9Our6PRrQJ5Vpcpy9Yijx74p9uD9mo05BbaVQpczXGXuXCqW4WjNOix7TDWkVUw50M9vysCF4uNIec50+4v4GLwCBHIiDLXhDJhgsq2qY0xqSvZ7fVKuosW1sWDxfqN5+kV22DfwhtZQELClSFQ93Sfv5iFi1bQx3mhUB1Vcjlup2h9fY2DjBi9VYQSL7rK5Sqbvalzrfpahju2lbfevd3NFfk/3AK/o7Xvo5fitJs3JWdKYHXCoDnEqAS9rSSqRbq+iPG1xqTceJ6h/LER/cP/8h9WDimGyn4IruX1Itq4RWkEJ3PISLBoyxlWlFMZ9fhKxJ2fN378ps27jsQ/fTGACXO0G9oRK8hnm/UsG1NvePJvYvBhSz0M9406FsdX1TFjqLHZ1vrCpvZeSy3oeDOfCkjIiNGd7ySyJiDDlS1/shd1WNCOuGUgc849nsdXy+6WwHnVof7N08tcwX/fD59Rpa5FY0dp0mW7ME21rMqmBY+UwqmXxltsra5PZWrjsTzIZZF86n3oxR8/OMe+D/txH8/6vgf78E//+U4H9vyQL5EMgAgPqzzvVFfP63Nze05mvvi74j09jg1O4L3zK4zlk+cgWPyE6kXP3GxlUph5ozX8Fa6jTrushlt0SrgMNNhIz3/bFUmURSbR5S5F8lDnJAXl4f/P/KY0YtxEEWVEMz5Osbq5SbZWASTReVBrd50vhyYNi9ZdCpkF7SWBFaAmslYEFhdeNCnS9BJYyNiXASTCuh2EeXV8iF0gUCaQEADCB+VfvQJr1cBBwAlopgs1iEGwCWiUATJIIDCA0LR+jVYIphbBI2DCFgeVkgHMScGhLJmXyFC7nh3qm6XzJZWcoEDXbCvaFeO8XWZo50q+KkbcPCWw6wFdhIgE3ENmALsQmCpmOJOpmTiLYTG+Lm5qQ9bCk2hTYV20KNHJTT/7F2vwiBHLWT5osCKclGfjqIg6FiAHi4J8QWYbuzJUg7scVUDZ6TWwp1eVsqFb4RdxvwRDl3mcGMXj/f6IqUcaLozmksXemyxJsUiaLz4zTZYmV/48aZLXsefNjTsYIufULpoqybc9dhc0yKGM5pFikU+Z9uVpTYeKZR7n5fzoAJc6qr3zYRGyu3la+bj+z+yHabDAWy6iTetMZ4Z67M0SOKHyexQiZNNF2PrPNuSiHsTbIpzGMgytOfxdCFQX8SdJJiFAA=)format("woff2");unicode-range:U+0000-00FF,U+0131,U+0152-0153,U+02BB-02BC,U+02C6,U+02DA,U+02DC,U+0304,U+0308,U+0329,U+2000-206F,U+2074,U+20AC,U+2122,U+2191,U+2193,U+2212,U+2215,U+FEFF,U+FFFD}@font-face{font-family:"Roboto";font-style:normal;font-weight:100;src:url(data:font/woff2;base64,d09GMgABAAAAADQYAA4AAAAAbVQAADO9AAEAAAAAAAAAAAAAAAAAAAAAAAAAAAAAGoEQG6NaHJB8BmAAg0oRDAqBiSjxJguFWgABNgIkA4swBCAFgngHIBuDXTV4boR30B0A/SqrlGIkQtg4ADTn8SgqORGK//9jgpJj28v9CClQBMoOC1I5OQ2PYNagsg3OtqXfRW8ldpQ1R73fESOsoRnNA1N6jVTplkYiJxIatjyz99Vy1An0Dfq4OC2aFs1/hgSJZ7y2or9fn4HjfNRcno/s/vlfu7t6zgP8wUiM6ExmBCl8PwSUQbxTP9Dc/t2CGtEixscAhZa4wQiploiQLknJGCmpVEmFAYIIqFgFSpXxKYOVvNqRJyh/GPfp8p0RGGYEd3G6D9QCVQHglfbAfjJhgATTSHcduGjTcYlQ/SphgSFhotmiioFmpTwr3gj7CdlPAICBbl08+wDl9BoAhNUJrIEbf8IqBrbxY2t8H3gLMzADrPiXcw/xPLQLRTRaCPWilWb5CiAgAQL+jzb3QENKcnVKcuQfGqVEidXRynPjjzWuVNMW+3zIgDOk3D2k3MWic+5i5XFVHBd44A4gKJDgJ1Ip4VMmnRgUqBflWIWUKlcP8RX4SsEhpy6E1Luzi8ZF7bZxldL0LsrGpaGo1bRQLVHtxiPUKolzC+pgJJBXKFweYyJNfGxkXIyPjP/f8p3N7J33f5IJ5dH3oNKNXYRiD0YiVM7+SX370trSWpl8FRnJQWFsQKiqWlFRNKO6RQK1FTBnpXr1OMw2Ecnf9v/9z7J5fA1siJCQkBCRkG7nfW0sh3q4CmxWj/eOo1ksIlQ/jsUaBAY9AA9mvLnJQaiwgQh7EiJWQsRLiEQJkUKQ0mQ8ULnIsJq1wXhkAmI6pyD5LzKQqMQDAoEBzgJOgMAKQ0ZGUMmFPMiHgo1DgOOIkIO7+S21jYDzAam/Zx38kFedPQY/cinQey4c+NfVgzziQf7eQAW4rwYOBhjHh6KBWXpw4Jgvr6bTOFw7nWaYSvpmsYPbqcxGM2XJq9eAdWu0xOxS7v/5VRFjztoRrdp6JJDMBsvSGyZN+t5auwiGTl/466RSxAa2asdOc1ktV79m19/53IeHsv4f1yPO9Tt1/ZPv+odM18ysV7QOWUEt+7rPxssWrFkqCpfrW7l5r7ijvnlbb+g71Xptquz0euSr3VFZ6xAc2PSiaW5ZlKcNV+mQpa/abenG1uzebeHJqRwYl6+WhXcR27CM6FYjoQvr2lwZMjew5xakaS6jTu7fpmRt1gvvIntr9MDf9hbtY7mZCq10EOz/BcXTTpal7tCASC58bnXeHK2ll31XFGHq/phIuO0CvzL6T2Pcg+jhtGSzSkXtGfNZZsFuzZZXvmAPpuhmLmO2T27J45612pgvA3LnLOquufyvVNen/lc+dO0AlInbwIviQ3C8CEYxmB+xuBc/wxEgjpokOcxUqDlJA0/THs/QZ+QsE8+zGC+wYuOi1PGSW6pddvv3QqZ9J2zPb8YBpMAyFAgF2IUKoQb7ECLgGPovwCmMYeIc9gjXcPwT18MZfm4RjKCICEdMZCQiITbEyIiLWrQkRyfXZEQ/+rJjGEM5MY6l3FjHWnHsYqckDnFVGre4q4LhjgIFJgQETP4fWTFR9hDWrBMpXBKDXwwhTxATQ0IMovQkpSElNZJspGUhIwNZCeRkpDJSUqMBoTUi9BnAMhTHSCxjEabSMhfPQipWMrOWio104sVIFyPjO0SpMuxuKcekIkZWlaowqS7rOh5ZPWFkN2MOhRd/E+OTBRhfLaG2bBnBik2UtnyH2LMHa99vOH8cQ9AkmEAJJMAmkQSTRx5cCSXIZZRBFvrAVQJNLWo9tOXMrph+1U8idb9EPX3WS4Bwg+GHIgyHH4kwFmYizFSY+bALgcWwS4Hl4JWzJjfTWFtb6oq0EbS5x9aW7W07O0uXcIdhrsPdhrsL+xz55UwStoqT4I8YP4O+9un7e/kQ/V/U/4GyPnpnyVl117k7r3XB1UVblzQu27hhdNPklpO7GvdOQyI3lMjGQkUoj95DDB45eiJ5XqQT4Bp2nf4jF7h57xvyx8zOzD7r8durIez71vdfTQoxRGTtp96Ofv9DWdxAHcG0HQ9KJypA35g/SnPyWFgQmBlg8djJNjqqudH4uDExYXLykEUIg8PgGlPYWBAYgRH4AXsv1v0IB2GOAydn6diROmUUgAdzrxgezsIRpK8mlcgGs8EslOfMBP/yAMGTN/aNMcXdget9XuZR+tKZ+smd9ElM50SWXDHO7f7YU53dqW03LcZq4WoRcaPoBQ/fYUGNyMaYZTXW+akVVLXq0afT7uzMAGH4kkt4ErVndmpPzSXfRPjcaVrQ+tSr4k4UalD5cKU7QF10ER5FT7JQqodyqlMhguFQIKCtnAW3Sy7jxUeYCDHiJKAkkUiTJecqjTTT7tre5DfMJLMsssw6253AXfh3ynQzzfa0Zz1vrhe97FWve9OnPvel+RZabruddvveXvsddNhRifzfRAIZ5FFEra2jq7unt29gcHh0bHxicmp+YXFpfWNza3tn9+D45Ozi+vbu/qkAXnv07NW7Tz/MtONyLS56cTEUph7LCPviQByKI/Fbyt6CLMkxrqa4muPq8kMjNFLzFd+wiCXSU4wSdBg844f5U+FUO7cdRDM6KkPs4wCHOMJvilAUWM0YzBJlGRNGalCHBrRgjAxsYRs72MV3YsnDy+Sj9pxGwYjAiI6VQszUlLrSUFrKWNnHIkPdilF5jCpjVBWjLbWtdtSu+q6x+nSMeWPMF2MxfVnzae0LKfehEXqknq/mm1k0S5beilkJ6/DMBsKyl9Cj6a/osKLvpxQw0+x1Jv2WWa+zx8KrW0X2jNiulNj3pfQ9Nf0CDh0GCmiZ9AZ79HCOGZM3vyq4VHD91t1+3Sx7gtfeV2RfFts6EjtTSlchkOx2pN4i288sF7E4gQhSIE3tVkI52uVMCMMFXrmLpHvzxn0iGqPYe7M4yB0+wSf4BJ/gFSwSmOvpsBhm6MV2RQDm7Tr+PhWMzY45N427TQjtBySxUunP1HqDeoAGaIEhGHub9DLzXmfRq6yDZQxuRbOrBm7LM21iFp7CM3gOc/JKf7YKa7AOG7Apb/WGbdiBXfgO+3AAh3AEv+U/ve4v/OOOxyg4Xi2EYhehYrBwKv5cm+R8f64LDS4yHrHLBfhABERl8V4vVJbs1SKBDNPGtcMaJ0NddAfB0Oxtlr0OEdtfYNH1MoX+xb53YF3oh8w6s8zNRLA0FzokrKQxk8Vu2Sckx+xPmRN7iCAF0rIj1jhTVzmQ09AYQ7Lflp1K/78zYCxm62Fm1aim6nn9gVLBQoULrJQMjOpSVQOFNco617RjIV0FEza4pjNlZKC1qfwnKKIaAcwhPkgF1EDTS1KHlC+iMtiboIyfxgG8F1F20dMTHB29fCHdydvHCxJd/O0dIdLTzdUegmu4z+/g6R3kBVaePo6eYPLLDn/Q8+WHNQNJlHW9ulAQaIE1VJn1XnxDeUZSZBrxQ4Yg4/lqdBSPKOqR20g24oIoIrQwBfXsM/nsyDkN0f77GCZoDAsXINCIUffc98BDmRF/vi53derWo1effgMGUdwKLERXmF8CCz4fweoMMJ6p64X/2DoI0e5FkHw2ZJl3MT1cFKKIU1Vwzdwx47LiLFr+UaewYXcCh5NSPoNVaJmHTXoc2cjlauQ17xOlKOt02OtkwyZf2Xhm2ZuvTNpKA0cqr9FLm70trdDT9ikS6gmTp03zch2tHWIEcl0AQIIijeeoclcqsNzLrkxKoM4Xx3eix+9rcjtMs6k6xSff2HuLb+pVvsznFJ+D3lAdpwfzoEL+1Wa9r8mqrcj4xy66UQ5nqGDbmE71yuVLdR3OaewY0QQPGPkRqFzgB+f6eXYeBC5sbZmlzArmitu6uBfkMPEX4l10Fgfmb6KVGRGJc8pIJOWAPuwn0E5UOdHH9fCktjhpGJeZZIAhQp0/kfiEvwaNPZFhG9K9xZofIIxUtTTvHEWizn4k4hx0R+jsibosMpsd6mkwCbl4GCUpYTCgZ0iEKGvLutVVwwVfdNnZjngm7KiVtQewHWheC2cdF7GC8+GqTbhVZzQC6UjKhRPEmcsLBjtckxxwhPOFFATw4MI5OBL6NyTwFWLRt9PiACvfFey8qtjMSdKKFQ+abEQs2usnOvO4gk8QxmeYX7d0fIQaTJNUME7+B8MkBfSrX4sUuIjfeQvBgXcuBnTWWl1FA7y4hJfgLr8qaw1gOtU1UoFnuIAZnKNZYfhv3qcC1yAIT5g4Kihp9GTJYaVCAzstOk659j+9/ZYusmaKT+xjAfEsKJGFpLCwtFDkQL8YLawJj+IBgaVDN0IogEnGRUkjCOZccTQrsyPUTfAtomtOE7PBLM9V8GwIHdgydwQP1psiBnJxs2t+i8tHade1oM8FDwFuB1wCuNWdrqmBPOi/C//Agw8lD7ggbwfUjVY7LlywwADUfAYshHHp6b3rf2zCY/68/s7cUKlZi1GPPLVsxzFSjOEYeo/e+/exESwWS429cAmG61iu47nO4OLkOst1nouHS4yLxKV8NvBs87nz55n/HR8f3THKhstUzdfqngnPrNiNssG60Hw6FwfX6Q2Lckm9+4Deu0V8/cDZvg+cAGygLZq0Oxw9PurRLVeOdAE/LpjPev9B8vzN+YT52Pkb8+vznvOO8+Jf/n556edipEMXBLize+3N1d7RenmqmeNX8oOxpn38hfzv4sT76ptFS5YluGnFqjXrNmxKlPTLeYElS/HHX/8cIwAvDwIIDCqYPnYyII8EjB8CDU76JDjr6QDHPhdsyuJFxmPjEXhYSIhpfBMwBnYSkc4jLCyXATxmzyKASaqXQIYWSgORzo6JMcjj0pPVlwJAtNqHtRBP+munPi69oLUuKukitdF9KGPWQmqA+Mp4KToG5e/vTeK1c48D9v4QN/P1xnFKvu+KEBg5h/1qwsmkYRFZSgB+dsMPlFxhOhFe4PGsoKrX2JbEYyl8hSr3wpFAATiRTg87WXk6lgGcOeP80NZOOy0XpiG00scV6wI+c/q4bMD9yrzVfMw+14EhIlgvYhQuQfMRVycLs/K1m0KnCg6bFWOnWPRcpcwxDkBlwkZoCLikhBnLZVzBCpQHOuR8MeG4lzDHOOmCUEubv/qf5OVrejNfXuMb7wWxdjUnxX/8Tf756APp0HHEQYf6yK85cwwzHHQj07fPnSYMzrwLVbbuN14KqP+rayxQI0NVARVAIw95wv63ck0h9woI2yjnp/MYRU0kqfnZplHveyiC+GCu6R3u4u1ym3ym3uiZa7XVRJzrgMIrigx0wczU20+W/Guz3Ojm8RsiijRyVMixvlTSgYKcD4OLdTjayXP25aZqYzFWhleq8Q6R5RtLfe6sXFvs3tnr3B674BhkOuiaHNzeOM1Qxi6obbVxAQnLgGgUTHMoPGbj4WEwJWQYA16HmD7JIq7wXAt3GpnqS6AC9Ziz2Ra+rCM0mmI6UtEWeN9mhyQMZJDpoXDiT6so8sGb/3mlon4hVwpq9qzAZO6QBVYoJoY43BZGXsESxJaE57WwLWxVW2qGthJfIuns7ExAiDpY8CjchcG8SBf4/215jBqLoEacUEWvQIYCfAyDV8KrmEKCG81GldRWFJv7Wk8rVSf8nJJUwt1E3QiPzVPzpdiF9fHqjPoDVfK/PMZnLv2APONR/1j+5/0lMUCl7teWtL2F0uuQcEqZn7G4dp28bbkmNZaPbtqwcKTsaQRHIRbS8HgIqAYazQbkTBWJeAyHAeT0j8rS3jjWk9im4lltaD1DhMrPBGi0ECSNB6JpxhzZrUtN2Grb7EakC0xZX/X2EL2jLK8pHfPrKWkfWo1GqpEtyeCXvjmS9ReN3FqR1WR5BdUhPDxccf1mRhZZILCseoicm+dcrd25/rQTO7homLcFlE21VD/RxONR+N9gpIUsDJXipejewzmZyufJGk7zlKP78Jp+KdDCCWW8ElnpqXCozhdIZYc336DtTYq3OS5XWmT31boNtySKDgtCKYMjrm+7d1MHWUg2aYe3HEZYM+pxu45kQTEnaxcn5sPRXB1YeQ4hSk1fpKOSU5wZ4A2nwaELheyVUVXsJWMwRTcgFvKM6k6RwImGp2tsTglvtM2HSgZzsI8ynbFnitLBUQtQ5bFZdVv55kofC00zniVknEZvZ28KWgcKzgztycgz6ey7Z2maT0QavaJk13oqMdPANMu9JFIIVd0vfOErcPbijJ1blXEhbkZv8OobxIlAm5ChMVkDBnWdR6iAMunSMsUHnKjAhNH5dIJY+EhuvBhiYSwLjAzWHoGu8kmkZKupEIlIyE1dzTtJUWT+1OpiweApqpqJwUBTiRS9nPtYhMNME7LZxtKok222xiSMzKwEorgRpCB7ZwOjbdH6Ku2EVk4YdvWB6Pn82w+bOiC5VrWHPrzi6ILDFMcJNqhP/RPDQFdnz1eXhI3/Exy6msLFGTtVD3YwiodTQLJ6abFHpMglYERJ0foz+rb4avx++Kk1U1krVTVl/dZ3upX2z7WQP8OwR8g980zvuiCPjD6CUN2t61kuvCdwYlTfpSBncnBnVo4Q9FZVT/yBcOKCA7WOoeg2461rfKNrszVNnFwhpo74SEUibP5XLzK5hwtqq+Wb9/KU2TwTlFAMrhyqNslCGn4YwCOaZHmJNyY56X/T4P5AFdH94TAPt56MR/GH++8H+gBk9tEMMHKD5jeQJiplrTxuOyLQlSafsGTQNO+r2GdmDvRWbQiGcIvuPpYU8HosnaNs6QywANH4RvZGJdfgfb/KTYsQIWIpaEckRelsCssshUK+rVaK7lROI/s5rMgEzosbp3fIVrjCQy77rZMdl7vzrPKKxcVNhfr6n/GC7sdfVLwecFa3wVvw92jme9Qb6/esbdy1unbn+irg1FlFugaPPEazqp7JwEilmyWFTSBkshhu21fvGCRdyJ3KNGsHjA6tbky9+8Y3cYUjlDs6eaTlWRc2bUZX+yQHqlK2uwQ7GxdiZ0xHTUtK7TypGIBJLeMEjUN1Wdf2hiN3pybn66jF7gJhJviqmYPWoZLtVG0VA2tmUMysKC8QmICPLVj49sjCTB2TVtkj+EzT9A2twbPaydjRZMbfSeWdqq2KkQdKkqno6sqnVoC8AhQqQs0yrsIjVqCKPBxyppxPClB8roeMepxxhzKM3sCFuK2A3aFr3pahypqyH84lOJoWFdjI9xpGa7SOGyxrsEob6/pnugP5goiDZiRGyHv28VYJG1DLUVCaepEO9Qyyudtni17tf6OErseSgHSeOft4jQ3f0kkns+jeE0ip+mYXMvpz15g3M0ez/MhJpCT4SJImWVrsC+SudLoPNiLUvOCeY9rFG9MAwYoANOEiJ4ZcEaBL4yUD8iKnGqTBp8EbxLWY9O5DABqgQWg5PQjX6bBGlpYvaV3WvSMoSNCWJDZs1HaLzp6a5wMifF/B2Nch12k86BGGkNcDAs6MQEGmzQSfcwLab9Xf4Rob0fxHePPGBD5T5b0MqVUOvO4x0WeA3l7Z/6r7XP0CsSAY9biKfwGfhW+8K21pceysYH/9iQfw8hjg28ewzlaAp2SKUwneIYR8q8Ii1smINRY+ZqP/obg70Sd2hUCIQegY6Lj9qOlFcYRc4mSm5/CdUFgq9tQoljz1xiB+0oYggKKaFZJaFV5a+53/oVS2hDPiPJqqJzj7jIctwQukr5hZKgfXOMp7F15XT3OqeTXWhB8kDvZST8w/QBvvzdZVP2hJdZD3I1sxWeeo29oKBelVzI61UA8RB19QrsyPox0fJ0ZuPbkLlBeLYkubAoOKm2+qRxe561PWkOR8PTjNyS4oxUfdMzjF3jYsJYD/hUBsSeNKRChZzYYCArW2k4f7AXN0CEz10fALjHdyDEz03/s0KxDoPwucjudNPbSbjDPq65OjyLlByYOlpRHvOf0rXhO3EjqH3yiVtT18+O3x6mSTg+9IZmjHG59JGy3Tr+clg5z9OywzZ9twOJ/ygvbW1OzQEKvwIHu/5HSgvWlK90uU6kcTEfI03rpVPdDyVeWzl28Rds3CPBB/5EjukHNS5AtQH5Fxvw3TEguSxCS3Wj5XlQ4lu6wvU87kdpKjAt9Ncpu8cH2BI+/QPYLNLQFSJClSbTPFXZMPZOa/K3xTfgo6wwt8W/eJ+7MSswdEiPl3Tc+Vwhtq0VxsOTDfeI2+hlwRZ/4D6RQpzUMhKA1yigbc3+RnZ1aUeKjOLyrPPl1U5jGkfnSpt2/sMh2e6jFvd884Lx3koYD3rCqTnm4gADwE3ZUJywz+ZF3Z397gUmdv0+yOBnlMjPqFPHgz0984smnh3QtcwMmCCW/EzLUllw51199+MVqGBvr0jjuHDn962ROY2x3JnclV2Z09sPVhLOGLn99gBFq5Mdd1d7Kvpup1wyz4g3jVwz/onww048FZ/kC8Ap/SlnezYQosHyp1P5IoIKmRGiUMxtb2p7de7D0aXHpFP0RkaBztaOhqffuiZ+pdk1sp8QOI3xxDRfXdaFWlXD6Ext9bxL9Jc10ajgtKfBMRtoTk3k3warKQSV+tPBMaM7v2d6D0MWbowcQiyP2feZktk3jpTrgTWg2yt+XCyT0hFzK5GyZi+v+8mnt99Ko/9vajTO6QC7k94V3Lz4dH1l/Bi1Dy0v2u7m/3Cks+9w/1fRnA5Llkvhq6VTXdXZD5pP129WwnkDpfivt/Tk8PHY3xWEjK8toeTwpd2LgILHV+anFyWBE2/gsYYeuKxPxvLZey5P4+o3no0RJl9zYsxHrqjh+UFP82kxbXjxAV1w8ngayhliKqOKOIKgIaV/Z/XVAIv28VIqQhNQvxkc+8W6fmzOWlk1N8Jyn7dhOSiCJVM+Ok/vH6APPO2KB4l4c6NkHxwvauJuCKHZGqOFeniDAaD6+lL8kJB0yzJ46+CzQFGChIi6jThNslOiTeRmiW2Eo8agLmvb48z6L9TfhXl2n4admije9X1OGUkOWR63xnLYGTcOJTXcLiYXLylLpoFU2AS4xBVFWJaAnfkbL+v3sTl9rOFYKM393HVZ3S59naFcVLSFoidq2KDZsIk4Bvj97YHelJuNkSdiKFqXcqfmD39Zvy2eI7/XM91alPOhsx/RLWE4+LqtAinDFo2o+wzhN2l0u3y+K5oH9hIPbibkXZIt0O6xeUfXLt2bOXuKt0r5sDqN7mpT9fn2Gd4fPiz2tgqijlQjqHe4ouMNXUAzt3OOCVrYuP8jNooHqwJpX6gExGQDZu7n2ZPBoM+PZgVN7/PcjFTa9Po4BvT0T1gtdBu7IM1WJxrK3+eyvzP6T76G7KqcOSsneMn9hGUc6Wjf6HH+mkYLXpBmE5tyD30Hizfpj4E16jc9VzlFqi9dX1KPCcuImAjrejey+Wjkam37x7gnb2DPVWTr3C9MLy39XXl27cBM2ddUsb3eR0/rsXVOGfxAZ63uYlApNxO3eQPXeB/TVqjfB23ON2/ElqDjBv3A4LKGncCKIRkRI9p7/R+iho//AYTjx/WF6zJ2zNOzeUeOGovLpG3Jiki4P6VAS5xQWCDNOr1lCaL1nkRWpZFvjr4kpxMye+7M1PPtp98wkplMgZfz/c2P5+bDwHvz5Ml4vladDgHE4xmp+xpTzzu/ehD35hPPuAWXhQ0Pc5kzPBm16K+ODOk/rZJ6WNiw8nyKCEWFubxAw4hLkOuZmmOVUQxt6kf/YMG4tEa3Zejt7bbIyNn6l4F+6ll+bS4h1R/80ys/z39Rv3E9C7hPE3o/utoMEWzgz4cFRcb2bUAjTG29+vEDBTBAx8/Yy+nfqxkft948kbUM04berjzhaclz6Pb1c096s0rJor7LAluMiwObnI7yjtMI+5SHPcbJdlzx8RLRYpZh8bkeUoaJdhXnQBHjGmV+XK+5M0y0prQxtDChHJFFAGO/P/vpkonjdM4kV55fVThzqJByitAIntWpjEGA+codBcAkrtJfQUUPa5vNiCdhIPhSAJ4MUx50PooobYzpx4F+p0ab7X4mgkC+LPUF38poWT4nvSD6gVwZVFOfaq6ye/dHJPdXwBbednhFCe3FhQkT9e6KufsNJb3zbTiinHd45S/+Yqep+EhiNHq+GWjpq+jrdSQM6f5e873qHwUSfUvAkFCkDTJN0HvCJG3p2nDFjro6hNHkrjS9nl8x+KTK3rpgbOc3kjpVQpKGXZwkjdk23uPxf3trkfN3+ZoWhEKXJG2gu7p3GrF77NYiDsEvbd+ZHwAS8pzwwUKABtQS1HncKH3vHigpe7aeoSh4MF/X9bMbLU9uFBhCX+fXrE/2RBcsjh87TI7iVkTopfOsF7/kG0v1eROknLIrSV8aJA+Yd15u4fdN5WQ9XX4R92iDhUDgX5tTc19QLklDzCU2NKzhLPYiFUgDshbNBb0v0R8dLrD7vDXl3WV00N5a4VGDnX9U9h+mqJliMOYRIVclntpapNGb1xyX/DlONEHd0U+gty4lEzOVGt9WPw6AE6Bi1ptUXp048ulHVr0xF2UBKqr585PwwGHGmQXHAr6Qr2wndh3+CEsEH2W1rEqDOVJd57umf8jZCNRzq70wyeqrBD71t7J/oGqtPLSzp9yxSt2fyaiSQioPFPsiKTm0M4k4QD36QmtjWOw/gDdKQzs7EIGcP4FyuY4Q0kfXRIKJhfKQ3ee5uSkvzmZuj/UCxSFvT/2xTmt0khv6DIOMl9fiQqon+V7cN/Nz0WRm6E9a+dfB0Oo0WMOkqYqo+Pf4/eJ7oMe4WB47rqkofTeeOPeH1GwlNqeymdTMN0RGvef+gqqUtZjNiF/lOhIJwSv/yfpsv5LCr41P7O05XjKAld4Pdti78pPAXiftTj11Qf891KDA6ZLK5qe1GbSfTqzcnPvBNhrSFrkKZIkC01VPE3jHle2tjz+XYcSunXd6M8pysRmI1tLu4R9yBA08tMYDWOoBfsHAfplJPEyYsQ2yZs3ns9o6N9FhnvRweRpsL23NL7OSfTD4kkWDPmMFwkcRqRJDiVSSTwkiStsrxYJ7LcXZdZpXuIXGXpIqGMdwVF76P3GQMEUZbbJDlYNTf7ohxBn8Wn5P3b67dSFl8EvTLsGTqecJyTOau8F6Ez1EWywBzwGvs4XPZR6OT8JS4NFRIYVEtAIOBNPfG7CMT2VVwpX+j/znmkeMSxD33lC+VXNk9Y6C0yzonMidMuqZy2hXU6FChGUEg7ZSe/RjsnPifC+M2A3fxjxZWyeaTr8NSR4tGZrYGy+SpR0Pm6m5rbGK4WRnzQ4igk6+QbG0PMyqyPUo8i3W92FJRy9kkMh87exj14NYVXX/B8qITyK/HrCPWXc/9f3P3CFV67d8R31TRTjymqk/n55FpCrYm9r2r6P/4BGvpXtBJxp9Z8gT6nkp0eX61vmXx6F61obCnIuz3RSyXrj4K6b5mewQurHy8t9fTb7gZ3dme1be0VdwzdCx4cbaxaDZ42uaY98iDp3sPCjp/r6a3d/UmqPyJKcgmHRdlZ4nrkEkqKs6mOSrh+l2RTAelOu9wpLK0PMXk3mUZXRVUTiYm7Pw5enD9p5ZJm4qhzoYluF92dRPdQULZvc6vJSGPJkAjK9a380f/0K2Z+Vr9r5dFE74vyeDN19xp98iX6q687M72KM+JZYkR98jxv/Rp++mZ7fhate/e8p+9VcbKlmsdtw3KBp2Jd1mZx+9vNkImrifOw1vEYMjlSLkOGY5yN9X36zYFmKcOy5UPsx/cHqCpeLyqryshue92u1dLxKjOr9Ha+eHx0zHZOb6lmQXZP6B6W+MxvqF1RqLe1XXiwRX7Arl5OpL+tRbi7XZ44NDVgrvkoBmRrPDXRbao5m2qI+aaHuntsVz3i1NTCov1svWrDixpVrjsEOnlFm1qiLlVxsYEpWQkyVidNrl5Wzbcm3a2jbS0HqSvpqMg3iZPg/5YyRZMXapEXHuyQhLYLEv0F/LRlR5TbObebXSQbz9PgA4zSL9KttPWtkXnijF2gk4GDi2Eg8gj4u12JEkSgHntpn5LpYJeUae+QlGrnkJKCOug4u17TdnTSM3By0dJ1cYMLedw1aM0sOjuIDs6iHMq/lFAlSC38T+Acj662RoCYjr2FvYufqzHqSk4MCUwIcrDxDVdQOdr/CIyLilpOEGSmouNuZmsZaqUtYUcO8w9JDnCRuuagyW7MYuqo7pQXFh5ZfcMZ1Y11nvW11K/7KwMsPaD4gFoG6H6RCjBDRePk9s4u+kdlKEkMM2X7mdq/3JHXQksc8O9hmiqa/Ckg6GXaK+p/ix2Ab4fO+x79jnpKo9XOUGxceP2mnXOSrpykNtGHwBEK7YXo3L6exEU7aqBWDiLX9jHX9Zb5Ftb2JKfUd98yik/IDwm8WRTvF5NcFOCfVJgESA/izKwurOCvo6Wrq1LjXHCrKiO1/DY2A0VqR+5V3xmcvBPmOpWfdElAnxOUu/E7LAZ2meoDjusViGztVGjfSVdtVPPUnWFj/Xp9TEAR2F+ITnbycHH0OBNHYzJ2dOKR1NT1+DDVx61WMXa6pU3pWR+22SvkMu82xbQPsU1AqSDy8XR+OX+CRnz+x6Ysr+wzyi13Mr+yYoRLd2f2p5ZhqV0qbAbZ+tTdtf5hEhuriwK+XcJ7OKD79cOi4tmHLVh4j4aM1k9W3ugrD3EKfuUvbeT2mGyepGfhmt1iYG7Zg+rHdFZd0UjqvxWoYOA3W2gSrubju2BpkRNvexKrBtfkWfMmE8X8aRFh98W47PqBmvTyj/2PEV6Lsqym/PKhwijVnM+dHRmteexpxORZctzAu9VX11h03j/bH56Ojn2nq9ssRxIR1y+V9Ld+ZyNYixmrSAgsipeOPyh++pV56D1X68BzEOU+HRoZZLCkV+HyZzgzJPZZVNCXpJUFmPKbMpM2ZKkL+G5mvh43+iE8JXTV+Mpvy4Lkx75PQek0heD1maCbHQ+/Pglwmej1CS67T4N9DYo/WpfFl88YOSr487AMTCB3DaGuqGHTVYcpGxX3NTIf8N6IFF97YcPmq/aTLsq+v4r0Exzemlw9hQ01FffBvYj70oRbvDBQ5PwWbMDnCstpq/Ghp6xEk4yNqr/5vtmg/og1nryRH0eL1Ek3xzBukcmZwyzqbyy3CH1fJq/BxdbpRgt4Q3fau8//8vzhLw88eJEEluJ/fa7dHBa6LVi5dJ/cv/h9+hq/zo3rb/ojvFa84x5HZ/7M27ovWCZcu91bkdJHLF/v+27521Xm3h/nGqZet0bd5DUWdCSheQ+/i9HtV5iZB7y4IxrlgYd9D+Xpl7YvA9NrVnUH52wHe3UVZxuyk4NQi7jCVZdTiTiqoOBys0Gw4J6Bk6uWflaknKS8khRRz9FBW5ccQUIV1aTh7O0OOMLBnvSV3E5Pmq4jpfkVSbFokqddZKWhlqqjvh0FCiHvnLV22j3ma8YemKhQNBhbDpQXo7+OSCloUMSw+9yBVqOZx1b2RNcPSj6qWZiRrUy1TqlZm2ioWxuBKnQKc4er8vixnDqrAgHbzB3Bwh23vhh+8SZ4xX1UetpeOa8+IboD+Plg53NS188GHJ7hoRSeIc6MzkjO8CFw8vyhXdRZks9576UDBDZ5JyQnRidAXkW7pku85sFD4kmVtqm2KZWTRKovjfOS841S6SDn8FwJVWqFNycZKhs6inLCJQPqDM4GRWUXJSdMDfkI6SC21DXM59IhZ6Gt8zUR8UUG8G/RbjoXGsrXFIqlwcagpGfT0O8KL0AiDRYUEg2kfz7deRAePpXf/U56R9TgsUzUjKklF2RXpCI3njDn8UtFi9taSVvIC1lqP7aYapzSvIvD4YCvfH9Z0wBkUkeuXn2Iak1ya3xMRmtNUk1aa2xcavj5XjnJJ/+6q29ealayf77Lde/8VKBrqDj/kT3zJ+9K3rTEdB1D5WUZ8gmH9/yqkE0WihoVy73I6W17Vi4sL9kvOi3xhmtuMJUjSqHtY23y6rWlv7e7Qx47nHM8I9p8r4Py1t9AWZ2A9IzN7EKfyNKKGJ3ZN5uoquoNTW0ZzxsxTqYBkfd8AyDmV/QvyaiveNGr4K2hYGCjxKbDZuem7FQcHBNfHOykbuesw6bErm+tYJ8euJ4ZcOajVozdVLRtUbi1p1u49ZKHhb+5pnqASY+fWXqQNUgE/AyR1wnIKvEJKawISki/k681/eTVCJxg4KxCmhs+WeqFGbAp8MQFxjhbhybYWvrfAOYlNTlTfSWu59pSp9kF54QOZM20Fbm+aUoKsgusiaSyKLS9JILUeKYH5RI+48dTEHYZdYHlLzWijR3fWVZA9cW/vMW+hJbd8ollk5yf4QFwZcy/ADhg7vV+4BK7BhszKTD+QyDdudIn906U+5OTas8CtzaSYO/eRT+Kcvl1YUWFUs9oAqxgDUVi75Oxf+dY2ik9cs8kglg3AUg5//fdlFtq+hFof00e4Faa2g08+MFmuVd2638AB7d0eUnloF+5nijzlcF2LLV8NJsnwDgIQuMNje/SWM7US33UTwMY3E8BnP+Fuub0EcBwJobTALN9E3qZPF8Z99kHyv7k6jsdlJF0lrn2HC+iO9ddtaDIjgZwqTEsP28/553jgiVejBOvvoNXRgME5yr3HaSDuKSzOmK5e2vlomZdVFTMAsvEiMTCf5TOCnN7TljiWLj7KlApLdEvvqSDBks6K5eWu9tX2ak8UOK5StnrHaB0UErTWdpac7Sjv2fj/px+Y3Z4gKrFDV+rEXvax6ShOeShd1DRrXvSMdIOK7d7r2HXJugaTx+1yvc9hJHLDRDrQDtgOF/+URe6kaEb2TDVejPmnzXf6yOgdFDK01k6l7uLU2u52qOiW3cL0EvLElikqpFEmbGCIx2ZFU3jWKEPchHEFAOqBxZJpBWtGeaqGiijTFFIX7EmI/YFRJQ5Q2QKCyvlctmax+5iUDmSQWVrMxIzILYImtzFJsaj+45tfDzOJ6o/Zbiu/0J6ufJAYdmonEJA0nJQyHKLOcj0WsoOyNpUFBsQalD4xekVBXmpR/qy7RvcW8k1kPmMAxHcjQVVXddJhaNdVl4516FRDbAfnPJCGoZJY3WQS6053kfXJ9HQ5YJwU9Om7Z0MwCRoJE91oDoc0wONFYtr5Y7JcGtA76O1oL5WlDdkePqlhusAmRdygDxkv6g5OA4vyXIlGmsdL+pjR5Ll8rIm66RKa46nOCNKx2yQE8yAqcwOgBPgkJOSYU5W4DSfARhmZbWo/IuUk0kmLeSoaJZC7+1Yrgzvlcc+PFWVk4S49DIkVUDqnANgBRyeirQlFCpsgbtgRa9BwqHCcm4Cb70GVgR/EpEWLdVbK5j1ylQ0qX7BnIpyYLYGDncDYo1DuNeIv4obQqoagauOfykKKRlWEwuMBRZLHMF1hUOmLHP8hyRRqWak75Lji2wkAKQiB+bybixqto6/ux+tZFw10GQcuK20w78nS8haOYcbJRuYBml7cQTAhOsHFDAAHMPB6fHFGKBs074ZqvCfgaf2kc3ip98IbLVCOXLnmZkiRtOkxzZbL1j3XG3CwXosELlxv07f/9SiONZY99wazyepKcE7xNTeV3aVXfXLTKeik7sAl/fRnORGYRS9jrSwGRkvdPUBolgq4OHGp5viCLGgx5LWru7Ifp4aO7w3eydj7IVBCpzjrmW3SLqB38XHLQ2MB6wusiN6sIxu50C997t64wV4eh7nZSh7V67ajJmE3eyUhngBgksCucHIFgU/41jscfxK6ENxXAnLy/WTjx5u9uY8/YyIV+AvSCf1H1jZd5UXUE3Of+cnzkpn0XcWaJds8bPF457Lyn5Xns2jy5ST6ssx+M3Vjd0xjtaaBpAPhHhv5W/m9Y10/9u0agbzU5lmwVkNHf4f+A1NFH2oXv14FmiXbE08jn/Xmu0LfsuyVVL7GbEXuvkW32sw7nGgPy4F47zV5x6Yv/0APgby0S8yZOuquap/Hmbxx8TxGmZHievBiGeoo1DDtOhNVD1lc4KHu6ZgvkvutcD2a0utuQEbto7hRd09b78opu1TNPi/ZMEhKRuC9Yl0pC1Jd+e+tBxMQl/XhJTiNgg+onlxO0OuAttrO5/bd+pO2llpzrwzumm06pZIXRH2Q7IiEvafBLUQ1FK2LmwxfVxmjIP8RtcvFeRjq+fZvpOrqx15PwwtfszNfbueNpLCvlyG001hYAg2hTPkJFYZrW8ky4ZUAnWI2+vO7YdVtVLgR3XPl1nX2WLskDwVVAf/Wa7bywwoueVq1xMaSJbfGPXIQrE4xLDAcnAPMq6el3vqMxK2a8+e/Fs+hgTOLRv35bmywELXiPB9Z/bCa9qc5OQsqPT78q4Yl2+33dYWRXbbow6Jc50riYQa4sbKqlose3RV+yzoIZpv1NmDFN+4282V/PJwDa1yWmOILG+7fblc1xjPfWNGWZe471ROUp42Z8gsm7CnPHDhOHH8VfODscJ+qIROD/FDZREPCGcornbORix3zlSc1bhshm/3PwKMK5w2f36P1vZkmX9QnTwGAJ/9M5MQAN+LwX+Orvj31bE4qgDgWBiAgMMjd1qAY6+8Ef7JnxgQV4c8cqZz4qzfVl6vkjeogVz2xOKODVv7/OuLgBhJHrbyh4/T08zZiqaRaFbDlmx65U164DuxKGAQgsAVDKAHYkAHnGTXeAqt0q8zq6pgee2G+M8o1gdvoTXhXL0wuYRlLxmTlSmWb0tuqF/lHoPdbjmzWKwjd87ePkpcDbVGbtZs4z9v2sMaZFeTiMtYf024cLhKFhOdckdNskmv7kkBUum9g9ZnOXX6eDgVlBSrstdYDGCPd3hRgem4SbEchdl8zal1K8PnNx8z1f0HAVXdmeXKYhgH7dFix5m/eY5kPPH1cw7/Rg+GzSAyfsY1Hfk0QtbtwnqhSu7ozDKRWDfYrs/8ix7OJVNzTQiYqVq3OBEzTt1MkVM6wrfUsRC/hMkAZSVBfbxlUjZNL8fw7LLXNDs55q27WafPTY+fufJnrJg1KGctCt9ERc5sngcOonWaQ4jcw7iqcHubYN0SWyqJRtkzdYeGSw76fA+bZpXac5ynFQ1N/vMKC3B0TnE/Df7/5WTPYMyXzgryLg7wXNdUtp3kFXnO4aUTAcL5xS57hlvwHBFKa0X/sOLJ6O4zhcJ422vZU68vfCpLTfFTvO28eRwBcMx1WQXA4gEb0AMFiAIPS7FTpwv+/yVhZpGy9Ob27DN8jq4T5ivoTJO2HZj2mcuCTIoyVphaJbE6KWAkYJFjKe/DtKbHAYz1oyqmMSNhOjGZKTQ45DsWNIj3NjiSyvTYn716NZ0IdIDqvQ72fl3kK51z7qVWq2lUxK3CZONmYboRCNwmTDQmCZODwNTgxmHqtQZd4TQ8JHhgMAW4vbhBmmOtwfDQWVoaZ7AbD1lq9bPUIhOSRpoxDdrktEh/oJoGya8UoYCrkXNscOO1APAg4RYgMCWRVd7FI4SHQGvwIM1NrRJ3mop6nHQlDLNPGh4pPBTaoUKTWqMFqAX9MQoZGQLySFnYmcAfdXNj13UMF1hIUQO4ty5mBIJd+QgMetnZ2G42DiPweLgzCnijeHpGwBs/NPDJviRhX4grZO9bmONmXGchH39X19PN0Y/pHeAc4KWtoaSia1i9BUWFRAx8HBICyeAaXXc7gkmVf4BhPl8m10DLK2JEaYDIWyUaOLsGedr76+3AxZSQfcP8TVcfmgq7kkxtw3xGydN5TA1vRyGm4MFzksGoGcChH7THgus75iSEhf47XQAAAA==)format("woff2");unicode-range:U+0000-00FF,U+0131,U+0152-0153,U+02BB-02BC,U+02C6,U+02DA,U+02DC,U+0304,U+0308,U+0329,U+2000-206F,U+2074,U+20AC,U+2122,U+2191,U+2193,U+2212,U+2215,U+FEFF,U+FFFD}@font-face{font-family:"Roboto";font-style:normal;font-weight:300;src:url(data:font/woff2;base64,d09GMgABAAAAADTYAA4AAAAAbJQAADR+AAEAAAAAAAAAAAAAAAAAAAAAAAAAAAAAGoEQG6NaHJB8BmAAg0oRDAqBh2TwNQuFWgABNgIkA4swBCAFgnwHIBsWXRXTzZ3yOACUXuICdiu8uzUldsU4ikpOjeD/TwfcGCp+ktULVHdLBwGxOjrNdtrp2Iedfdda2d36Ln4KN1GwonIEMXJTmNj0xHE4nbDe6JGNbG3dmXyOclD3d1Z++VF/iQIJxPRHfxnKjzIo9oTTIs7AcT5qLg/87w/qvq+BlOZMspXABiM5wbsbQfwDmtv9xkaHNVBBEWmJEqQkordRI3qwEVVSrYgDBJEswQBRDDAIG7GSqzXdvcejEIIFdmVSVdWiRkdGdXzkC1Wer/by7dyenvc2uN1fnmAsqJgVNmYhPBiP0Dhqmn9oG/7rKoALCzGth9XAS3DAL1Bt4TV2TnuA2QCBI3yqfticSNtfkqWqGQ+lvomHYuAXmKIc21E2bKr2KoGwOtZ69/7d7H+GQGQGQvK+SiVaq3R6uZeQfYcVzxcPMA0knRXt16IzLc0hsvvFff6Pvfqz7lojaecBD2hmvxcV9hYd5O0dVNRBhQPe8ekIp5SJA9TRLLKZpVu3t75ds554zZf5gsyU/nPkIHQQrXqWZmdX7LfuJBOsznSSSTKe9KBHjJiCxPtss/yEesAIMHs7++fU4UP/daImsxj6th4Q+Sld5+HJdQpUy0xEysPbidz/0hx8lVNulgw+HAxKNBmtdj0aiMz+HU4L73Jd6frtlmxn43Rgh+taAarXUBUudYXcKCyjXPfTfUhORKT+ZvX+Z7otMwQJYiWIhCAh7X649EPUHES7Kw+TPcSppVOtECrE9PsIAhQ2AKY8dn2SBoQuV4joLxCJQCQDkQZEphJky3X2qypSBq3TNSiz5iCWwiFIOBY1SNCNPwQCBZQHikKgRStDRzAoQjFKUHpwCFCYNA10nd40tgahL6NDSXbSt6EE/5N+8ggP3AAdYHttMfPr0EBgAOzRQQcFREXImKBSc3SgYFr+d2Xbt8Ow3VhbtruorWZ7V8/dsXZ7/Ftv3EvPiAq/yVcjdL/to3iNwSVCxqMPlY5eyU9MsI8Hiuxdh/OrKOdDlfWxfqJ2q4/plX9qXP0RXdM3A2fIV/cTXfmXxpV/FjqmjQ07xsyV1jV/G3TtXS+8sVMZe3XXFWK8hGO2rFt7Hml0w3v1rbZZL+27U960nG1bUk9YY1sxu27QLd9ANZtyTN/kwt4G0akkgKNu7XI261Tv7PZ96M7opY0tXunEO8zYi9hjpaHR1d/q0/S+uvQxmj+oL3i93TFvKDUXrGwg0jcDZqhhdmGhM2wcpaonV1ruYwX3e5ujWDwfcEJwaAIhgSxu3GZCCE1FZ7htxaKVn1CNV53jhA/WPCNHHqHUeqefnfcQe6Gcpufr2v3+iOiKbX1Wfkhxdeix8qSDE+yxRSOnYf8AigDBARSuULSTm6nRkSCPkRINXHTpE2QADjMmj7Bg7ShbiHAkj3Pm6oQsUkyNRqc0z1zSkk+kfPeHTQBJwClYBAvuYUAYwSPMMgOvsHUC+HCEEyF8MuMdgSPMJ0I5wzdncxYlUpFDzbmckxjFqEmKfoxkxCRmcmMRCwWxipXC2MRJUVziojLucXcpnvFWFd/4aYDiNwA2J5szlAcn4MMFHieBJQaUM0CRBD85oJwDiiLYKIGJMhipgJcquKmBnTqYaYCDLklPnwGEEYmwYAnNCnSsgWYDhB1YOADDEQycwcUFDFzBKhkoOUDJHQpRpRqfGrU41YnkUa8Bp0bwaAaPWySfZSuwVo8myoYXUF7awWjXLmavvEfvg08Q332H9sMfFwb9ezXAwIwgAZKCCmAUKSgJFGBLogQx0xCanXUIWg45ckrmmciUcW/uRrzwnVmkmP1IIKWk9FIySksvI6OcsIKwkrC6SA2pNUVqSa0tWmfSkrNoPaTVF28gs+HV1ahRjRvXpMlYoEEbtZ1p0IUGXarrTt79pBnbpBH3rFkvKr1eWW8Rxzua9Knsi5RgiG89/Dj8HAhzISwLIoegPJwKQEVoamDq4BrwaQN13DyZZOMgYYQI4EBg//RmFJnXzLKKTZBPwCC07Ez+ucDxxY+RQPyE8RNuex9KEAL15HT0ECaHIDDBsmM7nrwsn3zSiIGgOOPdKfFRVOAOSyD22LJHgAACih8Vgg5pzmxZ2s8sX76rQoUqVnylERNEAVHCchhWEFAEFAFdaFm0aiVqrbad0H6q+46aeUoBWG1XoWnXE+1BJrGsIqxh1jBL4utw9HeAyIvvO7KvtPH99X5nO9CetltkjmntkXoa51iLpyxSQBbu6zivq9RKqJqKRE7wONIv/R2d7Pjvxy5kG7RjSM/MKcCRrNzgU8Y080VksdjZeI2gm2F75banc+7sIakD1shcTgQyphiZqbiJb+j8TS6SddAP0HGCW1ZeAcxp4qRIkyPvHAVKVKhSp+E8A4aMmS3O81uxZc+RExduS0HL8J1Zsuy2O+66Z8Wq+9Y88NCGTc8898KuPR/t++S7H3765TfYfzAkqCNFjo585ffXFy5StFiJkqXLlitfoWKl6jVq1qrfoGGjxk1aZJRpZllklW32OeSYS+55hY8QMe98jW0c7VlTOOZwrCaB3RRwwuxHnuBnxS/8lv6kwrpJR9q5bOimcDGHJXe7DmQiOe8pg5fYwra0o8UGOZyTPHvA4JgUXKhDI5qrEYZYMbB6+Q/wE7/wW/qjJS3JpqH3lezsyXL6AOf64CIMuFHCBrn4gD18xL70SQsNUZyCuGTcw2FYHJijWfHQwkV/XrsoGMAINqEHbrnOa4LXBurRwD+APXzEvvsJLbR+GEeO4CgX7fkpIC4ZV0DUDm1s2MMe9rCHPexhD3vYe/49CKfZwYYpf+m1wJbf8VttOG/Zzjp7J5+fZcB0oc6yxKmXNhMyZ1zTrKLDip0WFrbzjgc2dJE55yBeWoQM6PL19qPr4zRzAs7Aoc6pEsdE2uTJnAbMlIZGZT5Qn7t2q5YQFKEMVdd9p8YrFYE09hPWwm2q8/4obRgxSXzzPoH4D7CBDWxgA2vYNuDet81R7LFJnLoRiOaqeoIujuGLK1s7ObYkz09KeOjOJv1556JgACNYwSbadnY5zFuO85qLeO4U1g1fjaBZX85t3cYd3MU9rOiv8k2v8QZv8Q7v9Q/5O3v4iH18wg/8xC/8xh/9b/7WP/yXDroFB69DXPQmHXuPQ9XCYxuR2ey44wQXDX6qQhzSkOXyF64AV7pxFaDGjefQHU/wnUukTVAMZ4/TvIVInGfQWPNdWvOVx3yEdnym2H+NJZ61g3twsVLBQxUXdXx28YUPVwRwRQhfFCuUoap74QmBe18Esh3WRlGa9dzD+oPn+OlgLzfKjUpjZCj69l/o4sD5NUvrd/Sbllon6XxgZnyy5IdatrFPzUxGf25pJJz/Cuows1C7uJ8BI6bmlLSZ/lYxgW6ALkyBDjwrhk2/nXnilwHBkPNVYFAApP3/qM2A+pOvtwdEVrJftOdPgREB4PxTkBcJbP9bFArmvzUyhn+R0DE27QWWZBYZDQZyzzEuJyYnQhGxF1MHZhHdvD/WbPZqT3MKQsyFsMCidiXcaTgMMf/vUWwxGREjTLhRY8ZNmDQlhPzru65Xjz79bhhw06Ah2EsBDTEWXLcCZvxmETyOABGUNm3AVUOQZ4oqSvhm7o6bs6ELWrlAyFbQ1befNiMsHMXSeL5DePHhJ0BQHDuCR7BwzzVvIahHI+ejaXoP0Y6O0Q2CjjBj/pYMyLXpn/Waz4SOAk0TzpL8qk9q7ho0wx2lUx5G57EmugxiCGTQCGSkqcLkdSS1ZDq8/dq9bVuiRVrgS1HrA6tseS77pbfkl3h9r+1arPrKK7y+DealV4eLqxiQ/3mfx5lPa6gJjXtMoxOhMMCeaT3a1SqRxQedw/hwYAoYQCmJhMVKfY4V+bJxMQRd4GW6uLt4ZC4WVwt+hbJwNmoxTjhKANdXUdalQ2VoDmWjpJDBQ38ELcYQ+AWnwmcWNC+8yUa8lwF7KKS/IX/653Hz2Mu4zazP5dIYzKqlk//0O2TTj5BOP59DTN/rojDsf8PYj+2Ma7Du6VkOc08lSQfTTNd64yUep9JeiJ4+2jXjgW4x/EZ664RXRObXTvmuObxQDeWMEMX77ozx8e4FeCF8YkkQhSOkfQW2Z+e8JBdbb/GEVhKL+8O2XDv1LOXcwHSoypG59kzPydggZZPDZe6e0mfXM7Dpj7HqsSzWZkNFF2fSurPjUYxhMtm1ronTxNx3sq01o4FqrQ1y4a7jlgnvt4v977x/gXrOwpAij4ECVWzUaeChywAfIyYOMftTLwF1coILoeISCyQk46w0SMqElOwUaQjYoCTanNngDxnJhGkkYYGYmhMiKRwAV3BB/VAEEiTbU4iPYIrDChdQxxYpfW0IE+gkKBIwIN275UCoUdfErxALwFkmrVOnQk/U/Kx0/chMHzRB/u+C/2DKfakABzkESFpsGhwc9FsRjS0LDYnRhX4+4CdFDK4P7B+ZOPU6XTVm1h27PjpAZGJVwzVbj+vpQtBoNCP6+Aso3GPcU9wDTgh3FCeCE8XJ4VRwOrhwXGfyJRGu/wcH/+YAwBo4NER1GTfnrlf21WU5NYx9jhPAHb5gWZxy+xHk32eb+Ew6OhNAUSADueOoHM9d2P7t26It3JwN+5Xm/PI/yMipOSUn5jhvn5N4kX/279n3Vwm7XYcA/Vypnah9nCvLXDH6mfzQREue/kQ+ekmSvbRl245dKVK98tobb73zXpr0n87fsAyZ/vrnvwMEYHMQQKAwQOUHYAeEWYDoAyAHKL4MlH8F4BU/P25lZBO5nvWp0TSnRhZShNOgEsA2gJIZHcSpTKaOzSW3uLJxJOJRsb4ucGDA2JVMMKGc22FYB7x2m6wcmVk7QZgyKAaPkG4bHpiRx5r69R0J5aUBGQ63+Jqr41C1n4xDCxjutP5W7BdGC9GlEUAr/WsZBPNOReWiSO1D0ivPPfhZxjxRloJCwKGm7rMuy3yjU7CJ4TTNSEuN+3xEEx3JCqsV+HzexgL4h8gqY8e9x5whVbF4YKoc5LaWHOiS2cvvq2UfYF6LpGGpZyvOVedAKhcdKifC3QpmWD7wxgrAglwfFbuJ0VJ0qgtQ+shrdfETOTxuDjb8Yxy0DyjjpDhPP4U/mXwmIfU52ODG9wUXKaHXd0nEIRhIpbtGNLgUVi8WWDiDDJUBWqeliYa8+ovT5KUCQpsLlxCeukismxpQfWjC7CAAcZhX2qZ8lrCpJZCRXNnZda7U3hI5JwHp8n+aGbiczXFm//zbR8+U5dT8sIToXEI6hRwX6dQE5dfgK8jX60AtTk6GuV8pvdR9sJiGaFPd85NtsxVxuBNs9lQU6qEHrkU1F70a9/QSffPhMt4U9+lVToMEJCwjT5MY1caphIeewo5RVh6q8t2nT7KJO3xOBwfLWenaEqDCLcVwj971pWELJjtDcw7UsUUSBrKo1kbhxG+oOPahHQatIQXNFTMnUiZAszYERoj7BvaI42Fnw5IA6JQkuLyDXUE2xcmEXTM3SWFnetRHZD8+6JB4UkeGsT8LRv+6ZPifYXvqDHYvj5+DDAWOno1U8zymkODB8sKaZ5IoV5QQFmc2PE4oSiRP32TNsnVfsFHOIMKc/u9O8mq1/mva+0jwrXOt+qR/evoPnlzWSZXhMcbvWUk7JOGUMj9j8QRbPLY9QeW2vQm9A1fKP2liTzQWacnTEtDJeOItyNfIxKX1sgbIV39S1vSmQw2LXkvb7kJHZNr2VwGtCBB133jwWGFh6ZvNL2p5F3bRP4ecjt1Cu+uwNxpN1GVv6fpkM1B2RF34xZR2fOg4vZTOACdOKDvrWXQOZU2CbotpaulJz5unf+ZlHahxxPVG1reoNrCJABH3t1lau8HFakvtC49Is/0NQQ+fM3SbVeoIKBMa+bj2t45H6x+IvHPIuynJxXiO1JVpRFtucD03c4eNZy66RDihjA8CI5x29DSj1atoGfDhER0f0uCYh3reJodLpgtn0I5qQShlcMWTyKTipS6ypDboZwO81Mb6KeLuW7GAzdRnOzsGdNEf2DkFE81QuSqX3NOEKWUwMw601xIJ7xnNswUUI6JLmurUNOTjIrUy6nBKuqOoBakMr628p/zCapYAdECr+aDMLHnlI/Zzg5DjV2GtPodd4oFbXxcNN51YmN4ejjEEqfFlQgWbnDUzlZJHLY5iNWMMKL8x70IvbPU18ibD1DdQllFVmAPePSKr6w+WS57f+JJrR+nAi1bLOpjZVkxudgehZMfQzBpTrLCnCoQdkKyFkiHvSK7TCd5DYgDe3NCcEF9nx/ZmhKsQi4Fsbpngg9cVDiHXRIwv6LA9Gs15g5u0Y3FT7Qy9+sqhg4Oh+7vY4becOKSFfmDqjMnY+NBBaWMXnlNr+bjCKcalPg/FglxVAGu6R8K9ZR8L3rKXmUotjRgmluMI+TXl4ysjNOOyFxswaYw0ZxqbmRbpKFLaHHRcNOhVakqHyV/wpY4IORJPoedBKF7IlDaXy4cFwXJOwVO50SgiCzwsyKUWM/92wwEfeuBCPuMoD+OYew2uN8jhDODqljAJgrRiZ4QrNrqhxRWrp8tHIhhvjpayoWmngCjkeuYc3hyEmA8omSyP416Tet2/H8W8UpGMYi2FqOLwlYlHT8upYMVBunnRBCzZ8HrdGMKdwUNMHv6a81+8ZVEjB8ShLDcXAXpl42ua62iSNvqao8LD3XTaCbRZUj/FmbsO23iQqBnnhz6qPOH07TCTOYyYG0phP2Y2mtOl36R9x2/GrYvT2KOzfCWQr6daawbkKNxhwWlUNcUhNSy/itdov0kuhwzKmD5fDxH6pBOlxVIX2mf9azej5zQH+2bvINjdU/u7CYWMSiKWVJjnVqqql4yoZLKSFCypkkRKLDZLugGuRdKzywZjqz1qicE0DksW827lH9oUrZjIUiLV7YB9fmc8KwZloU2KgqhxJH9fzfWV1IW5kYBKdcuyqgd5HeKlYWLhDgbzinLJ1b5kfrMkknhexAn7AQtsJlUm26AUAlMeW/q6Gp4p7OMJLyfOTcemaFyQTllGyDVBK4IaaNvdZMpbYqR6kLAdol3AfK9udJ0/07BoO75Tm61bAqQVoTELVigLw0xm2rRxBQ+5Zr/7zum2pIgzDilDq/WNvd7gyicLLxFEJSMcSKHLg1ExU6IfK8wyyN3Fe7SPByx7sEsH+6ZIb3IJlvUREvJ1ajw8slTJlwWknUlIkUUO9/qcvf26to/Q05mTLc1+5QgYqO7LOrnInYK6dixvKqX0WhfGiD0rt34OtbiPzW3wKIbcTtIkS4vtbfXqaH7zLT1VVVYHBLzBQFm+Gn588D3n9V8IrVZm69AADwBP4UEAqX2BgYDQ1YF8Ye2Wfh+vVNX8jKmra5pnfwc8dLLl4UTfUO5PNjykgYv+cKqH8TX1F2FMoUgoR+Zw8UJ7R/WeKvZdBZ03G+Bl/G63W7xneu67kHojGYGCJKRYOE1OJT0vdu0GQdViXa/pxjMNGNo0muAMGHrOJN3Ij8xRWw1oxCUDcUHDoZIgwB+iMrT4iYwa3xYRXjIeBvZz4dxK5qhpj3fZJLBAp4b9ShXjSq/vzg5cHJYD/O6nTeu8EkOVgQ/Zcf+xU+ouTlMM/sl3VTyjqqBk4R2Un9On8UKvb7KqaW7xCusicfEa+/iruaiaxoHcvNoSKrkwzTWylmRa4tO8NN/DOkoYmWd58nKGXN1Ha8rrKQfs/Yqk+ss/6y9n6CfUXqZSmtqy3LEuxCg7GzzZ3cDFm2xt5ROFF5s6m1Tf8b2xPUv/PjbDjc7VzLD9TeRp4E4Mt7X2jvD68cpQSiI81FAKBLWFbH1Mmy0q+7ors6/UUwtasuoo+fcciwaDfsZfnn1VEFtoanLbLuzul/s9ftTh6oiOUafLxtp6ZQ83V6bqypJ7a9E8EjFpLUle/ywCDE39fRw9w2KB7WQYh7QjnVgZEbKNHgddemhNMThsriWZ2Ev4oSE/fc81sUXUy+CwcZzRQ0tSGSBqT0llaxYJJoeNPXRbT7ql7dxxS2iXdNM5bBxrvGYRVAV8xa+N/I/hjWLQIT704dpxOLxx6OZJa4016VuS1moQsTUUPPQy+mVPcM9KNCSx68k5RqOSqiJi7wK72TPCMyjQPz5uflfvqfkSZFEDRLFnSXA+1E3+hcbT9R0llDhGbkv1yfqWNPpUFOZ340FLY9Mv5MvRX+30DfXNCPyEfjJgcNlFwR9v8IBggfPnc5Q8t5v2WQ31ecXXh8qiKIELS6HJ88/v9RHjPtVdL138B4JyglTvyovmcVY5NTUlOT3N6eSQkMEFAmV26+Gwbwot7Ez6yeyBhKEvW0tJ696Rg1Ry9kRXRVVnbXnRYGU/GRQS17YIW8Hk4Ks64sf/Jp8+/kRIU94JHr3Rm1kPCpF9F25WM/PmoDpt/bsz+m6VYzSMo6StIr+6duZGfffCJZtYcV11kO8b8mXDnJFl51C1vOkYNv6S4UGe79ZMXPw9jOQ+Od2rUw/9mBA6nYbzJo5OfqzO6dzo6Oy8A5ot/qI7/rGiLYZqxBHQkFcOSe7xPx0rXj4WN/Tv8b3Vv4+GqYUTceL+p+N7QvperyW8fYg57p8x0SU5doXvZnNd1VATyks3trM1LaO9PjmhvSoz/XI1KF5oPO8yQgyijsR7v0grCHw3m5T05IusECnLLNuBD7zF6dDsx2wnyaN5YiG6mwNfa/L6trvaujbhTt6NM3Fy3RQPue6YOFAPU7/gdwEjOwN52eJZSYr8vRiPtPgUKkQVIxGq+TOTSuZH3TViU/KiyEUZSHgU0vRour1zdr6T5DGcERvs2pdmItIlJurdN4CoWCcENCJ0RR6/O9NCPaIJ3E7+E6M5mZ84l9gaI/+P5gWYhIkba8oqM4aZxttRKvYZlrlKHCrcVi1XphL5f1THqS0/ce4+03PULPWWrM9v7+c9rcxCzLxPO+PffjvwOHZU6lgcMO04kAxv2v2XQF+QdgpqpBkpgFpDRWlpeaJIBC0in5YEaU/kIpe2IAwAhtb19tP4cEhMq9/xuEOXJuInfq5v+g9Zh9QO1Oen06qrY4jmlaj2rNScrpRuOTD6NCuww/fldePPhvRTMDoymX7mf2PrW7a/vJvUs6GE2bdimJ6/22emJqYG+AZwTmx576HGWqnJtKmas0ebBjnP6T/j2eWVpkv4w3n2GYD65GCHJbtPFmBoqb5MJztAvbG9rZ0CGFqaL7NCG5hIXz3vyEHouMLYVHgGJgakZFxRLzi3BecpQnW3m9uWPggzdLYG/1/JzqwLkTHAqZLTfVDwkTqoOcgrIyVPM48Kpo/mCXJ9NOrBHevbwv2tjTlKbUtteXbXDaQGDl6HBca2c+aUbOKs8kg/zX2qDOS87/gYAYZGBd4+mnApRrgP09x0H8OYKbxD5yY8fdDK4wbng68UeevNTeqQzZLHP3sWA6aTmQtZUkhTx4EySaFsLkcWaTaHhmwx1GQMO/2H03du7YuzVKA/St6+90acGWTNLeCWfH8L++rW8t/N/RDviN6hjorywZ4rSCyYJmXeLZJPN2XYtTY6BRZ8Nq2oplwJmG6CJ9suI005MvMxIL67yNhT3H13Ou6hS5vr3A7+ozeYF7dymtYyT/n7sSZLh85FVw1dy7k01dHtBOcRYnRHzlSct2Gxb2cb190HhdtBsXMUaumt651tMyXkoO7sdL+FUEJdQCfXnReXICBlJoFyaad77PJyKRjo6n8BjD5FLuhnX3cwPEd7CUXxj16Hl+/y/KbbeBfzaODeos/dW/2PIbA3Lu9w89904R1aZDrWaTCdIfFC4SH3tEwQd4rMuUdSWxzhYnH8NrRInuawE/nH83lPhEXy69LiQEzuYqV38Yvl/RclbZQOStGnFy+Xi8Gz9NCqUB5uK+cE5QRuNacI9ryTfQRcFOje6vlu2sPRB0IrgH2/QjQE+gFDQGNoEaLYsxEA2AQjJdSIuOpSlsGFbLi2lMLFHziefhAOijhCCL314RD+dcAL/gLQ56Lz0rCYtA+oHr+aWou98e4RJTwvJTO2Kd4xChI/Dl/ru3sd1QA1OBOWHzbk4TBx970HPchCXJBTWhRoynL9e3Zq5GVYG11xMGAhyiuE9nx88wQmCvWg5XV5WF3wKdJrh1vbJa1v4Ix5bHsONiiMzjOkcdiJtPZT7PeJ+qBvd1NzenZX6TvD6ONaq+Ibe96sHr/T8wr8Rf8+F594EtrpFgVYCKbLD216NrQtAWTsnZa98g3nyb/OK0/K296DBnfWZjrlc/mZavKvh3lxM7ssb7Tp+AdWvta7F+mqGhsqJY9ycv9NH5jhbs1kpatqm4LP672evcmt7d3lOvUMXodUvQJiQtJQnqh18Bf9//zUxEboNUxV8Il7b77MhyzhVawu6MdVXStro6GqM0Lrng5vnTkgq5PJ5zTz47R3DSlovViccskq7jd8A7o6pmBqNmoOCsi5qUGXs05F7EQBFqJAhKxCzlEqfLZOZ9l4BDjvqwyV5dfkKIggnsOSPcs3VTPFRnVGxESv6nRBNS4juYYMWCA/DI6uKStfnPsckg8ib65a4ChRQV9T9ej1CASde8r4CVKEM5ayQl6Agpjs7ASku5ExfIm8AlkJGWkhDQmicbtkFeDYVkEPqHn1+QTOc7Iq4CBRTf71NCtnnVnyt2AN+feT9NyHLLPUUQyT8z+bGuVwS/xUp+WWYP/6hd+3QFNE+cs1vBlRdSoKMI+DMBXhnZuTT09iotB3Oj5WBNb4nsbTizvXeQSUt+/LNu3Uk5BWTFFwKANZNKLy4agsfyZW2vfKHroNckm41/r4lnfswWn0fdszwZeouMhXUSoRyBXXsRXZJZDV4nbI8z+mVmtqHGKRsNba1ve4p4DsVhsbHZnnY20Raa9iUG1lFGGd+bSpZ+Rtfw4Z69MYnhZbGQC8BDPe557P7zlq2hkfvcBI5/HcghKUl70y5znHC+c0M/2rP1ztnUemx6O6z/2m03nATlYJh/fNPMWlFEEdFQ9BvAoFan9yOvN7cGirETm4zx0K4fNh3dKgcl9XIXJ6OS7e4uR2IHI3q1DgY9Lx/rxArjjRXLkFuYXcWNFArlz4fIV7Ki/x8PbnKPvpwTOs4LlmT7I96StfKJgikwxv8WMEK/vTaArVm9sBqMVtMh0vhjboX/m8wuxAf9uLdllB0y8mymvfb1y8YfxpG338sznwsRIAO0oABu3P5ujjz/+Na49r/1lT+2IkaHpZtnV78BnmzBbTbwx1vGiTAVPPv6WtY4kXqQp99XanZB08I4L9obRhOM0wRd3J4oKGm6yivXdsAFztDoJwQSpDKUFkxHjZSi8nHXNKexZDSBh91tJUMfs2eWeAb/XImxObKyI3+Z6Sn1xin7uHqfLcd2txCgMPclXL4Y3Pjo+nhKALtwFiwcA77DPAIDz/9P3N/pWVbkp6aXFibDGtikHY3gf0aKkm+nPmIS+byy/VWNvU1lPanmylXG67bG3b2tGc3dmc29FpY3e5K7Hj+cPo9sYmG1vNJEpWwscXWQmJz7Lj97Kyk/Y20uFZetIesD0m3c/K21N2IC4R7Yk6FB1Pe88lTwfl3L1sqGVFk9Hz5O9k0P10DR+TRcI5SrlnBzb/GXsUMK6orZQashSFVN/u6q4fygox0XGpM/Fwu0q0zyPgw3N8cTaSTtmkBmRy7fnPF8vkorH+hpabGWFm6i7lRrUSU7LDRLvcij1/logwBys9t3qjRUWnQ0EsyaG2nqkRnYrAUBr+qZOybDzSd+zRvQR6XG4VTQ0S/at9jl037ou31Fd4PV/3i2/IdUqLbST5kXwj5Z7H0BrlTva2twl3t8r29rRIi9Darpzsq5Oyvro46GA4m2AwO+iwONNrZ3h3dsbwdp8dZIy/tbT1T49IDscHtkY3ZqvbmLlYOBL1LvphiC1JadTCvDRVZ0FbE/Nzoarv2hN3i+JAJTGJfPi6nwQQ1sFZhoK5Pr/bWez/ifVsb6ucCM30OK1ZvofWICXSe6VdhNYifXvfwVMfVVwTE5uac15fUJFUQa+dxkFcLXIwNHAccuyz8LHStbG96It0gZTueX8PfxDDL/rk5uIJ+Xm3C4tm84pyiNr2dik2dtq6Ng4XtBwcQJRbPZ2Y3h/UX0us7Q/iO/FFm6ANlypUjc4r+wYYnDc3NrNzsTfwJdXk/s2lEAkR0cQXd/uB47qVrTniqq+mYWNgYexmoOLrXk2JSiiMIioZuejyN5wuJtZQkhIb47z9NHzMre2cjS7k3TYA7n4o3OX3Bc4SFer12IHMlMrQw2EoskoAttLj6tqALZUxCjCPoYOBVLYRFnE/e43x//ZzwNCgcen4mPkzvU3zUfg+1sxT5lzjn4E1cQjWVFSxPWNPfzgO7lT4lb/SddT2YwOGcFIlbbSomDZa61p5baSouHu4zp8uNDjVxys0mewWFpYyH5oeAaJdSIxMxNBQgLlPcmJqdkJUbjYSTkZa7k1f6Vmcuxrku5CXZq2HJSE66aamtIgh+yDDkWK9k1KkyEtzJ/bz4UyIBoIuozYyhawoOXVw7iGQbF1MzWxc7pSkXDZjakrwx/sXbz0Oprjj63wy1vtuWBcXW9Nvf16c7dfuXfI+tRcEzmvWYqIrxJLfRiaN1XM9KhZgV81yRb9s+FlVlnWfWq5vNGnd/FVC8DDl5pfnK2hLHTJgaFEloTo1Y5cF+i+XoYGd7D0/un65crQ9S0XITS9BwEJaydJ7NNc+w6xotbfZuk8qXhhvXCmnHVMc76luF9yf6pRm4h77zNolNtLpB1oGzDh4smdJqu6qHlv+2R032wtqnvYsodjsU+KK45JaEn0jWQpe9/bH0vzEfFW9hoPDp5/vI+t+344sjL3svJkVIWlbkKxZgHcadLojAZlXohl80B5JQUrHK1J6p7jaKMcu1feAbB//h1+Y8sC6oP8LOXFJqzGUlxk7e6xz+E5jlQunf7e1Pc+Svbgcn+G7fEGU+Em/NXuCLHsR7cUrIGp51S2ooefeTW+Hnlo8Ibfr5ypceJmyG76roW8s7r8fJG2Yj4B9qj0UoV5SJnWuXrdc9L3fFAtXR/95kp5DcjplWu/queilF/ChIipQb0RHTcBKyFDcqWHh960HTN5afXsCcnzawrYN8z+XQDM0DwtHNgqTgb6e7Bze8bT2ct7ZYzztUOMZPFfAkVXIEFwAxsM8MdVfitNZkA6VzlTOb6XVqb3c+p8jh8enw5L9orRAahjr5wjW1LFn9PD83NYLFnCRqCH/eZyelfckSz1FNXatMG5m5+cT979Hbs58vrQSGilnkZL5NWMjX5milvg4M/chQ91wgGZmvjXWT3+7wz81+0Nz5sdtgxezSTcWuGoTjha1DQBXs0pxR11PnrIni+c1JXvX+MhikLj+lSmorb+1P4D1w39joLcJMw8OMDcLkSEB/q2wGcamslpasjLamnLndLWkZC5og3TfsntwuKVxQYCy1AclB5QzKcpWWlv45sRpSU0tJTjCU+Du70IQSoPCV/w+IMazEmuLNROVPkuSccYaZ1a7mF1wtLTGskLdrsioqNioiPCImOgIsO1y6cs6RKDSE6KCnQ07gMHaSE/OgYxKSY4k3IOgXT05y0D6SKiMItxdMj52Yh+DymyKSnrkkkB27KJRWU3khYLCj2YELytLgjtifMqC4GZlTfCwgFfi3Mm+ojHcojG+wNJLE40nF79dOu5MSCcb/GPEMDiBclxyTZwb659mIwjCjH5hR0J/HT6JwhwadxhvHnccZzty5pdXylFGUpBIwOyD99tNjk3NTXCeV7G8Tr68tc1A0Knuat1VR8GL/1fr7hvcr9NNB42kLm2iNmyvX/e8HnR1aPRKWq4KNzFaPu4HEvDP89+6joEUkYvIKz7i6R1UCLuRK+TzkQVNVzovUX+qAWY9qmiutQycmypP/YH2/+/5PxK7vryeebwyfZUvy8YrVytjo8mAGT8c7YmGSA9aaZaqX+5cbsLUHc39T6NHigaiTmmGCa1lpQV1WUjcLa7iM8rx8m7Oqo6akk7GC46LlxcNe+no6EC89q4pVRnD+254HjxTt724PSW5tA2pL2gsupaWLi/izT7M4fYx9rTvJWEOsfbXfgHTzfTyJ0aBK94+fsh3KB+tlcMflIkIJ7yA+Bzp9NtOUUe2tE66UgszImIK8tLDKmOwXj5YHbcLutd6TdxcHK2O9YDwlyMO6vy8ho7J4Vl5UeGlldTUuqYszZWn27OoVD9lHxVJaVdigJWRG9HMwsUVih4GPgwKGNpxsAGqrrq+05yuoLWnjndNVHJqTZS3jpWXnsC8vqM6viAihlIYaXXnvLdpI8FEU4d4sRlvxNOh4mR00cjGUFXNQc/A0M4AeO307BMjcosiybV1MelFLUih3srK2iLwgg23GNKCaLqqmcn5efhamLgH1rvigZvXQ8/ZURv33OKcEK+Yp7gWm46Tk450iIEgj1iUeDZDZM9je1CN8D9Z6h990sYQRYyHB919xL5KSn+WOMDR5A3Y303pqLQ8gChw7QHQA9fBPlUg5sydOCxwgAQt7B9oC6Jz8KFFKxfQVsQC4EB7D76lImkbwgcMM/pRE6peCG/VMuauMox60IgYaTXvR8sD0D6QQlWyRYV/NQFAD648AuNlTQB9uHIQCUAkagKIQg0CQA818BcARKEWC0APtfAbAjB4GWJ/ckauQZBLtGKd+wHsx9dRBR/BGxzgTQ5i6AkLQr/hs3b44CgPAFXJrsAF7yj3kxMAJQonO37oIHyI6nMYgYyF+XC7UwhLiM5fP0mtADFBNCFWi95Ozsv5gdMfOg4QLYQRaQ/z3aAfzoY60xpGMA3zx5V+U1m/PjjJxTT96FOu3Kcw30Gn0L9gLj+JlM2yRlSph/IVwnzf6Sd1hTXkHHQ/dDwECCOSEObL7xTKxVj6SeLIt9kAs0EfdjSwRUCDMozCfEn0UzbmlPmKUYZefuo+/ZNT6De1xLFMDLLygDu0AplWSqcelal6+UnkYIZ7dEAMHcpMyI+Vi0IaiEHM7wOQmMC2Yit9MnuAs7XCcY4hs03GP0jXIshHgOZ2IplO9rK4p01LowIpIFVLnK33kIqJli7Z0aMtQC70Nb9yIEvHeKqRp1jomtThEi/rxxIojU0VpGQYFolXZrGLcwDVrFMy1nDa1UkhVfVY0gNUoJ51/Mw2XaqlOQPmCthUq5KMRbphSAvcxsnHst7Id2RLTUmrtOdjH2GZjui0wnoxyQA5Dqr0KHfHFb3ySHUYrUJ9MYywBAw4m0uXnV7ZeJTAUWplywGdTDA562KDnA4S3qPH9PkraZX2r+AjUqInKSv0NtO9An0pU9ofAR2QTHW7CuNcjjfXeFHdLseSLqW+vlWoKxqjjF/vMaKwwAcM/BCoCMYPETx0sXbEBc+DS5x5iMj7tdpghEDJpqn0W49dHasSDWBFmKTHpvL9pVNIDRJeTK00lYDf3DROOUVSLqwizDLDo1s0S6usF+AjzIozpa0fkzP3S8rJ+D1KC6xSFkLO0ncS5GrDvR8vyXtKycspa7JL810KlNr1snVhotikKEmjSZIdax7zm0rWCZy9Xz+lXOxV7KCSC00lvupYyW5fHUGrrCcxxQIlp+r3Np2gLw0FhuUKOBfZi/EOxA61509IsIOCFf9JAPS53VzUfdFr52ckWpHa2U/Yd0VRsjRXjTCNS3vR1B3SpbsLHvsReXBf75v/X3Z/jPaquurqzMUjTtrub5dXe5TsxUWnjYoZC3ByfnaYaqpVWf27i13lVtYe6FERu4rEK4B7WL77aMwohplB9wFGVVgJPanz/lCYIb67ZsBMT84xGvFW0Q5CTb1CMIgW7T4yZV4cpVk7CpKPblVFRuFXICpE8tFxbE8b4M463FpQFAdigRWEUUiLqagy2vx36+7UeYWIyY/Urze/2Hl/7299UtSV4zEWaLygpltVkdkZQEWQXLzPwXMPO3cBRuzvytNgBlEKXI8OS23KUXeydgFG7O9RM/MShPr7qzpJP2izeij/77czDKIK+6ZqED/dT3T3cLgLQHYMypPRMypmsosXjNjfjZ2OO0bQGOI9T77rY7ctV2npfR/MgmjqfWSqQNRq30UDSUwvoB6EH+/MMfuq/hZeADdSvheD6BgfxlmkWx9h5VZfACXg99H7lOMNC3Q7EJ+dvBQoUOPWYqP9mNZWz9X+fcdzmPA+tg9gCrI/pJ8H6f06vDMnJ164tC94SbijVfMs9TZtFE5++46GehnINm1a/Sz6YtPgfSVzbSqPQfcc3UjUcz++0x3PEmsmghvzDCL44AhiT0zzn3a8ktafXL5untvjumlyz/1R2dNiz6LvPe94Zpndpjn0R5C6IlDPYvzmVrjLE6iUoHueAyMJnDt27XTcOYummfGVK0Ek38l58n7Kt7rSnuY57/erc23O6rlp2tPHB8r0w3tIHD+yCwnzbb2H58CZQeGRXr1tkY4F9y+YP40JkauqzoRoHiPjSWeRVXx0q5N0zObYL27m0R/fmqmRTJKlRhSrAO3me27koV05XkYhpU1lia+2zhjFpn307ltL1XEXhfmub48hPe2sp7hkf2YlxVx4pqnuQ/mzaEm6/L4yBtvMuHe54hhIXt1HiIL4yk713Fc3TT33T2oV6tr9IZ3XOiE5aoZKdhqXad5cNr98BIjqPGMa/9s+drX/GAQfAeDRj5ySALwLlv/+xv1be4QehQGFoAACKDDrsAAUarpS/+veEeKwUZi1cuDZ4OfzV/lcZRnL7zY2HSRsvxHQ75HiLKpy5VQV4UVwpFMYJYNaGZLJG4XrrKM3TgY98IUntNGOJJjAr92EdIlPPrXmZ9IBSvsk6mpkfZsK6QOI9SsDW1byVjfQueIJ/VlkhbsjooStJt70N8ssKaau96Htrn4/sf2hbm/05tHXI+x7jvy+oLE/OnZLUO8dir1IoBc45p2mJUZa7YJ4EJMVDokMeLcBfAep6mNcOxSpRThWq/Md3D0/XnVuKc3qVKx3l0kv3/zKmrC4KRz1YSRSdfcDWYFQRfg3xmVXj4heDHB+bZNey3De066a6WRaT8iYxijs2p3TTqK6iMteENA4AhlW1w8RV4jMi8B/ZalZLSjtgnr5zZJf5MJCfquzrm3GtTX90kTtcI1rmVV+aMpnnrz7uHVJ+qoXtpeEXSyAvxRErk72twHOluYyxq961I2mKb+m2XSb0s5xscM5dJy6zGu/o5FuPNfcn5wVrL4oIhaFH/j3wwP/7XGc5bOz+zh5QDgqgci0ibpLQmxIPLUrjXg4XXhe5UunLLqoMkVbWGt5IPFePVYZjdqm2kGsep9/F2hpY9Lt5PxVd4kEFGzMubYYC3Gwgz5UQYI7NGDW0wj8eyvb7KAKEtyhATPdqINRS755MTmxa9ZvSitJ8HpQ3hdtJ8I5bXim3LLMOsTAWZEWtfmfeJXXuqCsDgKB8tiQKJ+UzUF0UubnJc0qPaskh3NZF+cjqKBMEigC2e+2hGe2+alqRbTcUu+bLGmtKNu0WpRvAoHWiTJNWaLsECg3tHKUe7umWNSPdgntGMoBrS+tEI2Q4jk1EVE96lHCpsO8ytLN3JJLRDbZ3mzYveui/o7ykn84pcUFCF7OvqGVt0dAO4nWm4GHzuiQ8LSHaBfYNe2kZbklP55tY+8nZmWOc0ga7SnaFfauon1h1/YI7VFXZV4uBJDPx8eNwex1kfH7KogIDUkYAYwxwI1HCKgfj8KmqBndbqbDeAxRJI5FseZs3xBME170YCqk5KhQkofgyr1WPQkkBQnlHZL48hq6BwpDEBZlzIA2XaasLPosWZKkWQrimQjnZ7cFX979uO2Zhz6VvoIEwqkqZchRpMpCOlRkifCGEUg8YpDllDiu3a8JFi301jbiMhnqCxI0x0gI3Q0E8iLJtRKkpKVahLEUijzySPvT8CShAeR+8A4AAA==)format("woff2");unicode-range:U+0000-00FF,U+0131,U+0152-0153,U+02BB-02BC,U+02C6,U+02DA,U+02DC,U+0304,U+0308,U+0329,U+2000-206F,U+2074,U+20AC,U+2122,U+2191,U+2193,U+2212,U+2215,U+FEFF,U+FFFD}@font-face{font-family:"Roboto";font-style:normal;font-weight:400;src:url(data:font/woff2;base64,d09GMgABAAAAADRMAA4AAAAAa7wAADPxAAEAAAAAAAAAAAAAAAAAAAAAAAAAAAAAGoEQG6NaHJB8BmAAg0oRDAqBhhTuVwuFWgABNgIkA4swBCAFgnQHIBswXCXTbXbI7QBIvvrdaCRCxjgATPZ2FLUwUcoI/v9zghpjyGPdg5ptW6AWuzqQmulyT62B3sOdz70SgoRw7CoowX6W9ZyKOizdBsI4bJSw/To859HuqOsX6f7krVPq7BPIN23Sh9QzLfLfBSt+GTkj/wHuMgkLOfqQ+pozXNzgRVltoeQMHOej5vK83xrw3mQyux+4QChMFTFLYlcjCI6vApYoKzzwvh/4db5/51QcsyhsLBk9VTVR51RMnuOOvlM5eo6+sw1Tm0WxFLUwsUqb/4j3+OcmKZyHSe4MvnVEJ+DLyNGc3icFgg9QkSUByOnp6Xk1J7vj3eqdYWFlBvlxq5La2/wu8cd7aye6whdHpoHbDu6KJiZF+9OnsxKWk8Qparc3U8KBemqRBfYB4JV7g1TiTiqqGTz+/b79Xs/L3vzZgYUPBDYRnrFVosIQOFAtQMj8DwDg6fUUG2ORfnyEQ6ptuawvt99+WnD9X+i6yhrdbTq5GygSHtWen1MziiDhEvyfzbK0/kgDbS5jmdXmCRVkphQo8nOQOq/+pa5WVal3eqSFkZZnlmplkDQ7z9bKNJJBJuCQpVmSeXZNeMARQwYcJARBekF2aX4ccXRBmBw8/+3X6s7c88/KLPLwviER2hCSlUhIJg+ztUTahHqb/YkSKZVM0tM1kbQFIta7t9l7fzJHiVroMkczFiHj5JW+f6gtQtGMywmHBHKjMCfS3c/1ITkRkZpb39ev2NvyGgkSJEgIIoXYsx8us8yNSnVUvi7D6STMLhOnASpAg9jlKRQUbgDeof2xFKUGoeuGiItDpKYi0tMRBAIiOxtBykUUUdE6u1GmPULu5oXU8SqQ0q0IAoEC7gncAoEWR6UiWBUVFReXlFAoCHATSWqYnvYMExs49NYUHwyH3nnLNwgOvW8nMhQOYQIcrwne+3Z8KLAChQQTFPjB3ByLHWpbYAI3/CZtRxxwWZ+Tu+yc9Ww4tO60WbYszOvMK1u8z2a6IjcE9hvIV0P0v58PuZyG8BYTEO96BKRt1MAnJfwIEzv+tLlcWMr0zE6Vy4f63noD3N31zD81zv71vuZvDnLKZ/eTnPmXxpl/NgaujR27bj/sUMA5f5+09E1Ws5nXVahn93mHGSt2NOt5buv2Ig0Zynbq8MFmOmv5iMtoN/FFfFJ3N+WQ6mykOJ+7Pj3JzzBwB96QM7lo24dHFc3igfCCR7/sR7bNVt4UdnAxempzm7d9Zgv7bvXq3KZedjr762OatqtTn6N5p/vgn8N23TE3mbiQ5ViJzjc8EKnWzxvqur6VXDy15AYNM1rROfz3l5DZ6zvFJ33X6TEJB7F80FMpnExsRsx9RBetAaEY1Q9c3Ml8lmXvb6OSeaaT78EZit2u0wzf1WZuRvZxNjJgF/3N5YjNcZBXDhI7tlEC84fCzZQgKQrJPBpFNpNjQmmy2FCdGixd+g4yhB5hknyUJRvH2FERTsknuHBzUk7yKdUanNb0yiXc9QlmvJzf/aNzgCxwToEU4JEKqcEzbTDwzvAE4JMps8E5ghma6wvEsDyFDS9a1Mzipc0qW9b88lUsqFHHx3oNfGvUyM8mTfxq1sbvdu386djR3849/OvV238ogUqY8XFw4JtAPi0BzSpJ04pQ3SnKnKOMBM2mEmXkKKNAfRepTZFalGgWtWhKbRpQpQ41KjVOJvqOFIyTC5auOdYUbKhjSwv21DjSfU7Uc6EVvannRoN0ikKmKLmdEJWqCNqpBp/dkSxgj3p8YipgPxXwTLKg5zzG7IlFWbRq5WteY/OGTwQOb21jseMT8t3vuB/+IfylHCABawQiABWJSEBSIzWg0yEdcKdHeuBc6RBbRhhgMjEyM+Nly2FLjqVIisM4eUuZ+QrYpzEMj2L0aMaIYfRYxohnKJGhQgwVZ1gJRpRkWClGlGasMjkaOUNWORitPONUYMyKjFOJMSszThXGrMm4ukzRnHEtGdeK2WvPxA45xhgRDsNdmHpXxndj6t0Z34Op9mJy78JbBCg4Dp397NdcBJIrz4hJc0g+oThCzqhGY952AXFISlaVhMhqmkk8bHKSHYlLkC/RQ4j+RH3DHa/gE+CUtp1qyEzCGjdfWQhkP4fZz+H8Yc8WRQBqO59XPYPhEAR2OA3z8R37fO8Tk0aEcsAGn+47KSooygT4lZ1AAvXZIzvZcbHIhoChw/rIcXG0ipmQ0ImYKFLSAI2YEByCq82h2gUXCxcLF2sVtUqbdu02VKdNUb8NGizQc4dqg1IAVovWalNrs4DOIFUNrCJ0YDowLRJ8ZoTMvhQE+sQhePDmu7hf7rk9vYd3726doiEPYfdO4qgN79pdC2t2Fayc5bGc+t1LmhFVXdbRjWsnipIHb73xU72PLOgbtqKnN1oF2QB9jeOn7BDch1fOmh3Mv1z4sP5yUq0uv8Z70RpANLJDzoDOmCPbZQ/eBr9+NGdXDtEQ0YAZB078iiimuBJKKqe8Siqroqrqaqqljrrqae7YqYvNmfjGvUfPXrx5vxW4Df+bctc99z3w0COPPTFn3lPPLFqybMWqN3Z98NFPfveHP/3lb/8UkBCBFEiNtMiUI1eevPnyFwhHxcTFJyQmFSpeomSp8hUqVqpcpWad+g0aNWneslXrdh27dO3WvUcvFa2MtDXSzkjHwX5ypn6En+FX+B3+RPr/JUapUd+ooVGsj8v/wW2XhSLWWGeD1zIoTVk6AyNN0uw2sdlvqEWe2RE/+MkvfvNHCUoB3aKgWxXrWSJZUxjAEMawRS522OUDH/kkUjyJFKIujqTLHJmXrkHJmgoDYSiMha3wLNVqUZ1sV7I9yfYm+y5240N8jE+JTL7k/uSB5LwkSiGpK5BdHrusUlRrtV4b9boZWlrLameF9PTitldKfYwLadzqBZqwjPKeXZ5zyHue+IXkqsTck5o6hIzLzpjGPMEEDwEa4ESXbPHEDZFZ8Q1mzcOyQqg323dugHNmhFyXLzGnpcYUIXmyU48D1UOjlB2qeehNdeoQKIAiKKc9dn3lHZUvg3hOMG83KGf7SBa59lCC2a4D3g9gERZhERZhHjY4yu9Wi0I33Jif2hmYpqp6nC7eCMLm5ERjR+L600UCdBNu03cYBBiCMViDbWs7H0c/J+EaKneyta4Y2O8+tzwPL8CL8BK87H6xvYNNeA9bsO3uHLvwAT7CJ/gBP+EX/IY/7t/fHvwj//sFKiFLnow9eKspq4ldgHXC4aQS26jTCWdBEqSV7EXJ417Mu1ICFWWS1oNA+XTv3qW8g0KL7HLOewjmZxkanvqcZr7yzAdoFMoEh0c5ay0RpCKECzUJUIalStAbPyIJ1qvuIYKlAIqg7HoTKF/ldzHIcShlqJ7n5SH/Hzxb3M8h1hBr6G5oicfS4DW6QuDyVCub7ybPsNJkOs83N9la4gUN7OhL2prmX9bBeCX+FdRl5sBOcdGEBbu5Z6vZeOw2gTdAryb/0RQaBlm+emfgW0NwQH7bB8JCgPD/R9VA4vsC/DwhuooFtvZ6X2hUCLi8L8w7GOz+G8aDxYcaWaOPyVE6QPkbHTE3xsHE8Erz2KQ0U8WsKKk+cEw/vDlPnz5aq6kK6lJpFSc81aqEBzVHIO7ff4Yu2HFDvAiRbhg1ZtxNE0ao/OLO69NrwKAhw+gYRjDfZnKQs0k+Gqy4mpB2FOgChyz4iRHJR8xqNujNqYG1PgRplpZDvkbUl580ZZyZF2djosP2EbTfAQfN9CgB42rtbrmdaqmVemm43khpl44T78cZs249ogOG9uPjqLnsEBLWd1FuqhO+x3a6odEi9qLVz22ON7Z2tQ9BQJdmAAVbXJ4gakhN5ASOx9jN+RGZA78U3X14UZfXclh6S3ad7e/9ac92Xee1T3wGi9brI41txlXo07e97FbNfffVsX7VPKlg16RerWqUyOEPjshKspMDSkkRE4rPUuTL/mIIpuxK0pq0YRnMm+5dpGZuHMU4Ka/skr+KDg6xpUzehQodwAT6R9Aa1pwGnqHbXa8u5l5NBUabc/7azIq9iOYUUTmE+nfdNWa0XbGb+M5vm3J+2ITzc3SKzndtGu3yaupxzEPcYOorGc8MI2lMBpRkP74+X0JWdHYtv+KDX7I7Q7ZoX4TzHpa30OG7oLziTVEo26LLQ776zYpx8PBdXLgdZXZeUfnl7F3I8E6WNdJ2fVdnTkmu8Lhs9r6ObVLD+vJIZ4Uki8RZYnVp7SzQYOGrmPlBJr6CUfxgqshs2HlOdOZFjpneYuNrLkgIzMEl55OdGaIV3+KChzDcg4ruK8+/894LHqcMzQWVmeTV4lNVV1pjRxpaOtPV9p96lRczvclvzC9y3kIRt0QkLJcLVq5LInBA26PNmC5IcZup82J0YFFR2DQOGGyjliKB9G8vtL2zNczMkQhrUOUiFDzGlFs//cKAfXca6tRH1iselxognyPx6dQ7meeaB7+XZ77QXB80QPYO/A94x1nRkB+yGzA1N40QIWisgVXu7tAgYaLB3/wSGjBIAg0Pc9Tp1GXUtAfe+OA/UqGs+1pP98teWAgajWZDn3gwIXSj0M1Cd8ceHMA05nEal3A1f2T+zocUpC0fpf6LAkSb/Kb+pqvGzHjorY8oadURcnfDLuzrOYWLz09AvudTZ3PTsYwDtwAOIE5RugbsyX8XN7CiFY68iFKaD/4BsZnNaGqTv18J7l12ee+DZb8cPfohgBeB1NOkXqBTPqW6fydfT3XXwm/kv0uTbs26Da+9kSHTW+9sem/LNoKs384fDKJsf7GHf/iPAF8tBAgMJphSCABqFKDfBAK4rSy4x0/BjePAgXXthjbrPUEDB4WMqQ1zOc1HcQUNRMjVBuhm+5aAnaw6A/WdKhdEvUCGRewW9SVUFwEgWcV4SKmCx/WV8i8Q2UYvafPrIInoDDfGzxAfG16Ek4b3H+5KHMH/tmBiw2Beag+7GCxiyLZfqSRjCGKk1lIxqAdqibQCAdTmw+Bd8tw3MRkZspWSVKJyIyRe+ipVjcXDEzCUpIOsDd/oaAUcJGBQyZY1a1w2yhQsSxvtFngy6aUmcJCZeyn1pJRyg2FqaC5RVUkUYluysxZz5O1W/SBCpOE5K1o2Nh9ZtAUkvn08RSLMgkcDzRXWaANoUpSlolczqqlUrQ7KsRw/BInMl/1oGiwx8meUcVL8V7/5T/knQ5rwzu0Xg7ddbgnDiyvWs8l5uBkDDdJmdc1SiF4LFmqQoWGgfDmVBDn/q8pyUkDYlmYyxJXOWOWKoVykUjB8FiEOeiydtpgTaD4GIXDJtpZw0mWMNF4X6Hl10IoSCEnX1Fux3qnq4Zuql8lyNK0iiLF89LeKvDBhbUN1dNagWpfz29xOlW5zgkWca9P4meyanTEP98LtkRoPtXjgWtRx0evwSI8vn1Oc1kZLGtiaQxKWI6MyxrhKrrxfyM4pawKrNAENtvE6P3SpaFAjQMXmaG/2A+GqWUEwMaG1qcdrjWHXyWoSBrKo46Nw4jmvbW0F4A/niI81KkVzw7MaKuE9AapJvbWqPeLqsDctSQFyJAkfuYQPiFTfFifXr07sIbqYzI489kbRyOJFlPBsFhxttwm51d1KDc53ZXQVMhQ4altN6S1MIcFZ9wxjTLB+q8eAqjtDqkW19ZiiqGDafLEQTmsjtrjYu+XyVNX+b7bwYfj7TE90PrnI/7lanSW1oQuPTX79SPv8qxRO6Z63yeJpdD4tbU8tWLW7nqHauFIv6XjfwQKv7S6ktVBpALkLRZRXuToLIbd/VNbnKhGL0OLbAVySB8C3glyGNt5CHFZC4+rgcRydtyw+XO7TjouBkNOljO3AYS/m0vRN7s/w1iZWVrkd852U9u54nTqdSpmzlVC2sWFYKOtP7qgBS24qxeDLVmegV8FjKp8mmkoHPoK8258u0Xz2bqkdtVt3d0/SxC7x0GiLFkD3jjqecW+uvjdjb/xPJMeQmxJ8GnxIuvVWvVvudY+7rtahd104oYxnoRFOLd7NavXoNUjE8wUt5xQtOY44scnJeO8BeBDdZ3kQShlc8TSyR/FSF1kqhxTxglLXWoYO+YHPCnXnbJiPpmDF0MoRqDlIxyor+UorbY+0z24FKGcI+rrNde14SAghIrUEasdbRXKFDqckMRPJKFs7G301rKQ35PxmPp8qrB9KUgGuhXuE7E0zj9V91AUM1N2GxgmmahPbUEGTgRppm5Mzm5zerEZ1SdijAY99pbJ/1JYn+COGGlQzzMyMSqO7Zsb7C3K1eWHbVNBHjRV4g7Nh6NLgrfHKcoOtKY3sAHKtyMgrIKVHsBFdfnTZ/tiQtCc3lHE6Z+6rFIdoJCz8L73UdSlCIF1NhVgMZKNlJ/B8NDqE3BGzzXPYzvEytcd92nMuI5e4J2k2mrjVeuNh30zang5e13i5UmMyDq61QPHxAbi5FsRigFUX25mboAc5NB04w4Nxzr/Aoja2XRXTcAh5A2UnNIysU+5h1FQqm8uvVIpbJMV5QDv5LiXyXBToTebBcV8/wCjMIVAPIv+kBjUhj81SGHkw4XlFaYOjokZiLjnWKl1yXMxybqosgkzXYbTYAxdKlEK48iWPetztkcOSg8dGonnMGMzQqMIP76YV4DJqrvVl6pvmLYlThH5T9m6kDHImaNEMKGl7Lfd/TzYXWMR/FLfHFvn/yeoMYtes+Kth0HYpQkajecZj5FXsg34schfywBZG1uQZ4GQKRnYs6qV4zghONAb66smzLprbzVEkPgbthYApIaEvkHNBx3gyP/Zx1hT+pRBzUpiOUZ5w9TQoy77QYIkwZp/Ebtg5tbbiEoskcGK9NvNcL92qFHLe82K0FjDBlzYROQr3uL+Nq528iuvPVPX0s6lcmxAzqZ7gapRQlEtjf5iN1m4UgL8RZN7mZHZoDmbh/oE63M8aSCtCun/ET8JLz5IcpeRVqLQoSZM/IrF8ymnGuBbJyC4DczwaGAHJ4R83f7v+IiiV0vHte+lxqe3cJMyE0TIOTn2H78h8avUsS9pEd7vBWQ/yBiOH62DgSjWqkLz4s1jf2bBIDNzS70D3DiYyf6KmdxQasBc5Rr+a4tWizH/BCaLa3toYcbNyaTBLz3WnSCbK/InAt4OkqoO2DhXKEfawCVNVoygqxyqS/9QTk8CPL0BaEU44rLjgzGxzCxAPh97W94PMjzZzr80U2mV0yBnvU6ZbjtA0wANXvNSESYibjFSrM2pqWoMbPWGdBvxlRQd0iDOWA9in2aHREoo7vex+nNc8GF0cSA6VOUQMehIZ0tgih0cTtumwRi0QepYKMiHyBUsdaNpLp8DQu9BexWVKhfPMPZyHRT23+xANuIvEE0oCrhNJmmRp8VJuvJni7//JbzXUBCEBTx+VwbMRxIOM562Z22U7g7TWupQOQk7OhVmyeHG3GQgIXR3xPntHQZ92duYCThs+Ub0HgNC88go3LPWZvUP8NGe1Mf7sQZwIT0yKPE3+Do2BOITszXo7p5yHkPKI9CT1sd/SH/4SSPPQQcj57EhbHa8M6RYf80zFBGLHYcP+A4Fwa44i3ISpiPPu7CjeZe9lPpanJwFDm0T7ugCGhS9NN/oDR8x6PRpxJSKuaHCqaIG5fTc5KdbiwcGJTvHBgcBnG3vUNwx2yFxZm1L4W85qXp7/faIP2Ww6nijSUcvafERYScHQXdLb0tBq9p+meQXVIqz8c5QV5FJjXFKSyqTnNG4+rG+68aiZ/xVloUWQsXVvODfzCj4mKT70UkaspFd7tEV1YNvsvUHsaMkonffxm2k6qZBCSKckAXNJWXpzW3pmUztRP6O5OTWtrZmUzWxm72Okb+dpa2hm61ti62t1ou9CenMrc0sLST/WTO86GPO/gAxsvWyNzO28DfUdvGx+fh6meT4S768JPoSAyO6mSI8ozXz95omRVurE1UwHh5JQy0Az7TTt1NqW/1nLg59LHnhDzWkdv3lk9alRcVyYVytLc3xadFku6sRrj6j8KCvtC1J2atreDiZW7qHAM18oEJYKSxTIMFuLrf1gnaIrGlcYxrjDegRs0siHbdoi3UVxGYaxXVvWIK+zHFn20THdQAxXHsX4iEgIPbb3rzrqaS6GSzfYtY2uBIHGZ+KO4lTxMFb7cjaX0yHipdJOU9wJrvTjfQcSneDMOhe1m3qXcTf6L3QbAzKF/fVd09A5LQOFvl63gfPYu9J3kO8hmY/vlpTowucCLbFWjFm8BjQaQnQXlbc3l2XR0j80FxV2thbPoxSHObfSn+bnpc4ji6e3iSuk7IwnqHV4wXgUSWzlmz4KMozMHxfCkm1qbPHZhRE5ZVVk+m3Uj7fubM31POM7ndn8Cg54S5PSEEomHv4necRwetffjYn7my9uusf3BJyLEU3rvjz5/3UFLA4yIijZyYRcUvrlyqwihAQXPTdnS2etGFalIacnPk2d+jIzNWsP914Yzr/vMD1HDlQjzmwyr6x+Rr0c/zLPe62bNzE/OTq1qLuyqIaWEacjywKy33qqRZ6h//9RXcGnz9xY45hb/cp1/xFaY0+/yK7ViulRvntt+DFbnf76V3FJeUMUasEwaNkaiA4Y3BSlHBAoew5qk7Ih+DbPC+GSGSMx1/8tP3z8d+VadMr1cEmPC2FtwcNbz8bHtp+xRbjHV5T/ppb+LMpNTyvO4/M5FEIgRIZlEJcyiEvZJJBZS1HUqbP2SGYk+K4Qi0I37yQQn//U2i/fZk6wYjpZdWoD/e1zvl+uVeEnrI0Gq9fj4osZ5ZS8MWBJD/87KSOcUCQjHD8Jqo0SWvVaGlp1WiDVm1OCuiHnGV8k55kwBj7lSLRUtol1GsHaLSQgJDrgUkKQMx2pX5sevP54tqe7vi04yMCibEwljP/mCa7/zvHpjf0DI7ItcjY5VkZ5hW+xd7mbYlE3igMsL581V5M5g31THE5axvRhM/RKzW4rTPfFsH9zfmSCxcYeOfWf6z2Jf3pXKuC330pvM8chDuziYMLuh1/ey11XNFZxXLkWpQ4qob4n3FjFvydqRJXtgMqjRC9iwrTIFK1VZJ421Xvww49WaAMMbWFnd2LsUkSV99koIeJwyuTe8ruLrZyqhIqceJZj6uUiydSk4FhScP4RME29f+jNsd+7KPlwefKdAhm2pu53+1GCG+MHuporqcMPef6s3pna31vXXnC4T8YTVN440VIjB7dc75PPJ7ErEwiXHDZqduk7///OP99h5xFQQ9XHMC1WVwOGVlzzZC4GVG6me6ePAYZGqJn+7A2mY6OGAaz+tF7+ZqoMMnvZUDpAcGPfu2PzY3JCIuPT3AAx35aFRoPrLxjoW1bxgkUKjNcv1SONHsMv4cch5tbrBMkB2jjLo99bj158Wrw1lkQkpcUUUYAAh5p6hrdf7fiQ8OCdSppYo/gbCKjqrY6oVY3DoX20o5NNX/1r1V6Bz/voRmXx0bkyrIcCt5C7VHzOy2u800dU/Kuf3yhYFLU9zVdjaaF1srUWXUTuBTwu1GRv7+xmb83XmL+BoB8IjAw+FEB9G/nn8TE64w436i/8FZWE/tQ4+IH7y5M51o0fdlWOFY0F2WmNlXkoH7DAtj3JlIy1Rd9Ji5X+os9nIWGIxt5Ga+ZHAbkreGNgOfL74fFIGL5hYmc6wL9k1r6zLj8lFt1Ok7IN4LxfuHkqv7U4MasuvxTEwJb4XW/lflkndmWB+j4i5U7ceEYLtTyvJjHMkRof6Hs33qsxrId/7i0VjSfPXB7PuFnWWFaf8JQSCYZR2DnAYEcBg31ScwmMrtCW4B5gXvEIfziMIKsrN17WdF2tb+zsqnkFOf8OMfU8Fp6itQsP0B7B2uzh0dOdWPTPmK/456nuYxPcJzbGBffRurGTooKhgqH8kaLdghjaGJzWTnMRX7vz6Onihq+Cn8L6s8W7j1bBZy7h5cvj3oXHx45H7+oCXKzSrD5ZILWRxlfzIxeEBt6NAjNxtCwOWIbjAJVJo4kxi9MAqCnxlXx82XjXP7DG4VDTyPXVzrNPL5952gGKKaUlpSVwtOllLSK6DKf/W6OUzL66u/2kNmBs9tt6RuDDvTO99RmWeOqkffTIEwa68TNF1J73K9m+0lFEf6nQZsY+whO0UHw/18+P3QsrYE1sB2agq4bl5KycQQ8zXS+8lz1k3jZn09afWXwPVNO8i2NYHLuZXKJaJr1DF5nE4HhNxLfnWSWDH1+wdHWz+OcRAnIrZsdOjJTfBjfRX1sSozdCU2XpwAztrBGhWdPXtmV+DjMPlz4g0CyqP1mVdRJLH4EJ/wK/0GeR+vi/C3mpTzb3oU0X0bW9kzleWebSmjj7rIfYiFlcaQNf0TFuFnJWBbzt6MjvCLtKv96uscJGeSfn5OnvhX+b/7YD3EX3Ns+OT4eSWGPahB7//PRy1LK3kp17aHRRZxa1NMc19PLYjfdS9M9DlIdZ3ZY1uzYV7Vmlj0DAeiieOmWPSrtDvwuR/mFhTlnuoqZoOjADHWzpSvSZY+IhwUlOCcFBINCvNHTxsKpUyQ9ZNQ0t6xHssfOhcvKb0c5A/xoRm9h54nqvaAvv1aaJ+agXYLv+nQD8TUpMMdGk2XXLLjnTTvdoH0zsPH02MgCf5JcVz+53nzG1/XZQq0KQrgT8/UqYrITKWwP7L6wSOInVJu4tZecv8kr946qJ/b1CKlrglfpLZ+1vfkHOvbIw09u6QM5ueQGaa3w/VsXHp3CJbHTAvGxjjcGRbk+siTIPo4cqnxBbrUswVsUdmVWPwU30/1enp8ZCU1iC2wHzks4UFsOYfCf5d5iFUfUk84pdIdY+r4FQPQdnIkqoUoMaFUBX6kclOhW0yF8HSeHjxjirA8pN1mYRFpkrQ93DG9dLGCYpIV6+4fb62gHaAqodtlax9oVvr9LTZpxxTR50JpecYHxAsg0ciNJgns2frdWU09AUSqAa8OsmJlFyaqfyp5hBOiUoKPlRV/8tmByjj3y/NadbzU9XosHH33JC/foBHaXcAxlK10Gn7r9HKtP5+5WovBGyvGhZKn+T0jCX9VmeibMU+H71Q85zT8Fgkae/Sn6VPAsW8RR8CjvcPBPnpg/LrvpwLYjocFL53fyNhX1Ohxw4KTELitS4UmeiqPVBk3LZdIgJoUn1blyb+TJXOPf5ITJ4daVXWhT/UOvQ7HqzQ7Pj6/so7D1N0OaiAPMNCmB/z2owsy287bfut9y8c+ihliieJtWzAsOPP8wVzn2Zvda/elUKzL6w1TCeZxtclm0tNjxx1sw60K0OqRu6RzIhqXfX/Ro3cYtwh7aOVpgTuMlJMSHgT4Zhmk9xLItDNyZp6lb+wU36+17hmcMLJ+ZuH6EJrdGXKYKjTzAUXyQVn2tjISyvKlSdFqGKtdo0VioNcWdlWbhSXceKnvm6c+vG4pOe0aDkhLCA1PIkVrR6Neh8xNWkP3pQk5qZlUrIIftVzt4PayiipmYVUapjJiZrYktK04nU8pCGO5O+NXn56VlKqIQE/JOJuLWJeNzjBFgyGYsLn4gpvQIQ7jsoQ50PfYBJ0rmik6ijNa6llqh2RS2JBX2eP4cirv2M/bcY3xmgX9h9nW6jI6mf5dOKnt7A3VLQvZiDu0dH0vqoVcQrkR6Ouf4PVQcujIa6VIQxBuw1JfWyfJrZZ5beoVfv0ZNaKkh5dXgvXQVzgsYx81Z9nIdiyrF2x/1Gh8yxRj66enLmGZqHLWmm/NY+ug45wcBs61zToGZndJ3V8SvV/Wxb3llXU33t8WAo7cbjyrqORkqRt5uNs1tQdkJ4bLAmNxcXa99/6Z21J7FXNPiuyN8LdLZXC2Ob6+v4m2pO4NtmGY5G0ymG0wzH2ak+e6OH01NG9wfsIWCsx9bYNiUmNcEvrDmmM1g2K1HXTE6mjsOvIzU7szyPoOJ50MI5/pz9KUYezgoc1GTcQHk1hsGaWa8Ojs+F5/DTg1lZXT1/a5VwZ0eVED/7K2j0q1WupUB5a1pSwmWNOCkoV7XD8BY+oQvxuOPR3Zer9BF7TXlDUxWfHJB+LViXXwesJ8f8SyoD/YsqAgKLKP4BFMq/ffKGeopKhkbyCkZmwUaGcLrYLrg0uKalJrU0tbpFQOCLdpk2tFfYB587GOyrK/VXxVjPWLEa31zMWp4c6h8YaG3WXZAPvCWXbIz0VSTFN/UUjaTPVHs1xCdmV8b6K+g6aR4oOZ0f2JZIzLiSFFQjbqasrWegLBNeiTgA/zSscIhSYJ+sUhw0XKqMnFEtFWIotbDiXQjtxRYG8/FsXYDAavOGde/1ebZ/n3MBhgZFz3+bfL5euIrPg6N9ZYeKPGtx+cyB5nYyF87/8mQ9ngm7FdWOzy6meEVigaXJu2bkZkXlyFizUS1jXPb6+JUGjI9HuI21G97XzNsj3NrSPQTxAOMWCDid7B41NMbuJRASgQ/xScQ505G6lTtDk/O3+rpqrwbjNE2LxlSolBU/z97FKBxEcHvI3vVYU8DQhsr6+J+alRrsw47b8sXeOrYADgaG5vpm0fEGZgYRz/AsJ5GRBThZA7bCjjRLj4q8/DfXR/2tq6V1flqceKkpt+YfkYGIqmp4f/Ukn9Am+oY2N2QrrGDBNaiQJmId7qQeV5n9vSmIoYnuL37lHz2efJPlzTpWkQEYGv2ayL68WmpsQi01C0CHbv/w/ntG/2RvbdLlWV0ZI7eRfNssk9rZnkHL/nPOZyt4COfkgnCuVrKG7teK7LItHXPu27j5BTq8FAWzFQFMVp+G7aprbstIZ2n1Wtfism1QSLx/ACnEcgib/6l/JKLbSspKzazLLuDxW9iMeM7c3XWDUhJrvKueVR2rjqMaVBvu29mIREgh1qYBLnJo2YiSdt6cQ8czMotARnffT5Zy15rIv8/yCVmvUmKXCcVh7+6npjbGhl+4SSa/KDbwGk1LdxqUvFizK3c151qKgTdzOtd+zhwDl8L6Gy3k5omUpLLVYdB67PBm+I27Ij1GuvC5qDtK4qdgFv21jv+7kpMf6nrGoDjR9+7Nco2ahL1lYoG/rYhhSaL37N2SYfmbHqF2A8sGOS0vfPsH7UKtu/21SM0v6aCFz6wPnaWOLo9Lvwmv0ZahxABcAVj0COAb9igkbmiX68zh3aM2JLbx6/1w+CypjmFa1Nc4HO7LlRfMHdw1voHcV3xy8z14iNXF7r0k5mXfilTx1vCdwOMebO5tRd5nbmucJFyLCTJMIqZ/y7obpuat6jWNIyywXPg3zDpU+4yUXfv0/mDd85ys+nmth0+9KQ37srkPoc8fCgjaKBW3t/WUKUtxFS1oa+r7uRSDpM8uezmlraStnPNbgAORqBT7hEhnl/iozvi44fg4I9sLWtpSktqaXlo6UtKauiDzeiE4PtPlTJ+HaueRMzVSCcxBsSTXExHuisLHz13UFofDGY3oghU4yhzIb+/05AsoM2LSPWrmJGbMHEApv2Srampuwg56MPM1+T4v3jf8Afouyagi4nDkJY8ZYCsR9M+/H1NR+QVDaeDtMQ3kQ/4GTsmowmi6j6/XbSCf99d3IqLK6PSmYK/HC+qbd5DRFOTxEFgbmexDw1ycw4NdRe1DQtxcQ0Md4KgoQs4VS+cXy8gF7oiB0NPL5yG5SB40hPj9g0P8Po7lsEdT0Df7Hl2J9BXmr405GvvzyEkMR29ib3ZvUi8b5sixL4GEY/z1wSKhTbRZzMjlpJTsFFB9LUzKliURs50O4vLz8vNwB52+zhTfcr5VYpkGar0k7VJtmG1W19Zl4P7t67h2lWOViVLhMf6BQW6L+YsdhkRZXgbjncBvcutxUAOr6VzfHNHQOTrU43tWBTDP6c3uGQqQCb4sINhtKX9pzd0q6CiiK3HXkTBOPSJUgibBSvnLg9OPwMQDjY+far5QMBRzJhilNFMpBbU5SPIdbPE5xcuy7i7KThoSzia3nWbbZ436mJiY4GzN1TnpyAsDH9LwL6erdBpKu3JJlR1Qm9dY0UXKLe1AqqqYx3Iy0X19N0kZ6H5gnc0h/5dqfjYoLaitui0G4xuLqU/YXBbmgLA0qcKlDNd9bRpHjFLyiHGJtQWEuOYkjE8lm1eqpr6RjpqpiZFhO4ikH0vn40AEX+qb4KMJBTGRNeUZpOYrpTIPF9fvMiUVypic4xGxsbJRVzU1S9QzhiaGC6PZpWgqLQhytKKj5XOU0gNrY3lrYwO1DD1+R8tH+1GjkpKpUcQpWVNFNXUjRVk5Y8VkY0XSB4UNSYm3uLcu3+Gbuo6GbzQ5OzmuoTY1u7IJVaxaReb3uExN94yvdjujp2JtZq6ibGytrWloCvy6SUbu/npyOM0DAifWxKwFjN0D9S6kGJ0/8VmMvNcxsBILqqIGJ0UNGCcxB16VlcJMxVjp2OU/MxKny//uPRnZeZzAz95sUit//Qt4JOw/ACeS+bEmOuWUw/IwM+QtFnrlP//sgdLJv5Y23wr2YAWCg+3Lqy5L21IHsM70Yj/1esc22uAp1+Zn17H1q9QdXNLAXiDVldDof7w1z9DxFzD5vYay9ecvvcM+UvUN8B3eWdrU9Wpu2WxXqRewA7FzRXfdQ3I5gLYXQW93OA9bLsyLvMTLvMKrzbVfmJHzP3g2c8MarDzI8n2o9YTXokQHf4clWfJqPrlG84zbZ5GplP6O8MuS1pZFaeJUml6P8wNnvkN/qCHyyZWTZ5RsjzAJ8TxMSz6ZXJ6JxvaI4CQdF38kk9Os6iJl6lXZz9gawt6mEmRu67BFim7lXMmXfHIx8ozJ7RG+SrUDROh+huYBhuXSB/8OZD7wsB29Z8a+dU1vWusbOKQ/b6jT0KdEhpCNOhqiN7yNnJE3JqOE35A4+X6TSd+K5X0Ct0NrFbN5s6yH4wGt4Ras9S5t2Iu3TYKgTI5OmKEj7IKj3lRG3SiQqx2H4bo54uU6VWy67KUPyIyJSVFUSk8k1USgIJkmEyspWtiBogbp2/q2TlnBnUF5T7EEu2iQCp5Lk+xLsQT4joyllNii143U7J7lLDeBcJuK277NvAiqxUNC+mFtVgqzTQFA+TXs895w3g6LROXLEcsQfghQVbjvclUXZL3q4FTQx5xKysPLu2Sp1l0H53XTRiHobozRFGnj7JaedGHFeHWmw8awyxCFDCwnG4deVkPU6f29SEHpjefcvKYVf4vLR6RSjHBb0qYVhE2mMFPskkmCA7RGdZA53LxlQGvkUtF2AR1hSUXfDCtHIFOqHRiRawg7Em+pwof66XreqBfJK6XxBDoP6ZtlBciG7J0cwx8YuPsIngc36buPxrk0uW6UvYzVgc92Iw/r0nborsQSGAO79Ip3nCudhtMtNPDr0p5H3Mdp4vVFF0JYQknKHEWnNMvJpnnHcQjzpbcqyZEsFgszSvljoSWeUhong1WcLMik65sDsZZsJE/cTdmIF6emPIZkFwJGUrDYbXBhP2gjP3URYNChPshOuj4bZQml10g/Ea3T0h8f8I/hZNNsYB+qMA3KcX+gM6xX1ZZIN/dCRdqMA8Ayin/5FgwC4Ibsv0o5FtU1t84/Al/Mtxo9Os5c75Ot97+kdPmsvk5lK3YNsAU72l0sAp5A2Bq+2yP/I+lFzPY7jf0uyV0iCnH27XfLf6R65OCnMoJNOADfzLd53iPyXdkjm8aic7t6D/dQRrs4qxbAznbsQUozXAFzVbu3hl3YFCUnZ3sEdw9cZ27MZPovY/iOkkpQnePHjT3oJXuIaB9vLtSgI2DnOeVvcZFpxx/8GCgUc4V6vie1gWlyakE5y/8PQc3vCS3q9jjZhCYwX+hzspRvmm2yiIjO9mZjuYj4Sca8h3w4pv2Urzr+4AHmWpKQm7HeH4AN93Y4VVo5c7lCbo8Z14AvjcFva8g0qKH7PzaBroajXoDScO2Jwz2JDNYHe5szN2NkD4C0sT2F6KyFxvBwBa+WpAl4vYq5u89Pey5kW6tfp90Zp0SqE/32e/X9JvcppXh25ZM654x8vJoD8C0wT3QK/bJ6EQn8eZH7wjVyQAlFYuS32d1gBnHoW+fmqc/gL3FqMctTJR4ITEnjPnqmcE58TvsUcHwncu6i9iu6XJPMZ985wvZwvzzYPmGfPIKln+rbd+jgksAx7bj3v2If3TQhvpDvx4zHkLHGdAZ9V6e3A1f4kkI4XcJFelyian9rCNSfiXsx+fePNI1XB5I3TebVCf89KfqKzebEeaFkx4zH8No68xnIs2q/jSVeDVwYpRIyCt8BD4y4gpfGaan83YwwQ4+LbL23uVZX2pPcsr1+uZIj0W7TVAyOOFDmSu4eGUpkZ33yn/+JykIdA+K/3gunPY+rCz8fWD/OCYhHka9yCekwvYv7FfMjv6GNO4rwSWgsknK3HpdFk/7Z2q651zvBCUfSBKbCE06sCOlGEjtmvsEczZt15G+fWpR66Nj+oXU3qNt7kua80tYWVyz8nuR+beNID1KqVGk2X/jstsa89QYHHea/syxhsK7udUcC9tLC4j0bJapOdsg5x0+InaN45bCDPly38sgPPQLoQnf9uZN2D1T+bua4AYCf/URZAP6Q1d/+vt9/r1mSN0QAN4IBKOAcdbnAjc1Xff+3wMa4y7Fq1CLzUTP/fP2wdgB7Oh9xdsRMstC9IGB+8S1ffn1NZt8itvCOTDazKnK05Yj2YQXLBPamIoB2cAU3kIMKyABTwL3bPvURoXqcGT4G46NsJBDWDOPzgsB90ZH5o23Lidnq2oYcKrhPRIjf/S1a3NAEkttMMzFjx19tnoopojdn/e6GzI7WGWUx06S2JPLe78h5kOpmghpzH4+YZdfHFS0Dp+PdYeMONFYVJhZHcZyETBLySHKZdO6jyrVnqEydPMYfuT7Jrc2MDjfVn1SdaFg5gbeZBZz7LZl7DwiEJluNTQQgrhXCBxpKrR/OrNsEQzlsrUZ90Y3l3+HSu1ak8RAwzZx3P69eBg9tKZ53nJYD38OQ8ypWvMZdmlCXD6O5U7u83pM2ejz7A606ObVOkzBEFWuTdgsqrj8iycevRl7LmEt7ge0hx+JQFLZu0znj83lCjnP8sA9D89xEOsaZDU3yFNIfIuepQqnfKs96/qcOz0b/P78mtXWXuDUtAfzv0MH/xzqsVH3Uv7oQABUTgJa7voZDXUGV84hOklXqZM/x71dUerxr1StnOynpS4J5o46rkXJ/ID/J3DcCQ6dI64Q6PiQqx9ztBIAbqnCZgYoHLEAN5MEZ7EAJzI8yBP/bb21smz+XJaszrpn/n68/KkeI8opj32ngfXi7YuG0YUFuBbOBIKKs0IfmZHNgnte6OIgkMrKKPDYU5BPZHCesfudhH6qTFwaucVkXBwlARCYRmAH9n7TFM1S/s3PM1NcnxbHRE/cK2cbdQr4RCNwnZBpZQnYQyA3uHHIva8yF4/CU4IlBDnB/cYdwhphP1igodQX3H1n0VGysTzJHkgIJGU1Cjq6L+ieqvEjZNMsAfHTWucGdlzPAk4T7jcCzINrEH88QngJZ40laXJ8Q8vk+/nlCLznPnjQ8U3gq5KnCcyFrOUM56x9M/ZYJAWqUfIJsYEC4vLgDBLgBQ6BBgg3gDWjargQX+3dl8LGpmbebBXbVUBRuVx252Sj/seBdIouLo+RC7PhirARP3Ku8V8m+EsLwfjY4wHvoHxrhG/FqTAy1dc2sdcVJkyDJKsxLEKnEW+X7E4iynng7LD4iYDMzVKhztBQZCpQFJM9E4X28r9Kutbg4fIBfCtltpQjRX6mwmQb79jcM9ZZQmgMED1gVE6FiGoylou1b5iMBDSBLnwsAAAA=)format("woff2");unicode-range:U+0000-00FF,U+0131,U+0152-0153,U+02BB-02BC,U+02C6,U+02DA,U+02DC,U+0304,U+0308,U+0329,U+2000-206F,U+2074,U+20AC,U+2122,U+2191,U+2193,U+2212,U+2215,U+FEFF,U+FFFD}@font-face{font-family:"Roboto";font-style:normal;font-weight:500;src:url(data:font/woff2;base64,d09GMgABAAAAADR4AA4AAAAAa9AAADQgAAEAAAAAAAAAAAAAAAAAAAAAAAAAAAAAGoEQG6QcHJB8BmAAg0oRDAqBhVzuGQuFWgABNgIkA4swBCAFgwAHIBtAXBXc5bBxAAPNb8yAOwbOAwVpneOjKGSl+P9rciJjDB+wO7MHVcsxAsGBS2MHwT27a8PwrZRWd4dI1LfrDPJ8mV82NrFM1Sx2flXQhZAHmlOnnOW9MOxAn6a9In+riKtG1GsGR1wQ2hZpUUNEVVXRhdZ5u5yB43zUXJ7vfw3Q++bP7AbRJQplTo5HLgEocjG6aFthUZgaVwu4/4ifs5997yV5McEC5UJbtBAkaJDCEaCIJbgUs8oV2kKL6QXqVI2q/Duun5pZEBuCuXUbFSI2aSAoBiEho8YGK6J69NwYMcaAsRERKhUOBCSilHgfoxCLnhU8D4wj3z8iGfE8Ka5vyU5iYxRCXEiXZ7V1Cmp0be2U4YGckP9F0lNhG+57AtBUOX1zqNN/nR0H7qQ4+UATIA0jSnKgbYDa5gEbbrHbLW6H5QMOM89/gtFxHkEHgLM97H+2cfAj6zpkNDOJ69KbCRDwf7imxnbCbY6EAVQIjjATviQnT45RF5DzQPl82R/75uIdKiDq5SLqb5drmf1z+Xe20/F1ncrd5EHVKGDdqdIsDDnghcAT/PyapZ1/ydxlNqVf/OXMAbkId+SLqkJWqD/vJz/z52fCC0kOs0eze8fZg+xRksLudtcVmOQuZwuIClgRqTtXyc7UGkRhKpyqq6wrPP8/rdlNbt62T3tbZ2UoVSFchBwn69Y372RLD3RVasmMQq5GuQWJ5qCQGI/vwjp4nu8+1bmWL5axBMCW9SU7B2iA0oXK+E+BWqBlFfNCbhTpGK6v28ciVkRCqb9ZPf5numwDU0KQQVIJIhIk2O6HSzXWRuywAnrgz2NsWfDZG3CUSrQsrkZgOAB8nvmhNOYB8ZEEmW8+pFo1ZIklkDp1kEaNkGatkC49cLvshTlnCsSl6EP5I25QfBIAQTDgPuAWCG6+Hj0QUpcuf/ubmlq3bgi4iQ0PxKIXgiJA/Jn5pfkg/kJpZh6Iv5paXghiBKC6etj8udJCIIHAR8DAxqmchwErDkOAG24dAQxA1e32/hqBmbVv+9qGYo7XU+N28pJpVJR4uaO3dTBueG3zR/Z1CXNBuUP+XRRtjHYdkaflB/4+unyxYbVPrg3nFw/012y7wbdfm2rZmecRt7dBbbf4tpuykGZ5vSHPflsHHZd95vPXANw+D62sXG6RY5k5W29e7qNtk6/wE3CPr6dkjuSonkUcHBzwOFtEvzeY+bU/QG88fzt/XKLnX9yt1up6wRP0B/5mmZM8ZVRCbg6saylMSQVTVsO3nbI67WeWdIiEWT6lstgfLSYq8va2XkwFo4s0cydfMRxS02vZ3PSBz23oi21No3L/lTAsPvRPQnxDufUazMTlXPt+hvmwUvRuVAucDdf6vNVTV+Iu805zMQXOU8Lte7LFQSYDcuKz/9fSHr0l+sph3XbvZU8TglPMUT8Y3IwRHsYI0jjjw40jWLJH58yDgA8/evzBBoL8pwgXwVAUnirOf5oESaZr8je1xgZmNt1xqUsOWNMYFRmAiiA+3IgKKeFFdEiNQBBIj3AnkBGR8GXmZBDZOf0cNjtimSEn12JlQRQiUxnVOKiOetzUBB1DDTGOqdaYx1xHLGOpM9Zx1BXnOFsRt7hZGY/4WhX/4K2HyfXv4rv4aRE+UjsEJKJgKlOMmYExUqxDhjEOGOOEOZwxgwumk2MtrljIDXO5YyYPzOPjT+PHHxLoj4RTwqkwIQLjIjESjVliJSVeRFKwQCImJWG2JRjTgjGtgyGrrKZtjV58a8NfZJ31+DZI0T7SIhfxP+myR7jdfG5CPfYK1Atv8Xlji4C3tvH47ACioYEbNIowZhImgAJkMIQBFhzhgEJHdMDDQizghI3YwNpoHT1cCFDCI3jhE+yIkrAdu4RIWFmMGf3oI2MQQ/xIIPAYEdRMJWiZRlAznaDFhEAxI1AsCBRrAosNQcSWwGJHEJER9NgnUXJTR48jBCVOBDPOBBkXY8DyyLFrXLFb3MxZEHiCCJQoAk8MgSeWwJJEcJKcxEqYwqAQ9KQTWskgyGQawlnxMbMhhMkheMldBhumOIWGAx10TD9/6G9gTNL0aIPPDEXMlUKqDNbKZKPaZIrYz4kSGbBhIEYXHiy4G1S+KI2favFXUpAR6YQAN9qpIyzTG3TjnL3R8ocO+uigf6y+S+ZBgN561C3/4UohEPgRjLgf3XHCFwfILEIYDujwTatQTJuMAEJj5A+ucZKHLrqwoKMDAQYVrMY+4xijXCBjGtNBzWKm5jFvZBESBA4CN0YKjdFgQYcFHRb0dSL+b4VeAipoCaxACakgErqEWXYwTTujEBDrKHFZIUr8EloJIjR2UmEgho4YOrpwCw4U/I3g+4fdYSG8yhKH9kFSRubXfOhbfaUH+p3q001ri1Zsw2bhN9altWGVLfk1vV7UI1a/U/3VW0UVX3x2h0W2KNStOUgwVfsDhv7aZd1Yq0EpBGU5deI9sDT0r0vNWkth9kwLmKL5wQt15mcAIOSgjzFnHvUVB2ASXNAHvlCxro7/U0H8Eog5CFRMLELGTJkxZ8GaDRl7Dhw5k3PlzsNM/gIECRVOSSVKjDjxEiVbo9c6612yYNGSZQ89smLVmnX/eea5F156ZcuuPfu+0xg0ZNiIKUZT4EAVGkDQ0ptZWFpZ29jZOzq7uLq5e/hqoJ3cvOKS0rLyisqa+oamljbt2nfo3K1nr959+vZnkB8wC/AHAgEVJBKIphMfs1bgM/AF+Ap8A74Dg5AhyDBkBDIKBYMQuuhD5pA95IzyRrVD13UYSREaiNeIN4hNxFskFMmfFE56THDFoyegt5beBnqb6KECYkdPBatIt0HYd9gP2E/YLzhnnDfGWUCiYrIrPn698UL1uuxjnpofApOwDFlLt2PXnv1y0AXTmezSztXuA4Oo8WLSz06UV4L8iDABy5B1c09Ya7uWx7OTdVifz0t2iz375aALrize4fDRYcamtPPlO2ucKIqiKIqi6ETPQYrHEY4BG52cdhGz9wPIbGTZtZnJzyvwoVJInCt1bJJZVUngjB03r1hFUbjH4Ynwd1ihkRGc09tZxIsJcqLSmWpj6rxpdI2ZszAYlbaordJFi6Xyze006HnuzcURR6WxNBGvlCY5Ej6JnPpKSkpKKtgJ+VqPocXEqXqZAHbB/r6NQbxRonrZRcOk+jknykcZmgITsAxZsu63wbMncyByjqQvrXLUXVTXBcOuuOqa627Ut8re++CjT7brDsWuPfsOfPPdDz/9qr/J/vhb/o0FTIqBG/hwCRsx96WHky+nNGr0jFnYHF4CIxFJrGQo0q7tRnRew+bDO4EWgHIkg1StgiM2np52IxqOpvkU853cVF4lZB+T2OREXJlwp+2NH7HFZFqqmHacuHCt6UTJTLbxSUOl8iUY590fKbtfEOAm/Yn5BxEb2CAbti1Fm/9hHwkkPKuMqHteUHpVFK+HBk1I3/CK0ndCg/e8VxloCf9Kim9YglX5JRId4yl7Y5n2Xm6I8Fegj1IQ4JJzin6cufozBcXQ8tlTRQVQ9/9LPQKVX83JToU5Pbnbp321sKIAEr5alJ4PUb+cK4Wwb/KLAd8lU+i21I1KsMAjsmF9ltLoFmNptoy0piKmVfdt7JJzx7ZUJys+QUQQLqinlsKDAaT97zFRGE75S5lyp51x1ohRY7LI/ssOOmC/Qw474qhjjjuB+ipwSNcE7w02/LWIyBSgYLbaCvzOCehqODU1Wxaue5SDiFcQxKpGDFv7TcgKQyz+U+K0aNOhS0+qU4iMxXxswEzc45GZ8dT8QJDR16mNL8gWDbxvzDctN803F53mR5B7asyyNLu7HR5mL1zA88KsQbRrONieGoqgFwIAlZZr/GeKetl2eePbrW36ak9t3fVlPVoX1tFVsDyW3UItlWVRmnpVF2pdZZRXOZRZoUqiBEWiiXzKgwxkSyghxC0m0Y9YSPhiXLtteqk1mY1gQBsPIxTAqHMVptsPmC4/Yf6GENEa+4R9IgRNtwPJLXpylH+YYjpDugS/yjZXxLDBiC/lphwwh/8AOo+MzjN+5TPalqER+XT2AG6sFozFKmvGDUap0kNyWeiyRD4XmOkabz4YiZWDwVgxGJqOMNAYRhbbvR++ZUSVz/JEZHlQ5maGTEqNsS+ku2+4WMSXgi+x7Jqvan1BOf5AwPMIPiDDW86X39kkp7uIEVxjjRAx1DvfbBhtfLOnScf7YSpLJiTEk0vhXKjyghvxyzviAad2RUpL1ZLWZAUtgc/5I7C48cWFwTOUeIwCT7B/Yes8Ao1tlpfIrDSqLDfh3U0jhYivBPe4lt93kQfvtbuPBubIcYuLfbf7AnPsobt/KrjGBS5zTq4Y99+B3ws8wQqFNXskR6443HkQ8eFPW6Bg+kL/KXgh3nSJbFioftXSErVSp1KNaq35mg0E6vN43P9NJg/6XLCQXEcFFjfTJftOASCB/efsmREU6eMEFpsLQQaqAGHbdOm+ZUgwvGh5PqOAuTA3QDqGgvWQTwHQuSg/8XwBehU99Gh58c1QP/AEe/yACfD5s6SABLoK6BnTR0IC/0ZAI+6BQ5jIYfHOX2QpBLLnHdtC6+yy2xnnLNmy5x8ajqr6a7Ie1KOFcByn49MewiQ3Sm6W3CMRSwwlUyUmEplELlFIyiW7Hp46VTAxOfl3T0IZSdD6rvY4a8qyd/ZjmHJiGHG3RFdisPd2EpcHP2Br9ivJ738ktf8n4BbgADtuy5e258cP76Ht2s5t2bLpnvb3Y9AwtbN0qmdhH3rp2b/xpw3fimhzEALe8sbzHM8b9pAfal1/Zp85ziVP/8T+6OIt8cIbm97aQlfrrfc++OiTbQz1f3o/zRo0GjNuwiQC7wYCCIaE/Z90OyBbAPUCsMFt1QD3/hbcuAEc2hZD1tv9j1DgUEtsQJjA/8lQiIevNs6VgYpNKULYBn0rNHWgNACl7EjMoEqUJvv/zYAQ7124jTyF3nv/TwZvtPkjKHFRcAj5YHP2oDhAeF/2ZjoW5S/v/0m7AfyyAVfVCm+L0bAOWpUmKUNg5hYPwaYTN2Pu1QFuLn/10+TDRll5q8iREheT0FsKN19c1MAe3gFbybpO0vtuIQUcPz5sSha6ZOzayZ+JmWeYz6D73SoOwM9CWJotmcz6AGbF7IxGJLloC85TnYUQ/fshfmNg7b3Pgplu1cfA0gMp6FnGwZzDKXrMMNxxwxzAzBmfBctWXBt8qy1ctmKsB/Yj3t2vL7f8fVg2F5ioKLNcRMor5Q56NIq+jJ1Hqe8jqKutqUNegXcSd6iPnGlpS8gsABJrEZgprlikYoFjRS0QSgVKhXLjzGxcRLERVwpmFQMYMxCNv9HyCDGOPQ0tm35mQanwXeB9E/RsA3SARtriej7K4/x5yXlJSsFL/9RsIE/4IKvXAlOkjj7IRpflapimy9puqguHxb4Y1/mK4XEw6lFnYoZd3etYVkI66EpQLnV5GF2BLjhsgj9RAPSvmJlBJj5IuCo9OKwwM89EJ7XzeS2Bj40hnKb7Wy03NhgTL45PceozfQlmM6cVe36A791IZBLooNu0TJHynh8M2q7ZOWdlkaYObGkd5Jj/BQssWJMoy423duFwo7ZWrEUquR0qR8hNaLPEDc0rI4sARXTnPsZFKyoG1enKEQfLIQJtrLdUB4C7XJ4UCbDgFrQr3mrhKYhFBIvOmtdeRxpEMdST7AgMeFusSEwiFIbNBJv6MmO2CiN2cW0tV9PLush3crnPzf883vnl4Fq9N+VmV6Ji4Djo//ueuNmRTLG8pHaIVT6bzMy+o4xTG8bHwmKJq/lVCxlY/cpgaTm3YDwKrwiRptuY1J8D7ercdHzR4raLaKa6gotkMeUHJl4unxm82tkpuSC6EnxGj2IpqvgeqYhOVnXCeSrkINQOybLBQl2I5URK18QxqxjdQYTJdsssxqTemIsbxFpx3cc+nl0xOisooRyH5WX6B4xajlFarew8szZ6/M+OMU+oNaHEK7HeE/LSOpPp3ag2Bot2kTshEzo0l3VsSLifq8E2C2pUrXZ93NTOs4owUcwUGIuH4mcHL9Xuntzflct9FS5VVEEvUZO2uw+4CfcLxpiEZMUUI6tYAjFyB5e0h3EL2oxz1f4m+Apo1wZFzxLGSUmcMqm3QF+LUyMRtJEhGqSTnBQMWaetbRVep69dyDYbBkJ3g6xSTh7FaMPeDPLgUiAxT3YpIA00I5rrL2eZgpPu5gpKpRoCRVEd6d+PPCdIYBUyG1Dd+UkboLaAezPetI97FfT0vLIqMils0LwQW/Wck96F34ZqG72XcuFDqJqd7skQs4wGzCAAyUY1dRs9aNFgimWlGoOIzponLqcDCi7PIgEDosokDR/W0cbojkX8TlItG4w1W+erAtUlvUhhpK71TXNIYCX9pXxbFP6o8RDIZTIfeFRJl4s1quPCl6gRgyC+s7Uo24mwH1BVvY5nMbAuT9Sy7+QWBImKqMoQNqEtzt7tQ+YhB9jULePkZXHtxJpmI4T0zsUSwDnQdnJtsXLJEGZFgyEGiGncCAOKJUFwdEFvYu0tVuCQtQIGj7Gu1rdraVL09X1AfxhYBLKZHZskY5QY7vygbVAaM/rMilRbOktSbSafzFznthlQSquIn3kkpKhMg4EE2lfdGlVr6BH/LZjLmV1YJFtlTmdlHZK/+NM8xcB9xph3yC32LECRya3kGlzChmEBT8ney11cTS8f5DvHi2tJQQofZCg7TNAhBihs+RirOYbyEiou+gTdJrQH9K0ZuvGUgzVVKwrWksqjDn8ITdsODppXwmdtWR0W3RpUIYqipm3FH+kmLIjonyuBL71PVjHlX4FvhrLzzH/IApqqKXJlLX4Iw4sFJ5/0vXrpW5CKB3bOnJw8fhu2ajYPQvQ0TIhtXjzTsYPM1SQsWOvCrCkqTBeLAZV4amAFCwQsw62+7Mhqk+j6V1eVqJ3+Yh7MFmY60/NpVQAfUtBqjrBTqLEXpcRFvv5kFiIpU9mh5uCgg9xNCbPTFTZasFWow5Nz6ofxryBii0BWSGJcoUPujSpuQhvKs8iI1ueYfpD9DJCvxdkRQqzhSkukCQQw0MrS8+ks1nN267Eb0MCEQP8QnVvYjvSEZ5CzggOVrSMbvzNIafm3bjiZhd2/SkqoeE2yApk1yy2uiC5XUhuHq7lRHhtzt8wg24xuQwzFA8RCrkRulDAfZ6JeFGkUzIcGCzxPhLAgT41oRgPwIu+t/N2vfszIgs5JcK4SSSS2I0z5oBOsFSeiUppC07AxthtEK+Hu7bBiOhZEUNxhN9hlF+c69TkTU+z3AsuGZfvK+aN1r3JBN2dtjswQd0EJKRR3sOegR90+VXCODroIykWBWic3bTdAF2h2lj/JRjKAJJICOvr9GJtja8WFs11iWb0fG0TDwJiMEMM6HEdJnD3eOfRMTb/+Q/ldHQsWBgVt8SLx2uOXgrAfq/K8qXjGi9nMJlC0ng4vcsXtPE8CBYK0C5oSPNLvKyH1f4Ebt9TOlMzbY7FizS0k1ewUso+4NDzWO9jFpQQ/FAX5O6BZ3W7+r1/b6iP97WdXSj/z70UTsoy3rIOQm813IMvLBIba6JOfNXkFSxr7fovabAuvz00P0JEhYMQPJO8Ws35A6RvnyHQCFBq/xiB8T2DumzsckMQkiDMHqGp+CCnnADyStgYWR3v5NNTRWHpPgK9KPh+fKth6NemwJLiy4qVhcb3u7F0Fl4Yzey3fuLJOyVfXdcuVu4W6ZxW/WmbWkdRfyt8+XwKZEsU6IPhXjMHF95QvW86vNR/buP205fTA5/4fC0Oz3N0CfN1t8X7/zp2mnj6qiQ4Niw+7RccqRydOrO5NshdSk4mE8CBAtV9Ob6hPSauvT8Yk1NelMFur0xq4DIysdHUMrbBYAyNrKs4Gfe6CIqOlhpnUXJOOjq+tP91Ym9zAqW9iowPHWBgaoowttXWMLHF/flairxIJFDSITPIOqr9zywqPyEc1LU2yKmd7Uxwdi/wMPdCnDbXIuStCVU1rv8XmbqVEFnTz+flovLl2+FuyA8k7LgaS22jlQw02fL9uo2FthkBbugJeZ8IZRiVnD1hk+i66Yg+VqKeQnoZv7eIRA2uFxo3iaAVGot7+rKgyEKb7PLLsm5IBV2Dk32p9qnzqZOF0evInBWas9p5mLINnCURj84q2avXXiMK294WdLxFUm1Rtp/g6/O9Lt53M9gYWb85RnlPattuoz6n526DXL95Yw0GeAXwGnoA9kOO3ClS8Qv1sEP5T+BvBNLA4fT1DLBFrBM5WDl1YvzYE/jzvDfuos//pixrUZkz+UePg6wclqM0P4ULIYBQ5pAM6hJsPm4oihXRDx2HyR2dG5IL6sjSAr9RtivgR7cpM/YixnuFxmTHs2nmrL0m5q59vnQyK2k0t9KRUA11PdF4IqCkJ9AskhHrEhDqyi8MfrPrQN74/+NeD0uxxhXyJci9qXPDtw0cCT4+zU2jkoLA4MuEWM5qRCeTKPIfGOvXZChJ3rhzy8S9Tju0xbtKg5brJM+EbCjlHL43Y5S2+50/+9RvgfsE49kkoJzrUK5RSmZKYXBKJcDxvARxPPJiRmoP+vK89RYzqGnl+5O4TTsGtDWEvLstYYpvLpy4zur0UDneXOfg45g5vbA3w9r4s433533TcOfj3O3jKKAYE1boohKlEPSAOczy79z/n8zOk6J4QJWeFwNqA3r3/5xf2/heShQfS6KvUhKAASuzTqGip38lBUb0BRG+vQFJvIAnYvnaPpFUNvxF82HHrw1pc+lOI3zH9FM9yN6jk+Qvr0NcDT4T9Yy3PY13vJIw9ojTTk2PqgEO48Qu2vTlpRMWcyAYPNitp1oXgMuMC3i/wf72nBikfUYOU74HEpkS98tH+QgraxcnDwcbPzZKN+rYXxmZezj2oKiyPitDDQTLZjppYjC48lhXUr6T8R9zM4ceSwU6tYmVDhiEup2Dv6PSRDVSu0bZgK9plG70byqITiT7xG1y7RUJ1mPDx62NtBN5t5remBKnOJasuuQPjpKlFRf+/eesOc86P+I2Z/McdtAPNcNZGW4nuQ6IgE5tmrubqImlxdVtUISR1GbgpWaqQLHjOZ7LenCexeD2lah85AjVQ+tiD6L9+r4BK3yskaRqLssD98LdW1tQZciLJ/807Tqtj3+fQ8R6BUa7xXyGkbePE+yucw3vI6kwF6EDDYraGSMP9A2MunS+/+WU5KRk1rH30rnq7/tvG7vzEY6VSXpWtI/JNVZrz2KVqDcWGCf6MTbGaqdXv6F34zsKPfvAgpNgM9812A0pf23xHjg1wb/fH+f8BSl/5/O02HAgFp0J8Tvm3PzhTU6QCvVyK0ySIs6fsSbz6bs5N28H6jH0q3p7k3Ddqbit5dfgSMKeBsfpmJpfV/iSluvyGtWMJ6sWkiXKzx68Tv++8+vb68u9yAjHIJ4JBBg7E6tZJmpuws7m54Y+dBP3C2JWrIOt8/mzOV93BlAmWmL6TmIoTGqz7Z7pxYpsenWKr7qdpL+Gbg/BQMG6Wx8d3uANmHUJCoxD+un09FyHafL/teHOBLlhWrhUY5O6ql2hjPhzcGiN+HEb11d0AfYjYP06pre/414UOA0kuH5trnw3/XL9zeueP5awiM50aEZ6ZFgNRB/Oc9qX46yS7H/9szFX9fMmXBZ5q68DjjhOaI+PbNo7/PnK7m6+uIbfDRkwJf62BMf6r9Zy08RgVlIvQEfdRYnEmLZREj02ACIDzQ7Ibw9fV3xc+SZlhSb15W7QTwlyk/i7NoCVSkvxdzXLxll4LNNfa0K6T63sVvMScheQ/lVXMYjodDwI5N89t/nr1z3wCCFxjLby/JDw4Ysz/+dLvK125xVkyKpNA7XZK5tbJsxOsL5Ia1glgdsdPmHPWLy2uvkO+ldzRDjl24r/cfnGm+x71Qq0Cs9y7SkLTjzwceSZ384TGCdnRZ90PZUDwn5C201IJRaf/6krlFp0Bcef5L7hVKxyHb89L3wZGE8s1gBpdM1AEaDeWA+TD2jfhtvoGcK6tBNBurByoA9ToOqBvVds2vyi/3nR5fQG40Ibyh/KBJO/hrJBUC3BMMH1uRdRXLfQkt4CjX/PRVs5ebpb+FnB2LKlk1jZm8NUgtPl9ziWbo9A9GKrBz0tLmExnG5STBfA+L/r7mfnEoH+SAPEpoMJPqV90++Sri7w/qH3R98IrdJMH4WmVhOgGSBNwbnEk+3MYv+XIILXOeJPe8BnjeoM0s1cjh88oh99yJISTncJjG8FuUxa1GYTI/HwrHWv2J8uzgQqPmEWBCV2DH5UOv29e2E3K16cvIuLyw2N7Qcyxd1LnDw3vUv4+b019tq8HTRvjry0rJdf5MeIpcLfHeH5kpVdUqghlSZRnfhgF3Kir6ajx7Jga64Cjj3KdIqu23nd72vGUAcJlfn+yHO/xp/EHPjmzxvv7+dzXHjBTQnYIvToyNT4W60e8N7hvdfB749Le8FxUzFP9qNwI+gDQHhsPT+5y4EwZYx8vdnNyyUHHcbGBCj9hEVvORrrOKCwdjb2pjsbQURigUw0rqa1urUQuD9spRkITqo5/r/Hh1YBgdy49zzF0kTeTyR7tDz8DYp//rxNoH4dxF4fRJ/83rSHVrN9vnYCc/T/7bLa9q5+ln91xNHj9c4A4PZijFS7CloMTmzDuSnLWmAVX/Bz7VLadh3cumg5ly4GJbGXM35cNeU9EzvC/I9dF/XrVWPBWVPHvD3Jrcv/XhjM91WlDkeTUfuANFR19aTHcjidC2EB58IyvAB/XPflSlvs7ZQNtICIfHvVSL6aAGN8LQi7+fWs+2elPFcI/BpQHP8dyQhpHP1w//L2lpp+YZRC1bUDKINJ7wIwbTt9kdRqxm2z5e655tmllKpJgw1gPj/wms5DrtK1L+9TXNbo7VMz29XWyMfPC6tx01pbUuWtjEmmbc3CwP3HJ9Vad+zZnJNnJ080TA8RrejxDHUNOOtwzLRr6LU4an1b1bu9o5wE2w3h8zASrY2FsgH38SlObNpWPLX8LhmXCKu5fOkTYyqlq2G/wVjJt+rgjSoRNv9t6szUtSofttL5+9voBgNuXPYVxx61lBO+WrIcJW8tgjwuCJv7JMw7xq+ceIjuqBydvODkhxEzOO50aV+WGRSPw0eBKmXXxcdMSkOLr5sHtuvZqpH3k9Sr0QMeze0oKqc3qXMP3njc7NDu+WeMa3uUErITqAPV0HRBv3LvJMXHtbad1p/XHJY6Jvc4KqZ3KbZs9069G20dfTfS1b3YoA+MxUdbA8wwcRb04CXFWFmnkazXS1LmRh6PDshIQ5+SQliFWYHv3Q8hSKIRXoX4TjdNDoHE31dE4hB4aB0Ja7Mm+XKabHPXj89niH6c/dp2bk3oqsTYn3XXu1fTLcvHu/zhWbXInk4iOW0ecAM2iNoOxSO57TF2rbnBeb9bCTz7Ykbv7zlQpz8GF4fc97/7v/pLs5+di7xPnx3vgNAW8lwMqqHOz5TG0hLRMRpJn6cR0YHVmTlpmelYJYXi0hJiVm56VW4CvmB1xL01JS8+6GR8dEbQwQHw6QApc2A2c6yc+HyAGzoGpx0y53ISsZj4WKaQoFCpc+22lUqiSolL0PWrmKGCyxV+LT7/9+hpmjUx1N9oxPHtahCY+fP3j8dIBFJaSkyLTXEyNEx161fqUB4KcioO66+3hSkimV6Po3NY+89kldm5WbER0iouFFdkm3nck1L5IOcjsuKF4gBzF3MM673q7ygvCdZI50w1QbkcpI5DhSKQXEhmOQE4rPvtnGvi8tipqvQPvX+0Iax1crahqrs4vc3O0sHPyYYaTwn0THe3tzTxq6LX5EkcbqypFqkqlrUqkRWrhog2F0uYidbO99rhJGnay1z6v1x49PTqCmeqzB8nDIkgjc0p4fJR/cF1Ei8+NWKIeRlmxWcivlZaUXJxF13I/Y+Yad8nyQn0y3hBEairZAbdz4dOcC5qQIOox9DGbi8yp8pfAhoN5ocWZ4iZGDImsA5e4EVzFjSwQKR25baSHMC5PzDRw0lZDoWBOoBXsawTvVdwDuqRxfHH508KSwKCSkqdl5Ximqj5KVUXfEI829MOggflYgu2ULf0x3XvKO/LxMZlf4ePmYN9KrxgJzmIfpLyVCkxPT62XWF/EzCyNvRXg7+1ukxp6C/DGg8zp7hesdBThshd6Pauj4zPvRviTGcp5lzLwrJhMZiM1rFdWX0VLd1LeOxEkAKEaOHH8+hjQ1YdRIGl2cfh6WIQoW/6CO9IRnxtppDZ6CJQHsJQ02+Idv3Obb+KNEeDqA63nYVhjBs4YpmmMhesZYoE7dHO+WI5/E6mUOwmBui5/DXXelFO6CaCVPbBphZzg8GOAp9z99uCo2tBILaayb7S8YmCsqpXTwynIzMQhAKSYucd3wdTEKdAHVORBolWyMCY+/vZueBd3J3s7f8958C3dXRpdfDXdXVNy19HJGM1HOP7V8SYKR49Oqh9GHKgIBTUcy69HVOdVVFkvJxgihTITEsdmCs2VfCPFmCAoQFNPUy83H3bt1syDw+BAeb7Hejg7qhNwDvkNWTujo77ItXzksdb2iQwvm4y6nMPVZ4Gb5rDhEipW2hNmY8XsuXo6zVN7JUwqV8ZY1b9D+qmqS2KdPvjF35meOrrL/mHJBkrf4YM3X6uy4p6nJkRCwF+H4eubO4t9670NOmLu+qEn4HJX4ZZtuWZJhk2PuyfNGmXtFEfnqDJq7vbWSCUDhxamGdPMLmba2NzRzayb4yuEuB3/07wObrRmyWBN3Sur2Gn6MIR2dPGxcyTYIQ4EOb+P/vcimB7PmILc+gz92PiAN6WwnJGM1xjB1BioL+uq0bphTNjsw3FfCNkFpedkotz+zpOafIQ6LhUWGAnsL576Q3Za1MZwb3ampbyKiXmVlB+6tUCh54Q6KNbHRM4xjAObwmLM71zGje4osRj3ycaBGVuQycTJhJpSl09NqwsOSZpqAd4E1OdbvzT7FFhDAhanj/ToMt+Qs6oLBoGszIJpYLESxVbP3vC251AZ2d5LY0XwBjrf2V4SnZPucnEh/9ChTNdd3u2txnGLeGvzNJzk7G7fiE4aAt4E0W8vpKPt9L3T1rHN/cNZE68vcv8gtsT3hKM7UvNTzobSZZBeeyyyjjcnVxCyHdacdZS/uz46bzn9RvhKmrYZ7sMoSnj6Ct4sEXK807oITjiOtC2DONnvHOmzH7V9cMsu50Bw5zP0U0Mjf0pWOck+4zJKyaQcpTMPj/jWvDux5etLtiX2ndyR2BNJTuwermUMRJCYPToivdbkpGMRnWdCQiOBngosGuw7MlKHChUeDIRRhgOBvcongX+7S/NKR4Q4wnag5SPvHBvVSCG7uXuVG6lRIksKevDrCnBdxes6CA/cc+BA+hBMzXaTo/lpNonJYq7n8QRT8101bturyNopgDPVM9CU5Ne4fkItPr+hdmI44eIXMAXcUQ2NvnZaxsZoPgPw8psDCkVHo/BoAzrKAPBrxaKNHOjQzKK1FJDsswwYvyDts4+HZlfcUF+/ZbDLPMrYigrNzd1IBAneS6DPnYoKSdCymY1ekKcWpuj5zVM/4rbpHPlzG12e3k8BuYTDNSLCy5cc4XHRJTzc1zOCAHECrqe/tm2VTjwmnVgHuAca7umxSNMO2/P/tLkDjnePeHG13G0qCnY5Kz5HlqABY2ketCu9meY9dS2Cf2eI/cmlS4nPe5/zLrzTxFvlme5F8wIeiXzRRJ3oW8QQiWQahU5JlAjZ7U/pI/Wlu6YBj8OC4mmd6lTvEg4s9O8ccUwyyzlnckZmOlmBEOLp5q6/1rHG8A2VQlm+rrcQbrzbsYe12Gxvvjs4fGd0PAqpALhe/5zvtiZCj7k4zVS41cCuK2cswpenL3FsSVEAmJcFXgwLTfbVi6dgbAm+/13+vzpW1pGBo9UW5GdVpkCoc6K5VzRiVVydNB3g1x2NZhxmG2dx9zk5CbCouO7Q/noRxYrzF4EN7pXlDVk5FQ0VReXlLdlZlc0VvQINJAK0oruBHMJRDJjXK8u/RJI0XAtdq/qqfHgjAr+fKt/oPbwNqdQb+dsFVsdyN9AJqYlREXeL0uKaEyAePZzmBFLMTQQSgUSDaRfOF2cnuQi6o/QDSIz0KHJdESO3sbZU6dHeu+f59df0LmzJZMeqIg3UiGD3ToumcQtqR0EE6PEkhqolaNHwtyMz0qoiAmgJWqFqRP8SUlx8CUlcWvUwDq6A/3f40bPDZtxGTsHtCHjjoHfTgcpIjoloqKKn3qmBFGj+9+TxCyDMzYww92gyuWCA0YOr3IAba6hrIoGgJt7IxQ91M97gsnT2RR9xQ5dA1NV0A3lJ1kXmp7HOlXTgUXP5/MTlz1PHd/rHWGB/S19jX9Q/Fd3opL4drZpb/f3NF92ofa1vAZgA0V8ANBD8G8XDgalUQ29yVOCJCqpqPuY2IwvAVdXS8RZEXdoU3J/M5fJ06pA48K74MDZhjBp8A1E6mqty2/24mBfV6124DoDsIbAB7hwdbuYLdj4DQQvwsaEWZPnA17ocymuya2Jvoqr31vreODKvLOWRbC5zMX/VgWr6YVAX0UF0Tj2sHlGPqsfU464TDjeI/wO1yoDSpnA1J7R+z3ttD/989TNu1xS5EEeuuiKj1e3+PdTpvzhtzfVFkHbELnhM0/mZ2aOYX1N4F3dUV4RZ3a5hONcBrKoVe1l1BYfq9u00nLvT3M4sX1zmEpjI+F9kPBAZjxJZCJe7aHFUXGCJS3lfm82lgdzdcwP4HFDYDRotN8LMQen1L+Odb8Jw7g+hGXXFCyo7+aEt/roeiL/DW3NB6ek2mPtVmscVPx8OFQ9PRrw6TWvAQtBWGozf56rFHBYV1onK3880JjMfHM5V7bE67JVYTJLY0ps0mPtjT5Rysp34HD2zk6kOJ9D9Li63kpJ2bpfj4c2cGhvBpqhgDg+DitOZ2C3lE1wJtQDiaGFoR2Fyx+JkGeTRVMAAWnnjQDs2ovht9xBbwqwkIU51WlF9QXgdp2GQL7n0ZRZLoyruCVwLRfEqEz+sCF5cvU7QgvEhdz/e3DHsfsUPanWQCar1TrjtvGXBw7ny7ZpJe0YIcHwO9TQc7Vr3cCE3QdrdmahuLtc5/O7fagm0qJiNN1qtnDC9r1NTdJoRx8+2rb6EFeHN7bTXnhT09DSVPJjUCSORTYwkSFRLXR6xy3wgISqKxEUPgcSCEBdjT0ss2eKuk0SSxIlB7QXizqwkiv2Uf1NHEz0P1qpqA3TQNT3JovgeYDA4xaLoHovMSCsyEEMP5FQXuF2OykZnMaxwwE3saw+G+zad24NiZnkCmWUdrW2JMLISZHeaW1uw4KUeShZQ+G5N+oGhhC5GQqeNoc6wvYqtrcPDOf4XO72E6kqQjiw9OVxIJfZGOEuPICv3LI70epQFbNxJoGuAR5NRpsVZx3B4DPnx5DQthH6KvOjUl+l23M7XHhh6PtDTe/gbHLaJa5gticfEPeSMeBLwLDWHeBVY7p/QuOCGxr8sFu1/9H5BGfwD8LP9O2vanPQ6GknNP1rTSlwNElGxfyyYY9MkTL8ze2TfsD9z4n9lPNlh80VvBXXRUlLTtFl+sPL7bH09xDwbYFMHwLv795gF4KlaVYPDEljp1TjdI1qOW4SKVlCw0zO9HFcgLnZ8K7Oq5F+0P2E7R8wefOr070NXYOQ2TDvI0bapxYO6OZ38ig+LqAWiYBacaoOqrl5VoR9cnqzLjY/TLw/04lN7UHWW5pscEMi1VdkYqwNo+6K59+TZZzx1AsRv2DSclrL2pzI8HUl14rQVBlUeTpWCqk7RaaCfKXEn59ED4Eabj8a+hA8uSejl5t8dQrU/waQC3IhaFfZBkJPzQzuojWj7iL5ufu6dXe+cCZFg/RiNUI7O/QC4EReRuYTs8WApuJGBLvjvVa8zGKPwsfWPZ6BQ5bc1WVjO2fPGXqvs/tkL39d/OmqaU6vO7JDDjuaSB4jQO1QpvoJeEU3ogfuTLwTCtK+aSGuEWeikovSsqQdpUgPOJSfCrP2plx4QyNnr5/y5WOie29ozwQYc70Mx8syd0izfEnfnvLN+h4nzufW5PLXgyQa01qz32ITh2HNtd13LCjEscdcq/+qQNNp9WzRr8Vy9xklXXWoEArBHm13Lum8kKZpUivrT2Jkgf4+FFa6VUTcs867eyJo1PJe6j+lnenWh3SEv+8Q262pVZZwBq+ncS+1vLW92X6PSaf9+wbrO+nc7fifgXj1h17Juyx1HIzw7b8aXCK3mqIBeiqiWkCdq1APngUrheHKc90gLsGOawPNGfzsDKa8oBKmwfQgJVS2svJpRbujRyNBLDYdWp8354mnRplGpmtUTp7uspyI6eq4LQRVbNrRwKrTGK5WxjgRCralTqqEQ5dNtpJ+cf54bU1CcQTX0XCmNZwddB1xqUmac4dXsWXSraxksk1znJGH0wpYvUtB52+lMG6xX85loaCVpaouOpOVstH6rGGk1r4RppCVFeTI8Nz9tf4+A6rnr/KF7J3lafyf1bgDAHz7wDwB/jfJvI9P3Nj3hhlvAjTCAgHPLxQK4cfNl8HvN+Cf3TbasNBnc/l/XX33aq0hmv+bWp3edlzxHUlQcKGsWrR65tBrpdwVFRknUOn6lpX7Jw6zkpG3ChbJXrYKP2e+dWNJq8s715pvZf+lseYvXJq3TTPxYEgwL1KMha2DUzYh4j0OC3LnXUwgbO4zVMNdidT4hCUObVsGwpOSQTum/B2cVrp+c76yTqrlGcDLAZnjM+xmeouvMSsnSZUeUd4ZVmMdhrYYXqNl2hLiOU9mKQptebrwhVa271o2UHWZ+CUBVQSYP9TUcAWg5vhr6LfM7A6aV1Hgt5505J7AakEWl5q4vtOcHo6bCH+s1weavrHucC4M9T4/+hKsoKXUdI6M7JU25xIZjZkxDfxe3EiUe+qJXttb1mm0HWcQO9kTp4odFjZNcX7mVG+dUqxR3n661w6AQ2kpEwfpbY/GqM3e95jzL7I8rwg5b2WsT4kzcmlYIHrqcnpXX7yQdIzBdJ7uzeXeS0C5UU2Lazw4ZJ0x8ye5Da0tetZu3HosC/zst+P8ywf92C/4/R/D/VkQgN0AAmCFrDkXVGeaZfrJcobxE8pc4z8ULdnVR0tRqKoL88hexyliVhkO3SBo+KOgEvV1Pt0+yOqdhLcAN4xICEItBgcgJOaBQFGw7toW+4H+XmfKiXKcpSiq47LbEtfcN8rVJ/HFA2nNjtwdTWraD1LLEhgiQ2APNaOKiomfSWhJ7UVcFSYU0lqewYjKLQEpsH/Ro1/aR6M5UlsReCOZCIgHgANQLCrEPlNhee1w7lQA0gSIcFSQbDgvSDQDAMUGiIUmQHACkBg4OUs8HcAXLoEigYCAFcLxwQBAguOMDEFBJgjCZU4kwaM0jID5wYxx8cUmULqilb2vsDpWgRKuqbODgeQBQSHDcACgFKCIoAyghKAI+BIOLznMXVOHlBErBMIcSoKSgKHhRQVnwgQfIg96ESsw8CGSLdtpCUEpdHuy13SALDhXRAXzcSWYUIrZhFIZD3RJ/bElgFIWJQkFFjRCcrwO+qPzVi8lZu+Y6UqmKi0i/+GeSKlIq28mXI30et1CZTGVdBfHnzUcIVe3aih0pG0pF0oTK6eIEy5Th+1coECVTqcjZs4UkU8vbknHiyiGbtBPlI7LzrPlSlWpOLBHes1NsvtJRZvvhSsK2C7+LKFpxvsxx/RVKJxVeM8qPKmdlGQe+wKKcs+ZDksIB7FwZbg==)format("woff2");unicode-range:U+0000-00FF,U+0131,U+0152-0153,U+02BB-02BC,U+02C6,U+02DA,U+02DC,U+0304,U+0308,U+0329,U+2000-206F,U+2074,U+20AC,U+2122,U+2191,U+2193,U+2212,U+2215,U+FEFF,U+FFFD}@font-face{font-family:"Roboto";font-style:normal;font-weight:700;src:url(data:font/woff2;base64,d09GMgABAAAAADRgAA4AAAAAbFQAADQGAAEAAAAAAAAAAAAAAAAAAAAAAAAAAAAAGoEQG6QcHJB8BmAAg0oRDAqBhmDuBwuFWgABNgIkA4swBCAFgn4HIBssXBXc8aA7AEM8Xxo+EhFsHPAIW/xIhG1SlO4E/39JkEfMqDP+K0ghJYi2m3DMWq3E0GJaSAASTmd3x0rHWD5s1ESniEfs4PtZjwu3MCnvvRnB645eiqb6yyKN8FBXm3RJD3XIjQNZBLjjFcq0P/GRjU/r9fTsSjIgcwREIQBlwJkzK/bd3/mBn1vvr4gatICw0QLCYIwYFdugNzZGxka1IBJRglQYhIUNoljoGYhV2IiBfWLQP3Ww/RNZuR5RABAHmKT3fn7NERfiCA2ad9Gk0kyP0GeR+CKueaLKP7F/uu1qv/2ThoSqlcwQIUTdWySRSBLJgVC+y8oJJgnORkTPy8YeFGBjiMwSo6GaovUf4s6eIkE6LDVtdWCDwBlrP3uk05qRLMPsrmX/cwdI5Vff7a5MFznoJD5gwTRSrpOconmC9rtLUT2CbAceyCrTMndyCh0QQ9V32uNQSOsMD4ygry269JQ/6JkFprdRq0Cuwkks1VCdFqDAgkqdsmjRUh5NDDUohTSlMLVh0fHoWvKVtN4wXXoPlU1m24xEjVuPUO/dF1U4+aYNQlXXGKzEInEYj//fXK9dYFm1rqzMV638zr29M5lJMsnCZDG/+AnYFoVCdkDoRE+FLNQGMk9jA9gg+0zE5ju/0jw5x7V0tkIY1SscIYRRp5PcHz+1Wps4S5R60EZ+fYxZ9+P2sIIG4ES9QDeGT8UghjN2EQJFHIAnS/GabCCchUDkXhJRJBElElEuEVUeskbdA9WsHdo+U1DOuwBxZUqQqMYKEufEQyBQwIWBc0Kg5WrHRQhqRgta0Xb5EOAsJDYwd7yzuy8oPiE3LUFGPSUtOn7UM8MzkmaBARxeCzzjSWlJIAjELwcGCqhz5BCGmt4w4Ixfb64MoEzBxnmsj4yyfXvbncjHvZS9Gy7EL2dJ0E9Xolbt06ZjKdk65POkbRnF4/wxq10TWAU/zj/ibVaWT7kosVIKP7UY0Me0vtY5/MpYTdugkPLh1ah+s1NfVxZMqxvarx22Bo64EHR6pn+AXujqpjBgNimyR1aQq1bsMGe4S5Kk8oVSiSkjnmSdnd6fROAhKuueZTNbq72Dbvh+8xwEZ/1D4k74q+oC3YAd/I0qnWGoXyIW5+DGNuyYEbHl/lfnatvo8JNzjhDOs3pNTYWtFJHSOd11Fmqh6AxN1yZhWYzauTXHmqYOfnELz+yo4+uRBsxx9V8enfPFunH09ccbPd3dBFsxK77F1lP2kw/YdEllq6P+1WTOyVNFsyq6YJc5bjIZRamo/uS42za7rm5gS51ZeksHa5YtcElwDhF4UaR2NM3Jdxj6TAgxZ0OKM5pNGKjMvd3Mhy8VHNUEtOqChNBQ3WrrMUjH8O8Grlhn6LsN7ADSEBgcgoOwCCJCEB4RC0RG/A1EBR9J0ZGziI3CH4uLYvTwsjVb5cUwZPmhhKIoZrFSHFrcVMYjXuriEx+NYYWlKewEak5wgu1MWMJ0JSKxusML3wAUfgJIIrnLkKTkcphlOuJoi6InioHyyKJQRDFTnLnCLBRCVZal0qyUYK0IG/GcWwE0DAi3FuGDCY0lhq9obBF+ivIXK0BBQUoJVlCIYkpEqRWl7hOi2y5yevSS1GetjH4DJA0qY1gZlw85Mf9Z4n6T/0R55BmUFS8JeeUVEa+tEfDeOsR336H9sAHjt38dPcgIrlJQGxkoHRIhRAjQEUVEQTxiiBiIrnQTigQBbPCBj2SIRWZALRnpSboTIlF6QzDKUSEZggU6xMBFLQSiHrhohEC0AolOINENJIaBCikwMQpUjAMTcgjFZMAWp1eaxnQ18xCJeQjGYkFqqFrGUqtYrXQCHfdAwgl0uIGOf6ASEuIJHUQrNgl3QiiRIZuoEEz0EsYkZo0lpMMLfPgNMSgpcMoTUR5eJVIq0RRFy020laQjYotYBgozVARJOWQRJsowV4yFwqgqsnwoVPICjygSO44LCqApi6Eg98LUEwzoEtjtSL8KNYRKfOeXQAGRRwl5lHYlV1IhAHWWtOwObA1BEEZ0xnU/y/pgnWAZwRSaEEKyZI+UkqPCAOkSLiASJV49FFBAFCHkwYACB6pz1Ywm7CEY7WhX6kTHLdlyKyOCIKBBQJdwDUu4IYoQogghitCGCSPFpriGQNwCiWdg4jWIHHeIlFxJwYDZkAR4yCRwEDgGkS6xqiKMIkIoIoQCEk9xSPalA8GLN+7GjBcrb3KbIPbX/DpXe7mv9sXip3q2C5vZpFZpUfTSuDIGx66hOzRqpR4y8XYtVG91VnHu5G7MvLKjNV3yDLbuKmJfC2MpGPAsEeikVaEvdp0L40qLTiaYQ5MJszRXl/Lc/AoArQDdmcZ/2FcjwCdi0I1nCna+NP89FUVfgciCgSNClDRN2nRsocsQCZkJClPmqCxZs2GLwZU7Lz6YWDi4AgQKFqpHr34DrvjPVddcd8OSm5bdctsdjzz2xFPPvPLBR5+s++6Hn/73y0ZgKGKs8+QoUKRE2WYqCIjUadKiTccWugyRGDFmxpwFKktW6Nx48uKjRWeNM+78F7DghS1yUYtezGLHo4wG0AEG4AawENiAH6PARNQB7wMfAh8DnwLrgR8Rfkb4P8KvCBsRQy+Y2DJOC0wHTBeMhJgxYu7BQjMSZhBmEbYS9jzsRdjL8MQXHhkeBV5EGBY+E6lM+pgMMhlmgnBNjBAWpDcFP0J+hvwf8itkIzQSNGMIhmsyuBkLTFJveIheF2xuGzwajA4YcAMbdXiPD/iIT9Z6emhoQQe6lrtvNzdQhoMnQrwYGfZJoeHQDQy4gR2FJ61Oyz3hHel+MKDfd/pDxkd8stbTQ7vK4bcZKloL6GhdLHd/vnEkChkyZMiQIUOGDPnsZYhANHHCIa/ezMT9zu1KwFHAVeXzrkv0Ll9UkUvlnKo4DEnDECPBPlEcovDEO5CBpEEWkSBMnvL2swuUlOhYvlNFRpV7VeWKCPNiqEmsS3iV01OEGSxgGYbNUovsXPRUS6xQjyiW4aVV9fKmJgdPwXsAVVRRRRV1UB46djc/CjdxRZPPwETY3E7O4a+cVFwxzYYY1MOcDGc1NCK6gQE3sMCO52D4EwTgBJuu66K+kRsEw+5/DbmKa7iOG1hyX1fzBm+xindYc98n+oCP+IR1/MBP/I9f2HB/J/iDv9a/6Qb/uCEF6RMnUUNpbqKhqalNXaGhtSZaJ0MXJBi7JoSZuua4UWGl3YnCyCyqCmP8DoLiShZIgChShyYWw15LOAWaeh+4lzdE/UpJZyCGSoYlLdbkvPIma2nlwlVMbmawgKUbSWbROnZjkGNlShTzPAvzlp+Ihi8sduXSIA2Kwdg0Ard7uDMBgu7A9JV17sy0H+V0Ly/30Qb3tufI+3t59D2Q6Tbyf0yyBUtQh/l+goQId7OFagXuhCDQVZDEljHgJATy9PpFnvCExBSofeIsORHK/4Op+SD/mbzYcMhixI+PeGZSZiIEPTM5MgE43ypp4P3cTpfrC8k4GQf2BkckSmgIKvcaq9SBZNLM8BKMQcTU+S0T285v4xlOY2LiEFFYNK5VGBvKEPMPHoVD2CnbpMswa85p8844q4TJNTvkoAMOm3HEUcccdwLuqoyGGBsp2cCKrytCxmagoIwZA77lBMRcRTWtrVZ64vziMLGPA0Vbg+HLLzinLKsQ7ZydElly5CnYpJabyRhR6awuujTr2MQ2dqYnE8c4GXn1UsouF++UgXId+PdnTRCGIbYziUThTOgmvU1Bc11Sm62MfUM9THpIBLJUBoTUdJr8keleRp3R/A5rTuu3WiuMD+PhWBxHR/uIGNzhMkyGbn2vF7VY/VVfURVQ3uVSyiVVgsjfrOV+LmY0+UlLWDzjFMUIwgcLDhjXq1W1OBjK5OAJBwsordGH0uYzlGZfoLRAYCIrJKWTMiTlx02HX26PZGdeLA0qFEh9mPWn2NRMlEoxHyBoRb8FDRCMvILiajeZmygTxAxuB0nYpo7ftkmPPx4RLtoptFbsZII77SJDdQP9O37Zxo4fNsnxs5Ci47uhK23u0SM0jzi1hA75ZgsQs93bkzEgJSQ967vkS7q2juODrdXjo13SvR3dI9cl4VglDXpNarVvnBKvPt4LWdoWThTJbPCrFZMcxWYrUCRFz4ujTwuB4mgp4iei0AqR7eczJQJaaywKC2xNyKRsURPUIWE1ZAKEpWc6npIRPSJDekz+S62dHhKN/LIFiZ2tSqxsHPnU90qVMLae4y5tbb8Xo91jsfWdTWgLadMyaSZvJd3aUb36jFrQdVKn/4gorhqJHwSvCriGrbAMmRBkypL416BLkuGMQY4bD0q8fhb06NMQHH26ipLUVyK3KpcGqqShmskkCDhAx0S74PziIRflwTMGcMBgRUOlAUixw/ApEUgM+lm6a/KEspACamiztquH8IDusToJC+aHJhnkMnye8ScxBeCcWK9NPQXugcveU+5yHy8aEMD6s8Ff8OS9q4AA+Tigbz6nERCgL6Vo/QtCQyQmM9Pfnx0sKeUzTUyBfvtMmHPeNa989A8xCqtO1vm6Xw8HgkajhdDql0URziScg3BBgiJBhaBG0CKQCVSCEyGDsI/4VJP6++/fyfoHrEHAQNyk0y647rVPlmRuEda/AEGBoLztxgSLOx4B6/t6QXedobLmgXMCG1gBi3ojTi79mTk5fNJ80nRSe5L+77anDekrUPm07Gnp06KnBVYtkkn9z5PKxzuR/Q5BgPu6Tt1R6t62kpcaXF+0T1nkiodfsr+8YiVWPPfCS6+UKvPaG2+temdNuYov3w+xSlV+++OvfwjA24MAAkUQai0SgDwTUH8GFsC5zwlwoQ8BnPlksGlrTaZudjoCCw2pRXGgWtGzPC90FBgtVoFAbVkEqCXYIGTsYJkoLMuRQXkUmGUTr40PIHlGaEZCFe6ctVo2WPm+sirofL5kkA0RYC6dobqruvGNWsH6x/ui9BOgLje869b4ZiSxqd4Nh40Gon5S6/Lh2M9CZ/RpKKAL+2f5a6VsaLQDMQhpNkjWSbZ6LwMNVisoCuy8z02zaZmsfQAPC2yHyb41z+tk4Ek5sAXmU9Tjft2fAV+ar7KtTVpOK9yI+QUjt9TIRe3/cV5rlufTy4YgJ6X0DXNuWaLS5kDzSt7uyaQ4RcEUsxvuWACYa/rFsR6bjqxMbAzt5yC9INiPeXu3OR35uzhtTyhVccmQVVRIrtwjT6z+Nt8l6tGQUF0PET3hAHXQSlkhzmUT4rZpgALmc5C5UEGQzVG2kzQQzuZUGYtSOVcpMTQNz6tZxsNuiA3rJJ+og/rJYZ/Tls2wywS9KdPYTOv+MhZ7PSPtpmkO6yVu3YM9XZpB8JPC+kTurzAPJ4GprAN7R0Yv/LGNMnuo7TIM8Zq3wdwdGV4LVgeit27GfT3oWVaOJJZrqbDfqcjNpyu61EY/GEQwzqtgiAXvWSr8wL0W9l1/Db2kHbKXEHRla4znxI0dk6XStGQLTK/e8EWd8r29V7KFf9f4cLrimGeIBZAj3bZlFSvvkTDsQru3DU4NqHdh1eZ8p2fSeoCBKtJm535xMQ//BaOCnoDMfBGOJbfxRkZefnZAlk08uqBR28ozDx1cYTePU2l0ZGDv/7HGN/4b6u6n8ePY6RknRXa4g90anSs66yYQu5OtuQ8pltolE6HWA3ds5PpwikK4shQph06SYBl6B+DNUSVnzMlFFrtDfXhWyUbme6LZ4Xh4Ev5jY/dH8addwwOrN2r3JKtEXlUrIq7ZZ7Widx01+bJZE1zNQFyd5Ewczw+cjVzYLwG5I6Ys90cA2aHyT6f1N2g7kduODx3uQky5Uk2u7Mph3rEFPHh/PwlRos/SazRA1XxPqZikqttGOtmPtPPZwGCiLidy/axMr52QGeCGMaWnmhpZpnV65zQUZxaXUDqCsIvAkGlNsl8S9+Taf02s3Hqp2ui1z9vriJZctpYdkKlHIboP/2sXdp9/yJpAxcnK4xNkU7Yk3sj17CSLi22oOsJjRo1LrGKZKr7F9gUJC56o7R25tSGnuw2BabUmPXSu+3AD+uUQYEoEyKUSl34iCibu0VTsUNLBLtOW8j+reAv8djORXO3OHODsOOVxpKJ3W8Ypqo2WGVGhnOnrnuOiwnLuy6pKEut6iGHFIFTDPOgm8FSSYTy/blfL7TKnmwKoAqgP7fnr8aaitCCt8JAu0SAUkVQFfYBhEQFUhzwUqGbMejZY2QhfwdXlVOJldn9Qk95Q4fYOJVtq60gRJ3ZTe6oGvygcjVuD5FETPwrWGzZ2pKfZZhisuQB1T91T3muxQdjwowo5nHA2JjvNLu7NZG7SnIkYdOoyxtRaRCJdniQZ50tw1jRfuwTP/ivLgsLgmiyamcLzBzkCqJbdirIO46TZ0CMU7jnDXdZTtWw4eqopL4UhFQjTtDKiPadCEQ1Tgx4O6gmhup4t9ekgtF7ZwanELlz4N8U8gyX6jjZV+D9KzyOYm01iYeK8ERcxOohKj4G5gAIcjnms+PXAEmEMdYYikSw/LMF9iZc42/sHr4Hz1aAxMlnPx+8rlrElFJaTRqOAB17VNmD5HCPmQGK2LnHEvDTDhlWwJD41VNsCJCioUC9HhRLFruo3VL0hPcVfQ3K2zg64bk37X10HCjRzniPMf6Q6m87KaAUI84mtN35HODwTdOEG0o234nru/6Vid7jn/tk/nqTaYmjU4eitQpQGhRARh2oGawg8/C8qgozJbWM37GIjw3eddaihqv2kbzjj24HeC07HTnCoJW6jzz0wEmr1dWdBGbNMIdKuyBxdURsnv5WgnT9AeV6xq8b5VzX+AhkZBVv8BP4nGGK1QVxL81As2ioyCr73k0NcXyWhfKy5t34HjLblOCcEEXka14XeZH9Ww6tKxjdGGVWSKBFMnL1RGPykwSTMurgzkL2C82l9BbLvp2plONkKNidmY1NvbVQF+FGAWEXDISC6kjiDqduazuRmu+dmdsS8IA4gR/KmU6Op1SrZkqUCWXKWXgq8iFUANuZ0LynMYtqr13Lk7iWUDhRR6ZJC53Vbe3NWx45c+GhgeOSz/KEWgZ82wRzWjb43rol8A954cZa1vvYM2UrQpNkg3zHJYHrAOldzM/pUUaRy1VVDTm3gTlULHZczVsZx3RqX2Ji9AW8JcnD+ZpVKSpKq+ZkOruvzXzKQ1YQTwVPwg+zXqxr/ih9+7r8tDnwyZP3H+XWP60hpS6XcS+1cSQUAp2s4nZTKZBtiPtvljmqq/+AAXcWMCtu7xvsap33aGtlTN2GDhoPAsmFZvxozs4stXAMIxfKqtLmo/GlfgQTFPRo45In+UNRoixxyCRV3sFvpwsvyQpa2z7N8mpOmZx8nsjSuUyCy3S2QdZYt1QSdbcL70UmcJvnScm59TIuP/xH4nagrAkMgRhuslG7H1wDHT1F1iSnX5jrLtvPDCeNEI77zkgACQmknYkPgbcOhAqm/c75vxRws+DfsJoai0lvZc1evaEIWVHMW2zSV6EdoTLuRP/K9PDs0eu0PovwePCE4EzPp5mmEpS5t2ci4XpbCkHZv1Jf0sHu0QtOcX3dFhV3nfeWytS8kh9uYy9yu/pLoLAB2egEdHQRYAcli56yPItnPB9BIcCUSjIbW2U8o9XUToeEGBpft6EBTDHqzUi4H8CNZ2l2DmotOVVp6jkvQtXOetvOIafR8H2Kk9GZSgN43M1hnrCO9DyZ/lfqe7vvb1xvgPC+eJGfAKHwHMyDsnk+Pd0qcTV3E7jt3Dkje7r83rXDh61liTkQWi+kfxHT3C/wQutOXxzerDNxz8+YxqQt956/IPv3+HzE7pYDPHff/Zn3tLJ8YrWuYGK6jV0wM1dVPDtfOYa2c6Cn2DBtXK3sG1cyRbq9aYlgxNVxbPznYQC/fO9zQODFSNYc2o3mYbqtwt3KkpzjSbZGvtW76GWm1buBSEZ2ytz3OjsPoZO25vTwzdH2+aXKqNNn7lzo1vfa7cvHUvd90V3Y/78Zd9MpbTFyQhYqbJ9ct1i8pAZHOdPNLdTce0adFMRhUG1cvkKQkKrcc0V6GPK+XuT3vbaqtzbqb4ofOGqgaDpxrSTbrrLBaNclrQT+AMPOVooEvttWWZp11/KFTKCJFb/BCU6pZZwX1E6W0G/UQZFlnLLjUQ6b50kEL0pEG26gzttwbQr0ph9UPbuqNB71IQx6W1yndycfyK6ThWEK7p7qdQy6S8/QrXICkxGfQ/4v8lgzb65yD2C60ILazU6AvnRboC5fy7xDLt94Bx7QAg/9t1z9sWKA0RI1+Wz7cMEdpEDYdjhjmxYTtQo1GHAjbF88PHkH2AJEYwOW/C7ijCoxX529/C+MF9dDCw5MCI1LKk9TKCh68zm959vPOuYTkJxWtrkFpoNDC7UriJQZy48KDWMkBDGLatkMPo8teYx+eicodi9DfppczlXNG8e3b+zIvTxGZ22IDYjMSAu5/2gmWgxojb0csiZZs0cOaX+Y0tMi1DigC1F1mPSetO6qbx5hmXH+HvKnLevxoW0fpM37xPDpPRTAgIYobwastys8tSYn9dgzMKHMflM+iPpyTn0rP3D/7ROJx/baXT3fUv1K0FYl7f4Dvef4hvL3rkkrfy21KHYwNTmvOzE5vAidZcXWKuJG60/nlVQzYl27l84aCSDzjvAO5ZwSenhd6Np+bdzDOKIgUN8Q//uPhreWf9/Fk59DE1OCgxJSw4PjUsBB+4uYHbP8Ypo9/JNfPnzfM5QEpzSUlqWP+teCzhvwPT0prG5805InBRHwCr89NzAXW1Rz8wKxLFK05uyV5IMQvuTcnO6ETuDyLiwqUG4lrdEwFsItYLVgr6GkAJxOcFuVMUxPfDQ9CkjwEzNY6jhzqt4wy373LlRuAeKkhs5/vLC2/vXKC7dYeEujt7kYXDrdSNnYM4NQHcGI7bMZfQZDIVBD+d9E/y8lnqDpF1gw0932TviQ5XAwXqjIaouxXje2FPE1ZVkF5t7ET+CBqFmV+69mJOOE3EuGWxZaswxr2Kpo7L1wl8QTTl2d6RVRFpJ8frvzwY4N3+2CDbVG6cIljpbuRH1vZe8vdhC3pBWfBskhfPFLPlvhyWk5NdtqWqJIwQ4BiwE7/FZJcXIhPHAzXTFYq3J23JHLnByKUW4Xws6L8L15FSd18vILeH5nGyV8Cr6aX+FVzMVCwy2H4L7XYSu2Z+U0Wk/+iL1xbXlDd3nobeZa0Vn+vq6ayQKgU5Y4+fdpg326by46XRy0NJ2ZBKOPUlabpYy+RWx6Pj7zYDzYr2Xblw+vXATt99ctgoF1hEsuOpS9GHfpSmfMgehOOeodp8w+dUh7uskBuRt5vtpEZP45W/674TkM6vzI9Z2ffS1gJfjnxdnw3jbYh+cgamCTQT2GkQE3U92R4xmZ+2wv6t6ab8Klm2/KT9LrXyOodg3j/6HBOdGYiMGjdY/2mV6G15FDa41RMOdFxSzRMfNq+vhsghjAyLR9FkHciVNsL2Vq/eVKh1fqqQqnV5on60/zAJIC+RsnsLNT102g5fVoPmCtH7nQ4Kk4dOaw00e0AN+h3ulwUJxOWXngjbhvCy6H3nR1Dq7BCezL0ratjYA1WYAIQmO9vzId/Zpc9rLut8R2N+yyanZ8cF7+9JAmRBuaZI1dK9DMC1k/NjlN+0i0QKrtkPsouf8I2o+RfLrUVni+mUpniDgS04OfUvLTo2IzM3HUw8ylgZweYRQWQfpQ9zyo9+jO2e9eXlPL/SvSjtielJeUGudPqQ6u3/HPnn+85tfn253HpbY236rauE+KzOeA+fGr2lP6po18GwQM7PfdRVxb9rxqod4v68w2Vxbl/0EKXoDtOZpjmqXp42kK1bdoDvJBhUSdRPRnKtIH4zatLbZC6nHqivsISGOG/oHPwOP6b/9iNA4+C5f+QMDj2ck38QbuEYNBA3/y8H2+Ej+5KBsx9erzOnt6KEbv2xWkfqJXN1QOOXP9kGgTWpwG8aW0t3FYdgEXvm84nDV0enVMZMzhx8ZXu7SVM8RIsYKUB/xkCdd/F99IUS1gxrYrLGO+7fqR2398jn6DMxs3dn8VgOlsTQ9L7bgaUXly9OHMLdm7xlJS6aBdv3nc84Z5YG5i6OYPjijTmkdqF6bhy0TQM4ICoHZkxdP6pmiABMxzbHltNTtlrklpTq1clYw+kZe9IP4QuAYmZM1ei8t+IaGGkfwlteXP56ytkTgJhRcYxo2OaR1TGopvAXuPPit653phMHTXAAVYsLb58z9wrfcL3Tt5AdLlpyl5KStkNCJP+oKv+RXloO7yqbn38Vx7J2i/Gq92RMBacU9drF/goRrgkh8NPF49vlkdCI+NgNmZgeSBo5vrFk1YcadQTc5OhnX53lu/EgJPG/2uGlw5E5IpE4+TqC2+8TezJfhZi4bpjKj6nK33HtlRqZMrQ2U+k3wTBlszR2FLziAsWEUVxWbtBoWUhrWoyDNN5mDgR7MUN3mEejhABB0ToV6OqGcvbc1hurn4sewcuq5nLAkUZaubBU+cPujniX5prl9Rkyxn2tHP+9/vxFRvr2tG/LVhmNBmnwPIz41nu0K8GOFAD+RdUwZy02oUbHsOpwzen9165elDtQBDNN7DYKA9eEfeHMFj+eabRf4hUkG+lCuanN57dN3YJuXiCeCjIixm8zSp2g0gFe62BvI03VV0PZEQkXiKM/2X1l+X0UASBrvTBBF5m//6OzJF4fvowOC5J/1rRnx+OSv1LBOx9USFe3PbdFx9pYwjYDv5QTKVx7AkTfuU1sNf6t6J9fjKmVCrlL2Dvq/2JTBmcf6lH+L87YSCyzJQ3R4kujUocAP0vemNbh42TFohUSYFCTmsXaRcc1aXEWaXLF3n/aPxwev7q9/luoo2XN5vhZU8mBVDv6DIUX7Cutf6ZuVS5FJY9HiGFduf5+LN8bUC5zUn42PIxZaub9iSXB76bAkhYY1rvcq8wfD/hF52anLmAnD5HPLG7q8U0+q8aFQ/osIt3DeSWqTL0AgzAfr6qblpe0on6+cq40OuJyylfO3Vu6gYIfz/sQCFL2mmbnmbNsczk66+0saQpoDov9JsqmazkhN18Ymsn9WCa6W3EI3LlD1mbwfE3km+Lp3/3fViN3AuM2NiohAs3D1ybRJ9fPnbw+UFjs343JY9qZNvSyoGAA4HPlzFv28kQKDYFuNkpIJ7roKDfLa/M+M34vb6BftNhbtZ/wOjgChy5gz4/ee3AjXsCwaER4M7adPzY6xbXAvORXhcqLdLzNnL80LN2zx2Wg12Olq48d9hdsI6S/kSWPqzpGMiuDWSXv+EWfXbe8w+PNJ5e6lJevfj2+Oabqity928QT6o+vfhsUPnoI2QQj7AjY3xioluhjzge0whkW2x1njW9bl19PYgmOYDpHlzrwq0sfn97bXn96WlVNsffx4ETHia4QlkDR3x8b8Hi5Z7thSW1DeWVkd3nriT0NzRXDeoau9Lmz3RlNLQcDVra+b2L8+Hd1bU0ME/Ly4pfnM1ITsqezpGVHX/xVHpyCh6/bKAWmf+EbGF/6f4UIZ0wSZDTlzOeNE43nvrqcMkSdogpYZQuYr5jgDH/bGaAWx59fErq/GdE5dZlAvjvCNmRku5qa1/okxB1NDGgjX9oIKgi5thehRsff0g8u0T028aPT8r2cGIkMVLDzyQGd95Ic90S6cx0zdN+5OBjEGwT4JmpDfIUNsWDbm3jxohzc813d71BNvWmUy09GRQT+fECzku+nZMDdieXD6VOzi73Du0bbtsVFsjkBkWVQ0peZnR5kL+/d8RI6WibsuSeoT78ULeqb5cqftROaneHqg9+bPG4v+v5wnfv8weFxSunAujn5ubpF074Q+Dprjh3n/ys4m28pLGciRjyjgx7ujFpn1jcZGFlRXdDmVX4Ju/QIh2Wxngl3w3YlkZcsJLMuojJ2+sI7Lvmq/bBX345/295P8Cng7mpY6KyICXF+iopaRjn2I7vnu93t3fw6CmrdA2xNXFxoQZBHVBGBA6MHQCBF9NpAwNJib39r3p3JSYM7ZIQpDi5UEyc3Pg0tzg6DXRZk/R1esKfBK91L78/UucwYe/C4FBXQrUKpJBY8r6hxhOGVzP29FS09hdmu9DS4zktaaEgKZPKzOaqVxnpTm7+L2Z8W0nTnqz47HKjNp0G3kxBa/3e0rSrao4kEysn9aBcCAKpUbCUobwDxQVqcoF3VmSbWdwGkSqGdeRyK2No+keJgL0P1YLerwT/3L4l9PeVLmCnoeaocyC7PohdHezrQg/gABe3u02xOWlmxyhuNMVH10HFFaNSDxJdVzRO6vRm5kiDQFR4/+wZ07n5UXrfiTM9vafODk1iIoISvT0D4iHMOzwo0cszKCEGdhaXWQ/1tpjTlBn6PV0eAf6INxGZW7+9dOvd4sm+zs6wIGWtcISrbvR3eXtmNEKRmPvsqDvLSHP65xpHPOL72ppEFd8D8TeTtwC7DGNzY/PdY4amJFMJpJlJFEMKsJgfrc52uNjZt/Fk/frCmTiL90FXdWaq2oeRd6Js5cA5FrXQch8pZfYr3Sr+A9Hv63IwHZpxR147GwR3DK7BCihGT4u8GoAPSTuulC/KfPmI+axKBOw04cuhJ5lFmQsF2ckIjBM9Hv54vzL36vzeBP6Eh6aVp59He1nr/pcLd3x6VLMpL8LJuV4suq2BE8fHvi27PSzxkJcLl+nUh1kGj6cyP6qbbZ2ueGw/fmv/QM+PvZ9h3MLdm+3BDHExIUi1YU5ev5meuD35ArK8jnvTs0s4vTo306NYlaPvfpJudtvWJGXZKF0/4tRwCCSxrM29HYyMhwMS0kQTCpRDONFAkd30FwsVrvtKJd+3tzS+LCh8Wvn6m/DVyng3UkdGymyuR2pHWDqtRSvgyXvD/SV7EtxT9953ZLakprUWpWTXR8TmHuoFJ2NLzCvMkKpTAItOC2I6O/ozXd38WKty4a3yYYn2VXauH4hZutDq8r+s/mqo9yZay0DMzatNBItiHQ+0tLUeP314tiNNw+efOx1eZj84fn7zvBKRKX/UQKBfTT0yZfD8E00BAu56k6WU6DlKXPlfUmNEKhEy7SBrVDp3Urq5Uxg1bj3Rjpdpm84blc74MhBo+862fIgRtjkkVnQtWYzbPnQU9lGmR08AU2sg99fr6q7XNa1l7beR29+Qtd56iZTi/Fh2GcFH3/M43eKeXfbbmtaq1iUZXTRBoDtlMIGf2n9gZ/oQn5c6RJ0e9ghPleAnK4YHhYNSBjUE5s5fP+Rhhxd7ccI0d5oNZhlvRF/tbNzZuILXlh4HgcytnKKs4KDiDC57R3po+PbMNKZ+b1SvvsFkxCSYRn9NLekM1eqPNXuopI4YjAklF3eHqnhmWzzYpE4wAPmvHzWfKFagEgNWspRV0H/xRbyMjrZTJjjX48pPzsYGWvq4OgrZwU/eyt+DRvf3sLLkejX7eYHkK7UKT3YFqmbsZXtI3DKIqq/XDctRDbtfdQdE3oBLpNcFemxHtRz+ACPAjfgPrjvVn6PKUL13pzwPYcH3/9F9XXYLqunO7bGIhEbdg/I/cSXb4xIK85AovdjifD6vNC8ePEQ+1h+XL5N+3+844A92C+GJXwd+bIA9CT/YXA+zFYwXprm5pZvnq6K+nqdcKmCsKLCxu3N3fn5Q9+I3rORwvGIV9c/BqoFKigSPTq98L7At/ZwUSk8KCy9U6c1I3p7cplK4tr9oqn6qMqEDbNV8Q9dC4fDH0jvHGLn8/eGzitPULR/ClePxNosH9W8t34qpUKOqgYkzgS3kujDG+LDaqjb01vsiJ14vLncwVQH7Te2dbTHqwk75mWSC9599o2K8r8Plq//5Aj4wu7p4s9H/12QsgS3k9gK8B6YF+3Km5Zdr2wfh7DW7T+vUe2YMrcBy18LR9rbGvmqk4LJUi57FDpPQIMsAO4NA90sBi3sWXQ9iMBjQ7WVH5PArQzv+n/H3dPspXf2jLW19I0jzRPfQ7qbmob07Lwu28yPR5ed8Te2Mo1FVgL/1JvL7r1hn7hi377++AJHyqA9Xk64OLt8CTj6l48egt0z5qnNpVcm2vN2dNUUHS5Goq2jn6LhU8j5rY91LoD6kOd5cyBb3d7KLzyqtz83Yt6ty595BVKv19S+rb7qPeZG08jK22lsbRcL+AdeBu25J46M7oM82Lcms0LIwYTCnqXE0J6GomJpikRLXky3cm3nshA8/1kenlmRYKxXP8GMzPPzY8NDAgeydV1NckrN/tKRxeBQ6Le8+vPtWcogfvJlix9pli/XI5OlTnc3iQfJliXtInLNFhcMWZW/NbKJrcLyzXru9jlKKRsndGwfP9IDtLXHiiLgaceH8yOo56C8+dORQ9o8SZ+FY9CBeb2o6cGz1ftdztpY+ALgyqSXYo3+DTFvxoYKDv7AmM/KfykgiiJnRKUdTP/VVHFyPq9OjsagS7OVMTebqtG9K1/df9fqwAwPv5LQ7M9xBdJhBdSObTq3Nd2d7/wvuv7MTRr8eYp5cubKeMwYqPQklZnrPPbQ3vH6pHXyX81SM0pjm5Rlgrg+BD4gZcUQcFcfEcfvEVIUDxVMgGWVUb9VYumR/IFmSA3VlmhMnO0UCMT1tPTMLW29wauMm52eyPN9djduY0LSRtpubhfWPtkx2cnsW2HpmarbeoHQoXx9t3brTKt96JurWG5bJUP7yYmO/9IbJMWmzINOSINNlQaZlQaZXbBGF/28BeBIBeAwBzBmw9QDVkPNkJ8UAIzNjNnnTcF23I5tu1lD+PcBPe5QloWuikT0Y1lZ6BSB4nkACCqfhaoGpfUeuyXlcxloXww2+6rP/zGqKAMZaLDI92vdjVizqFizyTrDsuyVY9v0TLPuJ7G9DHcKf3BP+NXeSMvzHcrCaFIrBzb4mazd5OjZME6UWoK1u07KemaiRen0kUjmTwarYG1I1lWyMoAzMJOkFhKmJAXPG5R4gzKhT+BGRLepG00X/jOx7s43elA2LEFKkIjezZuLA5aJMhHDJST5IoaiSeus3W+4DWuFAmkAHmpGZ1aGa+YOiNRy3g7xTTk/wJCOCcKZ3hI3sqDbh49RO0pzcPiXhijy5vcnwmo3bpmxMJrR15eGhBuQyYWaI5UZAAZmHS2VGqy0aH9ekTuOnbF+fti7fNmqqINtGbp7c9lEExR6ijCGcII+eKiDB2rN3rl9QQUhdsGhAWMhvQPiTobAw/8aFP/UIa3mVWiJ49ExbrcKaHopgwcsn4LcA1sU9kXKA5EnBbFJ1KV4QpJzZrEvmgi5ZrxDmwo4SbkQl4+WdYrQzhjrpYq0Acpowu4hydf0QOiZWI+hmuBX14Uw94W56Gxu6MFFz2Ytkd8zZEPI5G+Elq+kIEk0P9l6sMDK3wT6NHrUbewJKMKmFyO7J/hm2MDFxO5N9yYyFFqZ0gjkuKJDNNPIpYD8ycePCXF4mwFxiweoELmiZqG6TqdhxwNL5r1DD5a+EEYgUkrB05n0RSDS2GaBFDgFF1dTkeCAwbN6GJgHOuOgvC0FgPWRdNT8beNX6loUOYnG05KA+sB62j/6xC1v+2D+6RYP57LmHL5g/m9NCwHZO7InT4f10Mec/neXbw8NOYvnOJb0jU1phYHFq6GO9VjaAN61vMzNbUKq7Ts9ptKqrA/MoSnXWcoKz3g9corPzFHKPA3l95Fi1NembD11r7TrnmpvNhTVxuOVIU5WDfF4/aWJA/XsekJSNGqgXBMB85G7KBVkIv3MacA6j3pgLikHff7fgU4v9+zbIj2qrraM+TnpN4cuYCL84e0dAMZiX38k4a20a7KYoYB1hyEj39erI4aJA/w9Oq4HDAdbE/uVlxPlzOIzG9/ZEt9VQ2QDe7gLWOMgX57pyUO8ivXrwQXTHv3+ewwN9HuxfDqyG4wbI60bPZXGQqI4cLvq4RyM+crXmeP/GbmPTZHzf4N8BUJ/eTVd/lN83P+QfXqNOKxj3xr0y56KpvxIOdDi7kgxG03dyPYhrvtbGUpCnIZOKoxk7fAyu+O20Bfa77Q6I3eo2BILXwKTshXVcWjvO39TB9i93lcH80bKQX1MhXnSbcS/SuLcdD8pr/5uP9eT6zWyvVjzHJe3H+2bQnOZ0dDcO41lHPr7GsIzU0ebNPE8dNTmCxqeIy+SEaWn+raW+5kyt8/JefMtUedTl8pyWeLRl/3yNc9R4x1wgdZTt3p7g4+gtkzHr7Os7HafjJDDU/BLHc+Gijq/2Rxd9XKaRIL44zmVygjwa+5fL3HebC+zWWpNVm+E+Zk7jaQeQ40bOOenabRZx1ufWJ9/CEN70iRKw4XxwWm2D9auccPKA5KO4bRoebOBw1LRkaarHstpabHAlJMuH7+bhJfcRHqQFrJdbcRpdW4FOcS9yLuumWivPtpCjWV5H3z92h/l+YW09f9jhkxXRWTeJdz5qenAuMD+KxxKuztHci6Fwv2fVTae8zXINGt9wtyUGxqn5lYClp0koFl49qqnU09sTSzk95y1Gunu16wySV3z/Ob3/EdD6em4QdquhzepvuNgOUH1/kgYA9Rvz83/Sn+xRsadDCEGBagHbuRIc71Hq7+CKITazLeeBaWpFicXjPKauJb+a2PWC3dEdguYbodMOLW5s65r8WqIz+il1CIt8pT4UJRVLQf1ZX3Y6FIzBBXYwAw9RbK27oznlYHttYtFSTLtVZavJGRu27lu4zQgCU0nxtMKZZMVdiI6o100XMsdiiq2tc+hMmd5id+woT89RXb4zW3irr808/Y5zf6GzlpfMtCSw/aM+Zcn1Z6i+h1EWckYYqJsRGzvoj2ZIjTBpV05dJLn1OXQxh2ah12Obu4lzbxNXqhJLZg20i+RSVbC9l9x5eF3OYdwq66x3Pu1m5nWI8GjTFgGx+YxyS5FtDKB1TNcaRiocTDw4apOEXw4Ku0tkz4uc1XzalXBhoHAKQNA4KWRzwRl1qsY/Hs1TIDdtFIwPJQXtzr82mOUrRqox0f1ezXjHrXhqS0NE/7dGxRiXNf5hz1nRmzzfLVvI2OC8l0G1HftZIrgfK5uiULHNc+guvt3Itks5dLXUMlK+zwnbzbDKfk2MH/Jqj+xxTyY4/Vpw8mBw+nHg5DLgZMfIgHwKBIB5bujclCTmibjcRs1vDsNWynBGHxYc6qmK+U5ZFUgoPu/RRKU2ULqD/xSDjE7T0o/J9Gub90TEAs64kaD6xcxQARpIoMAZLkzG3I7g9IdqAw1Q4AwXtEvuuY41tUNjIvYj24b5WBaTv6DujdNnrbUlz1FbVllJgF7HG3lyTeUm6roo6jnJQFVRx6XJ0a/mAqTLaI0nlCdXT667loui/jZxOzCARgTYTyr2OlBGq39/oeIAgAVWaBu00DcGA05oGi2hHQy6ATl0LzhuYQ+WwBh0AL4ghA9xT3AEWZTwJ4vqs1VSRBDJDW8CYvaiWPyIGrhXTs9JJ8SBNyC/IAPwhklwARZhBw7BAsGN7HKJ8YQNP564Fc8zFgGnYIU4quCF4DJDI+v/qIwkQkCeqYEcT9hjXW2A/LYzxEBDWgjAox2X9iNETVqoaXR0P5qcxvUY7MfSwldpHMJXi18ReKqM5KagMqTsyEC4lKwjR2NGM5AsTWyUgCey2XSSdNHS49wxOHLmiSXxWxkzQMKULCKQ0beVA94DMgoHaZpXl5MQRqY3QmbGUgRpsJlFkpDnS6m0I/OnyJWGJzZO3sw7JQFNJ/cyQfSmGZJEMtD26ARtZw6bdFHFUj51lkwXxQDaZscWXxQAAA==)format("woff2");unicode-range:U+0000-00FF,U+0131,U+0152-0153,U+02BB-02BC,U+02C6,U+02DA,U+02DC,U+0304,U+0308,U+0329,U+2000-206F,U+2074,U+20AC,U+2122,U+2191,U+2193,U+2212,U+2215,U+FEFF,U+FFFD}@font-face{font-family:"unicon";src:url(data:font/woff2;base64,d09GMgABAAAAAF40AAsAAAAA07gAAF3lAAEAAAAAAAAAAAAAAAAAAAAAAAAAAAAABmAAVBEICoOTfILHJwE2AiQDiBwLhBAABCAFgnoHIBuSqkUhsHEA8oA1NDKQ2yGoCujTUVSuitn/hwRuyODVULsiRUSphUbM8X8w5+JhK36jlI39he4JIeySB/1yqXlhHd9zj8V5Z47Dd7di7TTLiI0FkVjAMJqp2KqVvYfR3zGO0NJHvEOkWw1JCElIICGhSkkjkE0CpFB6OgkECCG7tNAlKCAoCNgTG8KJdjyw1SM5S8MSPVCsb1BPAcudZ6nnnehjuXu9vyzlWgl4sk/w6ax4dcWlArz0h5+o6Wbc2y5BoMxMtJWjdpSwYH3+fGUvViEsmrQEtNhWPUgqIakgNi3DLOtn6u/z+/b7TUTsizVEd5FIfYdG0zuhYYkGcQfRhGcq8zLiVePflyGZhbL31rS+SnIn/1VJ3fESnT4Qn3Y5cQSGgBM79kAGM6xL91V9NYQmQ/h5Afn/lFN7a5flNqddLjPeFj5I19EVKFAwSHKATBwyNZb95s2QmwbpBpXJZ7HtR9G59ZOk5Fuq9N1hbdYDksYMiDj6YuL/v6pWC9DixFzOxlhUE1I91YaqAS4+wn8fIMEPUiOCkk2TiqTsI4OWTdP2mpToI4hy1KSUaGlCTNWWqY9VyvVU3bjes9X+n6plC1AXoPQOujvb2qu4ne6ceC6qHEu3Lvo/QxIcgKR2BptAKg15CdwI8NKQ8r63lCnHEEfcBNJpKCfyIuQQUpVjUV7pXMeidFuFVBv+qytB9i1lVPYACcIsJZTb7Dh/d0/O8oAHLOCUZS1rEwiKxyjEShWV2373R7b9/z0bqLM/k4uFcGQjxD1ExCSNX3XIXPXcwp1u62618YKEnjYhnURfAUCj/4fNEwCoPjoBgDXWbXH7A2TdLJiemhsZ9VLAW6KHXIWZSwHvY4AldA8NA8EA1qECeLBBaYwAjsDOtCGCwFu6/h9CsOD3sxqH6/lxSJC2qeKmJ4VfksdwL5TXI6D/BBQbgMEI9HpslTR+UqkKpugCSMEg1COKlajcnj4cAKIBRIdiKYDHqbGCCxHuCR0xDpEOUKwYWULEEg4oNyODFK2ErQKTY9EgjTzhoJCBgy0sFYCmEASq2XJQVTwPj0oiKalJGzuYrZgdnwpQmOJXVL2WggxjAVhiWDp6isKbmMSXnvBMOX0Atuk5O1LmJoLU8SZpCdoL76UWM+ON0osCwKD+z4TRRUE+/VQEf+PmaMwm1DTg0gtfuL3PRLdCkEu9F48IYFdAsNpwqwKHA3OqW+ymkQq+uBiMN2I+xLrWm3CaVbYf7lviMDATUdkCICaNdt4SNwZRZB2nHJmnRlh09l5ZZ12JBoC8JkCl5IUdqOoVRWAN/3jgmrU9imAGRM9tVYN3DRnnNMzUSB6h8Nh6Q/UebcoZGcplY7hTcWrhJRo0UEsROvjM2xs+hwF0rNtsXuShQiAjJRWUKGzbcbs5GpJhzNnxmWlBlSq9H4jN4UFtt1vmnLw2ufDAD65+67cJR+HBIM/dEAB4Si1SzCK8WLxfziWdirw4PbYFUFMqCtHraV2SqOMlqpyKKzgJekvXA5mFl0y4KN2+ZwBMGq6KMMfOuwszqfoaguEIdBlS4xBroHGXJyc59f60Qf+QiJgeWZ81dp77W4AIw/ODEJyFvFp1inwyw1UeCU/FUHSzyWPDTx+GhXCN/A+66GvTzg+IzF3u2k6vMeUQGTTJmTlwk9B5cvrAIURbXHFzJkCPC6sIHAsGSSmgxNB3ObG1e6YxyGwJyJOH5CDS1WmduCKCGsFwlru84R1hhAkA7fRL1asaslqc0ngwx5Od1/UYDlhGSDJdYU2mc7ywAeXxlmZ5Q0iTp5qGlskNqSmaNmDzxf5PCNmli+R2F3FqnbAvYd0tO2CE44t5AEfEU9T9T5RT7pmjic5WzpwGdFGXuowpUYbLDLhLnbGQaIvq/GLl7wax2GCHq1eVWoLSIKQRFlAEDXwEQSxGIr7kvcKY6+zBRU5Bx+gSvLNyarbF7GLyu4XDdIou+DXTRp1Tu/cuOIAfe/Y9CYcD+kmayLNaxam+3ZA2wXXdJpmB9SFeAsCkL79MadJfXmUsyRVXEN/TfxGscGNNsHAZd7ioGtxIJUUbkCsO4fk9zzCU4QpmsaWWWOqq8RXGJS0T2TSAnCZ1yqWkG7PjNJC6JMi4us2GXDu+FH4inqhipqm4scWmNX95vELTDV++rg6jqTs8GI01fLsoRMKLm9oQyXE/vsGwQIr9hsliSQEL5pKcYlymgRJf3KEI9W3KZbpKc5PMUv8wEaCXcW6jogQmV7FgRQvkqBr8culB3qTYgvW0b49BI8qMhF+XYuP0JNjhrVMNDh8usCBZmd/codE89y0/VJLO4XeKpfL9WbSWygaeBFzDYk0VKy4ft9mgl32QA0EVI973US6Z1d2x/ANT+J1nAERv1itdW2NVz19ud6hR8UFO82Or+nnEp2kWDl1REnQZ6SOMQI3IHW29lfeZZjO4MlKpYsCI4bA2f6PfDAs1gONtePy6MENR7c+peq/3saVWo6M5JHocnVOqelt9Yxt5Fmgv8LF9gvFlOegUkNAAC6aVmE2g5GK7glS9KDlvHM9X0yiTfuzg7PSAyg3N9l/ieByLFHLRwOWJg+rmRlWKUyfmaaDWhXFZScpSlmpCUILQhh03rSP1kf/v8LXuyqzLsJWZmX5v2qawWCzO03HlMMfwzskaixf9RSQPzEjkSGRtEHPz6gliZo/oGDpizs5LgX2C2aLHLN/Hs74BjL7CCWU8syCD8Me6gAfCy7HRp+XFUYaiRAzbUQZlBxqj90s/50IhSpYrHvl4wgabI/gaK9LnGkTc5M6wcHahHO0+Ynkp2ilPvBKffKqDaOSVSKbnp6kS/w1XgYx9FiQ9V2iZgZp9YKmAuyGpWkt5chUX9SnCrUjvljOwZHvpJHX4+nVM8kQVfCZPpgY7tl/pE1JcpONGKtPyyrmjGb3Xi8GnMrMmxNSm6r3aNiZF64bPgSIiyUwcMA6V2PxKpIJFKuZUWwWTj5vaTT3mI+xFnE5rZNBXIsQ+QRVtLj2cK+ypijRbGGdpVdLyQvshfy9GsCEHoFqK1aMswYMPY1cy5JSBekcjgizS0Bh1QjBh9VGALR+RyWCRLiGDTFTLUbPzYbuLtfPgK3IrcyalVijJKr11FgLYp5P+sVU3ttL/jueKd6CeraFVzCDdoaFx0xH/TaikMusoBPUqObbbzLV4BiLPqsal6ApViXR1GtpET8gbRyeNhjY2FHktswiK/89nViGqmncOsAe6Eea/3zvuafzabfL/RLzAvXWZ4nywsybMFUwgBJeumZpmCCUXCnw5awR8pIqAK4TBffBb44ztH1gzxd7lyXhsioccvb5Bx5XqyRrLtvjGXpPqRs5bb8PCznCwcRkVwYGM6qNXV7yyc592em2rxiH/AGT234lIDADtTOt6sMqoceMw9+INYZ98QYn8qkEPjGKMkOiav/xg/s+QJBRowVIf6PVr3YHgO7Od/liM3emV7kzojYjtTj8sBSpa43bbCbQnSMdwbE6hRh7pa1AIGZiRzrdijhRJNCyXycJspW/k+R3enSYMGrnS6b0zjEkWQWy4kdo3FTsdat16A+Gw53F6dBJ6XZyVHlTyJimzUGHwOkltLftcbvfkdhFYseWLwSskMFdpfTGbISYbvVHBipIo15HK1s0hIQryFv3uAmGPLkOi0/WeBNAFDu6WiL9zUFl0gn7tp3I2kYaDsV2TabdW/bl9gxc5ChaVG8+X/D+SBXWL8UUPo1gJ86LJMPgYqDseysYlszUt9aS6ys6j0/r2y4grERvCS/Rq13zfTdt+ba1mJIklWTzxYPHr4l7B0s46M7Oyjvu5ql9cklZaWijZQQUKqthlQZ5TGMzJQymFWlBYlNCLuHmVaGBxjMjKQVhgRYOSDIRhWn0qr4yd7vUXV7o177QVsQ17renfEFdDnaxfmYquyCKiL44iUZXoDEfQm/u/9xXx2jNy5aid7g5k4wehUMzkxloKNmBYfJ9/YLgczC1dsusN4/u22/+j+8tYwkAwkrFB/xGKFpn2cnZQLvHzhi2Rhm4l65PfoxtltFAd6UUoGdNv2gvZacpYOmTInyAHM8Baxai/B9WOjsP4pZ/+D+Pw1s3twTJEBrfalfMpZvyZVjuU+Nnu/p7MTxP1CN5KBq70GadHSXklXUGgcysv12JB0GKa3BbdmnUUF06PZBn0RCEj0TbXqb0ZtF74zjrMc/qJtZm+NyK0frHo9ES18sRkOjud/p9PuiNJBixS70zWDETU4QCRUb3Z93tFoW6iDa3xe6IHKqgjt2MDl2pf+y27K+U1uhd3Q8LmNjqE9ze0oqIosg+cBGxo5Ki70Prg8puA/UO2T0fskFHH4g2wa5/pWc4Wsmp7BDP7uVurgA8Ai4/FjSNr2Ft2fq+R0uZujU37JaKL+0ku9UV7fSU+VCCosnaGygL++pBOsMySerqlF0f+tmTkRikSBUc/v2Y7TKoa9rYWRiHGgAHLloCRlr2lPSOX0bbgj6zXa3exMNdqUHi8EMWof+JKzw5txhr40Qn3InOkmBnAQDZ0eXQA6119LhdAuj56sbPAjeiEh4qDzKo2WMbjcEqDSuSSW93BT99dP/iY2qGRGuBiTAxu9kwLFRUfv95d27rEOxxriYu8dvXJAoeK4ytRMbjR2WYvXvRjl7oGMLCJUQ00CBjYztCx/w6LTljv7i0sCeOljnhnvj1yY9yR+ebAtlFn7F25blq/0mFj/beGjo/ZVplFnmHMSZG/rWYxapAYaIcqzxU3Y2rN9wbvZq2Nm2xJuTafXfHKHT/KmqhwHG0fW+WPguFTlFu9tr/GFWsSrYrJl0GQcjGb91SqfjTj5dBtziA+6KMUddRW9TDLYl6AS7X0oIMYIFgQp1Z3gJRNJu7Djh+kscbFegoxTEg78A2oVTNqVs27OXVHGS/zA6V+GKnVrtZOzPin2Gx9q/brzD3tGBvNdEMnXo7fo8mmCEyr6y3PPt+6Ku19PvHmg46rpqDVr9LLP01+b8gktehAdzmojC4BipFwqmZcloEkEmRi9eIkxVAoVgpjyXeGhpEASeCiJ/0QT4d57nUBrdbXbFG2ABv2wGjZ9asnBUOincAZAiFqKCpMFrqURmrxo6gaMuBG5HnjgBEdE7nhZlFoh1LSGE7VksnCfLCTgOHnR9nxxBU7nOAmK72acQ2p9nsV2xpU9hKCu7HTY0XLX27u0LKzHLw+emHr/nVjPkygV/BmwABzOsUrkEWbdemByFeVoWTzCwLHzymVRdh96vhJKyhda36a1p8el9PW+GDvcxbOctXc/EBVo3AdzCsTFqXMrFifXvf2GiTSRCOYVQIfscdN4Zdz3NdM7WVyBbwoXF0yM37mWhIwFr4xIEX/uAxmQGJ5txyJrq+ylorf0ZBz7mgCkIQusRGE66bgPigtp6yJ0Zrcvu/ZFiP5de3i6trBMjTgM1Ax+S05CtLc9gIMmZvYeZav1+m5FC57KoiEbPnr645zEiY5uNVLu21dR97Xx+7eMLwf2IPRti+bWn7UBea9J9fK1qjGkx6ZURguqFdR++Yo3eSuN9A7E9uonqg+RWHdpqf/s3MaSy2LP+SP4Pe7/sivN21usHLmgUKU8/82CBbTU2KN8cWsTDTzhofNpytGI45TG0ctt0wDEcOPaNFTL6YxZ74+4nTcWhqSdKSGUWoUNrhtozbhSQH1UQYzsm0Z40ub8w23VeG27Snanzm67nwZkImrAbxzbOUavWJJYcZ0GaY+0EXA3XcNdOSOIw1742DaIPB4pFgXXAYp4vNmQO/Gu5KsshABWAuiJjUH+A5cxhtXMzX6VJ+szWNZx+Yt53irCuS+M9Q48pY8fB7z9wnZWeDlGzdtfc0ky80NDiTGSugr7W+loHAmK86CAopgChpqew5M44FJDxaI4DZnCVLcJds84J2e5pjesvPmMVRdaqGC2+ppm9XuSFcBAx32UW5v5ABwBzuk9tdGP7ZCMoNeFfv3hPw/vDJoFnOxQolXZpWbJdsZ/pG0zASHKwPZgHuhbUHTdIas8db58PUVxEl1xz9UW16A9qEE6usfF9lRLJmXPlnbtE5oxFK9d4k6Qe64yPbu5sqXEOH0sw5yuWijdEV2kBBxTkGS7hRhcUmGc9iGHtDdtDTqaKCxf8VVwl+GZr2rnGKnp15yKlrOcCj4WE+l0WVP1kGxKNABDeQJtPUp1JOhQml91fn5v/5dNtXU93tpTQp1tQE+RXr5ekIFJXWNTiHKlRH9/hhDvYXL7iFgg647ECsz7S91vhXXMzz25Os1qqj1kCOYU7u6QNJSVfXn3ufCYhNO+oZipXwNDe4cwKEyta16hUIu7ijq35yc/gWJJnVMrW895p5XXlDoTc+Y2rQ1M97O1vzMbJvcsX3MPMjkK1fdcfiIIp3iGxSyJYc78wZSLC4XZDAgg5gEa1s2OrOd/fHpGP4Pl8QVsSma04aBN3582hLS7RiQr5llloGqM8gulQ7FPc8QBl9HptGtQi7guOnWfEgMZ1eoUfn54p6J+NLzr7Dmu9w5IP5HhZK9DAntVl4E6qoUdeTjbrVY1VsJpbJr19s6iWWZ9TqJ5cakA8ZHW4Rgepu4kiaMqmVwQG0u6zUCTf4gSULR7Zi/zbWxULVGy0Zt+nF+XaHpXRsJ2tZDnSBfHffKMrqG5eY9vlJlLvE79so7++206lcjSnYbyJtJds2SF9/D4F6ZIGQn13I5yyxlO7ssNuHsspSNZ0vRVMKo7mbyoVZ2vjdlE4gbSHztxiKTcxoOelBqcFozd8Xv5sx24i4GxZe+xL8Lglfy3LqCoscunv90XV042Th/LquhWXvx2uoKVeKSWb9eflC7cOnqIASMLerajRGBQF1B1P3vkYR2UCDLNAaAR2zF/iIdV5TYqHqyxrplzv+RxTqd7LfNfVb5zFaPaaWV+nKdljubedA+F7kgekZOQIbTkgj+q0AhxHJGQ5Z1NZeTNKeSPX7q/Xs+rIFlKnhlWqbH7nWg4Cmv3UrPQAes/lZ6vYaQD66hRcmAGhyTaL2wuyRwqmuE+5P54qpZGgFFC3zmDc128OtyiuK3MFyoS6N91NAdvgsWMIMTbJ5dkrckwihEcptkADSfaNrlctj26nIrVv2MLpu2nnHjcUca1bCy35IKNKPGZN1AA+qITFcqDrOhldhAkshoRtz2CUjNE8LyiBjfoDOjZ4BmaakzjhBgZTuqsYl79JHQ+NRlErYjYbuLzHTagzvYA1xf1iP7f/vspjY5Z+44jLY0dW4juOXOUvtmE9pZU21V3C1cSDwpXaZnDY4pAuyBEs/MHHhz/7fzAUMe6Mfv5xisGoP0PCGn3ZFCNIPsE89qVRYCnZ8xTh+pTnlTgT3nKecUuudh5R2FJRf+qiTadEf6K+rco7q3f+e7aA/M/5l3jTecTo3jA3clUtkvwX4plb26qqwaM0ByEaDAcI8FrdDuxtoa8yeTFIBZCWroOB6D0ISwx3iSFFv5NqmVXe/ZAq9naQzX/YIY1f3ooDxDNou7w+My/x0Qik87ZsNnZBmaEQa0JGBzKEnTCthdHBZ21slgboLAE1v46U1tSNirXkDa/zv7u5Zq7A7MTYr7iTU3RPQCfzaD0VC6Ez+cRyktXp4VAyW+Ghjr/VNChq+VVdHfc+R3bubroGDucNdUMaEln4UNL92kZYdZ+DTo1zs0mMs6hRdWoP8NLRdN5j+0LhvsumDoqc6eLTfr6yNE1pRn0pB/E5Xjtamr9MY76vIG34kaiilogyegNHh5ewlNbegSt2AGMs0LERRumeAGfJBFMGyjI0Uc4197Gx/2FbG95ySyJ67gI/MMD9W3VpZcAxEvqf/efdJrAJlMC3+vW4uT5/T1/p2LWIxsiin/hLrpGLhYKdzbqJ5xfmpzR4I7wzZVKf9v7tOloKgsRBlbaUtVNSPkAzVtvNAgSHRsGHpaRRQwkKM95p/1uiZ1xqEVLlPkzkwPn3Wa/gbvGJmxykK1vEXvvGPnc1XTgQtlXRiown3dBJ4XgthSUX6roVDOHpm4ePtPHGgDjRExsmge1GtfvVzM+/yDn6NZm7J+n87rONUjHMGby+o8Bvic9EeH9UTjbvlCFrtb6WmTpaY6h0U6Y+ryrUQ8dcdn+5DbqzQbDBFHPe6waMZHpuFUdQfdCUT9vaY4UNVI8mNQ1Q6qpWIU5E7TmnnvZCJ9JsEWEQUQ90d7nvEg9L5fe1KxUnB599O7sPn811gEQJfW4K1w5QEdLe8v1xovBaOpG5bTuOCONf9fbJJhT7iVtFqGBVvnXYt8SRQFdf5H586jahyJHZlAeppbg9fnwsg/Cb2xTW+WaRAQZ90h6X7ad44m9cPRw+M0H4IG/zNsOLk1tNKHKbitdW20xheL8/hTTu/9hzIfTwVdZfxcWWEM7yc26tuf0nHlMh4ss/746vfpuAiRUO08XEjIs2Jgw7nmJQAXr9xFeILP6xS/aGW62HggAP0k2BwkfA+RjjeHS9gGBdTKgECzCOSpAvZIFtolhC3QjurCHxhjVPedk3GeLoIwAxOA54dqxQtKbmyGEmLqRi7pkFFOt622t9gIjnnv/h7qcYRClAFBR8mVqxQg1DXV5TrNdxT4QCttOdVeDFxuOGnYMPdWybSmBhW89YEI5DoiIc4DEfP9K3lHtxgDMi+6wSy9ZMzdtNBeSb7ht+je4IntNHMtAVpImjd52SHW01Al5PJM1AUplZufW/tn9387t/47Y+Cr3bCYz/6fWf+DG8sfAJiR9e9L4oRznqGzRNt7UednG3vuhM0/vWvkztppMdV2vSMpMLxkrHFP0ptvqhX+SgfhU5YSJqrLBTaDkjS0OgyqIlgNJyddFKOwAwHo/u5Z8Ks1n+B3VlJfPQayLDLjw2ppC2RJ8oFYDSH3dH8oI5cvvzB+KXftBW8m2LO21V7MvuDXnHZ+/pKYypNhb7d9zc1aP9MNPfgm43RXWgXPtbY1Xk8vFViQRX3LoUa068id59+O2Jtb/PVNlqQNYB0AS7GnKXSXeJS5WErp5Uwz41sbQtNWRWRh9J92KQdSvmYGgsNZp0vdYoa8T+XrV7wjjLdnvW3ROVpfuY4y8BLtc0+jurjXK+GfW65r+0YgZ+mQ7/9nHaOGteq31ni2g6+9CAH2DHOijZKnxBJmtWjazZ903RfVqf6jk0lTa06sXNmwrYsyXRpujNDHlg+x0lmD7VwG3bXkbCvpwwBE1oR520dQCUbrXYskWwZG5VRFd1Qlk8o11BqvP0xThByLXFcUN3VMZvHit6cbRiqA6vAg9iA0VOgnziSW3FeHx9W0yRQqU0mYYgSvOv3lEbtaBmF2z6pgjCWFJrm178aMQ8jNSm0oZD3imgx9Em3wgv8Y0MJCD+ZJnAegtXVTJ2nMJM5lr/aRapmWySLFqZjARJ7UoCsqTIWwwpurZuAgarIAzqWY+SlZXW5lbjf7TmkVVew2v6fAiJWo5yJNkGASw6U4yUyES0G6XaFUMkzNDH+/mee3pHJ2qF/n/mzPrn6cSPb5zLgLV/H+YvMrWrdHc8m8fG3H36tfwSrjHRjl4xYTu49mj7GmijKxEF6Ldoni16gapauDz6mWueiy3GCJGAtX2niXy1Et1o8TFfTphvx3wOnkno+6/hECp9gdSsjqAonuhcPpQo5/EuXaDiviAXA0fRKApxog8gzp8MXecmYjOW1dGditbaYa76OOXMA4Ej60qOK6dRxjKMI6HOQy+uSWlvvyPDrANbSmhSQtLdlDm2sUlQc7Y/tRwA986h3g12MjQIxjN32TQ10FDqLx5xLrFhU/2HEMZS9XQi+hFe9JfcpQm8VOmfl8rl2gEhbqKwSGfIEgQ1IlP7FMee98AshNc15GGgMp6tUxVSN9g7EDKbbiN3XeNqOsdkkajxLdDdVYnd1PaTA9n1CZPPsHGK796f7nxC08n24Mn7BT2hDdkWXa2QVCt/Dfcn1fAUZTL0gsfvvzmE8vIbeCT+j4aVplLR/ZNR8rLk2db/pIAS1AJ7iUWHG6DLlp29neO3M826O+R699uKiZn3am5sVuydhgAd+a8tbT8wmhqrSUaridpt8W9uvkw9NZKMqYo5PUnJ9H8I8YvgrVsIF/pnmTI/UxKZm+UK/zV+8hKbOl+L1wiH66vH5SixsHSV9esMeCIGM+YABLQAOWOjPWozbssZ0atNnqWZ0yA5JuWJ1xwwhA54EYiQhNc6lwbbbA6sF+QdjBZw1jexQ5KRvVTJecj2doMxVOGU3zsGEMh5XQUFEohCVjHBFGh/nYWiariUsPx5YIuhkgsttWbo9O+9etQ9Z82/6CPe62DWslBlGUliVgEDpduNZD0UbTrCJ3QH4s1xbbA5JU24ONY+Kd7U6qYLdMQQU6Q/d4wh6JEMUuNKoU+kSJpc6WKcbe0gdN9USvTtumHG2YzhbxsS0WvLQg0kEtlRibU0bpS4PqS6TOiQLshI/mGI5Q457wCjp0oQqVVUkQa4JuwBfIeT2i0eUl15XUaXj4VN57sk+k2UQoWUENSjV0dWRZDrWK+FQgpmT1BFaBHMaWJN0YBoznl7Qf871MR8WtlNuyb11O+YpJdrvErkhtoeMTDJMNrNkYoIw0RxgfONvJtx38su9yEbeYb07gZDbZrtqXs47nURxu5tp3ppt+2n5c8WnCG8ll7+72/hmWNQ/ocVxKRskq+ULubYc71D7Jpezbr/97YoZ7e8ByyMy6TXbucqwB+KbRfR1LDkGhD3nO1/hRFm/MbSWBHsJ5chKvap+jPNnvJ775LpksQTuc1/oRzlsz3/+OAEo46RqukvXkpWj3Bar+R5PYxg2pQ+X9KBRdV+ITrrgwhc2Y3l1HvZsTBrFaQ6Hep0IrY9gh74Ems05O63KnAe0DZqfHRmk86O//BIICPceSRN9v5kbwMVJVFxg7z9oxOMNtppSiZh3fbbsUiQKa9u51FzpVBfLeQd0GOLFXlMa5nLQoVxR0MTdXvTi0yt/IS5Fd1gc25zoqe5NSJzhX8rcZO7ejfpQT9CU52TBOm1YpMgqfXVQXX8SGIW748ijjqAGCMujwWkV8l/LpKl9QjHl1ZIWMgdbIA03e16UfFh8nB9nxSF+RDYflveTbX+U6ab0eCSeaNhX7gA1E0RQZFk+vhGNUor0Y19AAGA2iHSlnIwBQFOTRk2tSKCRZJpKZwdu7mUHuCpqnjcbA7YUS9ZS42VHejUL2kgurIPtx/CxhOY7ys3XAVWF5Spsry1AvJl/8QTYYCpwiQFyWIpwYl2FV5dolI+NB3Nq7jGEsLykYFuihA4wZwzvjy0tAIyj3XB4aRlOG7UbMJhpXTTrk1UsbaHNSwHjpM3YP4P+MbNit8vZX4co7r2d6UHWViIY7Ze2uaMiK64MdOFltDU2+FWLfVI8x1ACe66c0lO1LdBqsacV0LU5fm0Q1slBJB8dLHH0Bo2TPNbPseS/LXmxn/oUW/ek8IisMrlhZ8pfLdV++TPN/nzoG4KkTPqCSzgQnVhiNJMkMjgS9jPSrdwDRohdtQIUgawGj2w8qPVaKUs1LCN/51hJM4Xc6S87oVUFQLaOTeHoRc3grzDfKuxPWa2ODM2tCX/GDTKrJ04heROmgkttU0ZXECi3VHKJWVVCnvhYtVHHqeFL3qB/PSPVfMUQQ3cB31W8TH2p7I7yjeQ9vZHuwzdIr+bpHj7cHY2cLtZxM1HiZfGbphN7WIHOy7y11X0xN/FjeIdF8F9J8Xu0pye18JwE9dniyGPMiRehZn6evODhsuj5TOo4m3TdSw1R/xzeq9S8hR2TrGqfPf2bev2lQ8I627emKPM4E1Qe1QNceew4yPEUVsp6SRfurpdeWD4xC9LYD2zN+odvzOoR3z8JfrfniZM0VLbescVWtK4ccckbBYeBqC04AmAeVQ7B1BalQ3GBTwNenUrGqGsOSFCNbqca8t2sk0aWATJI0bWS9qN7jh5o6131cnxUeR1ePvM73NoQhR8bj9x0U7YY0VUEBNI1AfiW2i25BtfQnWr5Rw+BW4IbIpQZnbQpFNxB6CDR0ncPWoxXVSbJK0JD3OWE8Vh+gZBLqxaeJUwltEJV8aNErrBWLLBWXcZNsxdKfqPFsE8lq8Q32wJu5lop/awV+HAhztI0HYV6w24xPxZzI2K9PKUvLf/kz1Tc+QmgshlejSqoui/4opBgTjfRCsGtiRxnLUQqVHPq1ImJSsDET9mJOd0EpnsBi4u0v1M6azhVF9kRLQ8GODkgoYp4YSx9sxo85dM05DqBrUxXEvrOrX1d/RsAGOlfEy09pIBK5v3CGNVUtvzMXkerBGRLP/LPOUphgVGbHZ4vLmuVt2mARBea7VVG2hu8VxC16zAPniSfxyy9oacaea2+vjISKz8/X3WbgZo6D3sueJVzdfDUABFxtXm1sXbL4QzQwGnP1fP6SsfvzZ3r92bOAjh6qM0WURnz33VYx1dVFzCCReFgi6rChsHuGogt9rG+w4iRsBYlJgtatl/FEMjfFCaz1Gu8QfkfLjLhzP0VwRGWXRhMlvuVZrdgTCotFkoXNvi/FM2Kr/E4yjUxbsJrD4RhsZ1XBHJQ7ymCQoctKMikqN5Tb44wEPfPMNUBH1xdmM1SppZm+0MJChJG1tPQuoxC7fXpLy1RzNkMKMh48NJm2HczIOLgNwQM8yMMHdsB9U7iIzWasG8Q+q0ejMCfMsNJzdgtw+8LOgNRTp6p1AuGn2hciWLpU5WmuuYRGoadk53hlLV78V9FSOuAlcRvUkZyQF8vBkV0hubdvu9N/HgrNHTwCYHYwJI80JXN5YCl9urt1nXdmak5g6SiLwmKjyaKwv2yfLf2wIC4QXe2BRqHTj6X9D+1zMjo3pR+NwmBnMuMDrR9AfXAvob7wc3ubmtjSAi5FlVYNYbFDVVGll8DSpWpiW+3LyC2EGw0hALjqxmJT01Sq53djY+8+V6nSUmPH4rWaOB7gxWk1a7fD2VECgT4qnAkaNgKNjhZymOMDgdnXb8ZmRs+NbYV2+AV+M57f9VPTYudGJ4Sb1xeF/mvhmS7VjOrqDA41cJNZgGsQpplp+hCVYbW1+vdKiJ7aToVpbVRI775vQCbPLC7/9s+2FTVjeIRYzzdpOj9OImME/BhSE78qG1+KLPt5RedQeIjq8M9SQiXS82JJhsftn4tqAqUBMk2EH3+Flc+vmcHFG2q+fklOR8y5+lZzmuif/ydk1tc/CsXPQJ4+DddRuWD0LQ/hxWm1cQiCUM1UyALKCS4XoRxM4mt0sDKD+NyMEE7s6TNpejeKSBOoDFDm1+v1Cfjxmi40iYTuqhnHJ9whhBSkuUZEcWv5Ks6cjgVrDwXxu5Fon6FINz8ohAGjFN+yQ0IW3lAlpyQY3HzEyiKrNGTVdSfT6fwuRHrhSrGPW/Q5pSSrtrn2uhHE5v2Bm5g4a1zJWfcccAiwbbRvyHAlKCvXRvigWvOQvFYUShNRXoYCUiczLAUuo+MTSIDUxDsTbItfffH01eEpuVSnFERlJGZnu7e4UchsN3rx4b4c4fWf/6cVTj9cRr/AJlPcsC2l2dOTLMVIsWGAswmsCz/pB2INQ1EqWXB6jzziYZQerQ9ju9Gr6BfYYSPF6J8Q+yh56JuMZOCFBmgsM3w6M3xOrAoHw3lq/BxJDf17JqQAgMT3xNTExor+9kosQuzJ4QP5cLzqUmBDAwCji+L+e1qhjsVWE/DbiMCmMcw0nKIWbDayaWmsNffp8wGYAJp0P7DjSM5m5jJxZjJjrWQnsso1sQnVHIZmMwkMk63Z4dgj6kVagsFAvN8rEqodP5tsfIy/26F5CXxD/LZO3ty4Idc7hJkMeatxueAvy3EtTT6dKa74AgeXg33R91+0VMZXCZpFx6UIi8JiZabLnpcwiHY7Ygc1gzUi9eEz1wLIwXPyHBCoNbtEfdBnTG1uOQSV59ZiPkN98oIC2Qj1RyZoEDvFDcwfqSOyggK5EzoH0XN1griqj9L7Ac3Oco9ylzvLTgN+3BpzB/h0TS7/G/7OhREgXqiLwYjTOZ/LnS8yA6fY+8trs4hlxeGsLLW+A3HeYidwgtj8+qBmJzNErc16680F9Qv94je5CzarCRMxCksQQ5zSh0dj1bu49XlYh9twVxCgK0zUiLL60QOqGsvqRBv07emATKbshE21QCPFGj5gK4R5M1pTraaolnvolcKeMyErJxBiH2PCQyQABG8Dp9+y2SHViSJwLKQrlFW/l9gZ/5P9sjI0IX0hN0N7Q0IyptSRWeRjTSQmqZa9pmPRtwZw8mhEUn5+MrCkiEgpy541M/D/dpXQrhCQdEQqER7ZJU2O8I+OCoiYQzuPCkVHd94/zwKi/O2bipaKGxVV+amp5flqu+HSmhPklKpCZdypPzo71wfoIM99PUW5AwPK1OaqAvmjkU0aYkFVlUL8008xkidPlMkJGVHsQMVWjSNFe8/E98bVQgb7Yd3levykvV2rvXCBCwE3ml9dhx0AaECQ0OXBAzZxMH/wgXuO+u2DErtWcknfqlmcH+1ZWYb8I38hqSD9ye9nim9oY6OS8vRJMrPsm0QN5EZEivZAB8UEBr5bty46hsm74+yYV+ZXGxMx0XZQ+cUJrf2xmZmxtC9L2m4plU1x/zi3i5dKJKu8zSRzLzqdMm8VmZjKi/GP7hR2/intLrH0BXtSkOD2x7ldAYP7AtfA2bg4J9O5nXsWzK9bprFzQGxeykbCfunsgC8uq+T2Rkb2civXd6yrjNmQuSGG2xVp43Zy+Zkju7jr5nZUdlT5ILt/apFyjrGI398UFQnvV+jS00t1OKE/gK0IbwpXsGdOpPHL/X/YsHX4dONyYpHg56/ObMqTn/SuJuBKz71PsmnTtrIqN1cHjx33Uy7WEdjTCsx6vbk1La3V0gpWsvcHQOU1iwVuwo0hw80tDhAv+ssRt7bOqsBFs1/CRXChGIDVddZzm9EFDHiWcTWwuSyw8GwwQoFMi997P38y/Sb8qZ+d4HL8xPcC4qqHzJADkpjFI+ba2hFKMDMvCa4br9cpTorPfQxIHlVTMqBIT+gGDwpWmD1IYIokH9R0Ez1Yu6E5RqaQx80k6Q3kylNcoshpba0qbuYt0VJOdXCO2CzeTKAgkZhBHLNF8EjAt6bgV76kDkumkUiFRDJbd4/0VminT8+sI+cRWavIMlJ3hK1ilip1OG3NQr75qi9xlXYSydQIHgkEIwLN5KRAmsqFuCAIsTadQa4kZ7H5Tud5ajKnT8tkVtm1n7JXVuRKNSzNjuu3Cs9aQ8eYYOgMhg5ODO1gPyWjTX3oYEnJw7fqpOnsi48e8dnTk4zGleWhBCzEY8ccExMulwNMfnGsXHkJubQtd0xOuhQwgOEM1Vj1MBg92If0oeSjeUyOgcrJbF2m1xAA99tWZhwrotv1IC4IBrDNNSfcbT0rrAukEfDv2os4Zk4363cGpI6NzXlzpzvAcfbnlYLSNxE1nOjS6Dd+LQGlAf6OUlUDsYbfQuSo46mzfeI5gkPwMYHRRwgNDYE1wTZ0TY0l0BLCTGFSjI8eVVYuK0WotVSYZqYCeAwAYIfHqWYaTK4DcT5TKW1NbapmmHJODbFsKVNBd7Oe3NPXl1RidUPFMpYuO38eJIgVWYVnzxixKKtbSXJv356TblZ6vk+gADcbA8u6lMouyyKFYpHl0SOK9xgjXCeN/A3E5gMbm8IaZ/13LOB6I3qXkeIKx3anXzUsx2JPMqHDbhsIUMABARQKFxnGsn5bWbLR2h9lNLnM1l8GRMekhQQBkkZdLkPKLu8OVJJB2qGZbJMtuvnkCeTEydwSe2vtWeNBvzf6zBU60qM8LZy2H5De6Jh9ZS7b9ogYNd4rS0ajHZ0xshdwWfXrD4AOqikXh2fcGipWGDZiD5sdANdAk7ZJIJMJsr+++qbsJjJC1pGM5LXH1/nalDx8qTEtzUn5UvvaDMG1wxdfsyiszz32uhAezCtcwYGTga/lDjxDHhc2gBuHjerqajUCI3o+o99YAZuzj0ISmFpz1yYpkyCZ+a4HUN0YMmbN2y6Zu7Mi2ImwfvhiaAsM7Gs9jICoat9kNbPqNqy7NJxkBMCuers2ptfV2cCgGHjuwZb+zjGf3I/pd58zZ4D7rSWn/5D4+weUBpj837x+DbjU+sTXNW7kZWdzDhXmYsvGRjJM5ug4XWfwIMQX0sHxRU0ghJ+SISg7D93LyWH2MA24UrOxex93DsQhnCXYM3Rmd0LtkElw4OH+OtJ0RpgjAPqSz0eoi1a2wIWD9qrk2M4hI8Izu8ptfhN+uv7+Hl6GcSFbGNugxz0M9+fEibPLZoQBcpUj0NeFAYvqkiBiZKq532ybKi6QFChigzrgSrfZrHniYSO78oNHOi51j2KDkHhONbrgUZitilj64XHYCmjGHriH/47Pn+Dz8OdwNoDfBG/i/3ZPPNqPx50rdO10y9Q9aeuy2nCbHdgsAAGIHYEREHe8fhxBECuAbRAMAdsgwmNR2FbYyrONqUoHZbSdo2NXXmPrPG6x71SnNlpIG+MBkLK23kmObCIRmyKL3kL7zoksSmQMiY8fVRxTHusiE01SMjnG9PFpvAorIYsvw6I5+hA7n+4jzms0Nz4DCesW/Iv2RNvB/+U3dOiWrl/TqwmBPQxEXT3Lb3NgDMdD1/NmuSak6NaGzNKxbdGCxozl5oY1fTO73mzyzIojp/gFx3rqqgiGgMGKJQ+Z9N3+s8DVqZcajVIuFKSmFhQAHa3pbYYkemZseGDQBfStWa9bdnJzmFPM7ceMW0VNy9/0eOr86YETAHP9g/wX1bVjfdayy7b4/xCevyFracMQGWCPsDTBMDafmFVN9+uEm8jTC0/+5tRVceKqjOkJHkI8wSsy0ZgeV1kVrru2drKQWNNIma75vOuMqXL7W6iETGd2TmqI05M3BsFBxosLDzamFhSk6kEAQvLrEatyxlnDpVZHUbGzPPEMIKe7iosdduCTUwRNFZ3YLwLBF2xnRZMAI5dtlckxdv/+uF5VrUJBfm/ifn9+2ez9gjWxX3ob+SAG7Wsds/KAjQFaxKF5typr4rts1Daa1alNtDb/pQBGw3kFQGftQtRTq9X605uqNZkuonI/DNfk8K0WXSpsCts+z1mM8KnmwtYTcNFog669NSuMAEjgel0EDOpsbeF6/fDw2rW6LI508V23YlyHnBot75Y+TSzmz9BbPK2GvjIYTz8VgqN/F4KhnwtBN1KZ6607wksaf9P81kzE4TSaFo0WB1J+fs8snGmIs/U7JGip4/4Drs4bmmEMN7JnF3957YMZcY76LTPo9UueEB6Kn9EEEjdD05SZyUV5h5alXL6cmUz7tvLhJIbsVpp2ZUBDkg5naE4foM8wcoyc2WV//07Fg/DMYnk0SHx63GJxMp0WS2dhA7+EBCPj47bxcQQmlfAbCjvBKA0+7+YQK/vSF9kAJmVnZWk6W0vn2OdeTGuysrJtkHS5UEXZtcGHggUGrHbg14Vgl3QtOnu3Fd+7XO3Dly4jlzfSfhbfIxTYVm4ZRexkWhH47t+aSbO658HyUTul0weGIWvjyQ48dG3FTC6hTl2u37hRW6c+EHRgg+93vaxiec96T+C5vkexG+u+Xug+uVEvZt5viO2p7KlVGWo4XkHXia5b2hjaEe4efTmGjNXIdhu5NsS22IoYb3J4EAV8qGhZ3GEcTsuYMweCGBx4cjjwrbPjZDIRac2ryTzPZJ5PUooI5dFx8bzvq0LU/GtDZy7HWi2gizaI1xLxZDzkseWHq5TiG+LMkA1KAqssRxmiCDabQ5TBipz4Z88TCpurseF+//rdZx6OrW5+QITG52QgCxVQBZWD5hS1fU6mUBuolOTPC/G4aPmVU7x4mVSjwWASEjAY159UFs87dSVajsODyXT+hEBRr1ixoueCCf4KmSpgHN94saIiM1P9YsPcuWCiua3d1t7mYM5gTk4g0w2O9vaz1+HszItBgZOTHz8y+YFB0dEDxycmnUynvbDQUVjkOADOB48T8OM1rFPhWWQGnjADBi5nE45VNiTx/tzYfmpbAXKANp8ZerFWpDHtDsul66VpbTV6PVKbllaLOF7TliYNIQVpKEFZSR8oaKrocv8igAqL0udpi375pUjrXrdFhZDgi3tXRVORxnxQ8wobf4Wn6xJi5TW6zyLZ+uKSNSUzYvBG9v+S4xPTA6WMAOcGDA6rSG1WdGJo6Mhnk4q01MjI1LQqt/9GEAhclXOtkxL0/Qzq4g3f+rrh4PEpzehKZnPhglryTT2sooa6ZVEYlLJW0auLaiZr5Fv5waehtAu3E70J2hnTsrNnNGRiUdyRy7TQdztNgs0sjoo0MEdUViGjgJeWbpw8WT0JALqCaej/p3CBss/aNBK8/BgJy9ry4Ds0jsCE9UlxxgxD5ExDmzR9sDQbilm37ld/fwEBzZ8MCGRZXJtSb0GbkMldx44LFBO/JVHNNFvgD4ZLNgn9DCs53zD+GStDo9DhByOHBRzqwBIsCcv3xZ6ZZY2KnmU964EVoInYHWeorP9/iW2oAQDgmR5f7lVVJ+MSPruTIZgHAEhfOp2aeSU+xQlfNGladljQJIMZdKKJHnGG7KQkgzaO4O6xbKivmkT2vHI1xbShy6l0dtaEo/egMWJrNBil3dmcsdmq70uOq3nX4T56CsijCJdWOIxgTJOTimyD+ovsDkW7fygnEGH41QDAfcx4duSMXnXOAaIj0huFxV3YhgmWtwABABqkWbZqjMvcLeNBIeZTnwVgfLvE5pYckdwqPWoAo4OBlZWOaytxODfQEscSAiAX40bFGNeI64k1cMns2LYGHeZLAEbs9t5gx7501xTlFPg52njLJviC7WqqEAgqmrqwXwS2StwOY3+j7vfXBowWyAbZQAqlZle5oZDWYuTRCg2pGJbv9iTsXj5HnzunMNDTjRBQ0L7jzh1v7zvP2nYUBBDcPAMLOyhAg0HwVAYbjoizWSphRnRpgrTaR1HsVsH/gu2r+BA+8z7sF/7tBHfNXQ5eY4UqllinKPKpljbDD/y1GrIYzwobdclitNCAsBEkX3htYGWEZYTOi1PHHbfrFkvowVdjMbHjWzFojy+Bq8GAbpVE/WPx6vlxNVAHtdK9oyTS6F7xYo9l3Smh4rvQKB3z2O5PQ30o+vOF8d3Jy7aihz71/0lkM6OiH4FRWlRF5aqvX8X1UrUqM9ePk5zix9HmwmopD+FFjd+Lj46GuOnREfdeRdXHp5bKFSml8VIaVcbn0WQ0CIay80oVCkNptlT89euqyopokJj3LDNMGiwNbhK+/DRrh6o1JiSoR/QRdbF7onvicu7x2PVBIGR2zFbVrBefZsbIgmXBWYz1JwiEE+snJqoWJzSJClclF3E2d7/FDTSLF8VXdf++uYi5Kq0QuGhGi23MZrcZYZY/h2ljcvxZoxbEClutPI9YBMYqIKN9j673zDJonGfHuhOUcfiwBtHA2yBa8e9CNAiBueD8Q4ytR/MRw2EwK1gfjTfcU/+5xrrh4YvCLsBio+tP7J/BNCbWE5ii5DEghqfqAsdox5uxwsdDVtJXBZ/miGkXieqyUjk+ZCB4Vcipx4cuujOEVno8czBIWl4uDfqWeXzVKiZZTkoOj9Xp8quDPUo44f/1YK5aRfsmHr4oV6TwxWJ+ilw+fNEZF++C52JRCi8l3r0ksmmnT8vMZE+bOU30jdLV1GTWja47rqnXgli971idKdIU8d13/YKpbrxS9FbszKfk6T4sRMhopXizyl1VYBkbv23oOrRXC8dstpJaPJoHD4qLD20DGlw5DH/B8ex2kHR0aP8+h+PO3XPOEyc+fHD89wOIjnN37jhurNjkdtK7dxhmXLX9q6pC8y39LptpApafyxerZ2c8F3aVo892yahDSDLCnovLnzc2JeR9eBKnPrjdVPIwMHkoAtsPquOefEjtbmp8bptRfJRtia9ooebOnJmrb2ItN1VPjcNO2Gze3k2qNNUTT/4EX0v89rDdttSFg675DqNxYgIdR2+Vq0OCgbpuZm552pyMDPLLspI1XrOlxsi0l/XopUZKQxWtlmql1tLk4al2789YKD+/+mGRcVUAPR6q3Xirv1aiUzG3aWCGff5ylOr8t8DryMR8iTr97g1J5R8bvN4VOrofZ8RgAGDmzKdPhTqBtRNbtUyBvqlJzzsqanL5ef9U0WPk8hiu5r/OpU8eWsVwBrbost9m61qU55TZfYXupCvBOWetiz3iSdLlvcrLJgHbKDIGWREr1JyxB7bBEPkgj7DvjX3G4RNpBSXw3raVU+5/JJ+98ynrhqoYjUS0fh+EIGX9EFpXh8NXceN59cqCoJbZzEuo5M684ZtnbW8QigbJpbQEHr+Y0tdUIeBXNPUNyOfFf3RxpVGXP07KhJDS1qW4rbxNDlc4u5TOBVDKvPLx47BckPik7NXLFHZdolI4/6NrgVCdMUeY7hIuyFAL507eP8EZiXXs4ZevHJMDkhJOLDPSJJaEl+SLSiIlYlN4OItbIhFzTIZwEwe46jiKUNODugQUgeAW+6CxSMHgvNOBedrlGiZFaE70YIkHVfXfnR8Cy2W7ftftA+OgsDTvUVhuaM5eC3v0Iwd59aKli1ZTBsscLuECtYLlk45HVc2hujlzeby5c0axucpVWhVuEoshusrRs43F2tbjMDt6t9HpgdI5iyoA0bGh+L/+ykjIiAeD/EEQnzHC1YmvMEenXn8wqyQtdUC23t19vWwgNW2Ye3A99anpgJAxdPGSLPnNm63i8+dzFBt2h6FPNullo137K6nUyr5be2jmyCwIYJyDUzATIVzNxCfZ7E/54Sn8BKDRRhXsOPpRb/eY1eK5K1LndMU/eurEgGGeySKHFO0aizAm445yS2iYaakKWWh0beGeCW7vll31S4RTqTiN3ZIKoCu7bm1j5hB52WZn45jKaJlZKs7MaEtFLLMkkmZInBugj0IHU5+7xmIQLkUA4CaJsgFi1ygcCQJO1w24lhxQwK/oB+Fd+lZXdVQtZ1UOV17UVGorYy68mdvfv69/3pt5NhfZv+gNSKrKM/FJr1peLk9a3tNXpEtUQ0HX8HAWOWXOtY5jfS97jazqMhZ7uWq5na8jR2XT4xuAi4Y9bni9GTrs37n/QFdKNuXicCuAkEN61fJ6X9y+pfyKlNNCADGID5DSdUAx7OFyYY/lb58uOZuErFi5so+oyyw6bsRMHbFv5coVSNIfpNXzj2FBmrzt9pvdvut/fsM8xxETMF6+vb40O8j47iPPz+7HeXneG3/ehD4qEi3s9hodty9Pnj6d3qx9E81riWiOBx59W+Db51sq/jSV+mylhfUUrpnYL4KGXmxL2Y/zCpW+mp7t+/a28uu3sXt7xgrRsz08Zolc+Ifn9sqVlUfMo668/Wf0hm9frwSU1Pd1tdGREbfdl1pLe8NA8N0el+EwVr++621am/176fpV174oTuWYXf27vNq2DFnGxRIIWO4+y2Gsr9hfsX4Z3K9vh0ZrtxbfHmqU7WsZAhJQvviBQ9uzsn5/W4+n1lIR4Pj6t78/zO2HBrQ2BKv0/NqqVm14lLhK61db66cNuU0E25tXJT7asCoBSSiQa5dtwwdFHrRkEfa9AEs6AHr9SFVVmG5OF+/VHkfUbx/KTgcPqdWx8/9pbHS5hExnZeWdO7dL0BV0bwwl00gaDVMbqtWOaz+EeamSjOR/XlMych4T2rzLziTLSIv0a0tmY6YxWQnwK0/fxqrr1qxTlgyNqxg2HevCUcovsOysoLnVtzSQug3Rm3YUb/WU019hXh5BX6+O7u9/YxkCAFJEmlaLMB68EdWZIkyRBTO77iQmYZvo3uqwOCEo5dQpx7ZtQUAivzRv0DnNt9vpV3t9/uDGjp4sxldCyw7cujX70LQH3bTP4CrUItWODadPG04dqAohK+syohR1QNz8W+jasTW+//u7ckeneuThszv5Ycn87zeePgXyD/ygbNwXpRldywB/N5b1bMqiBwUt+/6cA1X87e2V28YMQU2PJoq7cTYnPNd7U0eGtrraWz932uzrSAxTU3fDxzx4y0z7J6PORay8DsKTp08/NHNmhPrjixPziyAEQSD4twZZ6+QtoZYD56dXb30w6hgdW7oMMGAwcR8edMFRlutRFtjhauQqBxQDoAuA0bewre30MUAwVINasTqX76/CPShtYxXwcgFLOnYmc9MkCYZgmNpG5dXoDJc8rDy7FbYWfW0eH3721rbqS9hRXHlUdYy6NZeqY7y0fMuff8pzBKExkB3SJGTo8M+fK4GFhp3J6olMQ0batcsJaISN1cCQWnUZe4h2nVIP6VO9qWrIgxYXLEjQ/u93Hs9uYYcTxDzl9mBN+CvFqoLIZCVk3WXrntEojdmxIyZmzZoPZdlbP4KU29LN/g5/7ZYtupgz/eGsaW1iVu+ZDcLsqDAjJ92DGF/ZnJOpVqWkqNQ5uuaqJE/PZA4cGiXM3NT2MFrcxvcP7z+ji9myRevv8P/8dZW5rBXbeOIG71C0vjmW2vwzxsML074ccryPS1LFL8Tr50IKfmqqnPusjpS9L97dM+69A1reXtFXy210baMQrZePJnRnG+mZYZmNjVuFbjRmXkAi6WUas9MP6FFV/vjktyc1NPWvKQTMJXRi9nZxVby2H9NHxJDBjpyahknbSBQnMI2TlkTIRJMwaTNZyqKIZvH2giT0JQwhaH8irdgq1GqtwmKkgqtL2jwYn5gekjyHXLL7bZHUmGriPNxFKz+Z7Ok5JeXfO9mJgl3MhlTVVVNZM9+BpKOv8ho29uj1Rdorebq8K9oiYfZsbGgDWXOZiKljXqluccTHNQwvXUNcvKOl+srwrVyvj/9HDOJuSRiv6E668deTD2KLI5tZ+7PDlISCZq5W47n8QFSHOUm5++MvNO/xA18Wp6WVemqb6rKz2dN6lt5iXPzlwLg3zc7a/SnJ3BFVe9lTo23mFigJ2rBXIOXnh8wkaM7U/EgDt7oaaH7k9FJCScyiEJNbdHdQt3NC8cPpcgWLHR4uEZfDjh/k9lfnzmZVKE6flluKsJjcGyiECkkV+RbXvrh92zundS8sgjyBJ1RU6K69fQEBCIC6nqtiYw9IqjwHOt8rRgh8iiyLKEaZqNSNAb5kKAYwAaHQQLZYyotAiMFyQQCSZINMWOowjEOXAqHMIFq0mlogEoYLfBl/rXv/tO1pO0jeBdbuzCV4f9itRhUZ+j63LP+yKMW95Z/69Qqh8N8p/NeHw8hFkt27sjAkkyK/iblt637+JT6Jl/EP/eSGKx8vBgUGhjSHycJk3y3I4WRzjviVUEooBgnYFbfZudm5q5sRxmKFMZihLFZo8z59sg4o8aOD8WMpBKnUPEDsIxYbG4uB7hApgBykAeuWgUp8w2d8KUCQHsR+2N5O29gJCtAgBl3f7KiGL4OvXVOSRk8IF2NwX+B+29RyfF9QhsvwLZLs2qlvsA82TuDLjRHWB5KXALjn1yNdbR7plWQ7uTIaNnLl02FfQnT48bH/EfI1nrIznu930YzrHrX22GgbIDMyLzobk0gQ5xAsV1VULNdygRQAAHridAoEgJ9X19cgoE0NkiXFPalCuXRKZy8X6uyUTRHJqJ67vkGk52QtUcRFQllwpgEiH/nYvp/0IlWM9WWIZHEB1zn57YGgY0c9HfVAbVuzek390SPmU2SYtqqtVpkwuFOYvTDXeymFEfniBZePxfY16QU3WbKgzs4gKVtdPlWFeUpl8aB/rOYK+LIVghBIvym8Le3fMsLr58JdVvud0l4PUM/78Y/whBG7ipOA/1WB/wMJfsWDMxIC1XUet/RyBAIGE7ixfoB4ODdAdkWSBn/pR4AQLcDYGQEWowkMTGBgAfr2ngEssMYG+ayp1X5qgMQTySkJylMSlBcxYYVSPyVeeUq88iLFr1DoM59qZrb6Y9UE0skFB0IVEU2EmJMIH/n97GxdrNnfJEhNLRH418Z2TcvAnJthzjC6YdDDKn/e/D6s/sTpoweWTdX6l2gm3XzObDD3Zg8DdMSZKOt0yyaoPZ8F4Avfg2mKSqlF+YNec2VmiHQArFy7R3XWzNyz0qQ6s7sFPhduWknlmDnqj4mW33wzor5z9mx39xsEZFwEimekN2ohPoNM1pgOaV353s8I3I6ZNkzcVJMXANubEtt29qjo+IoVZanwTGciZe+24B87e4HoaL2tHl601cXrPk9BPPBgsX4rKTtzKblnc2rMLAxMbgmFx3CyApCArt9yH/zcWuQfdTbZu7pPXZanluUkenz0/lZZoGPq57xRCIZhgFaQOB8SDx5MvLnNy0u6h/Gv9Mzr7uz//suIfOmFWnJJKh1++faPl8BYZwfF2CPzrPAYD4asyBgEI0VaAcDAnnR03Bq3jKMD2/1t2xyVFQMDDg2x/dJqXvvlUWTc17a3LWsfLHi9VzGM9RhWvNorAMA4KHQfirVXKajmwQA2Gps9HtCUct0+7J7NsLZ05WlhmDOlLgjvFxjDjdorXYVLIy7bihutdHbmfrPYb4Rfe8/CzYIPZof1e4X3RwqMdcrKynM2m5N1YyOOkypjX+1SqrLTYp+rltUum4mKpsPWT5xDC9yTXsrd0kPqcfUhvnJ0ocUwg0EAFY0rNH5+g/GNSBZUz3NaQnSJiWRrNbcGMSqCx84dbp/eyEteulL+9hee8uppfoJQpMx+8HsBkuFx+3GJOUgaKMuMvX1baGQxf9i5/C+NoLoVaWWqwUuaw8RBIsQRCOeJrrDm01n3YZ34dNm80oPiJ7LfTX8YWUkpzUal2mhUO5UuAow7fTopryW9aWIEH1Ic3vGQ4k5o4ZvTz+fl9Z1cyWYXcZ7pzWQCJtfAVVaUqziqQgkSKlpbz7OVZeVKRTnL2SOtIpU6ptQ3nxufwD18S2PUaiZoPB/SGRLSpaGvDwbPt440Q6OjCEDYl21MNR1PiE+q1CIwQ5tzwtzlux2lQrVK1Ob0k97JDGebSK0SciMUH88VX7Ayoypn9AFAgnjdnK29cunWvvmlIf59r7ti+YZ0nt9P6un+pm+lFWfJs0gFIbNWUkHybh8qvbZ7oaouee4b4Br8GMM6EVeyv0hJn7SoSMoV0ifKcvyz/WYa335V1yP16q9vT7jCk5ZR/uR3XwtgJDe+ySJ3q7vbTcvdLE0SBBa8Bokb3KeKTTjGLIYc0FS2rAAAoqkjZrT79On71AaDGmTUxELIzisg/Qjn2X8R4r3e+Pxwpp/lBIBhqwPf4D4MMAzSX/1xJ2whgWt8smBOZDU3/49Rr+du71kM9hgydsy6BFqCLGEz2LVbeoHiSXB7sHWmsIjDk+dioCRZ2rELItGFY90Rk6CYc5EdEZqIjsg3XwJY7kiC81JSWEg6xz5XWal5oyRg8eoAPFVMJQSgTjg6PoC1ITjYFgxS80ORtn3m7S98cKxyDjkvcf8oYmU3xjGHUQNWTJvoyYvi+IfR4M1BJI0/7/Ms3jFkhV2bxMdReZg5DDytEnR879bIwKdj/JzMBqVfrx05BEhBY5iDBMBa+1ZRWqst/lm4LanVuN79cAvlxqCs+ALhHcHTTl8gPPL8yid03AYabtMcVYYlWWfoTktWaTlNKPWtDzGocn4vTtvQoGeu2MSFkNpMZknJnC0xdH9dchbD7f8/LKp1U0pBDtYY0dKCKalXscPjRSAK3GYlZV4/8PQR8SaeTC+ovUrEu/D4f/H+W3yYYhr+J2JeNvEngjfNLPmyeFbI2b6CLR9a/ASeKPnTBp7+zBArRDTCTSKI3XlHUc5f1r06h3X/GucNO2dhO28mc4LpZP7LxIEy5iPmeyb12k/qxFbyWpcUVPECbuvVQ1v/ub7qxFvSZ5y3zkN+/aE2iJ/izs9ivDTdvQS1huQF54VPWza1pjOc7Y6nkvAKxU41yKDRi9qLSxawmFgPui+b44bGY33u4QhZmQczNCgyKY880z3hRnLznqKkpJ7jSZ25PRmDjpOSN9Lwchw7rs1CHedJHSesgEuTF1ujXpzLiWjIuTGKUS/Pl3JmlP4CoLfZVXqlEo6abW5qNPN8sTZ9BW/zwrwKvQ3ry2MR/UaBQRsnH1HEqhUlJVhstZxYNxKHawhAXP7u//j0+zzkAy/J5+hF+gXya/YulA2zt8q3fspdsn5JgH7XZO7clXfghx27/rsLuGi8JwIuj7cYs/kUrQWJzJtqzGX7fJt2QQhAjFwYhu+/H83pNaG9p5BZBN9HfjQH9bpCISmYfOq3PSaSp1ronjO7Qa+fPcM9e6GKFxmzHZVH19AzZtRpwzSh+TV914+MoVgzrDgxXJ2Rl5eJneYHyUKa7SXxCQlE7c3eh/ymYTOTUOg0fEIu9nvk6/vIz9dkTj1ZdtZ7qLfgQDAEYJC0cx624ORhAXRu7vKx+XRt55UkXiwBoPnL06eNiDOQRvb0al9w2Hf2Dy/LiMtlZvDzgPF+o+1eW2RvnOTnRmfVF+meIEKYL7oy3mvzzxYrNF8x/WvxN1Z2r8SxaSv1bu0GAP5I7MGHL71RjwCPvxnfJ2WUi8pnyANOiAS63mhA/JMkP7ph3FAYFJqG/n4UYn9MQvzjMZUMBhvFWYfGotEBaFyuH3+dm4TBzMPQMJUUhgTFX8dJC3D3R2H80OHo41iJEe/iHQwfDND5RNIwie6+EIYWgQ7M0tXWiSP5YQR1AMVmMCoxonp/eYN/CPG4VnjsksuWi1lFvpgQj/2y/aS4pKRjbe28PXuXenkt3buH1952LGBxpPg3pUewu28RS7xcJt/lEdnLsqxT0h8ge7H1Q9PxxDvLpHvZ2Tfj8qVR8oyAGeSxS5caeztf6kxZ3gqCT0TkDBV8qroKheef4ONRVVXXVKoZkfuRQoS5DQGytuY22edxbG1uFYlnwwL3xTHCqUxmPJqGxy4WLyaIIiJW18D3ly5henoylyy9D9esDuJmEhaLFmMDMegMH+ZUYcxi93eabFNrwPolQoJXy549vLb2I0mJKiIxNjNxZ7t5yj/VapLHkF6/RypbJpLVMMcpIjo9j0FXIJH16no03QPtccGQ1yERF3N8Q3D4UI5fnUSxCX8473i4z4yhz2IwfNF0CkChgpX+xjxZELnsofiYU5NjWo7bi2RS4pHatlu2oWVMTj4pn3yb4I7exTFuwEcRc4EYm5h41Fw7Zc+GahKpesMez3bzUdwWS8ROa/PcszG2jXummGuPJCZJiBJciJ9vsUTcYci74OFxIc/QIZYU+/qF4HChvn7Fnnfk5V2ErRfzVIKmVxN6xjPHtDxgSfJuDW3srRK2TmA8blWd311VKWT4oT6Xvrvqvpgek09hhKNQWDQuU6y4S6DJaHXNUdhl7DQ3Jhm4o4l68cMMomhW85gyf3q4b7CHOx5Di2dRa0WijTKynEKRUARZdRF57bbok9HmfhW+5QH+Z1fqsU9kauTBSADtqbjwUxSTzPzGYnvzrPr1z48/HDBsPqMhtWzYE9RmPpKQKPb0lGB10NwWdOgoqRoI5IODiQkST08xkCNlAjWVq0kROdToEQd8fYNxuGBBir3Tc8Q0XF6OJcJHwn/IZIYLuAn2/jTOEU7afokCcezUEJhLWu7BcHdEBAMP5sXMcw9E0+J8mNWMmMXu7ovpwmqx4fFUNN4dzBPOwzMiIrph+F7LEiZh3O2HZM4BTvIPQvBN3hDVUNpwkxlVcoaj4jBZf8EbTPXPn9Ctdc93IBzmNHPg8AGym0V30+uB181RA1EgzQ1YI9sjAWctoTyiwloxaLGutnSUAvrwDx2RnVxiZFckGn2RYl9EZWVH5fKLHWhrMdgBFNShW9yiPZcPMVA5XPWmarjyVKSdR1a9vjUlUybnxty6HCAV9Hx/kjTide/KwNFT1FPPTuZ/non7lC++gdTJL2I9nhvZ5qmf8YQTdbEosnOFwZhW983TbhPdWJeBkCETkUwkMJnZYBMOQoI1C1I5+Wy2gTM7NSlVQESRxHwT9+mjOiMnPT4uPVet/vgpLv7FR2fk5tLcnMX/+CL+LnTx2z99gngstElzIahcah4NHsRrc8u5r6gyxDBzjY5Q5qRxmqX+7bEnPTwux2oivblpORgUsPd7IhckggJQ+IZeKZH3dSFIb79c0ddbgHR1ySVhlf8DM2MUdTpaXsZ+WZlSUeYDn5jvmcwJq/0b4WInr+LGLVgAS48e1WiQIlIU4ySRjzgWuckEA/ttmMWoxKArGbYyag+ljZJBMVMoPesbiTtD9tb3UtofhAz9997TKbzF++oM1Ya9dUC45CC9Eo2ppNvK67V2BIJPj7084OY/R+aRBp1ytfs6ZbCtMXm7wbtqVJbV0lD+qNMkHxKJSer6fSt+9dLZpgi1Ggdb1rIav7UOWrIDR1iz1P7n+4Mrzr9vWpdE16PRevoSbtrWMlE+0T6VypN3vS2k+yfpfGYgrG7lM94xnJb1wS4cWF67fpl5NOvue88yVunktzeHGpfsWSS5d0Q+2K6ra8/arbj/bbJUXO65e+GSw43Vuas1NzNyJZNciSWHYbrKd+/aiC4TrC3sx3hD8erVcCX+XdkDq1UufyPQGW3WCQAc66FX0/BezOpraJWHt6QVhmezs60tMB1mVJXnKAOVgeaiX39RxUfUlJzDnEtTzMh1GsW/lMsK2XLTzTADWR6qSFy9pnzN6jJTBrisIXYV+6J/Vbjpb4VMqfqvT5RSueo7UU5JLjOX0dbGGFlJyUlyCTsAkCgysfNli1ZeRWrabC52cFtGxraD6ZWzg+8EYg09cKp+X2fAl1/BIXvVte1uB9f1MzAbrYBHC/v773fVK3mHg4T5KhCAAPChVhaGwBkZaVKqKbGvr+rRoyqlkmiipkkzMkqK/yOb5V1ZciQrS9SH/nUC4Py3WrLHg4j2R9N+LC7JfxXSNGELaM7bVFbmP/jbIVAsK97eLOv9XqTLAkVtwfHB9rO4L3CNSHo+M6LOzX9BVncmnDjk5xP1q1wmf+m1tn/hPOaUKd5n+tq/1D45bat5jWfuxHDezVB7RY5GSLW/SWlqitePyNRzrIO0BFZu0Xtj9fnvfvz4+tmWkbAOQtgb3vQ/P88PxEE7+sXdbbP9pl2YMTO8Y+G8bcQPnmyii3gGliclSnxXek5Z6atNVyiKt0b+Fwx/LpkmSdxY665pAtC23G1qfTMK7+6JdUO5YazuFKJTqUh7ANwAcUn/wnmMt0FevZ1MBrIXpmJuf98HmHPYbC7K2wYYaFQMymdNiYm/tW7zwnm98iNubsANjXopl8t+jfJxx7l/AC8BHWTC3VkL/OvcIjIBBUzbmMmay5rxjoPyoJPpAIVGBVw02k4/qf3S3nfGe8oU5uGDSPWWsC2T9El9UVfe8qXrIeVmFaC41ZxHwJbIrerzGVtuRIVcY2BzBdrRyLCRLcghpDt+K/JbAWxXVRvf5xaxEmgHWeeQqT96UdQ06W9SbcQvx30iMO4RPswWLLaF6RPhjln9kJyyKWR7lqrJj+zY3E0HBfrNuuHnJfJy3Pg4+rx7OWfa7VUtER3z524Jh2J7ib0GFO3iJ9JoicR3JSFopa82SR7/QjxX3BdPPT9F0iX59DlBa0zx3BCJ6JFL0qk8Q3QR2Z4fiNsWzuuYGT7jwjS/2dvuvkDbB4kfPP1obutr36Oan2YGAR1dV6ijG2az6bKusJCAx477pRCrE/wexGQG8WSwj09QkHOWHgzFc/T44E93sO1A5YrKDnbVpaphTVVmVczPr+f122y2eXMrgYT23cKu/2hM2Z+XXv/LeBAuGRMIAIX4t1vMnjOCITdKyN9/s/86/hgn8HHryoPXhDuQw8jifUvgw7Dgr1kwBh4/cW+eEvBPKoDQu3sjIy/8mmnLnN9/m3T761wj/zLPYufBCMyzw3ZEtBAViwUGMEj4OBTRfDo4r5Tue/yx3J85fjr95KrErDpttykvW2ZYJANBAHRQbbSzNJMKqHbqWZoPTFF7ShUzfZcydtNm71gAdLZaUL83DYwahcZZmh31IWeBlt4xFZMf1QGafZRqn3RQBwec6iMCjeS+sVx+5VxmCVEIBUnTd++KEe7a7YIgIbGkf/PjD7c5H8w8X/ag7PzMB85tD8dlKOSShjAGagtBRHpFXm68r5FEldEcVDtGo6aY39U77tU5PkvuxRwpw9xXxkZHEX/zGfOmo1HREOt9wHhuEx+/KJOl8EVhb64vXEHJk6xOok9bFqDjLJiaLwQ/8n8EwvypCzi6J51Gj/eW5K0gN1y7Fybip8hkF4+LDQ/8g6liU2YZ5vsQ5uqr+2tk+ypXr/AvdZvaM03CAuc7sbpyn6xm/6lFzN6QcgwQ5PNAO5yOUGBTr5ENgR/NaYeO/qooEonLareCvs9VHWYL+/3HH6MXV1XNbbXZ+m2L5oJR2rOgvwELTQOQem8YCnDzjyBG+FMCLHwFTlgbrKwNG4xc0oBLKlypUvnSQxVACQggB6hCO1TGaaFpYbVGVUcoR1iaeIfj8UKlLMV1rOxogxgfxXE4k+2vKYf4HQMBYsbB+Jyc+IScbFbJzk6Iz86JWS5O5SsUF++KJcMXB4Of6qTYTxuWiO+WUv4+8cv7IGbiQ8wH7xjv9+3LiJoAEADwx5M9XqqMZR4/eJQZVS89Xg37/7XTr2li4otfZ9Aqg4P/7bJxRZiRqGmfnISKX9gclWh4FbbCqJoT9gOGNkYHUAKEnA9kuk1jjwcG8ICLAT6EMLveHRCoGtYqP9yEy+K3ihvIDLQqNcTl7V+/dhYSy+kHzOZ59J/o8/b5A3S1x/Bq3CujqhH3E65RZXyF2wz/GrXEGsNXQEkGQxKk4KMtZZX2RQpFDxOZHZeeo1a/+BgX9/GFM9Jyjdw04/kn49PzUE46CFYsaxziOrFwztRqNxGYOrW9kLgqb1BSzlFKIsolgozdyR+ujCgWAtwQZvR9pL17dvuc2YvXPfga6nnkv8H5YpYkWFa80NOP4PJe5++/ztsV5Of5+uqHQFagiNFLWwRrADL1dCAjMIIW0dkBWhYpM5nkeXJTicxAiZKSSRaZUQlIuh9JNlO+2/kdxUyOjAxgBgRaFkkTo4EBFX3kTn3UNuSZ/vKjXB3ow1lgdQm3E3LqZh8J2lRJJT4gr9/g5Dglv4b/OhI+ojjZf4hXW9GjhUiw0lFG7W/U+vV+4OIsK4P0mPlG1aT3lfrKIpdMdwPT2JXM6qnYMH//MOxUZjUMKGBO5FJfma+0Sa86c7V0//6gwhivd/+evJIVe+s3ofC3W7i+Kyf/fecVE1S4f39pDT8HhUalIUjaBC1NOfwaKbWfOhG3bdvDNjAE7MAlfPCX94rD6vmsAElHhhpLR22uUcg1xjWe0+L61dUv/P/b/+uZ69svcvovl+uxns3/OYB86RoCAD00Smg7+5usnjEM2AN7VfaEodfXD5Q9nlY/PsGAoHPUEKjjKnFKrYurqUWNkOl2cnySMx1nR+9hPWNqKc2kU88hcXrsacceD0y72LGqI6DqsxLA5j49ZPOQ/ZEDSYTBXMzwHLBK2RpkpJUeQFyotFI8aBqDh70oL28fkpdC57LqkCwpuT3vYLefZ812mh6fBMzU8XOEFxUjar8lhqJ8BzVGHV2qjMBAwszxwHEDs72ENe09V5KknoHflSXbt7LtpONQP9HI+qRwnR//GbaaGhiePMkyGnlFO2Pci5jTnHeeU1khOHQAbOoNJt7ZE2ZEpRDP80ufYFZfWmHjBgD/mf2BcavcO+UzzhMDeh/Pnlm51sxlj2+58ec0dIG0hng474UZMUzFrWX6A24eGEAo7xnjKv1/FADPgAFUuv6PQFuTtDdqPcjJDTqlgDpyAlJ2RAevvNVWCk5bXBvAhlBMIIBppqEXOCRhyAmsyYYDHqBgxdGppJhjINnwJXfgCXw4FpKqMQIIAot+ONgNgx8gAiz5Egp4gTKOxmQix0Cy+UvuwA+EcCwks6IEIAG9rY1/RdQ+0Ap48nRQDZpA458guZswKgYgoAY0g5YFnfsB6EAIokCM+DQO8x+ovAowgBpQi9fTACpBswj+6uwtf91gR1vE/0l0ANS747O7fC2oObAXvPKuOT4V81Vg7iS0EfBshpzWfsbRYAo=)format("woff2");font-weight:normal;font-style:normal}[class^="icn-"]{font-family:"unicon"!important;font-style:normal;font-weight:normal;font-variant:normal;text-transform:none;-webkit-font-smoothing:antialiased;-moz-osx-font-smoothing:grayscale}.icn-location_pin:before{content:""}.field .field-widget .addbutton .btn:hover,.field .BodyText .addbutton .btn:hover,.field .field-widget .scrollable_container .scrollable_toolbox .scrollable_selectall:hover,.field .field-widget .scrollable_container .scrollable_toolbox .scrollable_clearall:hover,.field .field-widget .scrollable_container .selection_header .scrollable_container_clear:hover,.uib-datepicker-popup .uib-button-bar button:hover,.btn:hover,input[type="button"]:hover,input[type="submit"]:hover,input[type="reset"]:hover,.lst-head .batch-opts ul a:hover,.lst-foot .batch-opts ul a:hover,.lst-head .lst-batch>.ListToolMenuLink:hover,.cspList_tophead .batch-opts ul a:hover,.cspList_foot .batch-opts ul a:hover,.modal-dialog .modal-content .modal-body .input-button:hover,.field .field-widget .addbutton .btn:focus,.field .BodyText .addbutton .btn:focus,.field .field-widget .scrollable_container .scrollable_toolbox .scrollable_selectall:focus,.field .field-widget .scrollable_container .scrollable_toolbox .scrollable_clearall:focus,.field .field-widget .scrollable_container .selection_header .scrollable_container_clear:focus,.uib-datepicker-popup .uib-button-bar button:focus,.btn:focus,input[type="button"]:focus,input[type="submit"]:focus,input[type="reset"]:focus,.lst-head .batch-opts ul a:focus,.lst-foot .batch-opts ul a:focus,.lst-head .lst-batch>.ListToolMenuLink:focus,.cspList_tophead .batch-opts ul a:focus,.cspList_foot .batch-opts ul a:focus,.modal-dialog .modal-content .modal-body .input-button:focus,.field .field-widget .addbutton .btn:active,.field .BodyText .addbutton .btn:active,.field .field-widget .scrollable_container .scrollable_toolbox .scrollable_selectall:active,.field .field-widget .scrollable_container .scrollable_toolbox .scrollable_clearall:active,.field .field-widget .scrollable_container .selection_header .scrollable_container_clear:active,.uib-datepicker-popup .uib-button-bar button:active,.btn:active,input[type="button"]:active,input[type="submit"]:active,input[type="reset"]:active,.lst-head .batch-opts ul a:active,.lst-foot .batch-opts ul a:active,.lst-head .lst-batch>.ListToolMenuLink:active,.cspList_tophead .batch-opts ul a:active,.cspList_foot .batch-opts ul a:active,.modal-dialog .modal-content .modal-body .input-button:active{box-shadow:none;text-decoration:none}html,body,div,span,iframe,h1,h3,p,a,img,strong,i,ul,li,form,label,aside,footer,header,nav{margin:0;padding:0;border:0;font-size:100%;font-family:inherit;vertical-align:baseline}html{-ms-text-size-adjust:100%;-webkit-text-size-adjust:100%}aside,footer,header,main,nav{display:block}a{background-color:transparent}img{border:0}hr{-moz-box-sizing:content-box;box-sizing:content-box;height:0}button,input{color:inherit;font:inherit;margin:0}button{overflow:visible}button{text-transform:none}button{-webkit-appearance:button;cursor:pointer}input[type="search"]{-webkit-appearance:textfield;-moz-box-sizing:content-box;-webkit-box-sizing:content-box}input[type="search"]::-webkit-search-cancel-button,input[type="search"]::-webkit-search-decoration{-webkit-appearance:none}ul{list-style:none}*,*:before,*:after{-webkit-box-sizing:border-box;-moz-box-sizing:border-box;box-sizing:border-box}.sr-only{background-color:#FFFFFF;color:#000000}.sr-only-focusable:active,.sr-only-focusable:focus{position:static;width:auto;height:auto;margin:0;overflow:visible;clip:auto}.container{margin-left:auto;margin-right:auto;padding-left:15px;padding-right:15px}.row{margin-left:-15px;margin-right:-15px}.row:before,.row:after{content:" ";display:table}.row:after{clear:both}.col{float:left;padding-left:15px;padding-right:15px}.col-12{width:100%}@media only screen and (max-width:767px){.container{max-width:767px}.col-12{width:100%}}@media only screen and (min-width:768px){.container{max-width:720px}.sm-col-8{width:calc(8/12*100%)}}@media only screen and (min-width:992px){.container{max-width:960px}}@media only screen and (min-width:1200px){.container{max-width:1140px}}html,body{-webkit-font-smoothing:antialiased;-moz-osx-font-smoothing:grayscale}html body{font-family:"Roboto",Helvetica,Arial,sans-serif;font-weight:400;color:#333;font-size:14px;line-height:22px;text-align:unset}@media only screen and (max-width:767px){html body{line-height:18px}}h1{font-weight:300;font-size:28px;line-height:36px}@media only screen and (max-width:767px){h1{font-size:24px;line-height:30px}}@media only screen and (max-width:767px){}h3{font-weight:400;font-size:18px;line-height:24px}@media only screen and (max-width:767px){h3{font-size:16px;line-height:20px}}p{margin-bottom:10px}a{color:#1E3765;text-decoration:none;font-size:13px;line-height:20px}a:hover,a:hover [class^="icn-"],a:hover [class*=" icn-"],a:focus,a:focus [class^="icn-"],a:focus [class*=" icn-"]{color:#1E3765}a:hover,a:focus{text-decoration:underline;cursor:pointer}strong{font-weight:700}.body-small{font-size:13px;line-height:20px}@media only screen and (max-width:767px){.body-small{line-height:16px}}body .branding-bg{background-color:#1E3765}@keyframes spin{from{transform:rotate(0deg)}to{transform:rotate(360deg)}}html{height:100%;background-color:#f6f6f6!important;scroll-behavior:smooth;--symp-screen-mobile:415px;--symp-screen-sm:768px;--symp-screen-md:992px;--symp-screen-lg:1200px}html body{min-height:calc(100% - 64px);height:auto;position:initial;background-color:#f6f6f6;margin-top:64px;min-width:0}header .container{display:"-webkit-flex";display:"-moz-flex";display:"-ms-flex";display:flex;-webkit-flex-direction:row;-moz-flex-direction:row;-ms-flex-direction:row;flex-direction:row;-webkit-align-items:center;-moz-align-items:center;-ms-align-items:center;align-items:center}@media only screen and (max-width:992px){header .container{width:100%}}footer{padding:0 15px 15px 15px}@media only screen and (min-width:768px){.container{width:720px}}@media only screen and (min-width:992px){.container{width:960px}}@media only screen and (min-width:1200px){.container{width:1140px}}.content-container-inner .header-content{background:#fff;border:1px solid #ececec;border-radius:5px;padding:20px;margin-bottom:15px}.content-container-inner .header-content .header-text{-webkit-flex:1;-moz-flex:1;-ms-flex:1;flex:1;min-width:0px;width:100%}.content-container-inner .header-content .header-title{display:"-webkit-flex";display:"-moz-flex";display:"-ms-flex";display:flex;-webkit-align-items:flex-end;-moz-align-items:flex-end;-ms-align-items:flex-end;align-items:flex-end;-webkit-justify-content:space-between;-moz-justify-content:space-between;-ms-justify-content:space-between;justify-content:space-between;margin-bottom:5px}.content-container-inner .header-content .header-subtitle{display:"-webkit-flex";display:"-moz-flex";display:"-ms-flex";display:flex;-webkit-justify-content:space-between;-moz-justify-content:space-between;-ms-justify-content:space-between;justify-content:space-between;-webkit-align-items:center;-moz-align-items:center;-ms-align-items:center;align-items:center;color:#6c6c6c}.content-container-inner .header-content .header-subtitle .header-subtitle-content{width:100%;overflow:hidden;margin-right:10px;-webkit-flex:1 0 0;-moz-flex:1 0 0;-ms-flex:1 0 0;flex:1 0 0}.content-container-inner .header-content .header-subtitle .header-subtitle-content .header-info>span{margin:5px 0px}@media only screen and (max-width:767px){.content-container-inner .header-content .header-subtitle .header-subtitle-content .header-info{margin-top:5px}.content-container-inner .header-content .header-subtitle .header-subtitle-content .header-info>span{margin:2.5px 0px}}@media only screen and (max-width:767px){.content-container-inner .header-content .header-subtitle{display:block}.content-container-inner .header-content .header-subtitle .header-subtitle-content{margin-bottom:10px}}.page-utils{position:relative;margin-top:15px}.page-utils .back-links{font-size:13px;color:#6c6c6c;line-height:20px}.page-utils .back-links a{color:#6c6c6c;display:inline-block;text-decoration:none}@media only screen and (max-width:767px){.page-utils .back-links a{line-height:inherit}}.page-utils .back-links .bc-sep{color:#444}.page-utils .back-links ul li{display:inline}.page-utils .back-links ul li:hover a{color:#1E3765}.page-utils .back-links ul li:last-child a,.page-utils .back-links ul li:last-child a:hover{color:#333;pointer-events:none}@media only screen and (max-width:767px){.page-utils .back-links ul li{line-height:16px;height:16px}}@media only screen and (max-width:767px){}.page-footer{width:100%;display:"-webkit-flex";display:"-moz-flex";display:"-ms-flex";display:flex;-webkit-align-content:center;-moz-align-content:center;-ms-align-content:center;align-content:center;-webkit-justify-content:space-between;-moz-justify-content:space-between;-ms-justify-content:space-between;justify-content:space-between}.page-footer div{-webkit-align-self:center;-moz-align-self:center;-ms-align-self:center;align-self:center}.page-footer .footer-links{text-align:right;font-size:12px}@media only screen and (max-width:767px){.page-footer{-webkit-flex-wrap:wrap;-moz-flex-wrap:wrap;-ms-flex-wrap:wrap;flex-wrap:wrap}.page-footer div,.page-footer .footer-image,.page-footer .footer-text,.page-footer .footer-links{width:100%;text-align:center}}.footer-image a{color:#6c6c6c;text-decoration:none;font-size:0.92em}.form-container{display:"-webkit-flex";display:"-moz-flex";display:"-ms-flex";display:flex;width:100%}.form-container:before,.form-container:after,.wizard-container:before,.wizard-container:after{content:" ";display:table}.form-container:after,.wizard-container:after{clear:both}:not(.has-sidebar)>.form-container{-webkit-flex-direction:column;-moz-flex-direction:column;-ms-flex-direction:column;flex-direction:column}.form-col{float:none;vertical-align:top}.form-col .form{padding:0}.floating_content .form-col{background:#fff;border:1px solid #ececec;border-radius:5px}@media only screen and (max-width:767px){}@media only screen and (max-width:767px){}@media only screen and (max-width:767px){}.has-sidebar .form-col{width:calc(70% - 10px);-webkit-flex-shrink:0;-moz-flex-shrink:0;-ms-flex-shrink:0;flex-shrink:0}@media only screen and (min-width:1200px){}.sidebar.sidebar-right{border-bottom-right-radius:3px}@media only screen and (max-width:991px){:not(.non-responsive-section) .page-title-wrapper{padding:0 15px}:not(.non-responsive-section) .page-main{margin:0;padding:0 15px 15px}:not(.non-responsive-section) .form-layout .form-container,:not(.non-responsive-section) .form-layout .form-col,:not(.non-responsive-section) .form-layout .sidebar{display:block;width:auto}:not(.non-responsive-section) .floating_content .sidebar,:not(.non-responsive-section) .profile-view .sidebar{margin:15px 0}}@media only screen and (max-width:767px){:not(.non-responsive-section) .page-title-wrapper{padding:0 15px}:not(.non-responsive-section) .form-layout .sidebar{margin:15px 0 0}:not(.non-responsive-section) .form-col{padding:15px}}.header-menu{display:flex;flex-direction:row;-webkit-align-items:center;-moz-align-items:center;-ms-align-items:center;align-items:center;padding-right:10px}@media only screen and (max-width:991px){.header-menu{padding-left:0}}@media only screen and (max-width:767px){.header-menu{padding-left:0;width:150px}}@media only screen and (max-width:767px){}@media only screen and (min-width:1200px){}@media only screen and (max-width:767px){}@media only screen and (max-width:415px){}@media only screen and (max-width:767px){}@media only screen and (max-width:767px){}@media only screen and (max-width:767px){}@media only screen and (max-width:991px){}@media only screen and (max-width:767px){}@media only screen and (max-width:991px){}@media only screen and (max-width:767px){}@media only screen and (max-width:767px){}.skip-links a{top:-80px}.skip-links a:focus{position:absolute;top:0;left:0;right:0;width:100%;display:block;padding:20px;font-size:16px;background:#ececec;color:#000;z-index:110;box-shadow:0 8px 10px -5px rgba(0,0,0,0.14),0 16px 24px 2px rgba(0,0,0,0.098),0 6px 30px 5px rgba(0,0,0,0.082);text-align:center}.container{margin:0 auto;padding:0}.container #frame{padding:0}.container .row{margin:0 auto}.container .row:first-child{margin:0}.page-title-wrapper{margin-bottom:20px;padding:0 15px}.page-main{padding:0 15px 15px}@media only screen and (max-width:991px){}@media only screen and (max-width:991px){}@media (min-width:992px){}.topnav{position:fixed;top:0;width:100%;z-index:102;transition:all 0.33s ease-in}.topnav .container{width:auto;max-width:1140px!important}.topnav .navbar-wrapper{position:relative;background-color:#1E3765;height:64px}@media only screen and (max-width:767px){}@media only screen and (max-width:767px){}@media only screen and (max-width:991px){}@media only screen and (max-width:767px){}@media only screen and (min-width:768px) and (max-width:991px){}@media only screen and (max-width:767px){}.topnav .header-menu{width:auto;padding-left:0px}.topnav .header-menu #page-brand{background:#1E3765;height:64px}.topnav .header-menu #page-brand .nav-brand{display:"-webkit-flex";display:"-moz-flex";display:"-ms-flex";display:flex;width:100%;height:100%;padding-left:15px;background:#1E3765;transition:none}.topnav .header-menu #page-brand .nav-brand img{max-width:120px;object-fit:contain;object-position:center}.topnav .topnav-search #page-search{-webkit-flex-grow:1;-moz-flex-grow:1;-ms-flex-grow:1;flex-grow:1;white-space:nowrap;margin-right:0}.topnav nav{padding-right:20px;padding-left:30px;margin-left:auto}.topnav nav ul{display:"-webkit-flex";display:"-moz-flex";display:"-ms-flex";display:flex;gap:20px;height:64px;line-height:64px}.topnav nav ul li{height:100%;color:white}.topnav nav ul li a{color:inherit;max-width:120px;white-space:nowrap;font-size:14px;text-decoration:none}.topnav nav ul li a.activated,.topnav nav ul li a:hover{padding-bottom:19px;border-bottom:5px solid white}.topnav #user-avatar{width:30px;height:30px;display:"-webkit-flex";display:"-moz-flex";display:"-ms-flex";display:flex;-webkit-flex-shrink:1;-moz-flex-shrink:1;-ms-flex-shrink:1;flex-shrink:1;box-sizing:border-box;position:relative;margin-right:15px}@media only screen and (min-width:767px){}.topnav #user-avatar .user-tools-container{position:relative}.topnav #user-avatar .user-tools-container .badge-toggle{display:"-webkit-flex";display:"-moz-flex";display:"-ms-flex";display:flex;-webkit-align-items:center;-moz-align-items:center;-ms-align-items:center;width:30px;height:30px;line-height:30px;border:0px;align-items:center;color:#949494;text-decoration:none;margin:1px;overflow:hidden;padding:0px}.topnav #user-avatar .user-tools-container .badge-toggle .avatar{font-size:13px;font-weight:500}.topnav #user-avatar .user-tools-container .badge-toggle:hover,.topnav #user-avatar .user-tools-container .badge-toggle:focus{cursor:pointer}.topnav #user-avatar .user-tools-container .badge-toggle:hover .avatar,.topnav #user-avatar .user-tools-container .badge-toggle:focus .avatar{color:#1E3765}@media only screen and (max-width:767px){}@media only screen and (max-width:767px){}@media only screen and (max-width:767px){}@media screen and (max-width:767px){}#frame.no-nav{margin-left:0}@media only screen and (max-width:991px){#frame{margin-left:0}}@media only screen and (max-width:992px){}@media only screen and (min-width:992px){}@media only screen and (max-width:991px){}@media only screen and (min-width:992px){}.breadcrumbs{white-space:nowrap;text-overflow:ellipsis;overflow:hidden}.breadcrumbs li{color:#6c6c6c}.breadcrumbs li:last-child{color:#333}.breadcrumbs a,.breadcrumbs .current_path{display:inline-block;white-space:nowrap;text-overflow:ellipsis;overflow:hidden;max-width:180px;vertical-align:bottom}@media only screen and (max-width:991px){}@media only screen and (max-width:767px){}@media only screen and (max-width:767px){}.buttonbar-top{padding-bottom:15px;display:"-webkit-flex";display:"-moz-flex";display:"-ms-flex";display:flex;-webkit-flex-wrap:wrap;-moz-flex-wrap:wrap;-ms-flex-wrap:wrap;flex-wrap:wrap}.buttonbar-top:empty,.buttonbar_top:empty{padding:0}.buttonbar-bottom{padding-top:15px;display:"-webkit-flex";display:"-moz-flex";display:"-ms-flex";display:flex;-webkit-flex-wrap:wrap;-moz-flex-wrap:wrap;-ms-flex-wrap:wrap;flex-wrap:wrap}.buttonbar-bottom:empty,.buttonbar_bottom:empty{padding:0}.form-readonly .field-label{line-height:22px}.form .widget-readonly{font-size:14px;line-height:22px;color:#6c6c6c}.fieldgroup{margin:0-20px;padding:20px}.fieldgroup:not(:last-child){border-bottom:1px solid #ececec}@media only screen and (max-width:767px){.fieldgroup{margin:0-15px;padding:15px}}.field{margin-bottom:20px;max-width:500px;width:100%}.field:last-child{margin-bottom:0}.field .field-label{display:inline-block;color:#333;margin:5px 0;font-size:14px;font-weight:400}.field .field-widget:not(.field-widget-daterange),.field .BodyText:not(.field-widget-daterange){max-width:500px}.field .field-widget p:last-child,.field .BodyText p:last-child{margin-bottom:0px}@media only screen and (max-width:767px){}@media only screen and (max-width:767px){}.chip{border-radius:50px;border:1px solid #ececec;background:none;text-align:center;line-height:28px;font-weight:normal;white-space:nowrap;overflow:hidden;text-overflow:ellipsis;display:"-webkit-inline-flex";display:"-moz-inline-flex";display:"-ms-inline-flex";display:inline-flex;-webkit-flex-direction:row;-moz-flex-direction:row;-ms-flex-direction:row;flex-direction:row;-webkit-align-items:center;-moz-align-items:center;-ms-align-items:center;align-items:center;margin-right:10px;vertical-align:middle}.chip:not(.search-form-filters-chip):hover,.chip:not(.search-form-filters-chip):focus{background-color:none;color:#333;border-color:#333}.chip.chip-disabled,.chip.chip-disabled:hover{background-color:#ececec;color:#6c6c6c;border-color:#ececec;cursor:default}.chip.chip-sm{height:24px;font-size:13px;padding:0 12px;margin-bottom:10px}.chip .chip-value{white-space:nowrap;overflow:hidden;text-overflow:ellipsis}@keyframes chip-highlight{0%{color:#1E3765}75%{color:#1E3765}100%{color:inherit}}@media (-webkit-min-device-pixel-ratio:0){}@media only screen and (max-width:991px){}@media only screen and (max-width:991px){}@media only screen and (max-width:767px){}@media only screen and (max-width:767px){}@media only screen and (max-width:767px){}@media only screen and (max-width:767px){}@media only screen and (max-width:767px){}@media only screen and (min-width:767px){}.field-widget-tinymce ul{list-style-type:disc}.field-widget-tinymce ul{padding-left:30px;margin:10px 0}@media (max-width:414px){}@media (-webkit-min-device-pixel-ratio:0){}@media only screen and (max-width:414px){}@media screen and (max-width:767px){}@media only screen and (min-width:1200px){}@media only screen and (max-width:767px){}@keyframes loading-spin{0%{-webkit-transform:rotate(0deg);-moz-transform:rotate(0deg);-ms-transform:rotate(0deg);-o-transform:rotate(0deg);transform:rotate(0deg)}100%{-webkit-transform:rotate(360deg);-moz-transform:rotate(360deg);-ms-transform:rotate(360deg);-o-transform:rotate(360deg);transform:rotate(360deg)}}input::-webkit-calendar-picker-indicator{display:none}@media only screen and (max-width:767px){}@media only screen and (max-width:767px){}@keyframes rotate{100%{-webkit-transform:rotate(360deg);-moz-transform:rotate(360deg);-ms-transform:rotate(360deg);-o-transform:rotate(360deg);transform:rotate(360deg)}}@keyframes dash{0%{stroke-dasharray:var(--stroke-dasharray-0);stroke-dashoffset:var(--stroke-dashoffset-0)}50%{stroke-dasharray:var(--stroke-dasharray-50);stroke-dashoffset:var(--stroke-dashoffset-50)}100%{stroke-dasharray:var(--stroke-dasharray-100);stroke-dashoffset:var(--stroke-dashoffset-100)}}.sidebar{margin:0;margin-left:10px}@media only screen and (max-width:768px){}@media only screen and (max-width:768px){}.floating_content .sidebar{background:none}.floating_content .sidebar .sidebar-content{background:#fff;border:1px solid #ececec;border-radius:5px;padding:20px}.floating_content .sidebar .sidebar-content .sidebar-inner{padding:0;margin-bottom:20px;border-bottom:1px solid #ececec}.floating_content .sidebar .sidebar-content .sidebar-inner:last-child{border-bottom:0;margin-bottom:0}.sidebar-inner .sidebar-header .sidebar-title{font-weight:500;line-height:20px;color:#333;margin-bottom:20px;padding:0}.sidebar-inner .sidebar-body{word-wrap:break-word}.btn{background-color:#fff}.btn:hover,.btn:focus,.btn:active,input[type="button"]:hover,input[type="button"]:focus,input[type="button"]:active,input[type="submit"]:hover,input[type="submit"]:focus,input[type="submit"]:active,input[type="reset"]:hover,input[type="reset"]:focus,input[type="reset"]:active{color:#333;border-color:#949494}.btn:active,input[type="button"]:active,input[type="submit"]:active,input[type="reset"]:active{background-color:#f6f6f6}.btn.btn_primary{color:#fff;background-color:#1E3765;background-image:none;border-color:#1E3765}.btn.btn_primary:hover,.btn.btn_primary:focus,.btn.btn_primary:active,.btn.btn_go:hover,.btn.btn_go:focus,.btn.btn_go:active,input[type="button"].btn_primary:hover,input[type="button"].btn_primary:focus,input[type="button"].btn_primary:active,input[type="button"].btn_go:hover,input[type="button"].btn_go:focus,input[type="button"].btn_go:active,input[type="submit"].btn_primary:hover,input[type="submit"].btn_primary:focus,input[type="submit"].btn_primary:active,input[type="submit"].btn_go:hover,input[type="submit"].btn_go:focus,input[type="submit"].btn_go:active,input[type="reset"].btn_primary:hover,input[type="reset"].btn_primary:focus,input[type="reset"].btn_primary:active,input[type="reset"].btn_go:hover,input[type="reset"].btn_go:focus,input[type="reset"].btn_go:active{color:#fff}.btn.btn_primary:hover,.btn.btn_primary:focus,.btn.btn_go:hover,.btn.btn_go:focus,input[type="button"].btn_primary:hover,input[type="button"].btn_primary:focus,input[type="button"].btn_go:hover,input[type="button"].btn_go:focus,input[type="submit"].btn_primary:hover,input[type="submit"].btn_primary:focus,input[type="submit"].btn_go:hover,input[type="submit"].btn_go:focus,input[type="reset"].btn_primary:hover,input[type="reset"].btn_primary:focus,input[type="reset"].btn_go:hover,input[type="reset"].btn_go:focus{background-color:#182c51;border-color:#182c51}.btn.btn_primary:active,.btn.btn_go:active,input[type="button"].btn_primary:active,input[type="button"].btn_go:active,input[type="submit"].btn_primary:active,input[type="submit"].btn_go:active,input[type="reset"].btn_primary:active,input[type="reset"].btn_go:active{background-color:#12223e;border-color:#12223e}.btn.btn_alt-default{color:#1E3765;border-color:rgba(30,55,101,0.6);background:#fff}.btn.btn_alt-default:hover,.btn.btn_alt-default:focus,.btn.btn_alt-default:active,input[type="button"].btn_alt-default:hover,input[type="button"].btn_alt-default:focus,input[type="button"].btn_alt-default:active,input[type="submit"].btn_alt-default:hover,input[type="submit"].btn_alt-default:focus,input[type="submit"].btn_alt-default:active,input[type="reset"].btn_alt-default:hover,input[type="reset"].btn_alt-default:focus,input[type="reset"].btn_alt-default:active{color:#1E3765;border-color:#1E3765}.btn.btn_alt-default:active,input[type="button"].btn_alt-default:active,input[type="submit"].btn_alt-default:active,input[type="reset"].btn_alt-default:active{background-color:rgba(30,55,101,0.05)}@media only screen and (max-width:414px){}@media only screen and (max-width:414px){}.btn.dropdown-toggle:focus,.btn:focus,input[type="button"].dropdown-toggle:focus,input[type="button"]:focus,input[type="submit"].dropdown-toggle:focus,input[type="submit"]:focus,input[type="reset"].dropdown-toggle:focus,input[type="reset"]:focus{outline:auto}.btn>span{vertical-align:middle}.banner-msg-list{position:sticky;top:64px;z-index:10}.banner-msg-list>div:not(:first-child){border-top:5px solid #f6f6f6}@media only screen and (max-width:767px){}@media only screen and (max-width:767px){}@media (min-width:768px){}@media only screen and (max-width:767px){}@media (min-width:768px){}@media (min-width:768px){}@media (min-width:768px){}@media (min-width:768px){}@media (min-width:768px){}@media (min-width:768px){}@media (max-width:767px){}@media screen and (max-width:992px){}@media screen and (max-width:415px){}@media only screen and (min-width:768px){}@media only screen and (max-width:767px){}@media only screen and (min-width:768px){}@media only screen and (max-width:767px){}@media only screen and (max-width:767px){}@media only screen and (max-width:767px){}@media (min-width:768px){}@media only screen and (max-width:991px){}@media only screen and (max-width:767px){}@media (min-width:768px){}@media only screen and (max-width:767px){}@media only screen and (min-width:768px){}@media only screen and (max-width:414px){}@media (min-width:768px){}@media only screen and (max-width:767px){}@media only screen and (max-width:767px){}@media (min-width:992px){}@media (min-width:992px){}@media (min-width:768px){}@media only screen and (max-width:767px){}@media (max-width:414px){}@media (max-width:414px){}@media (max-width:414px){}@media only screen and (max-width:767px){}@media only screen and (max-width:767px){}@media (min-width:415px){}@media (min-width:415px){}@media only screen and (max-width:767px){}@media (max-width:414px){}@media (min-width:768px){}@media (max-width:767px){}@media (max-width:767px){}@media (min-width:992px){}@media (min-width:768px){}@media only screen and (max-width:767px){}@media (min-width:768px){}@media only screen and (max-width:991px){}@media only screen and (max-width:767px){}@media (min-width:768px){}.floating_content{margin:0;background:none}@media (min-width:992px){}@media (min-width:992px){}@media (max-width:1200px){}@media (min-width:992px){}@media (min-width:992px){}@media (min-width:992px){}@media (min-width:992px){}@media (min-width:992px){}@media only screen and (max-width:767px){}@media only screen and (min-width:992px) and (max-width:1199px){}@media only screen and (max-width:767px){}@media only screen and (min-width:992px) and (max-width:1199px){}@media (max-width:768px){}@media (max-width:768px){}@media only screen and (max-width:414px){}@media screen and (min-width:767px){}@media screen and (max-width:768px){}@media screen and (max-width:767px){}@media screen and (max-width:767px){.hide-sm{display:none!important}}@media screen and (max-width:991px){}.hide{display:none!important}.block{display:block}.text-center{text-align:center}.text-capitalize{text-transform:capitalize}@media only screen and (max-width:767px){}.text-truncate{white-space:nowrap;text-overflow:ellipsis;overflow:hidden}.text-truncate-3{display:-webkit-box;-webkit-line-clamp:3;-webkit-box-orient:vertical;overflow:hidden}.space-bottom-xs{margin-bottom:5px}.space-left-xs{margin-left:5px}.space-right-sm{margin-right:10px}.space-bottom-sm{margin-bottom:10px}.space-bottom-md{margin-bottom:15px}.space-right-xs{margin-right:5px}.space-top-lg{margin-top:20px}.space-right-lg{margin-right:20px}.space-left-lg{margin-left:20px}.margin-lg{margin:20px}@media only screen and (min-width:768px){}.padding-bottom-sm{padding-bottom:10px!important}.neg-margin-x-lg{margin-left:-20px;margin-right:-20px}.no-margin{margin:0!important}.full_width{width:100%}.flexbox{display:"-webkit-flex";display:"-moz-flex";display:"-ms-flex";display:flex}.flex-shrink-0{-webkit-flex-shrink:0;-moz-flex-shrink:0;-ms-flex-shrink:0;flex-shrink:0}.align-self-end{-webkit-align-self:flex-end;-moz-align-self:flex-end;-ms-align-self:flex-end;align-self:flex-end}@keyframes ball-clip-rotate{0%{-webkit-transform:rotate(0deg);-moz-transform:rotate(0deg);-ms-transform:rotate(0deg);-o-transform:rotate(0deg);transform:rotate(0deg);border-color:#1E3765 #1E3765 transparent #1E3765}50%{-webkit-transform:rotate(180deg);-moz-transform:rotate(180deg);-ms-transform:rotate(180deg);-o-transform:rotate(180deg);transform:rotate(180deg);border-color:#6D247A #6D247A transparent #6D247A}100%{-webkit-transform:rotate(360deg);-moz-transform:rotate(360deg);-ms-transform:rotate(360deg);-o-transform:rotate(360deg);transform:rotate(360deg);border-color:#1E3765 #1E3765 transparent #1E3765}}.secondary-text{color:#6c6c6c!important}.icon-size-sm{font-size:16px!important}.text-overflow{text-overflow:ellipsis;overflow:hidden;word-wrap:break-word}.text-base{color:#333!important}.text-gray{color:#6c6c6c}.no-padding{padding:0!important}@media screen and (max-width:767px){}@media screen and (max-width:991px){}@media (max-width:414px){}@media (min-width:415px) and (max-width:767px){}@media (min-width:768px) and (max-width:991px){}@media (min-width:992px) and (max-width:1199px){}@media (min-width:1200px){.display-desktop-block{display:block!important}}.line-height-sm{line-height:20px!important}.background-lighter-gray{background-color:#ececec}.p-group p:last-child{margin-bottom:0px}.button-group button:last-child{margin-right:0px}.text-and-button{display:"-webkit-flex";display:"-moz-flex";display:"-ms-flex";display:flex;-webkit-align-items:flex-start;-moz-align-items:flex-start;-ms-align-items:flex-start;align-items:flex-start}.text-and-button .btn{-webkit-flex-basis:fit-content;-moz-flex-basis:fit-content;-ms-flex-basis:fit-content;flex-basis:fit-content;min-width:auto}.text-and-button button:first-child,.text-and-button .btn:first-child{margin-right:auto;margin-left:0}.text-and-button button:last-child,.text-and-button .btn:last-child{margin-right:0;margin-left:auto}.monogram{font-family:"Roboto",Helvetica,Arial,sans-serif;font-weight:400;font-size:16px;color:#555;background-color:#ececec}.avatar{overflow:hidden;height:40px;width:40px;line-height:40px;text-align:center;display:inline-block;border-radius:40px}.avatar.avatar-md.avatar-square{height:60px;width:60px;line-height:60px;text-align:center;display:inline-block;border-radius:3px}.avatar.avatar-square:not(.monogram):not(a){display:"-webkit-inline-flex";display:"-moz-inline-flex";display:"-ms-inline-flex";display:inline-flex;-webkit-justify-content:center;-moz-justify-content:center;-ms-justify-content:center;justify-content:center;-webkit-align-items:center;-moz-align-items:center;-ms-align-items:center;align-items:center;border:1px solid #ececec}.avatar img{max-width:100%;max-height:100%;width:100%;height:100%;vertical-align:middle;text-align:center;margin:auto;object-fit:contain}.avatar a{display:inline-block;width:100%;height:100%;line-height:inherit}.avatar a *{display:inline-block}.avatar:not(.monogram):not(a){background-color:white}@media screen and (min-width:768px){}@media (max-width:414px){}@media screen and (max-width:767px){}@media (max-width:1199px){}@media only screen and (max-width:767px){}@media only screen and (max-width:767px){}@media only screen and (max-width:767px){}@media only screen and (max-width:767px){}@media only screen and (max-width:767px){}@media only screen and (max-width:767px){}@media (max-width:767px){}@media only screen and (max-width:767px){}@media (max-width:767px){}@media screen and (max-width:767px){}@media screen and (max-width:767px){}@media only screen and (max-width:767px){}@media only screen and (max-width:767px){}@media only screen and (max-width:767px){}@media (max-width:767px){}@media only screen and (max-width:767px){}@media only screen and (max-width:767px){}@media only screen and (max-width:767px){}@media only screen and (max-width:767px){}.hide{display:none}@media only screen and (max-width:768px){}@media only screen and (max-width:768px) and (orientation:landscape){}@media only screen and (min-width:768px){}@media only screen and (max-width:767px){}@media only screen and (min-width:768px){}@media only screen and (min-width:768px){}@media only screen and (min-width:768px){}@media only screen and (max-width:767px){}html{background-repeat:no-repeat;background-position:center center;background-attachment:fixed;background-size:cover}@media only screen and (max-width:767px){}@media only screen and (max-width:991px){}@media only screen and (max-width:991px){}@media only screen and (max-width:767px){}@media only screen and (max-width:991px){}@media only screen and (max-width:767px){}@media only screen and (max-width:767px){}@media only screen and (min-width:1200px){}@media only screen and (max-width:991px){}@media only screen and (max-device-width:991px){}@media only screen and (max-width:767px){}@media only screen and (max-width:767px){}@media only screen and (max-width:767px){}@media screen and (max-width:767px){}@media screen and (max-width:767px){}@media only screen and (max-width:767px){}@media only screen and (max-width:767px){}@media only screen and (max-width:767px){}@media only screen and (max-width:767px){}@media only screen and (max-width:767px){}@media only screen and (max-width:767px){}@media only screen and (max-width:767px){}@keyframes loading-spin{0%{-webkit-transform:rotate(0deg);-moz-transform:rotate(0deg);-ms-transform:rotate(0deg);-o-transform:rotate(0deg);transform:rotate(0deg)}100%{-webkit-transform:rotate(360deg);-moz-transform:rotate(360deg);-ms-transform:rotate(360deg);-o-transform:rotate(360deg);transform:rotate(360deg)}}@media only screen and (min-width:415px){}@media only screen and (min-width:992px){}@media only screen and (max-width:992px){}@media only screen and (max-width:992px){}@media only screen and (max-width:992px){}@media only screen and (min-width:415px){}@media only screen and (min-width:992px){}@media only screen and (min-width:992px){}@media only screen and (min-width:992px){}@media only screen and (max-width:992px){}@media only screen and (max-width:992px){}@media only screen and (min-width:415px){}@media only screen and (max-width:767px){}@media only screen and (max-width:767px){}@media only screen and (max-width:767px){}@media only screen and (max-width:799px){}@media (max-width:500px){}@media only screen and (max-width:415px){}@media only screen and (min-device-width:320px) and (max-device-width:480px) and (-webkit-min-device-pixel-ratio:2) and (orientation:portrait){}@media only screen and (min-device-width:320px) and (max-device-width:568px) and (-webkit-min-device-pixel-ratio:2) and (orientation:portrait){}@media only screen and (min-device-width:375px) and (max-device-width:667px) and (-webkit-min-device-pixel-ratio:2){}@media only screen and (max-width:415px){}@media only screen and (max-width:767px){}.btn{display:inline-block;min-height:30px;min-width:30px;padding:0 15px;text-align:center;margin-right:10px;border-width:1px;border-style:solid;font-size:13px;border-radius:2px;font-weight:500;text-decoration:none;text-transform:capitalize;cursor:pointer;white-space:nowrap;line-height:28px}.field .field-widget .addbutton .btn:hover,.field .BodyText .addbutton .btn:hover,.field .field-widget .scrollable_container .scrollable_toolbox .scrollable_selectall:hover,.field .field-widget .scrollable_container .scrollable_toolbox .scrollable_clearall:hover,.field .field-widget .scrollable_container .selection_header .scrollable_container_clear:hover,.uib-datepicker-popup .uib-button-bar button:hover,.btn:hover,input[type="button"]:hover,input[type="submit"]:hover,input[type="reset"]:hover,.lst-head .batch-opts ul a:hover,.lst-foot .batch-opts ul a:hover,.lst-head .lst-batch>.ListToolMenuLink:hover,.cspList_tophead .batch-opts ul a:hover,.cspList_foot .batch-opts ul a:hover,.modal-dialog .modal-content .modal-body .input-button:hover,.field .field-widget .addbutton .btn:focus,.field .BodyText .addbutton .btn:focus,.field .field-widget .scrollable_container .scrollable_toolbox .scrollable_selectall:focus,.field .field-widget .scrollable_container .scrollable_toolbox .scrollable_clearall:focus,.field .field-widget .scrollable_container .selection_header .scrollable_container_clear:focus,.uib-datepicker-popup .uib-button-bar button:focus,.btn:focus,input[type="button"]:focus,input[type="submit"]:focus,input[type="reset"]:focus,.lst-head .batch-opts ul a:focus,.lst-foot .batch-opts ul a:focus,.lst-head .lst-batch>.ListToolMenuLink:focus,.cspList_tophead .batch-opts ul a:focus,.cspList_foot .batch-opts ul a:focus,.modal-dialog .modal-content .modal-body .input-button:focus,.field .field-widget .addbutton .btn:active,.field .BodyText .addbutton .btn:active,.field .field-widget .scrollable_container .scrollable_toolbox .scrollable_selectall:active,.field .field-widget .scrollable_container .scrollable_toolbox .scrollable_clearall:active,.field .field-widget .scrollable_container .selection_header .scrollable_container_clear:active,.uib-datepicker-popup .uib-button-bar button:active,.btn:active,input[type="button"]:active,input[type="submit"]:active,input[type="reset"]:active,.lst-head .batch-opts ul a:active,.lst-foot .batch-opts ul a:active,.lst-head .lst-batch>.ListToolMenuLink:active,.cspList_tophead .batch-opts ul a:active,.cspList_foot .batch-opts ul a:active,.modal-dialog .modal-content .modal-body .input-button:active{box-shadow:none;text-decoration:none}@media only screen and (max-width:414px){}@media only screen and (min-width:415px){}@media only screen and (min-width:768px){}@media only screen and (min-width:992px){}#page-search{width:270px}#page-search .quicksearch .quicksearch-form{display:"-webkit-flex";display:"-moz-flex";display:"-ms-flex";-webkit-align-items:center;-moz-align-items:center;-ms-align-items:center;align-items:center;z-index:3;position:relative;height:30px;border-radius:3px}@media only screen and (max-width:767px){}@media only screen and (max-width:767px){}@media only screen and (max-width:767px){}#page-search .quicksearch .quicksearch-autocomplete{width:100%;margin-left:0;height:30px}#page-search .quicksearch .quicksearch-autocomplete input[type="search"]{font-size:14px;max-width:100%;height:30px;line-height:30px}@media only screen and (max-width:767px){#page-search .quicksearch .quicksearch-autocomplete input[type="search"]{padding-left:15px;background-color:#fff;color:#000}}#page-search .quicksearch md-autocomplete input[type="search"]::placeholder,#page-search .quicksearch .quicksearch-autocomplete input[type="search"]::placeholder{opacity:0}#page-search .quicksearch md-autocomplete input[type="search"]:focus,#page-search .quicksearch .quicksearch-autocomplete input[type="search"]:focus{background-color:#fff;color:#000}#page-search .quicksearch md-autocomplete:before,#page-search .quicksearch .quicksearch-autocomplete:before{font-family:"unicon";speak:none;color:#6c6c6c;font-size:18px;font-style:normal;font-weight:normal;font-variant:normal;text-transform:none;-webkit-font-smoothing:antialiased;-moz-osx-font-smoothing:grayscale;content:"";position:absolute;padding-left:15px;line-height:30px;z-index:100}@media only screen and (max-width:767px){#page-search .quicksearch md-autocomplete:before,#page-search .quicksearch .quicksearch-autocomplete:before{display:none}}@media only screen and (max-width:767px){#page-search .quicksearch .quicksearch-autocomplete{margin-right:15px}}@media only screen and (max-width:767px){#page-search .quicksearch .quicksearch-form{display:none;width:100%!important}}@media only screen and (max-width:767px){}@media only screen and (max-width:767px){}#page-search .ng-quicksearch .quicksearch-autocomplete{border-radius:3px}#page-search .ng-quicksearch #quicksearch-field{width:100%;border:0;box-sizing:border-box;outline:0}@media only screen and (max-width:767px){#page-search .ng-quicksearch #quicksearch-field{padding-left:15px;background-color:#fff;color:#000}}.topnav .topnav-search #page-search .quicksearch .quicksearch-form{max-width:400px;display:flex}.topnav .topnav-search #page-search .quicksearch .quicksearch-form .quicksearch-autocomplete{display:flex;margin-right:0px;border:none}.topnav .topnav-search #page-search .quicksearch .quicksearch-form .quicksearch-autocomplete input[type="search"]{border-radius:15px;padding-right:30px;padding-left:40px}.topnav .topnav-search #page-search .quicksearch .quicksearch-form .quicksearch-autocomplete input[type="search"]:focus{border:1px solid #ececec!important;border-bottom:none!important}@media only screen and (max-width:767px){.topnav .topnav-search #page-search .quicksearch .quicksearch-form .quicksearch-autocomplete:before{display:block}.topnav .topnav-search #page-search .quicksearch .quicksearch-form .quicksearch-autocomplete #quicksearch-field{padding-left:40px}}.topnav .topnav-search #page-search .quicksearch .quicksearch-form .quicksearch-autocomplete:before{padding-left:15px;font-size:16px}@media only screen and (max-width:767px){}@media only screen and (min-width:768px){}@media only screen and (min-width:768px){}@media only screen and (max-width:767px){}@media only screen and (max-width:991px){}@media only screen and (max-width:767px){}@media only screen and (max-width:767px){}@media only screen and (max-width:767px){}@media (max-width:767px){}@media (max-width:767px){}</style><style>@media only screen and (max-width:767px){}@media only screen and (max-width:767px){}@media only screen and (max-width:767px){}@media only screen and (max-width:767px){}@media only screen and (min-width:768px){}@media only screen and (max-width:767px){}@media only screen and (min-width:768px){}@media only screen and (max-width:767px){}@media only screen and (max-width:767px){}@media only screen and (max-width:767px){}@media only screen and (max-width:767px){}@media only screen and (max-width:767px){}@media only screen and (max-width:414px){}@media only screen and (max-width:767px){}@media only screen and (max-width:414px){}@media only screen and (max-width:767px){}@media only screen and (max-width:767px){}@media only screen and (max-width:767px){}@media only screen and (max-width:767px){}@media (-webkit-min-device-pixel-ratio:0){}@media only screen and (max-width:767px){}@media only screen and (max-width:767px){}@media only screen and (max-width:767px){}@media only screen and (max-width:991px){}@media only screen and (max-width:991px){}@media (max-height:600px){}@media (max-width:991px){}@media (max-width:768px){}@media (min-width:768px){}@media (min-width:768px){}@media (min-width:992px){}@media only screen and (max-width:767px){}@media (max-width:991px){}@media (max-width:991px){}@media (max-width:991px){}@media (min-width:768px){}@media (min-width:992px){}@media (max-width:991px){}@media (max-width:767px){}@media (max-width:415px){}@media (max-width:991px){}@media only screen and (max-width:767px){}@media (max-width:767px){}@media (max-width:767px){}@media only screen and (max-width:767px){}@media only screen and (max-width:767px){}@media (min-width:992px){}@media (max-width:767px){}@media only screen and (max-width:414px){}@media only screen and (max-width:767px){}.font-size-base{font-size:14px}@media (min-width:768px){}@media only screen and (min-width:992px){}@media screen and (max-width:767px){}@media screen and (max-width:991px){}@media screen and (max-width:991px){}@media screen and (max-width:991px){}@media only screen and (max-width:1199px){}@media only screen and (max-width:767px){}@media only screen and (max-width:767px){}@media only screen and (max-width:767px){}@media (min-width:768px){}@media only screen and (max-width:1200px){}@media only screen and (max-width:800px){}@media only screen and (max-width:1200px){}@media only screen and (max-width:800px){}@media only screen and (max-width:767px){}@media screen and (max-width:767px){}@media (max-width:414px){}@media screen and (min-width:768px){}@media screen and (max-width:767px){}@media screen and (max-width:767px){}@media screen and (min-width:767px){}@media screen and (max-width:767px){}.jobs-detail-header .header-title{margin-bottom:0!important}.jobs-detail-header .header-info>span{display:block}@media screen and (min-width:768px){.jobs-detail-header{position:sticky;top:64px;z-index:99}}@media only screen and (min-width:768px) and (max-width:767px){}@media screen and (max-width:767px){}@media only screen and (max-width:767px){}@media only screen and (max-width:767px){}@media (min-width:768px){}@media (max-width:992px){}@media (min-width:992px){}@media (min-width:1200px){}@media (min-width:768px){}@media (min-width:768px){}@media (min-width:768px){}@media screen and (max-width:767px){}@keyframes loading{0%{-webkit-transform:translateX(-50%);-moz-transform:translateX(-50%);-ms-transform:translateX(-50%);-o-transform:translateX(-50%);transform:translateX(-50%)}100%{-webkit-transform:translateX(300%);-moz-transform:translateX(300%);-ms-transform:translateX(300%);-o-transform:translateX(300%);transform:translateX(300%)}}@keyframes actionSlideIn-left{0%{-webkit-transform:translateX(0%);-moz-transform:translateX(0%);-ms-transform:translateX(0%);-o-transform:translateX(0%);transform:translateX(0%)}100%{-webkit-transform:translateX(-35px);-moz-transform:translateX(-35px);-ms-transform:translateX(-35px);-o-transform:translateX(-35px);transform:translateX(-35px)}}@keyframes actionSlideIn-right{0%{-webkit-transform:translateX(0%);-moz-transform:translateX(0%);-ms-transform:translateX(0%);-o-transform:translateX(0%);transform:translateX(0%)}100%{-webkit-transform:translateX(35px);-moz-transform:translateX(35px);-ms-transform:translateX(35px);-o-transform:translateX(35px);transform:translateX(35px)}}@media (max-width:992px){}@media (max-width:768px){}@media (max-width:992px),(max-height:719px){}@media (min-width:992px){}.jobs-detail-header h1 a{font-size:inherit;line-height:inherit;color:inherit;text-decoration:none}@media (max-width:991px){}.job-details-app-form .form .field{max-width:unset}.job-details-app-form .form .field .field-widget{max-width:unset}.job-details-app-form .form .field .field-widget .chip{max-width:100%}@media (min-width:768px){}@media (min-width:768px){}@media (min-width:768px){}@media (min-width:768px){}@media only screen and (max-width:767px){}@media (min-width:768px){}@media (min-width:768px){}@media (min-width:768px){}@media (min-width:768px){}@media (min-width:768px){}@media (max-width:768px){}@media (min-width:768px) and (max-width:992px){}@media only screen and (max-width:767px){}@-webkit-keyframes move{0%{background-position:0 0}100%{background-position:50px 50px}}@media only screen and (max-width:767px){}@media screen and (max-width:767px){}@media (max-width:767px){}@media (min-width:768px){}@media (min-width:768px){}@media (max-width:767px){}@media (max-width:767px){}@media (max-width:767px){}@media (max-width:767px){}@media (max-width:414px){}@media (max-width:991px){}@media (min-width:414px){}@media only screen and (max-width:767px){}@media screen and (max-width:991px){}@media screen and (max-width:767px){}@media only screen and (max-width:767px){}@media screen and (max-width:767px){}@media screen and (max-width:1199px){}@media screen and (max-width:991px){}@media screen and (max-width:767px){}@media screen and (max-width:414px){}@media screen and (max-width:1199px){}@media screen and (max-width:991px){}@media screen and (max-width:767px){}</style><style>.page-students #frame.no-nav{width:100%}@media (min-width:992px){.page-students #frame.no-nav .form-layout.has-sidebar .sidebar{margin-left:30px;min-width:0}}@media screen and (min-width:992px) and (min-width:992px){}@media screen and (min-width:992px) and (min-width:1200px){}@media only screen and (min-width:992px) and (max-width:991px){}.page-students .floating_content .sidebar .sidebar-content{margin-bottom:15px}@media screen and (min-width:992px){.page-students .has-sidebar .form-col{width:610px}}@media screen and (min-width:1200px){.page-students .has-sidebar .form-col{width:730px}}.page-students .has-sidebar .sidebar{width:100%}</style><style>/*! * Font Awesome 4.7.0 by @davegandy - http://fontawesome.io - @fontawesome * License - http://fontawesome.io/license (Font: SIL OFL 1.1, CSS: MIT License) */@-webkit-keyframes fa-spin{0%{-webkit-transform:rotate(0deg);transform:rotate(0deg)}100%{-webkit-transform:rotate(359deg);transform:rotate(359deg)}}@keyframes fa-spin{0%{-webkit-transform:rotate(0deg);transform:rotate(0deg)}100%{-webkit-transform:rotate(359deg);transform:rotate(359deg)}}.sr-only{position:absolute;width:1px;height:1px;padding:0;margin:-1px;overflow:hidden;clip:rect(0,0,0,0);border:0}.sr-only-focusable:active,.sr-only-focusable:focus{position:static;width:auto;height:auto;margin:0;overflow:visible;clip:auto}</style><style>.field:not([class*="space-bottom-"]){margin-bottom:20px!important}.field.last{margin-bottom:0!important}</style><style>iframe#teal-job-tracker-iframe-stable{min-width:380px!important;width:380px!important;min-height:100vh!important;height:100vh!important}</style><meta name=referrer content=no-referrer><link type=image/x-icon rel="shortcut icon" href="data:image/x-icon;base64,AAABAAEAEBAAAAEAIABoBAAAFgAAACgAAAAQAAAAIAAAAAEAIAAAAAAAAAQAAAAAAAAAAAAAAAAAAAAAAAD///8Ax3NN/7xsSP+8bEj/vGxI/7xsSP+8bEj/vGxI/7xsSP+8bEj/vGxI/7tpRP+7aUT/u2lE/7dlP//SeVD/////AOCBVv/SeVD/0nlQ/9J5UP/SeVD/0nlQ/9J5UP/SeVD/0nlQ/9iKZ//blHX/3px//+CjiP/lsZr/zXJI/////wDegFX/0HhQ/9B4UP/QeFD/0HhQ/9B4UP/QeFD/0HhQ/9B4UP/Rflb/2JFx/9uXd//cnoL/4aqR/81ySP////8A3oBV/9B4UP/QeFD/0HhQ/9B4UP/QeFD/0HhQ/9B4UP/QeFD/0HhQ/9iSc//bl3f/3J2B/+Gqkf/Nckj/////AN6AVf/QeFD/0HhQ/9B4UP/QeFD/0HhQ/9B4UP/QeFD/0HhQ/851TP/Xjm7/25Z3/92ehP/jr5j/zXFI/////wDegFX/0HhQ/9B4UP/QeFD/0HhQ/9B4UP/QeFD/0HhQ/9B4UP/OdEv/145u/9ybff/fo4r/5bWg/81xR/////8A3oBV/9B4UP/QeFD/0HhQ/9B4UP/QeFD/0HhQ/9B4UP/QeFD/0HhQ/9qWdv/eoIX/4amQ/+e6p//NcEb/////AN6AVf/QeFD/0HhQ/9B4UP/QeFD/0HhQ/9B4UP/QeFD/0HhQ/9OBW//amXz/36SJ/+Gulv/qwK7/zG9F/////wDegFX/0HhQ/9B4UP/QeFD/0HhQ/9B4UP/QeFD/0HhQ/891TP/Xjmz/3Jx//+CnjP/jsp3/68W1/8xvRP////8A3oBV/9B4UP/QeFD/0HhQ/9B4UP/QeFD/0HhQ/9B4UP/SfFT/2JFx/92egv/hqpH/5LWh/+zIuf/MbkT/////AN6AVf/QeFD/0HhQ/9B4UP/QeFD/0HhQ/9B4UP/QeFD/04Ba/9mScv/doIX/4ayT/+W2o//ty73/zG5D/////wDegFX/0HhQ/9B4UP/QeFD/0HhQ/9B4UP/QeFD/0HhQ/9OAWv/Zk3P/3qCF/+KslP/mt6P/7sy+/8xuQ/////8A3oBV/9B4UP/QeFD/0HhQ/9B4UP/QeFD/0HhQ/9B4UP/SfFX/2ZJy/9yfg//hq5L/5bWh/+zKvP/MbkP/////AN6AVf/QeFD/0HhQ/9B4UP/QeFD/0HhQ/9B4UP/QeFD/z3VM/9iQcP/doIP/4ayT/+W2ov/ty7z/zG5E/////wDohln/239U/9t/VP/bf1T/239U/9t/VP/bf1T/239U/9t/VP/dhV3/4Y9r/+OVcv/lm3r/6KSG/9p6Tv////8A////AP///wD///8A////AP///wD///8A////AP///wD///8A////AP///wD///8A////AP///wD///8AgAAAAIAAAACAAAAAgAAAAIAAAACAAAAAgAAAAIAAAACAAAAAgAAAAIAAAACAAAAAgAAAAIAAAACAAAAA//8AAA=="><style>.sf-hidden{display:none!important}</style><link rel=canonical href=https://ecc-uoft-coop-csm.symplicity.com/students/app/jobs/detail/17860b208317feac1449f4953038c9da><meta http-equiv=content-security-policy content="default-src 'none'; font-src 'self' data:; img-src 'self' data:; style-src 'unsafe-inline'; media-src 'self' data:; script-src 'unsafe-inline' data:; object-src 'self' data:; frame-src 'self' data:;"><style>img[src="data:,"],source[src="data:,"]{display:none!important}</style></head><body class=page-students><style>/*!******************************************************************************************************************************************************************************************************************************************************************************************!*\ !*** css ./node_modules/@angular-devkit/build-angular/node_modules/css-loader/dist/cjs.js??ruleSet[1].rules[5].rules[0].oneOf[0].use[1]!./node_modules/postcss-loader/dist/cjs.js??ruleSet[1].rules[5].rules[0].oneOf[0].use[2]!./node_modules/flickity/dist/flickity.css?ngGlobalStyle ***! \******************************************************************************************************************************************************************************************************************************************************************************************//*! Flickity v2.3.0https://flickity.metafizzy.co---------------------------------------------- */</style><my-app ng-version=17.3.12><header top-nav class=ng-tns-c2484691837-0 ng-reflect-enable-router-link=true><size-detector id=header class=ng-tns-c2484691837-0 ng-reflect-id=header><div class="display-mobile-block hide is-0 ng-star-inserted sf-hidden"></div><div class="display-sm-block hide is-1 ng-star-inserted sf-hidden"></div><div class="display-md-block hide is-2 ng-star-inserted sf-hidden"></div><div class="display-lg-block hide is-3 ng-star-inserted sf-hidden"></div><div class="display-desktop-block hide is-4 ng-star-inserted"></div></size-detector><ul class="skip-links ng-tns-c2484691837-0"><li class=ng-tns-c2484691837-0><a href=javascript:void(0) id=skip-links accesskey=c class="sr-only sr-only-focusable ng-tns-c2484691837-0" title="Skip to content"> Skip to content </a><li class=ng-tns-c2484691837-0><a href=javascript:void(0) accesskey=m class="sr-only sr-only-focusable ng-tns-c2484691837-0" title="Skip to main menu"> Skip to main menu </a></ul><div class="topnav ng-tns-c2484691837-0" ng-reflect-ng-class="[object Object]" ng-reflect-ng-style="[object Object]" style=height:auto><div class="navbar-wrapper ng-tns-c2484691837-0"><div class="container ng-tns-c2484691837-0" ng-reflect-ng-class="[object Object]"><div class="header-menu ng-tns-c2484691837-0"><div id=page-brand class="branding-bg ng-tns-c2484691837-0"><a class="nav-brand branding-bg ng-tns-c2484691837-0 ng-star-inserted" ng-reflect-router-link=/home href=https://ecc-uoft-coop-csm.symplicity.com/students/app/home><img class=ng-tns-c2484691837-0 alt=Home src=data:image/png;base64,iVBORw0KGgoAAAANSUhEUgAAAKYAAABYCAYAAAB/EIweAAAABGdBTUEAALGPC/xhBQAAACBjSFJNAAB6JgAAgIQAAPoAAACA6AAAdTAAAOpgAAA6mAAAF3CculE8AAAABmJLR0QA/wD/AP+gvaeTAAAACXBIWXMAAA7EAAAOxAGVKw4bAAAAB3RJTUUH6AcIEgw1pPM7gQAAGBtJREFUeNrtnVlwZNd533936X3vBrrRQKOxDzDYBrNwOAuXGVIkNRQpU7Qp2WFky0mklOQ4iV8SJ5WHPEaVh5QrZceplFVlKZVyqrwoWiKKlrkvnuFsnH2AATAAGmuv6L3vmocGMEMKQ7JCSdOS7+8B1eh77+m7/M93vu9/TgNC8sGvmlhYtBji/T4BC4vdsIRp0ZJYwrRoSSxhWrQkljAtWhJLmBYtiSVMi5bEEqZFS2IJ06IlsYRp0ZJYwrRoSSxhWrQkljAtWhJLmBYtiSVMi5bEEqZFS2IJ06IlsYRp0ZJYwrRoSSxhWrQkljAtWhJLmBYtiSVMi5bEEqZFS2IJ06IlsYRp0ZJYwrRoSSxhWrQkljAtWhJLmBYtiSXMFkIQBFxOO6Io3O9Tue98YmGapolpWn9K8+eJKArEoyFssny/T+W+85F3wDRNPG4nh6eGcDntzC2uMT23agn054gkiQhWwLy3ME3TJBLy8dixCR45MobLaSdXKPPG6av87Zvvoyja/T73X0msLt/knkO5IAgcntrDyWMTlCt1DMPA43Lw2Uf3k+iIYFhR0+LnyD0jpk2WiEeD2G0yya52ZEkkky9SqTSQJKtmut8YxlZgEEAATLOZo26nWebOj2aQMTF39vtwqmDu7Hfn9d3tbm+DD7Uj3n3AXfuKAp82G7mnMFVN593zN5FliVh7kN5ElCs3Frk5t8zSSgbRSoTuC6Zp0hb2c+LoGG6Xg7WNAtlCiUjQx+kL0+wb7WNucY1IyM+DU0PMLa5x9tIsBycHWF3PM9AT48LV23hcDiIhH5Is0peIomo612+l6OlqJ+B3kyuUWdvIs6e/C1XTOX95lnpDZWSgi43sJj1d7bz13nX29HeSL5SRZInjh0ZYTxd49d0rVKqNT5UrS4HEwf+42wbDMMkVymyWqkRCPqJtAV7/+6ucfX+WUrWOwFZPvLuL3ZUhfXDbB7l7211HAObO7x/Vtsm9czHhrrtx9+cLgvCB9GN7P9M0P9Tenc/aPofdz4mdEPPh901z9/MTPuZJiaJAKOClWKqi6cau+5imyfBAF3/4jefRdINSpc74cA+/9zunuDm3wvOnjiBJIi9+4RFCfi8njo6zWazwpWcfwjBNvv6Pn0KSRNojfh4+vJfJvT1MjfZRrNQwDJOv/qMnEEWRWk1hqC/OM585hMft5MTRMXTD4NdPHUWSRf71P3uG+cUNHjkyRrQtwK89eZh4LMyxQyMUNivcnFv+VMFr14gpSSK9iTYiIR+H9g3ictiZX1xnaqwPSRJZTxdY2cizWayQ6GhD1XSqtQZejxNF0ZAkkXSuSGc0TKVWJ+D3UK8rSJKIquoUihWibQEaDRWvx4mq6RRLVdwuB16Pk1K5xka2SCjgwedxkdssEwp40DQDwzDwelyASaXawGaTaCgamqZjmibZfGlHBO1hPwG/m3SuiKJodHWE0TSDYrlGNl9CkgQ62kN4PS5M06RQrFJvKLSH/dQaCplcia5YeCuNKVEoVmgL+wkFvGTyRTRNJ+T3srKRIxoJYJgmDrsNl9OOruvkChVM0yAS8rNZqpDOFv+/H9QHBCwIKKrGu+du8u75aX7jc0cJ+j089egUToeNjvYgLqedb/7xX7N3KEGl1kCSJURBQBAFThwd5/yVOQRRQJJE5pc2eOnVCwiigGnC2UuzvHXmOqdO7mdlPc/rp6/ylRdOEvB7ECURURBw2G08+egUyta9j7YF+Oaf/A2RkI/NUvVTj6g/FTFNE1xOO7//u5/j937nFMMDnQQDHsJBH6N7unn6sQM8/tAkM3OrzC1ucOrkAVwuO5Vag/HhHjBNvvwbJ0lnN+nujKAoGieOjmMYzQd0cGKA1Y08hyYHMU2Tk8fGqVTr6LrBwYkB9g51M9QXJ1soceLYOLIkUqk2ePjwKEG/m3KlzrGDI8RjITK5IsnOdg5PDeFxO6jWGuQKFcBkoCfG+EgPoiDy0AN7KZZrPPfUg9TrCoZpksltbuXRIU4cHSfo96JpGmPDSRRV48SRMRRF4/gDI0TbgvT3xGgoKhMjPQA8/OAolWqd33zuYXTNIBLy4XE7sMkSzzx+CE03cLscDPV1YhgGJ49NsLKWo1Sp3TNyftKIGY+Gee6pB4lHQyytZmgL+xEEgURHhEjYz+zCGvH2MPlihf1jfaSzRUaHEiyuZIiEfNQbKpN7e1lZz2GTJabG+gBYWE7z9MkDJLvaWUvn8bgdPPXofg6M93Pu8iw3ZlKMDydZXssiSSKRkI/OjjCLyxli7UFy+RL7x/pYSxeYXVj72BHiIzvfLpcOQDDgIRGPEA76MA0DwzSwyTKJjgiJjgh2u4yu69QbCuVyjVK5xmaxQm6zzMz8Co8dn6At7KdQrFAsVdksVTl3+RZOp439431cvrFAOlfEZpPxelxUanUUVaMzFkISRTZLVSrVBsP9XciSRLFUJVcoc30mxVo6z/JajuszKd567zqKquFxO5lf2thJ4EcGE5imyemL09htMqGAF1EU8HqclMtVoJlHX5tJkc4VWVhOUyzXiEYCvH9tnoVUmmRXG5qm09URplKpM9gbR5ZETl+YRhZFggEP16aXeGDfICMDXaxnCly6vkChWGHm9ipg4nLaOX1hhlK5RrKr/WfiAQuiQLZQ4o///EecvzSLLIncml/l+kyKeDSErhuIWx16fDjJYF8HpmkiCFCrK7z6zmXaw35ssoxpwkuvXeDP//JVVE2nUmvw377zEm+fvYEsSdycW+byzUVqdYWGqiEIAqIosLKe4/zlWXoTUQzDQAAqtQZ9yRjjI0k+7WXuIsymyrP5EgupNLlCiUqtQa3WoFKtk1rNcjuVptFQQRAoVWp0d7XTn4xRrtZxOe2srud58/Q1kl3t2G0yHrcTl9NOsVzj+q0UbWE/iysZXE472lYa0Bb2I0sSqdUstYZCT1c7mewmhWKFjmgQj9uB02FHliU8bicet2MrNdBYTxeoN1QMw9i5hPmlDZKd7YwPJ1lZz1EoVlA1ncs3FllLb+70ZlkWcTvteN0OMrkikiRwcHIQgLmFNURRYH5xHbfLwWaxSjwWZnJvL2uZAoXNCrW6wg9fOUck5EWWpJ2h3ONysLyWIxLysX+8n2KpyuJy+lNFkW10XcfpsPOVFx7jn/zm4zgddorlKj945RyrG3lSq1kKm2Wee+owkiRRqynUFRVV01EUjTMXZ3jn3E0UVUVRNY4eHOEPvvp5pkb7EASBF597hH/+4pME/R7W0gV+9Mo5hvu7iEdD1OoNVFWnWlN46fULzC+ts54psLSS4ZnHD+HzuKjWGnzaslz48L/sMwGPy0F/MsZjxybYO5Tg3OVZrk0v8ewTD2Czybz8+kWuTi+xvJbF5bTTn4yhajq3lzbweV0E/R4WUhsE/B4aikp3vI1CscLqRh67XcbrdpLNl2gL++mMhVFVjUy+hNftAKBUqRPye5BlCd0wWN3I0xkLU6srrKXz9CaiaJrOQiqNYZoku9oQBIHF5XSz8NiyTrrjETweJ6nVLIZh0tcdZWklQ6FY2SlYbDaZgZ4O6nWFheU0kZCPeDREOlskVyjR39NBvlDGZpPQdQObTcbndZFazWIaJh3RIIvLaTxuJ7W6AsBATweZXIm1dJ54LEQ46GN1PU+uUPrIhyFJIn3JGKmVDPWGuus+pgkBv5up0V5cTjubpSqFzWanS61mmBjp5fbSOj6vi7HhJKsbedLZTSJBH5l8iXDQy83ZFbo6wjjsMqIk0hULoxsmi8tp2sJ+/N6muDK5EqZpsrCcZnSom2K5ittpJ1+s4HQ0ZwLH9iTZyGwiCLB/rJ9MvkhqNbvTCc0d30q4q0hs/v5RawKE3f6XpMNuozMW4hu/fYqpsT7ePHONV9+5wr/4ytM4HDa+9Rc/4d3z02RzRRwOGwCKqmGaYBgGoiDgcjnQdQNBaG6TRHHL9wJN13E7HZiYaNpd+0gijYa648Fte2aCIGJiIokCgtCcsnM57aiqhiRJADQUFbtNptFQsdnkHU9PFER0w0CWRUwT6g0Fw2i2a7fLIAgoiorL0Tyf5pqAprBFQUDVdAQBGoqGw25DVbWdazZNE1EU0XWDekPZiYaGaX7AtWg+ho+vyj+JMKF5bhMjPYwPJ5lbWOf0xRm8bgcPPbCXmflV5pfWOXJgmGhbgI3MJheuzjPQE2OorxNdN5ieWyERj+D3uiiWq6RWcwz1xdENg0vXF7i9tI4J2CSJAxMDjA4luDq9xMJymsP7BvG4HWxkixSKFXweF+evzDE+nETXdbo6IsiyhGmapFaydESDyLLEzdllVtZzHDkwjN/rYrNU5Y3T16jWlF1tpV3tIkEQcDsdnLs8y+t/f4Vbt9dwO+28e/4m/+flMywsb2w9DJXPPDRJJOSj0VCZGElimCYjgwn8Xhcel5MH9w/RaKh0xcIku9rojreRK5Q5tG+AfaO91OsNDkwM4Pe5cTps5Dcr+L0uohE/fq+bUMBDta6gGwbD/V30dkfBNHn8oUni0RADPXFGBxO0hf2MDCaavXtPN3v6O+lPxoiEfDgcNo7s34Pb5aAnEWVlLUcw4OWBfUNbDkSMgN+D02FjdKib7s4IIwMJ/D43Loedx45Poqga3fEI3Z3thINenA4bE8M99Cdj1BsKxa28dfv+bYtw+/UnGcI/SfFjGCaDvR38h3/5AuGAjyce2cethVV6ElH+07/7MjabxPtXb/Nvvv4cg31xHty/B5/HxZH9ezhxdBxV09B1g69/+bN4PS503aA/GeNLzx4n6HfzmeOTnL00S6lcY3w4yR9+43kCPjdPPjoFwO9+6XEkSULVNCZHevjai08yPbfCF589jtNhZ3Som9/6tYcxDROH086Xn38Ut8vBk49MUasrfO3FJ/G6nWi6ybXpJRqKuuu92XUKR9N0dMMgWyjR0R7i337jC3zz3/82z586Qmo1AyZbOZ2Jy+XAZpOw22WG+js5MDHAUF+cxeUMC8sbOB02DLNZaIiCgCxLbBarrG3kqdYa3JhdZm5xnb2DXays55FEoZmw98Y5cXScgd4OBAHsskR3vI3x4SQOu22rXRObLGGzy8iSiMNu24mGmCZ2uw1JEresJEitZhnqi+PxODEMg7aQj8HeOGPD3axt5JlfXMcwmhHT4bBhkyUaikqpXOPw1BDxWJjh/k4yuSK3bq8hiAJ2m4yuGZ862f+kmJh0xSIA/Jc/+z7f+avXaDRURvd0k1rNkuxsb9o6osi7524wM7/Cnv5OnA47swurfPfHp5meW8YwTd45e4PvvnyGSrXOWrrAy2+8T0c0RDjoxTBN+pIxag2F//zfv8v//t5bqKqGqmq8+s5lfvTKeWp1hVhbkM+eOIDH7eTm3DLf/svXWM9s8u2/fo1rM0tUag1+9Op5nA4bPYl2DMPgzTPX+d7LZyiWP8KhuNcNmNzby+PHJlhLN5PpppfoJR4L8/knDhP0uXeqvMXlDG6ngys3FrFJEuvpAoO9HSTibWTyJXoT7QiCQH3Ltxzs60CWZQzdQBRFcoUyxVINXdfRdINMrki+UObm7DLzixsoikbA7yG3WWY9XSAS8pHNlXj7vRtsFitcm17i8s1FREFgT38nAb8bc7uD6QZetxNJFEjEI0zPrVCp1IGmPVKrN5hf2qC3O0p/Twey3EwNVLXpzwX9HjL5Im+fvYGiaFy/lSIRjzDUF98a4lU8HifyL3CaVhQFdN2gUq3z49cvsrSaZU9fJ2cvz+J02olHQwiiwJc+/zAPHx7lrTPXUFSNIweG+fWnj+HcKs5eeOYYhyYG0HWDvYMJ/tU/fYaZ+RWWVrKIgoC0laYUSzV+8MpZZhfXCQW8vPiFR9g7lEA3TN6/fpvRoQTJzjZ03UDTm36yphnoukGsPcgffPXz1Ooql64v4HE5+eKzxzk0OfCR17irwW6TJaZGe+npjvJfv/VD/uhbP+CLzxzn0vUFRocSnDw2zqXrt1lcyfDm6Ws0VBVZFNF0gxuzqaZ943JQVzQWl9N4PU4qtQYCAvNL66iqjm7kub20gQDkCiVeeftSM780TabnVhBF8c6sjAnFUo1rM0vcuJVCEASuCymqtQZnLs6gak1B/+St9zFNtoqg5oyMJIqous7tVPO9crWOZhiUKzWm51aoNxRUVcfndaFpOmvpPACS2DSSNV1HEARKlTqplQyGYeL1ODEMk5X1HHZZRtkaHn9R6IaJJIkEfG5e+NwxCsUKg70dDNJBKOClvyeGpuq8d3GGkYEusoUyhmnwk7cu8T/+18sE/R5K5Rp/+j9/zHsXZ3j+1BHmFtfJb5YpFKs7RZyuG0iiSCTk5flTT1Ct1UnnivzRn/2Q6fkV9o32ceNWCo/byeceO/iB2a7tnDqd3eTm7DJ+r5tiqUq5WudPv/NjLlyZ+8jZwV1zTMMwUTWd8eEkD04NUSzVmF1YY3RPN8cfGOHKjUVeefsylVoDRW0+lG0vsTcRxWG3YZPlZoGi6Xg9LkRRpD3iwwT8Xhd+rxuf10W9oRIJ+nC7nHR3teGw2wgFPHTGwthkiY72IB3tIWqNBrFIAK/XRdDvZrNUJdYWQDcMwiEfkZCXfKFMqVKjoag0FJ2GolJrKChK02+tN9Tm4gOaBUqtrqDrBubWa0Vt2imKolFvKNQaylY72s59MU2TekOhsbVfrd5AUfWfieA+SY6JCZIscuLoOAfG+xkZTJAvlPG4nPzJt1/C0A3awj68Hhdvv3cDA5OuWAiH3c7+sX76uqOIosD+8X6Sne3098Sw22zIksj/feUcjx2f4PyVObKFMrIs8vhDkxyYGGCwN87Vm4scnBygOx6hJ9HeDDiVOm+cvsrByQFee/cqxXKVR4+O8+q7V3A67EyO9PAX33uLY4dGKFVqTO7tIdnVTl93lOm55a059Z8eznetyqE5Ddyf7OD3v/I09YbK371zia/91pN8/+/e47svnWazVEWWJMaHk5SrdeYW1+mOR3j0yBjvXbqFy2HnyIFhzlycwed1EvR7uXBlbmvRQAzDMOiIhihX66yl89jl5iqm0xem6e5sw+txcnV6iX17ewkFvLx3aYZ4ewhV0+nubCObK5HbLBPwuSkUK+QKJXKFMtl86WfiFd4PPnFVLjTz8PGRJLdur5HJFfH73Fy8Ok93ZxvxaAhJEllYTmOTZeLtQXTDoDcRbY5I8yvEoyECPg+Vap3FlQw2WWJ6fpWp0V5m5ldZS+eRJImpsV5GBhJcv5UitZrZmmVzkt8sk84WqTcUFlJpDk4MMD2/QrXWYGq0j0vXb2O329jTF+fyjQVGBhOomk6sLUjA56ZcrfHG6WuUyvVdq/J7ri4yDJOl1QzpfJGBZAePHZtE03VW1/Pki5WmJeS0M7qn6W8tLKebghZFBEGgoWpcvtEc+svVejOK2mT2DiZQNY1aXWchtYHH42RypJdr00uIgoC4dbwoCIiCiM0m4fM6dxYmG4bJ/OIGbreD4YFOiqUahWKF3kQUl9NOJlf6lV8BbpgmF6/d5uK12817LtzxbheX0yyk0jvvA8wvroMApy9Mb70vcPHqbe74i9vL4QTeOH1tx0UwDIOz789y9v3Znfa+/7dnd47bXhrXPO7qznFvnL62ZQ3Wmh6nKHD6wsxdy+ru+Jj3elb3jJjQzLOmxnrpikcQBAFN07l4dZ7UahZBEJAliXgshLFlgoeDPkYGushtljEMg1pdoVZX8GwVHwGfB4Rm7qJpOgICCytp4tEghmHSHgmQy5ew2WScDhubpSo+j4uGoiKKAjZZot5QEUWR26kNOmPh5mxMtLloYX5pg5X13K98xPyHwEcKE9g1Od1tadkHfbs7i0YNw9jp0pIk7cyrbv/Y/pKbKAoIW13w7sWs24a3dFcxtJ0jbn92s+cJdxbP/pJiCfMOH/t1vI+LPh/evi2kn9q+NTTA3aur7173uLUa09xu54Ofoxt3igHzQ203pyF/uUW5c7/u9wm0CNZ3JFoMTf/FmfWtjPUF5hbCMAxW1rI/M/vplxlLmC2EabLjmf5DxxrKLVoSS5gWLYklTIuWxBKmRUtiCdOiJbGEadGSWMK0aEksYVq0JJYwLVoSS5gWLYklTIuWxBKmRUtiCdOiJbGEadGSWMK0aEksYVq0JJYwLVoSS5gWLYklTIuWxBKmRUtiCdOiJbGEadGSWMK0aEksYVq0JJYwLVoSS5gWLYklTIuWxBKmRUtiCdOiJbGEadGS/D8G8Ukisy5V9wAAACV0RVh0ZGF0ZTpjcmVhdGUAMjAyNC0wNy0wOFQxODoxMjo1MiswMDowMLQYw10AAAAldEVYdGRhdGU6bW9kaWZ5ADIwMjQtMDctMDhUMTg6MTI6NTIrMDA6MDDFRXvhAAAAAElFTkSuQmCC></a></div></div><div class="topnav-search ng-tns-c2484691837-0" ng-reflect-ng-class="[object Object]"><div quicksearch class=ng-tns-c2484691837-0 ng-reflect-config="[object Object]" ng-reflect-is-quick-search-collapsed=false><div id=page-search class=flex><div class="ng-quicksearch quicksearch" ng-reflect-ng-class="[object Object]"><button class="icn icn-search quicksearch-toggle avatar monogram btn-reset sf-hidden" aria-hidden=true></button><div class=quicksearch-form><button class="search-back icn-chevron_left btn-reset sf-hidden"></button><div class=quicksearch-autocomplete><label for=quicksearch-field translate class=sr-only ng-reflect-translate>Quick Search</label><input id=quicksearch-field type=search typeaheadoptionfield=label autocomplete=off role=combobox class="background-lighter-gray ng-untouched ng-pristine ng-valid" ng-reflect-typeahead-option-field=label ng-reflect-model ng-reflect-typeahead="[object Object]" ng-reflect-typeahead-hide-results-on-blur=false ng-reflect-typeahead-select-first-item=false ng-reflect-typeahead-wait-ms=300 ng-reflect-typeahead-async=true ng-reflect-typeahead-min-length=0 ng-reflect-typeahead-item-template="[object Object]" ng-reflect-ng-class="[object Object]" aria-expanded=false aria-autocomplete=list value></div></div></div></div></div></div><nav class="ng-tns-c2484691837-0 ng-star-inserted"><ul name=menu class=ng-tns-c2484691837-0><li class="ng-tns-c2484691837-0 ng-star-inserted"><a class="ng-tns-c2484691837-0 activated ng-star-inserted" ng-reflect-ng-class="[object Object]" ng-reflect-router-link=/jobs/discover tabindex=0 href=https://ecc-uoft-coop-csm.symplicity.com/students/app/jobs/discover>Jobs</a><li class="ng-tns-c2484691837-0 ng-star-inserted"><a class="ng-tns-c2484691837-0 ng-star-inserted" ng-reflect-ng-class="[object Object]" ng-reflect-router-link=/employers tabindex=0 href=https://ecc-uoft-coop-csm.symplicity.com/students/app/employers>Employers</a><li class="ng-tns-c2484691837-0 ng-star-inserted"><a class="ng-tns-c2484691837-0 ng-star-inserted" ng-reflect-ng-class="[object Object]" ng-reflect-router-link=/resources tabindex=0 href=https://ecc-uoft-coop-csm.symplicity.com/students/app/resources>Resources</a><li class="ng-tns-c2484691837-0 ng-star-inserted"><a class="ng-tns-c2484691837-0 ng-star-inserted" href="https://ecc-uoft-coop-csm.symplicity.com/students/index.php?s=calendar&ss=app_main&mode=landing" ng-reflect-ng-class="[object Object]" tabindex=0>Counseling</a></ul></nav><div id=user-avatar class=ng-tns-c2484691837-0 ng-reflect-ng-class="[object Object]"><div class="user-tools-container ng-tns-c2484691837-0 ng-star-inserted"><button aria-haspopup=true aria-controls=user-tools class="contact-summary avatar avatar-img-cover monogram badge-toggle badge-toggle-closed ng-tns-c2484691837-0" ng-reflect-ng-class="[object Object]" aria-label="User Menu. 0 notifications inside."><div class="avatar monogram ng-tns-c2484691837-0 ng-star-inserted">AB</div></button><div id=user-tools role=navigation class="ng-tns-c2484691837-0 sf-hidden" aria-label="User Account and Tools links"></div></div></div></div></div></div></header><main-layout ng-reflect-enable-page-header=false ng-reflect-enable-sidebar-nav=false ng-reflect-enable-skip-links=false><div class="banner-msg-list webview-no-top"><div class=ng-star-inserted></div></div><div class=container><div class="row block"><div id=helpdiv></div><div class="nav-mask sf-hidden"></div><div id=frame class=no-nav><main id=content-view><toast-alert class=sf-hidden></toast-alert><div class="page-utils webview-hide"><div class="row ng-star-inserted"><div class="col col-12 sm-col-8 back-links"><nav breadcrumbs role=navigation aria-label=breadcrumb><ul id=csm-bc class=breadcrumbs aria-label=breadcrumb><li class=ng-star-inserted><span class=ng-star-inserted><a href=https://ecc-uoft-coop-csm.symplicity.com/students/app/home> Home </a><span class="bc-sep space-right-xs space-left-xs">/</span></span><li class=ng-star-inserted><span class=ng-star-inserted><a href=https://ecc-uoft-coop-csm.symplicity.com/students/app/jobs/discover> Jobs </a><span class="bc-sep space-right-xs space-left-xs">/</span></span><li class=ng-star-inserted><span class="current_path ng-star-inserted" aria-current=page> ML Developer – Software Automation & Dev Infra Intern </span></ul></nav></div></div></div><div class=page-title-wrapper></div><div class=page-main><help ng-reflect-help-hints class=ng-star-inserted></help><div name=content ng-reflect-ng-class=floating_content class=floating_content><div class=content-container-inner><router-outlet></router-outlet><jobs-detail class=ng-star-inserted><div detail-page-header class="jobs-detail-header ng-star-inserted" ng-reflect-auto-color-id=bc997a3059404ec36afefcff4c8866 ng-reflect-logo=https://s3.ca-central-1.amazon ng-reflect-logo-alt="Cerebras Systems logo" ng-reflect-logo-router-link-config="[object Object]" ng-reflect-avatar-style-class=hide-sm ng-reflect-title="ML Developer – Software Automa" ng-reflect-subtitle-style-class ng-reflect-buttons="[object Object],[object Object" ng-reflect-info-loaded=true ng-reflect-infos="[object Object],[object Object" ng-reflect-tags ng-reflect-title-label=13d ng-reflect-intersection-callback="function () { [native code] }" ng-reflect-intersection-config="[object Object]" ng-reflect-title-router-link=/jobs/detail/17860b208317feac1><div class="header-content ng-star-inserted"><div class="flexbox full_width"><div class="header-avatar hide-sm ng-star-inserted" ng-reflect-ng-class=hide-sm><div class="avatar avatar-md avatar-square space-right-lg" ng-reflect-ng-class><a ng-reflect-router-link=/employers/bc997a3059404ec36af aria-label="Cerebras Systems" href=https://ecc-uoft-coop-csm.symplicity.com/students/app/employers/bc997a3059404ec36afefcff4c8866b1><img src="data:image/png;base64,iVBORw0KGgoAAAANSUhEUgAAAHIAAAAyCAYAAACAhY8RAAAABGdBTUEAALGPC/xhBQAAACBjSFJNAAB6JgAAgIQAAPoAAACA6AAAdTAAAOpgAAA6mAAAF3CculE8AAAABmJLR0QA/wD/AP+gvaeTAAAACXBIWXMAACWAAAAlgAFsVQgHAAAAB3RJTUUH6AgdFi8vBaYtOgAAEP1JREFUeNrtnHmUVdWVxn/7vkdVQRVVxVDgQAtUAQbUCIhjjEaQKsUhJtqO3R2Hxk7a2MYYI9p2HJK2McshjUuj0bRGzdJItMUBBVEUUdGOElEDGMBiktmqV0BBDe/u/mPvm3vr8Zh0Acp631pvVdW95557ztnTt/c59YQvgMzoGkIlFaS0CpVDgcMVDgH6A/sAvYEVwChgYeXUhV/kdQVsA+nP81Cmtj+IFKMME2GMqtQB1UBlnj7TQOc9PdG9HTssyJW1B9E5u5EsqRQwFGWswhmY1eVDGP1UpWRPT3Rvxw4JMlNXTXvQLJAaHMCFCucCf5fTbAOwCJgH/BWoR/gMZRkwd09PdG+HbK9B4+gBKBSL6LnAdcDAxHMhMB+YLDAZdKEiLQI9FfZD6OntAv80i7JUkU/RcGXQKWgrn7xgT6/BXoFtCrKxtgaQbqBXAv8GVPgtxazvIdDHBVpBjlQ4BTgQs9YqtrR4BZqAJcAnwBsCL2IW3FJRIEOfG3kFuea03hRvqiAMsv2BG4DzgKLoNvAw8JDYtXMUTsIEWLyT7w+BZQIvAxNBZyBsrJiyaE+vy1cOeQWZqa0BqFb4NTA60W4eMC5QeVOFCxS9HEs1kv1ksXiZAVYBLX5fgJ7+KWdLa80AfxD0zpBwvhBo5dSCQHcUWwgyUzsA0O4KE4AL/LICM4BxYrHuGrfCyEpDoAGYJTBVzVV+CmxOvCErEKjSG/gacARwIuaGU4n3fCAwHsInQVorCsLcIXQQZEPdAIASUf0Z8BOgE7a4TwE/BQ4FbsNyxghLgceAyYI2KXIg8HWsMFBD7G43AguBDzFhvaeqJYicBJzvfQfedqqg54OsK8TNHcPfBLl5VF96j3mFxVNGjwW5Hejqt6YDY0FGgN4O7O/XW4HngTtAsyDfBk7HhLy9WNmCCXWSwF2ClCh6pcL3gDbgkjAMJpWUtNLlucW7ay16YGGkGNiMxe21u+vlXxQCoMf3J1MSIMpghaeBQX7/L8CFwADgTuLkvwm4VeBJhbOAHxALOIkQs+joXUHO/XliTHeRhFKigZ4G7IvofUDLbiY9I4CXsOrUWmAM8H+7cwBfBGmAjV2gGE21ZuViYiGuA64DDUFuIhZio8D1wGyPoyPpSFxWAO8Ds4EPMMITYLXXQ4ChwHCgp8DzxVk+adeQ9qxuBiZ627DytfrdvRZKrHRfOaQbRg8iG7aTRUaopRkRJqLMQuRurAgA8BlwjcDcEO7CBBJhEfB74FlEV4P0Q+mvyP7ARIHNFVOVpjpKUYYrjFJ4piUlGgZKtxfro35CCthppIUQDYNiRC8ldo+Lgd8iXIDFPYB24C6BOSHcQyzEVuAZgVsV1gPnoDIGGAKUCfoJ8DpQLyykUQ/YWMEZr6/nuZnihlwRC7GAz4m0iAIMVYsJER4RaFb4V4y5AkwT+KPCL4HD/FoL6K8EmQCcCvwISy2SbLgU6AXUA1ROXYJ55K+uG/syIl2+qIlMdflILIYBLAAeVTgNS/YBVgK3eQlutF9rBf1vUe5R4SrgUqDM74XAeoGlgcj8lNCypye6tyOdqS6vxBLzCNMxF/ldYpb5IrAJY6cRsXlR4C4VuQK4nNhy16SEP7SGTHp4edOnT63cUDmnqaVnpj08FHPZTeSPg50xy43c+zLiylASJcT7m21YFamTP7cfxjgXJN4h3n5f/7R536v99+2hzPvu5WNfCjTmmUMpcYFks69XCXAARhSX+PzBCiDlPp5e3n65z7c1zxgk0b7K5bMMK8JkAdKKDBL0QH+gVWCyGhUf5tfWYynJaKCvX1sucIsio4DvJ4Q4Oy1cf/gbSz+au6H1bODnvghdXCD1wHNYAaHenynGThBcjDHacr/eALwJPAC8lVi4C4Br/PcZwC9cwc7AcsHXvK+ML+7JWAp1sP8dYox8uvf9Z7Z08+pCOBO4CGPbXXyRlwBPYsRuRUIwN2FeDOB+TPmvAr6FpTSPYaHqUIxUnuCC6eLCyPjY78fSnmhMlcDZWNGkGlPKVkwRp/kc5qcFPcqlDLBMbe/wX3wiAO8K1KsVzyM8Cboa5B5id/pnES4pm7Kw0id5JFvWU/cDjvZJRMn/z1w43XPaVmGp0Ejgx754uLAiFr3OBXkucZmvh2vwAb64ZxIXNyL0Agb7Il8GvJpzvwiL9ycA3fLM4XDgm8CVGFsXoA9x6nYExhmOTzzXza/dkRh/pDTiYxoIHIcp5isu5GuBK9iyyLIfpvhDgYvS/vLIJXyM6lpEDko88I5am8hqG4CnQE73TgDWFwfc3PnFhc3A7zANBrPCRZi729c1KovtYbZhZcDLMBc+B3gCc13dMO0+zgVyLaalS3Imc6iPIYtZ+CbMSsqA/8AsUYG3XRFWYlzguy6MId73bDpaZQXwHb9Wj7m9TljJsYcrzek+nqv8/cnnT8KsfwPmAsWVrh0zkCbgBcwCN2Be6ywsExjkfb4F1GJGFVWbpgHv+pqM8XfMBNaniQkNwCciUqmxC23zRR/o2gHwIYR/VYKrXQAIvHzf4qaZwPiEENdgDPcp/31/H1QWuNk1+lLv4yPMHb6bGMvjwG98wYZi7ujWHEF2xorz/4mV1Jq9v1rinHiWC/TjxHNPAf/jinI85pbn5PTdAjwI3OvC7OTjuB6zZIBzgEn+7iS6YkWRmzHXHVW4lvg8y4AprngRnnZlG+LvORALcdEe8Fs+j3U+x5MwK34c2JymY2ltCRaY+/jfGSxAH5do87EQVKi9EKC9KJDJP5q7pi/GanFh3Ym5kSi2zcO0X/3aPxG7rYdzhAgW+H+HaV7af95FR81vwdznbxLXyrGYUurjeCBHiGB13t8D38C0/bQ8bSb5eDOJay9jsekJLM2qwlz3jJxnV2Dh4BW2xDT/KS7wiLht8nENwcLMPj7+CPsDx7pA1wGTk52miWNT6Al9EXF8bEY0g0q/5CKoaUkUVxtK0sG72I5HdLRjEaZducxuc2JQX0sIfSTmBZK12BCLAxH6YW4tKch5uRPCtDRSMnUhHZan72r/mfK+qxJ9b8DISYYt8QG2WRCNfzCmkMm5vumffNjH53sM5g0rXKilxLtKgY/rHYwhV2Iu9xGs/v2+C/RVzPjCtHcA1tumnJdGE4tYqSrSJmh5QtgtFAVr/GUR4ViFxaOtoYzYVaeAOraPTsTEKsJarGyYRAUxMUhjbnN7KPW+o/luxJRxa1iIhZ1oTLlEZCn5U5tRmAcZkeeZdjoqW4Ax3/EY8eqFWfCR/rkQ8yK3AE+mc164tVN1f9vBEPu1PXEtINQSYmvDX9gVC+r5sJk4Xwqx+LSarZ8hisjChm2MLdl3ZB1ZzGU3bqfvxVh8jdoUYda/NXRPrFWrr0euUHLHNRi4HSNoYMJ+Gct5M1iY+Cm20xSNqw34FVbiHIWx4YOw0FeEpVS34zGyCYuLQmwlWcxS0qgUYS43QpVARu25HkBZe0tYg2lwExajqjHXMXErC7EWY3NDMM37Ixb/cre5oq2vaKEyedrkYg3GMqNK1W8xN5nK07f4XNt8USJBVmB58+t0jFNgljEy0Xa5z3t7JxJPSgjxL8BYjIlHhlSJ5Zm5aMHc9FuY9ffGcuOrsdMV+wIjAp94NLEqhM+IN1TLxR6cl+j4YEUaiBP68k3Z8LjiQGYTE5au2NHJ4xMLmMLyslMwF/4ssVWe7gNqTHw2YC53HBaD8sWrfFjnfUfKeJYLJtl3M+Zyr8DIRnOOwALgEizXjciI+CJeizFufPxTMVe8LUFG23gR5gN/oqM3HE7+Pd3BxIRvPWbB9wBvJPtPY27lGL/Qj1BWI7ocIxpd1aT+EeauAmAghJ1AZuHF8xBOXV9X80DRCwvGY6bfC6PQj2KMboEP8mR/z2WYlZwIfBvL6R4C7sPO+xRjFvHPGIE6GtPW3BQhH7KYFZ6AKdIo7/sBjBh0xRLzf8C8x2FYWpTrCntjrPs7GMEpxljjMGLOMB34X7ZvjSEWVyMcjbH2N3xND8QsrCrRRjECeQ9GdCYAz2AW+nXMzUZ9r0/nvKAG0ShmHO7XDnaBrHUB9Qc5xk8HnIu514Oas3rFc0f1+fdTZy27DavWlGG+/Pw8E7sOS8pvwpLbYcBRPrgmX6jSRPs22KnC+zLv+15fhFFY7tdEx1qter+RZ4gE0oCdLTrWhX5qnnfUY+eX1mLWkhRmPsG+BryHWd4+WChZgXmNqI67gDhGdsEU+Rv+942YwrVgShYRvzeASalxNd27Ywl0iS/e82KdR8cgK8Ryrr54TBPoInC/mjsc7u0O7lucbqsuLb7v2VUb3ic+9tjZ7yvGMGf6AryPxZeZvhDdiNljkQtvKWZN1/kkwSz9CMwdzsUS6VyyES3025gl9fB+S11JWjEF/rULfLUv5smYhtdjVScwT1KamEMDRlKuxSwyKrFFKVQzFltn0NHK12GerQ9meaU+5xIsdN2AhZxqzP0/gRUNuvrYSrE42h2z4hVYCLkO+FAa62r6okwmzr2uxs6uPO+TaMNOmTdh1ZCoXHS1a9lDxJvMmwQeSwcyYcw7y1e89tmmgVhZq5cvwAKs0pEb76Ly1yGurSkshZmDUexkLNmXuPLU6Pe3daqgBHNdQ1yTQyw1mo2ddo+UoBTLDdMu6A/996HEuWIj5vpn05EACnH5Dl/kJfmHQzdXxH4+tmVYvFxKnM+GvlYN3uYgzLP0duVYhcXZuS4LpKm2pth3/C/2F70qynkq3EZ8rnW6C/N2zHoB6gX+EShSi22RS1BgqcDLxYG8XRQESzXQWUE6+Eyenk8BuwZBVmgRY15RHjhChSPF6pFRgeBY4GSx2umnfq2fwgSBZrGtnjcxTRLgAIWLNod6d1N79t4NreHgpub2nRhWATuLQAAVZmLMDCyW/MD/nu7XOgE/VAu0E4hd3bDQCEUlZtH/hbmriMqnAFHLOwvYhUiNq+lBJ21bH0oqhbnNFBaQPxTbBD4RIy0VwH6gd4OEWFxMYTGtDsgKegciz2DFgY2YRS8V4VERNo5f2LCn57vXQgCa6qoB6anKE2r5F8CfBM7zFOMGLPDbvw+I3ojKJRgdjqj8jEA4W5VVYaodsunOQDexo3LLgWzllMLx/10FqxeGAdkgXBsgd2MF3a7AcLXa3y+xBPQsTPBnotIuwk9UmYO16SZwa3s2WFVc3Erp84vBrHHTzg+pgM+DAKD8pQUECqBTsdwkuvc94BwRrsHIj2LUfK2oNLVtKnlQbHP1+6DTUkEYCbGA3YwOFYjG2gEgOgjlQeKyXQPwYxFeUeVWYIXavxBk0iml6wsFd/llQAdB3t84jrP/fiIqfBNL/qPccCXojSCT1M6rbix8Z86XCx22hMZWjkckoFmaX/d/1Il2QfYBuQ24XJRiLeQSXzrkrdo31tUgkFblIqxYGx25aMWOcNyiyFxBswXL/HJgq9svTbU1CBqEyClqiX50RDL6Ro+HRXikCKnPgpZNKXzNyp7ENvfRGuqqaU+1Sads0XCM6CR3xrPAR2In4F4Q4eOWFtrLukLnZwtWurux3S9MaqrtR3vYmZS0HoDwQ/8fyj6JJiG2fzkLeF3ggxBWicoagYyKomrbOd1eKgh4V2G7goyw4eT+IKSy2WCYKmOxnfOqnGbRFyKtwvb4MkCDKj8HPi4IctdhhwUZIXPiABCKVPQYrGAwEtu3TG3lkRVqO+zvdSsQo12Gnf6az4ppCwBaG+qqXw3gTVUGgXwL2+qKDimXYhuidgJOaN7TE93bsdMWmYvG2v4oSkDQGZUqFQZiO919sIL6OkQfABoqC19Ntsvw/6KdQFWbRggUAAAAJXRFWHRkYXRlOmNyZWF0ZQAyMDI0LTA4LTI5VDIyOjQ3OjQ2KzAwOjAwZHZUswAAACV0RVh0ZGF0ZTptb2RpZnkAMjAyNC0wOC0yOVQyMjo0Nzo0NiswMDowMBUr7A8AAAAASUVORK5CYII=" alt="Cerebras Systems logo" class=ng-star-inserted></a></div></div><div class=header-text><div class=header-title ng-reflect-ng-class="[object Object]"><h1><a class="job-title ng-star-inserted" ng-reflect-router-link=/jobs/detail/17860b208317feac1 href=https://ecc-uoft-coop-csm.symplicity.com/students/app/jobs/detail/17860b208317feac1449f4953038c9da>ML Developer – Software Automation & Dev Infra Intern</a></h1><span class="header-title-label secondary-text align-self-start body-small flex-shrink-0 hide-sm ng-star-inserted">13d</span></div><div class=header-subtitle><div class=header-subtitle-content><div ng-reflect-ng-class></div><div class="header-info ng-star-inserted"><span class=ng-star-inserted><a ng-reflect-router-link=/employers/bc997a3059404ec36af ng-reflect-ng-class="text-base font-size-base" class="text-base font-size-base" href=https://ecc-uoft-coop-csm.symplicity.com/students/app/employers/bc997a3059404ec36afefcff4c8866b1>Cerebras Systems</a></span><span class=ng-star-inserted><span ng-reflect-ng-class=body-small class=body-small>Toronto, ON, Canada ― Hybrid</span></span><span class=ng-star-inserted><span ng-reflect-ng-class="body-small text-truncate block" class="body-small text-truncate block">Co-op</span></span></div><span class="header-title-label body-small secondary-text display-md-none display-lg-none display-desktop-none ng-star-inserted sf-hidden">13d</span></div><div class="align-self-end button-group ng-star-inserted"><button ng-reflect-ng-class="[object Object]" ng-reflect-is-disabled=false class="btn btn_alt-default hide-sm ng-star-inserted"><span class=ng-star-inserted>Save</span></button><button ng-reflect-ng-class="[object Object]" ng-reflect-is-disabled=false class="btn btn_primary hide-sm ng-star-inserted"><span class=ng-star-inserted>Apply</span></button></div></div></div></div><div class="sticky-footer-sm ng-star-inserted sf-hidden"></div></div></div><div class="form-layout form-readonly has-sidebar ng-star-inserted" ng-reflect-ng-class="[object Object]"><div class=form-container><div><div class="form-col no-padding"><div class="margin-lg ng-star-inserted"><h3>Job Description</h3><div class="text-overflow space-top-lg text-gray p-group field-widget-tinymce" ng-reflect-ng-class="[object Object]"><p>Cerebras has developed a radically new chip and system to dramatically accelerate deep learning applications. Our system runs training and inference workloads orders of magnitude faster than contemporary machines, fundamentally changing the way ML researchers work and pursue AI innovation.</p><p>We are innovating at every level of the stack – from chip, to microcode, to power delivery and cooling, to new algorithms and network architectures at the cutting edge of ML research. Our fully-integrated system delivers unprecedented performance because it is built from the ground up for deep learning workloads.</p><p>Cerebras is building a team of exceptional people to work together on big problems. Join us!</p><p><strong>About The Role:</strong><strong><br></strong>As a Machine Developer - Software Automation & Developer Infrastructure Engineer, you will use your knowledge of testing and testability to influence better software design, promote proper engineering practice, bug prevention strategies, testability, scalability, and other advanced quality concepts. The position will play a huge role in the quality of Cerebras software. We are looking for engineers that have a broad set of technical skills and who are ready to tackle the biggest at-scale problems in HW-based deep learning accelerators.<br><br><strong>Responsibilities:</strong></p><p> </p><ul><li>Write scripts to automate testing and create tools to allow easy development of software regression tests</li><li>Help identify weak spots and potential customer pain points and drive the software organization towards customer focused quality metrics</li><li>Implement creative ways to break software and identify potential problems</li><li>Contribute to developing requirements specifications with a focus on developing verification tests</li></ul><p><strong></strong></p><p><strong>Requirements:</strong></p><ul><li>Enrolled within University of Toronto's PEY program with a degree in Computer Science, Computer Engineering, or any other related discipline</li><li>Experience in developing automated tests for compute/machine learning or networking systems within a large-scale enterprise environment</li><li>Ability to take responsibility for monitoring product development and usage at all levels with an end goal toward improving product quality</li><li>Strong knowledge of software system design, C++ and Python</li></ul><p><strong></strong></p><p><strong>Preferred requirements:</strong></p><ul><li>Strong software testing experience with a proven track record in scaling highly technical teams</li><li>Knowledge of UNIX/Linux and Windows environments</li><li>Knowledge of neural network architecture and ML/AI deep learning principles</li><li>Prior experience in designing and developing test automation for HW systems involving ASICs or FPGAs</li><li>Prior experience working with live hardware systems and debug tools operating in a real time environment such as networking devices or live computing systems</li></ul></div></div><hr class="no-margin ng-star-inserted"><div class="space-top-lg space-right-lg space-left-lg ng-star-inserted"><h3>Additional Job Details</h3><app-form class="space-top-lg job-details-app-form" ng-reflect-config="[object Object]" ng-reflect-resource="[object Object]"><div class="form form-readonly" ng-reflect-ng-class="[object Object]"><form novalidate class="dynamic-form ng-untouched ng-pristine ng-valid" ng-reflect-form="[object Object]"><div class="buttonbar buttonbar-top ng-star-inserted"></div><div class=ng-star-inserted><div class="fieldgroup ng-star-inserted"><form-static class=ng-star-inserted><div class="field form-static-list space-bottom-xs ng-untouched ng-pristine ng-valid ng-star-inserted" ng-reflect-form="[object Object]" ng-reflect-ng-class="[object Object]"><div class="field-label field-label-readonly ng-star-inserted" ng-reflect-ng-class="[object Object]"><span> Position Type </span></div><div class="field-widget widget-readonly field-widget-text" ng-reflect-ng-class="[object Object]"><span class="chip chip-disabled chip-sm ng-star-inserted" ng-reflect-ng-class="[object Object]"><span class="chip-value text-base">Co-op</span></span></div></div></form-static><form-static class=ng-star-inserted><div class="field form-static-list ng-untouched ng-pristine ng-valid ng-star-inserted" ng-reflect-form="[object Object]" ng-reflect-ng-class="[object Object]"><div class="field-label field-label-readonly ng-star-inserted" ng-reflect-ng-class="[object Object]"><span> Compensation </span></div><div class="field-widget widget-readonly field-widget-text" ng-reflect-ng-class="[object Object]"><p id=sy_formfield_compensation_CAL1726540308811 class=ng-star-inserted>$44 per hour</p></div></div></form-static><form-static class=ng-star-inserted><div class="field form-static-list ng-untouched ng-pristine ng-valid ng-star-inserted" ng-reflect-form="[object Object]" ng-reflect-ng-class="[object Object]"><div class="field-label field-label-readonly ng-star-inserted" ng-reflect-ng-class="[object Object]"><span> Remote/On-Site </span></div><div class="field-widget widget-readonly field-widget-text" ng-reflect-ng-class="[object Object]"><p id=sy_formfield_symp_remote_onsite_PWF1726540308812 class=ng-star-inserted>Hybrid</p></div></div></form-static><form-static class=ng-star-inserted><div class="field form-static-list ng-untouched ng-pristine ng-valid ng-star-inserted" ng-reflect-form="[object Object]" ng-reflect-ng-class="[object Object]"><div class="field-label field-label-readonly ng-star-inserted" ng-reflect-ng-class="[object Object]"><span> Job Location </span></div><div class="field-widget widget-readonly field-widget-text" ng-reflect-ng-class="[object Object]"><p id=sy_formfield_location_DPU1726540308812 class=ng-star-inserted>Toronto, ON, Canada</p></div></div></form-static><form-static class=ng-star-inserted><div class="field form-static-list space-bottom-xs ng-untouched ng-pristine ng-valid ng-star-inserted" ng-reflect-form="[object Object]" ng-reflect-ng-class="[object Object]"><div class="field-label field-label-readonly ng-star-inserted" ng-reflect-ng-class="[object Object]"><span> Work Term </span></div><div class="field-widget widget-readonly field-widget-text" ng-reflect-ng-class="[object Object]"><span class="chip chip-disabled chip-sm ng-star-inserted" ng-reflect-ng-class="[object Object]"><span class="chip-value text-base">2025 Fall</span></span></div></div></form-static><form-static class=ng-star-inserted><div class="field form-static-list ng-untouched ng-pristine ng-valid ng-star-inserted" ng-reflect-form="[object Object]" ng-reflect-ng-class="[object Object]"><div class="field-label field-label-readonly ng-star-inserted" ng-reflect-ng-class="[object Object]"><span> Job ID </span></div><div class="field-widget widget-readonly field-widget-text" ng-reflect-ng-class="[object Object]"><p id=sy_formfield_visual_id_JRU1726540308813 class=ng-star-inserted>18344</p></div></div></form-static><form-static class=ng-star-inserted><div class="field form-static-list space-bottom-xs ng-untouched ng-pristine ng-valid ng-star-inserted" ng-reflect-form="[object Object]" ng-reflect-ng-class="[object Object]"><div class="field-label field-label-readonly ng-star-inserted" ng-reflect-ng-class="[object Object]"><span> Contact </span></div><div class="field-widget widget-readonly field-widget-text" ng-reflect-ng-class="[object Object]"><span class="chip chip-disabled chip-sm ng-star-inserted" ng-reflect-ng-class="[object Object]"><span class="chip-value text-base">Winnie Ai</span></span></div></div></form-static><form-static class=ng-star-inserted><div class="field form-static-list ng-untouched ng-pristine ng-valid ng-star-inserted" ng-reflect-form="[object Object]" ng-reflect-ng-class="[object Object]"><div class="field-label field-label-readonly ng-star-inserted" ng-reflect-ng-class="[object Object]"><span> Required Qualifications </span></div><div class="field-widget widget-readonly field-widget-text" ng-reflect-ng-class="[object Object]"><p id=sy_formfield_req_qualifications_ARS1726540308814 ng-reflect-ng-style="[object Object]" style=white-space:pre-wrap class=ng-star-inserted>Requirements:
• Enrolled within University of Toronto's PEY program with a degree in Computer Science, Computer Engineering, or any other related discipline• Experience in developing automated tests for compute/machine learning or networking systems within a large-scale enterprise environment• Ability to take responsibility for monitoring product development and usage at all levels with an end goal toward improving product quality• Strong knowledge of software system design, C++ and Python</p></div></div></form-static><form-static class=ng-star-inserted><div class="field form-static-list ng-untouched ng-pristine ng-valid ng-star-inserted" ng-reflect-form="[object Object]" ng-reflect-ng-class="[object Object]"><div class="field-label field-label-readonly ng-star-inserted" ng-reflect-ng-class="[object Object]"><span> Preferred Program Clusters </span></div><div class="field-widget widget-readonly field-widget-text" ng-reflect-ng-class="[object Object]"><p id=sy_formfield_preferred_program_clusters_NFS1726540308814 class=ng-star-inserted>Math, Statistics, Economics & Related Programs/Math, Data & Statistical Sciences<br>Engineering & Related Programs/Engineering Science<br>Engineering & Related Programs/Electrical and Computer Engineering<br>Computing, Information Technology & Related Programs/Computer Sciences<br>Computing, Information Technology & Related Programs/Information Technology</p></div></div></form-static><form-static class=ng-star-inserted><div class="field form-static-list ng-untouched ng-pristine ng-valid ng-star-inserted" ng-reflect-form="[object Object]" ng-reflect-ng-class="[object Object]"><div class="field-label field-label-readonly ng-star-inserted" ng-reflect-ng-class="[object Object]"><span> Number of Openings </span></div><div class="field-widget widget-readonly field-widget-text" ng-reflect-ng-class="[object Object]"><p id=sy_formfield_num_openings_DYF1726540308815 class=ng-star-inserted>1</p></div></div></form-static><form-static class=ng-star-inserted><div class="field form-static-list last ng-untouched ng-pristine ng-valid ng-star-inserted" ng-reflect-form="[object Object]" ng-reflect-ng-class="[object Object]"><div class="field-label field-label-readonly ng-star-inserted" ng-reflect-ng-class="[object Object]"><span> Approximate Hours Per Week </span></div><div class="field-widget widget-readonly field-widget-text" ng-reflect-ng-class="[object Object]"><p id=sy_formfield_hours_per_week_GGU1726540308815 class=ng-star-inserted>40</p></div></div></form-static></div></div><div class="buttonbar buttonbar-bottom ng-star-inserted"></div></form></div></app-form></div><hr class="no-margin ng-star-inserted"><div class="space-top-lg space-right-lg space-left-lg ng-star-inserted"><h3>Application Process</h3><app-form ng-reflect-config="[object Object]" ng-reflect-resource="[object Object]"><div class="form form-readonly" ng-reflect-ng-class="[object Object]"><form novalidate class="dynamic-form ng-untouched ng-pristine ng-valid" ng-reflect-form="[object Object]"><div class="buttonbar buttonbar-top ng-star-inserted"></div><div class=ng-star-inserted><div class="fieldgroup ng-star-inserted"><form-static class=ng-star-inserted><div class="field form-static-list last ng-untouched ng-pristine ng-valid ng-star-inserted" ng-reflect-form="[object Object]" ng-reflect-ng-class="[object Object]"><div class="field-label field-label-readonly ng-star-inserted" ng-reflect-ng-class="[object Object]"><span> Application Deadline </span></div><div class="field-widget widget-readonly field-widget-text" ng-reflect-ng-class="[object Object]"><p id=sy_formfield_job_deadline class=ng-star-inserted>October 14, 2024</p></div></div></form-static></div></div><div class="buttonbar buttonbar-bottom ng-star-inserted"></div></form></div></app-form></div></div></div><aside role=complementary class="sidebar sidebar-right ng-star-inserted"><div class="sidebar-content padding-bottom-sm font-weight-light ng-star-inserted"><div class="sidebar-inner sb"><div class="sidebar-header row"><div role=heading class="sidebar-title col col-12"> About this employer </div></div><div class="text-and-button space-bottom-sm"><h3 class="space-right-sm text-overflow">Cerebras Systems</h3><button aria-label=Follow class="btn btn_alt-default ng-star-inserted"><i ng-reflect-ng-class></i><span class=ng-star-inserted>Follow</span></button></div><div class="sidebar-body text-truncate-3 space-bottom-md">Cerebras has developed a radically new chip and system to dramatically accelerate deep learning applications. Our system runs training and inference workloads orders of magnitude faster than contemporary machines, fundamentally changing the way ML researchers work and pursue AI innovation.<br><br>We are innovating at every level of the stack – from chip, to microcode, to power delivery and cooling, to new algorithms and network architectures at the cutting edge of ML research. Our fully-integrated system delivers unprecedented performance because it is built from the ground up for deep learning workloads.<br><br>Cerebras' current customers include national labs, global corporations across multiple industries, and top-tier healthcare systems. In January, we announced a multi-year, multi-million-dollar partnership with Mayo Clinic, underscoring our commitment to transforming AI applications across various fields. </div><div class="flexbox space-bottom-sm ng-star-inserted"><span class="icn-location_pin icon-size-sm line-height-sm secondary-text space-right-sm"></span><span class="text-overflow ng-star-inserted">199 Bay St. Suite 4400, Toronto, ONToronto, Ontario M5L 1G9Canada</span></div><hr class="space-top-lg space-bottom-sm neg-margin-x-lg ng-star-inserted"><a class="block text-center font-size-base text-capitalize ng-star-inserted" ng-reflect-router-link=/employers/bc997a3059404ec36af href=https://ecc-uoft-coop-csm.symplicity.com/students/app/employers/bc997a3059404ec36afefcff4c8866b1>View full profile</a></div></div></aside></div></div></jobs-detail></div></div></div></main><footer page-footer role=contentinfo class=ng-star-inserted><div class="page-footer space-bottom-md"><div class=footer-text></div></div><div class="page-footer ng-star-inserted"><div class=footer-image><a href=http://symplicity.com/career_services_manager_csm target=_blank><img src="data:image/svg+xml;base64,PD94bWwgdmVyc2lvbj0iMS4wIiBlbmNvZGluZz0iVVRGLTgiPz4KPHN2ZyB3aWR0aD0iMjAycHgiIGhlaWdodD0iMTVweCIgdmlld0JveD0iMCAwIDIwMiAxNSIgdmVyc2lvbj0iMS4xIiB4bWxucz0iaHR0cDovL3d3dy53My5vcmcvMjAwMC9zdmciIHhtbG5zOnhsaW5rPSJodHRwOi8vd3d3LnczLm9yZy8xOTk5L3hsaW5rIj4KICAgIDwhLS0gR2VuZXJhdG9yOiBTa2V0Y2ggNTQuMSAoNzY0OTApIC0gaHR0cHM6Ly9za2V0Y2hhcHAuY29tIC0tPgogICAgPHRpdGxlPnBvd2VyZWQtYnktc3ltcGxpY2l0eV9hbGwtY2FwczwvdGl0bGU+CiAgICA8ZGVzYz5DcmVhdGVkIHdpdGggU2tldGNoLjwvZGVzYz4KICAgIDxnIGlkPSJGb3JtYXR0ZWQtRW1haWwiIHN0cm9rZT0ibm9uZSIgc3Ryb2tlLXdpZHRoPSIxIiBmaWxsPSJub25lIiBmaWxsLXJ1bGU9ImV2ZW5vZGQiPgogICAgICAgIDxnIGlkPSJFbWFpbCIgdHJhbnNmb3JtPSJ0cmFuc2xhdGUoLTUzOS4wMDAwMDAsIC0xNTY1LjAwMDAwMCkiPgogICAgICAgICAgICA8ZyBpZD0icG93ZXJlZC1ieS1zeW1wbGljaXR5X2FsbC1jYXBzIiB0cmFuc2Zvcm09InRyYW5zbGF0ZSg1MzkuMDAwMDAwLCAxNTY1LjAwMDAwMCkiPgogICAgICAgICAgICAgICAgPHBhdGggZD0iTTIuODIyMTQyODYsOC4wMzgzOTI4NiBDNC4xMjEyNSw4LjAzODM5Mjg2IDQuOTcyMzY2MDcsNy4zNDM5NzMyMSA0Ljk3MjM2NjA3LDYuMzEzNjYwNzEgTDQuOTcyMzY2MDcsNi4yOTEyOTQ2NCBDNC45NzIzNjYwNyw1LjE3MTM4MzkzIDQuMTMyMzY2MDcsNC41ODkwNjI1IDIuODY2ODc1LDQuNTg5MDYyNSBMMC44ODQ1OTgyMTQsNC41ODkwNjI1IEwwLjg4NDU5ODIxNCw4LjAzODM5Mjg2IEwyLjgyMjE0Mjg2LDguMDM4MzkyODYgWiBNMCwzLjc3MTU2MjUgTDIuOTM0MTA3MTQsMy43NzE1NjI1IEM0LjcwMzU3MTQzLDMuNzcxNTYyNSA1Ljg2ODIxNDI5LDQuNzEyMjc2NzkgNS44NjgyMTQyOSw2LjI1NzY3ODU3IEw1Ljg2ODIxNDI5LDYuMjgwMDQ0NjQgQzUuODY4MjE0MjksNy45NzExNjA3MSA0LjQ1NzE0Mjg2LDguODQ0NjQyODYgMi43ODg1MjY3OSw4Ljg0NDY0Mjg2IEwwLjg4NDU5ODIxNCw4Ljg0NDY0Mjg2IEwwLjg4NDU5ODIxNCwxMS42MTA4MDM2IEwwLDExLjYxMDgwMzYgTDAsMy43NzE1NjI1IEwwLDMuNzcxNTYyNSBaIiBpZD0iRmlsbC0xIiBmaWxsPSIjOTk5OTk5Ij48L3BhdGg+CiAgICAgICAgICAgICAgICA8cGF0aCBkPSJNMTQuMjExMTYwNyw3LjcxMzQ4MjE0IEwxNC4yMTExNjA3LDcuNjkxMTE2MDcgQzE0LjIxMTE2MDcsNS45MTA1MzU3MSAxMi45MTIxODc1LDQuNDU0NTk4MjEgMTEuMTIwMzU3MSw0LjQ1NDU5ODIxIEM5LjMyODUyNjc5LDQuNDU0NTk4MjEgOC4wNTE3ODU3MSw1Ljg4ODE2OTY0IDguMDUxNzg1NzEsNy42Njg3NSBMOC4wNTE3ODU3MSw3LjY5MTExNjA3IEM4LjA1MTc4NTcxLDkuNDcxODMwMzYgOS4zNTA4OTI4NiwxMC45Mjc2MzM5IDExLjE0MjcyMzIsMTAuOTI3NjMzOSBDMTIuOTM0NTUzNiwxMC45Mjc2MzM5IDE0LjIxMTE2MDcsOS40OTQxOTY0MyAxNC4yMTExNjA3LDcuNzEzNDgyMTQgWiBNNy4xMzM1NzE0Myw3LjcxMzQ4MjE0IEw3LjEzMzU3MTQzLDcuNjkxMTE2MDcgQzcuMTMzNTcxNDMsNS41Mjk3NzY3OSA4Ljc1NzMyMTQzLDMuNjM3MjMyMTQgMTEuMTQyNzIzMiwzLjYzNzIzMjE0IEMxMy41MjgxMjUsMy42MzcyMzIxNCAxNS4xMjk1MDg5LDUuNTA3NDEwNzEgMTUuMTI5NTA4OSw3LjY2ODc1IEwxNS4xMjk1MDg5LDcuNjkxMTE2MDcgQzE1LjEyOTUwODksOS44NTI1ODkyOSAxMy41MDU2MjUsMTEuNzQ1MjY3OSAxMS4xMjAzNTcxLDExLjc0NTI2NzkgQzguNzM0OTU1MzYsMTEuNzQ1MjY3OSA3LjEzMzU3MTQzLDkuODc0OTU1MzYgNy4xMzM1NzE0Myw3LjcxMzQ4MjE0IEw3LjEzMzU3MTQzLDcuNzEzNDgyMTQgWiIgaWQ9IkZpbGwtMiIgZmlsbD0iIzk5OTk5OSI+PC9wYXRoPgogICAgICAgICAgICAgICAgPHBvbHlsaW5lIGlkPSJGaWxsLTMiIGZpbGw9IiM5OTk5OTkiIHBvaW50cz0iMTUuOTkxNjA3MSAzLjc3MTU2MjUgMTYuOTU0ODIxNCAzLjc3MTU2MjUgMTkuMTk0NTA4OSAxMC4zMDA1ODA0IDIxLjM0NDczMjEgMy43NDkwNjI1IDIyLjA3MjYzMzkgMy43NDkwNjI1IDI0LjIyMjg1NzEgMTAuMzAwNTgwNCAyNi40NjI1NDQ2IDMuNzcxNTYyNSAyNy4zOTIxNDI5IDMuNzcxNTYyNSAyNC41OTIzNjYxIDExLjY2Njc4NTcgMjMuODQyMDk4MiAxMS42NjY3ODU3IDIxLjY5MTg3NSA1LjMwNTg0ODIxIDE5LjUzMDUzNTcgMTEuNjY2Nzg1NyAxOC43OTEzODM5IDExLjY2Njc4NTcgMTUuOTkxNjA3MSAzLjc3MTU2MjUiPjwvcG9seWxpbmU+CiAgICAgICAgICAgICAgICA8cG9seWxpbmUgaWQ9IkZpbGwtNCIgZmlsbD0iIzk5OTk5OSIgcG9pbnRzPSIyOC45OTMzOTI5IDMuNzcxNTYyNSAzNC42NTk5MTA3IDMuNzcxNTYyNSAzNC42NTk5MTA3IDQuNTc3ODEyNSAyOS44NzgxMjUgNC41Nzc4MTI1IDI5Ljg3ODEyNSA3LjI1NDM3NSAzNC4xNTYwNzE0IDcuMjU0Mzc1IDM0LjE1NjA3MTQgOC4wNjA3NTg5MyAyOS44NzgxMjUgOC4wNjA3NTg5MyAyOS44NzgxMjUgMTAuODA0NDE5NiAzNC43MTYwMjY4IDEwLjgwNDQxOTYgMzQuNzE2MDI2OCAxMS42MTA4MDM2IDI4Ljk5MzM5MjkgMTEuNjEwODAzNiAyOC45OTMzOTI5IDMuNzcxNTYyNSI+PC9wb2x5bGluZT4KICAgICAgICAgICAgICAgIDxwYXRoIGQ9Ik0zOS43ODg5NzMyLDcuNzY5NDY0MjkgQzQwLjk2NDg2NjEsNy43Njk0NjQyOSA0MS44MDQ4NjYxLDcuMTY0Nzc2NzkgNDEuODA0ODY2MSw2LjE1NjgzMDM2IEw0MS44MDQ4NjYxLDYuMTM0NDY0MjkgQzQxLjgwNDg2NjEsNS4xNzEzODM5MyA0MS4wNjU3MTQzLDQuNTg5MDYyNSAzOS44MDAyMjMyLDQuNTg5MDYyNSBMMzcuMzgxMjA1NCw0LjU4OTA2MjUgTDM3LjM4MTIwNTQsNy43Njk0NjQyOSBMMzkuNzg4OTczMiw3Ljc2OTQ2NDI5IFogTTM2LjQ5NjQ3MzIsMy43NzE1NjI1IEwzOS44NjczMjE0LDMuNzcxNTYyNSBDNDAuODMwNDAxOCwzLjc3MTU2MjUgNDEuNjAzMTY5Niw0LjA2MjcyMzIxIDQyLjA5NTg5MjksNC41NTU0NDY0MyBDNDIuNDc2NjUxOCw0LjkzNjIwNTM2IDQyLjcwMDcxNDMsNS40ODQ5MTA3MSA0Mi43MDA3MTQzLDYuMTAwODQ4MjEgTDQyLjcwMDcxNDMsNi4xMjMzNDgyMSBDNDIuNzAwNzE0Myw3LjQyMjMyMTQzIDQxLjgwNDg2NjEsOC4xODM5NzMyMSA0MC41NzI4NTcxLDguNDA3OTAxNzkgTDQyLjk4MDYyNSwxMS42MTA4MDM2IEw0MS44OTQ0NjQzLDExLjYxMDgwMzYgTDM5LjYyMTAyNjgsOC41NjQ3MzIxNCBMMzcuMzgxMjA1NCw4LjU2NDczMjE0IEwzNy4zODEyMDU0LDExLjYxMDgwMzYgTDM2LjQ5NjQ3MzIsMTEuNjEwODAzNiBMMzYuNDk2NDczMiwzLjc3MTU2MjUgTDM2LjQ5NjQ3MzIsMy43NzE1NjI1IFoiIGlkPSJGaWxsLTUiIGZpbGw9IiM5OTk5OTkiPjwvcGF0aD4KICAgICAgICAgICAgICAgIDxwb2x5bGluZSBpZD0iRmlsbC02IiBmaWxsPSIjOTk5OTk5IiBwb2ludHM9IjQ0LjU5MzEyNSAzLjc3MTU2MjUgNTAuMjU5NjQyOSAzLjc3MTU2MjUgNTAuMjU5NjQyOSA0LjU3NzgxMjUgNDUuNDc3NzIzMiA0LjU3NzgxMjUgNDUuNDc3NzIzMiA3LjI1NDM3NSA0OS43NTU4MDM2IDcuMjU0Mzc1IDQ5Ljc1NTgwMzYgOC4wNjA3NTg5MyA0NS40Nzc3MjMyIDguMDYwNzU4OTMgNDUuNDc3NzIzMiAxMC44MDQ0MTk2IDUwLjMxNTYyNSAxMC44MDQ0MTk2IDUwLjMxNTYyNSAxMS42MTA4MDM2IDQ0LjU5MzEyNSAxMS42MTA4MDM2IDQ0LjU5MzEyNSAzLjc3MTU2MjUiPjwvcG9seWxpbmU+CiAgICAgICAgICAgICAgICA8cGF0aCBkPSJNNTIuOTgwOTM3NSw0LjU4OTA2MjUgTDUyLjk4MDkzNzUsMTAuNzkzMTY5NiBMNTQuODE3NjMzOSwxMC43OTMxNjk2IEM1Ni43OTk2NDI5LDEwLjc5MzE2OTYgNTguMDY1MjY3OSw5LjQ0OTMzMDM2IDU4LjA2NTI2NzksNy43MTM0ODIxNCBMNTguMDY1MjY3OSw3LjY5MTExNjA3IEM1OC4wNjUyNjc5LDUuOTU1NDAxNzkgNTYuNzk5NjQyOSw0LjU4OTA2MjUgNTQuODE3NjMzOSw0LjU4OTA2MjUgTDUyLjk4MDkzNzUsNC41ODkwNjI1IFogTTUyLjA5NjIwNTQsMy43NzE1NjI1IEw1NC44MTc2MzM5LDMuNzcxNTYyNSBDNTcuMjgxMjUsMy43NzE1NjI1IDU4Ljk4MzQ4MjEsNS40NjI1NDQ2NCA1OC45ODM0ODIxLDcuNjY4NzUgTDU4Ljk4MzQ4MjEsNy42OTExMTYwNyBDNTguOTgzNDgyMSw5Ljg5NzMyMTQzIDU3LjI4MTI1LDExLjYxMDgwMzYgNTQuODE3NjMzOSwxMS42MTA4MDM2IEw1Mi4wOTYyMDU0LDExLjYxMDgwMzYgTDUyLjA5NjIwNTQsMy43NzE1NjI1IEw1Mi4wOTYyMDU0LDMuNzcxNTYyNSBaIiBpZD0iRmlsbC03IiBmaWxsPSIjOTk5OTk5Ij48L3BhdGg+CiAgICAgICAgICAgICAgICA8cGF0aCBkPSJNNjcuNTYxNDczMiw4LjA0OTUwODkzIEw2NS4wODY0NzMyLDguMDQ5NTA4OTMgTDY1LjA4NjQ3MzIsMTAuODE1NjY5NiBMNjcuNzE4MzAzNiwxMC44MTU2Njk2IEM2OC45MDUzMTI1LDEwLjgxNTY2OTYgNjkuNjQ0NDY0MywxMC4yODkxOTY0IDY5LjY0NDQ2NDMsOS40MTU4NDgyMSBMNjkuNjQ0NDY0Myw5LjM5MzM0ODIxIEM2OS42NDQ0NjQzLDguNTQyMzY2MDcgNjguOTI3Njc4Niw4LjA0OTUwODkzIDY3LjU2MTQ3MzIsOC4wNDk1MDg5MyBaIE02OS4yMDc3MjMyLDUuODQzMzAzNTcgQzY5LjIwNzcyMzIsNS4wNzA2Njk2NCA2OC41OTE2NTE4LDQuNTY2Njk2NDMgNjcuNDcxODc1LDQuNTY2Njk2NDMgTDY1LjA4NjQ3MzIsNC41NjY2OTY0MyBMNjUuMDg2NDczMiw3LjI2NTYyNSBMNjcuNDA0NjQyOSw3LjI2NTYyNSBDNjguNDY4NDM3NSw3LjI2NTYyNSA2OS4yMDc3MjMyLDYuNzg0MDE3ODYgNjkuMjA3NzIzMiw1Ljg2NTgwMzU3IEw2OS4yMDc3MjMyLDUuODQzMzAzNTcgWiBNNjQuMjEyOTkxMSwzLjc3MTU2MjUgTDY3LjU1MDIyMzIsMy43NzE1NjI1IEM2OC40NDYwNzE0LDMuNzcxNTYyNSA2OS4xNTE3NDExLDQuMDI5MTA3MTQgNjkuNTk5NTk4Miw0LjQ2NTg0ODIxIEM2OS45MjQ1MDg5LDQuODAxODc1IDcwLjEwMzU3MTQsNS4yMTYyNSA3MC4xMDM1NzE0LDUuNzIwMDg5MjkgTDcwLjEwMzU3MTQsNS43NDI1ODkyOSBDNzAuMTAzNTcxNCw2Ljc2MTY1MTc5IDY5LjQ3NjUxNzksNy4yODc5OTEwNyA2OC44NjA0NDY0LDcuNTU2Nzg1NzEgQzY5Ljc5MDA0NDYsNy44MzY2OTY0MyA3MC41NDAzMTI1LDguMzc0Mjg1NzEgNzAuNTQwMzEyNSw5LjQ0OTMzMDM2IEw3MC41NDAzMTI1LDkuNDcxODMwMzYgQzcwLjU0MDMxMjUsMTAuODE1NjY5NiA2OS40MDkyODU3LDExLjYxMDgwMzYgNjcuNjk1ODAzNiwxMS42MTA4MDM2IEw2NC4yMTI5OTExLDExLjYxMDgwMzYgTDY0LjIxMjk5MTEsMy43NzE1NjI1IEw2NC4yMTI5OTExLDMuNzcxNTYyNSBaIiBpZD0iRmlsbC04IiBmaWxsPSIjOTk5OTk5Ij48L3BhdGg+CiAgICAgICAgICAgICAgICA8cG9seWxpbmUgaWQ9IkZpbGwtOSIgZmlsbD0iIzk5OTk5OSIgcG9pbnRzPSI3NC40MTUgOC41MDg3NSA3MS4xNTYxMTYxIDMuNzcxNTYyNSA3Mi4yMzExNjA3IDMuNzcxNTYyNSA3NC44NzQxMDcxIDcuNjkxMTE2MDcgNzcuNTM5NDE5NiAzLjc3MTU2MjUgNzguNTY5NzMyMSAzLjc3MTU2MjUgNzUuMzEwODQ4MiA4LjQ5NzUgNzUuMzEwODQ4MiAxMS42MTA4MDM2IDc0LjQxNSAxMS42MTA4MDM2IDc0LjQxNSA4LjUwODc1Ij48L3BvbHlsaW5lPgogICAgICAgICAgICAgICAgPHBhdGggZD0iTTk3Ljc5NTA0NDYsMTAuMDcxOTY0MyBDOTguMzc4NTcxNCwxMC40MjQ1OTgyIDk4Ljk5MjUsMTAuNjU0ODIxNCA5OS42MzcwOTgyLDEwLjY1NDgyMTQgQzEwMC42MiwxMC42NTQ4MjE0IDEwMS4xMjY1MTgsMTAuMTQ4NDM3NSAxMDEuMTI2NTE4LDkuNTQ5NTA4OTMgQzEwMS4xMjY1MTgsOS4wMTI0NTUzNiAxMDAuODUwMjIzLDguNjQzNzUgMTAwLjEyODYxNiw4LjMyMDg0ODIxIEw5OS4yNjg3OTQ2LDcuOTM3MTQyODYgQzk4LjE0NzY3ODYsNy40MzA4OTI4NiA5Ny43MzMxNjk2LDYuOTIzODM5MjkgOTcuNzMzMTY5Niw1LjkyNjIwNTM2IEM5Ny43MzMxNjk2LDQuNjM2NDczMjEgOTguNzMxMzM5MywzLjY1MzU3MTQzIDEwMC4yNTE1NjIsMy42NTM1NzE0MyBDMTAwLjg5NjI5NSwzLjY1MzU3MTQzIDEwMS40NjQ0MiwzLjc2MTM4MzkzIDEwMS45MDk0NjQsMy45MzA1MzU3MSBMMTAxLjg0ODEyNSw1LjAzNTE3ODU3IEMxMDEuNDQ5MDE4LDQuODUxNTYyNSAxMDAuODk2Mjk1LDQuNjk3OTQ2NDMgMTAwLjMxMjkwMiw0LjY5Nzk0NjQzIEM5OS40NjgyMTQzLDQuNjk3OTQ2NDMgOTkuMDIzMTY5Niw1LjE1ODY2MDcxIDk5LjAyMzE2OTYsNS43NzI1ODkyOSBDOTkuMDIzMTY5Niw2LjQwMTY1MTc5IDk5LjQ4Mzc1LDYuNzU1MDg5MjkgMTAwLjM0MzcwNSw3LjA5MzEyNSBMMTAwLjgwNDI4Niw3LjI3NzQxMDcxIEMxMDEuNzU1OTgyLDcuNjYxMTE2MDcgMTAyLjM1NDkxMSw4LjMwNTk4MjE0IDEwMi4zNTQ5MTEsOS4yNzM3NSBDMTAyLjM1NDkxMSwxMC42MjQ0MTk2IDEwMS42MzM0MzcsMTEuNjk5MDYyNSA5OS44MzY5MTk2LDExLjY5OTA2MjUgQzk5LjAyMzE2OTYsMTEuNjk5MDYyNSA5OC4yODYxNjA3LDExLjUyOTkxMDcgOTcuNzE4MTY5NiwxMS4yMzg4ODM5IEw5Ny43OTUwNDQ2LDEwLjA3MTk2NDMiIGlkPSJGaWxsLTEwIiBmaWxsPSIjOTk5OTk5Ij48L3BhdGg+CiAgICAgICAgICAgICAgICA8cGF0aCBkPSJNMTEwLjg3NDI0MSwxMy4xODgzNDgyIEMxMTAuNDkwMTM0LDE0LjUyNDU1MzYgMTA5LjkzNzU0NSwxNSAxMDguOTU0OTExLDE1IEMxMDguNjQ3ODEyLDE1IDEwOC4zMTAwNDUsMTQuOTY4OTI4NiAxMDguMDc5ODIxLDE0LjkwODI1ODkgTDEwOC4xNDExNjEsMTMuNzQxMjA1NCBDMTA4LjM0MDg0OCwxMy44MzI5NDY0IDEwOC41NzEwNzEsMTMuODk0NTUzNiAxMDguODQ3MjMyLDEzLjg5NDU1MzYgQzEwOS4yMTU5MzcsMTMuODk0NTUzNiAxMDkuNDkxOTY0LDEzLjcyNTUzNTcgMTA5LjcwNzE4NywxMi45MTIzMjE0IEwxMTAuMDE0MTUyLDExLjcxNDMzMDQgTDEwNy41NDIwOTgsMy43NjEzODM5MyBMMTA4Ljg5MzQzNywzLjc2MTM4MzkzIEwxMTAuNjQzNzUsMTAuMTQ4NDM3NSBMMTEwLjY3NDU1NCwxMC4xNDg0Mzc1IEwxMTIuMzAxOTIsMy43NjEzODM5MyBMMTEzLjU5MTY1MiwzLjc2MTM4MzkzIEwxMTAuODc0MjQxLDEzLjE4ODM0ODIiIGlkPSJGaWxsLTExIiBmaWxsPSIjOTk5OTk5Ij48L3BhdGg+CiAgICAgICAgICAgICAgICA8cGF0aCBkPSJNMTE5LjI3MDYyNSw1LjQ1MDYyNSBDMTE5LjI3MDYyNSw0Ljg4MTgzMDM2IDExOS4yMjQ0Miw0LjMxNDM3NSAxMTkuMjA4ODg0LDMuNzYxMzgzOTMgTDEyMC40NTI2NzksMy43NjEzODM5MyBMMTIwLjQ5OTE1Miw0LjkyODQzNzUgTDEyMC41MjkyODYsNC45Mjg0Mzc1IEMxMjAuOTQzOTI5LDQuMDM3Mjc2NzkgMTIxLjYwNDMzLDMuNjUzNTcxNDMgMTIyLjQ2NDE1MiwzLjY1MzU3MTQzIEMxMjMuMjkzMDM2LDMuNjUzNTcxNDMgMTIzLjkwNzIzMiw0LjA4NDI4NTcxIDEyNC4zMDYzMzksNC44ODE4MzAzNiBDMTI0LjcyMTExNiw0LjExNDI4NTcxIDEyNS4zMjAxNzksMy42NTM1NzE0MyAxMjYuMjEwNTM2LDMuNjUzNTcxNDMgQzEyNy41NjA4MDQsMy42NTM1NzE0MyAxMjguNDgzMDM2LDQuNDM2MzgzOTMgMTI4LjQ4MzAzNiw2LjE1NjQyODU3IEwxMjguNDgzMDM2LDExLjU5MTY1MTggTDEyNy4xOTMwMzYsMTEuNTkxNjUxOCBMMTI3LjE5MzAzNiw2LjIxNzkwMTc5IEMxMjcuMTkzMDM2LDUuMjIwMTMzOTMgMTI2Ljc2MzEyNSw0Ljc1OTE1MTc5IDEyNS45OTUxNzksNC43NTkxNTE3OSBDMTI1LjA0MzYxNiw0Ljc1OTE1MTc5IDEyNC41MjE1NjIsNS4zNDIxNDI4NiAxMjQuNTIxNTYyLDYuNzU1MDg5MjkgTDEyNC41MjE1NjIsMTEuNTkxNjUxOCBMMTIzLjIzMTY5NiwxMS41OTE2NTE4IEwxMjMuMjMxNjk2LDYuMjE3OTAxNzkgQzEyMy4yMzE2OTYsNS4yMjAxMzM5MyAxMjIuODAxNzg2LDQuNzU5MTUxNzkgMTIyLjAzNDI0MSw0Ljc1OTE1MTc5IEMxMjEuMDgyMTQzLDQuNzU5MTUxNzkgMTIwLjU2MDA4OSw1LjM0MjE0Mjg2IDEyMC41NjAwODksNi43NTUwODkyOSBMMTIwLjU2MDA4OSwxMS41OTE2NTE4IEwxMTkuMjcwNjI1LDExLjU5MTY1MTggTDExOS4yNzA2MjUsNS40NTA2MjUiIGlkPSJGaWxsLTEyIiBmaWxsPSIjOTk5OTk5Ij48L3BhdGg+CiAgICAgICAgICAgICAgICA8cGF0aCBkPSJNMTM0LjgzNTI2OCw1LjIwNDQ2NDI5IEMxMzQuODM1MjY4LDQuNzI4MDgwMzYgMTM0Ljc4OTczMiw0LjIzNzYzMzkzIDEzNC43NzUsMy43NjEzODM5MyBMMTM2LjAxNzg1NywzLjc2MTM4MzkzIEwxMzYuMDY0NzMyLDQuOTEzNDM3NSBMMTM2LjA5NDE5Niw0LjkxMzQzNzUgQzEzNi40MzE2OTYsNC4yOTg1NzE0MyAxMzcuMDE1NjI1LDMuNjUzNTcxNDMgMTM4LjE1MTMzOSwzLjY1MzU3MTQzIEMxMzkuNzY1MTc5LDMuNjUzNTcxNDMgMTQwLjg1NDAxOCw0Ljk3NDEwNzE0IDE0MC44NTQwMTgsNy42NzU5ODIxNCBDMTQwLjg1NDAxOCwxMC4zNzg5Mjg2IDEzOS43NjUxNzksMTEuNjk5MDYyNSAxMzguMTUxMzM5LDExLjY5OTA2MjUgQzEzNy4yNjIwNTQsMTEuNjk5MDYyNSAxMzYuNjc4MTI1LDExLjM0NjQyODYgMTM2LjE1NTgwNCwxMC41MTcyNzY4IEwxMzYuMTI1LDEwLjUxNzI3NjggTDEzNi4xMjUsMTQuOTA4MjU4OSBMMTM0LjgzNTI2OCwxNC45MDgyNTg5IEwxMzQuODM1MjY4LDUuMjA0NDY0MjkgWiBNMTM3Ljg0NDY0MywxMC42NTQ4MjE0IEMxMzkuMDI3MjMyLDEwLjY1NDgyMTQgMTM5LjU2NDI4Niw5LjY0MTUxNzg2IDEzOS41NjQyODYsNy42NzU5ODIxNCBDMTM5LjU2NDI4Niw1LjcxMDg0ODIxIDEzOS4wMjcyMzIsNC42OTc5NDY0MyAxMzcuODQ0NjQzLDQuNjk3OTQ2NDMgQzEzNi42NjMzOTMsNC42OTc5NDY0MyAxMzYuMTI1LDUuNzEwODQ4MjEgMTM2LjEyNSw3LjY3NTk4MjE0IEMxMzYuMTI1LDkuNjQxNTE3ODYgMTM2LjY2MzM5MywxMC42NTQ4MjE0IDEzNy44NDQ2NDMsMTAuNjU0ODIxNCBMMTM3Ljg0NDY0MywxMC42NTQ4MjE0IFoiIGlkPSJGaWxsLTEzIiBmaWxsPSIjOTk5OTk5Ij48L3BhdGg+CiAgICAgICAgICAgICAgICA8cG9seWdvbiBpZD0iRmlsbC0xNCIgZmlsbD0iIzk5OTk5OSIgcG9pbnRzPSIxNDcuMDcwOTgyIDExLjU5MTY1MTggMTQ4LjM2MDcxNCAxMS41OTE2NTE4IDE0OC4zNjA3MTQgMC4wNzY4NzUgMTQ3LjA3MDk4MiAwLjA3Njg3NSI+PC9wb2x5Z29uPgogICAgICAgICAgICAgICAgPHBhdGggZD0iTTE1NS4wMjIzMjEsMi4wNzIyNzY3OSBMMTU2LjQzMzkyOSwyLjA3MjI3Njc5IEwxNTYuNDMzOTI5LDAuNTM3MzIxNDI5IEwxNTUuMDIyMzIxLDAuNTM3MzIxNDI5IEwxNTUuMDIyMzIxLDIuMDcyMjc2NzkgWiBNMTU1LjA4MjU4OSwxMS41OTE1MTc5IEwxNTYuMzcyMzIxLDExLjU5MTUxNzkgTDE1Ni4zNzIzMjEsMy43NjEzODM5MyBMMTU1LjA4MjU4OSwzLjc2MTM4MzkzIEwxNTUuMDgyNTg5LDExLjU5MTUxNzkgWiIgaWQ9IkZpbGwtMTUiIGZpbGw9IiM5OTk5OTkiPjwvcGF0aD4KICAgICAgICAgICAgICAgIDxwYXRoIGQ9Ik0xNjcuNTc5NDY0LDUuMTI3NzIzMjEgQzE2Ny4xMzM0ODIsNC44ODE4MzAzNiAxNjYuNjg4ODM5LDQuNzU5MTUxNzkgMTY2LjA5MDE3OSw0Ljc1OTE1MTc5IEMxNjQuODMxMjUsNC43NTkxNTE3OSAxNjMuOTM5Mjg2LDUuNjMzODM5MjkgMTYzLjkzOTI4Niw3Ljc1Mjg1NzE0IEMxNjMuOTM5Mjg2LDkuNTY0Mzc1IDE2NC44MDA0NDYsMTAuNTk0NDE5NiAxNjYuMDU5Mzc1LDEwLjU5NDQxOTYgQzE2Ni42NzI3NjgsMTAuNTk0NDE5NiAxNjcuMTk1MDg5LDEwLjQwOTMzMDQgMTY3LjYzOTczMiwxMC4xNzk1MDg5IEwxNjcuNzAxMzM5LDExLjM2MTY5NjQgQzE2Ny4zMTgzMDQsMTEuNTI5OTEwNyAxNjYuNjI3MjMyLDExLjY5OTA2MjUgMTY2LjA1OTM3NSwxMS42OTkwNjI1IEMxNjMuNzI1LDExLjY5OTA2MjUgMTYyLjU4OTI4Niw5Ljk2NDE1MTc5IDE2Mi41ODkyODYsNy43NTI4NTcxNCBDMTYyLjU4OTI4Niw1LjIyMDEzMzkzIDE2My45MDk4MjEsMy42NTM1NzE0MyAxNjYuMDkwMTc5LDMuNjUzNTcxNDMgQzE2Ni42MjcyMzIsMy42NTM1NzE0MyAxNjcuMjI1ODkzLDMuNzYxMzgzOTMgMTY3LjYzOTczMiwzLjk2MDY2OTY0IEwxNjcuNTc5NDY0LDUuMTI3NzIzMjEiIGlkPSJGaWxsLTE2IiBmaWxsPSIjOTk5OTk5Ij48L3BhdGg+CiAgICAgICAgICAgICAgICA8cGF0aCBkPSJNMTczLjYxMTYwNywyLjA3MjI3Njc5IEwxNzUuMDIzMjE0LDIuMDcyMjc2NzkgTDE3NS4wMjMyMTQsMC41MzczMjE0MjkgTDE3My42MTE2MDcsMC41MzczMjE0MjkgTDE3My42MTE2MDcsMi4wNzIyNzY3OSBaIE0xNzMuNjcxODc1LDExLjU5MTUxNzkgTDE3NC45NjE2MDcsMTEuNTkxNTE3OSBMMTc0Ljk2MTYwNywzLjc2MTM4MzkzIEwxNzMuNjcxODc1LDMuNzYxMzgzOTMgTDE3My42NzE4NzUsMTEuNTkxNTE3OSBaIiBpZD0iRmlsbC0xNyIgZmlsbD0iIzk5OTk5OSI+PC9wYXRoPgogICAgICAgICAgICAgICAgPHBhdGggZD0iTTE4NS4wMTY5NjQsMTEuNDk5Nzc2OCBDMTg0LjcxMDI2OCwxMS42MDY1MTc5IDE4NC4yODAzNTcsMTEuNjk5MDYyNSAxODMuOTI2Nzg2LDExLjY5OTA2MjUgQzE4Mi43NDQxOTYsMTEuNjk5MDYyNSAxODIuMTE0NzMyLDExLjIyMzIxNDMgMTgyLjExNDczMiw5Ljg0MTMzOTI5IEwxODIuMTE0NzMyLDQuODA0OTU1MzYgTDE4MC44NzA1MzYsNC44MDQ5NTUzNiBMMTgwLjg3MDUzNiwzLjc2MTM4MzkzIEwxODIuMTE0NzMyLDMuNzYxMzgzOTMgTDE4Mi4xMTQ3MzIsMi4xNjM4ODM5MyBMMTgzLjQwNDQ2NCwxLjc4MDE3ODU3IEwxODMuNDA0NDY0LDMuNzYxMzgzOTMgTDE4NS4wMTY5NjQsMy43NjEzODM5MyBMMTg1LjAxNjk2NCw0LjgwNDk1NTM2IEwxODMuNDA0NDY0LDQuODA0OTU1MzYgTDE4My40MDQ0NjQsOS40MjcwOTgyMSBDMTgzLjQwNDQ2NCwxMC4zMTczMjE0IDE4My42MzQ4MjEsMTAuNjU0ODIxNCAxODQuMjE4NzUsMTAuNjU0ODIxNCBDMTg0LjU0MTUxOCwxMC42NTQ4MjE0IDE4NC43NzE4NzUsMTAuNTYzMDgwNCAxODUuMDE2OTY0LDEwLjQ1NTUzNTcgTDE4NS4wMTY5NjQsMTEuNDk5Nzc2OCIgaWQ9IkZpbGwtMTgiIGZpbGw9IiM5OTk5OTkiPjwvcGF0aD4KICAgICAgICAgICAgICAgIDxwYXRoIGQ9Ik0xOTMuMTY2NTE4LDEzLjE4ODM0ODIgQzE5Mi43ODM0ODIsMTQuNTI0NTUzNiAxOTIuMjMxNjk2LDE1IDE5MS4yNDg2NjEsMTUgQzE5MC45NDA2MjUsMTUgMTkwLjYwNDQ2NCwxNC45Njg5Mjg2IDE5MC4zNzI3NjgsMTQuOTA4MjU4OSBMMTkwLjQzNTcxNCwxMy43NDEyMDU0IEMxOTAuNjMzOTI5LDEzLjgzMjk0NjQgMTkwLjg2NDI4NiwxMy44OTQ1NTM2IDE5MS4xNDE1MTgsMTMuODk0NTUzNiBDMTkxLjUwODQ4MiwxMy44OTQ1NTM2IDE5MS43ODU3MTQsMTMuNzI1NTM1NyAxOTIsMTIuOTEyMzIxNCBMMTkyLjMwODAzNiwxMS43MTQzMzA0IEwxODkuODM1NzE0LDMuNzYxMzgzOTMgTDE5MS4xODcwNTQsMy43NjEzODM5MyBMMTkyLjkzNzUsMTAuMTQ4NDM3NSBMMTkyLjk2ODMwNCwxMC4xNDg0Mzc1IEwxOTQuNTk1NTM2LDMuNzYxMzgzOTMgTDE5NS44ODUyNjgsMy43NjEzODM5MyBMMTkzLjE2NjUxOCwxMy4xODgzNDgyIiBpZD0iRmlsbC0xOSIgZmlsbD0iIzk5OTk5OSI+PC9wYXRoPgogICAgICAgICAgICAgICAgPHBhdGggZD0iTTkyLjIzNzAwODksMTEuNDUxOTY0MyBDOTEuMzk4MDgwNCwxMS40NTE5NjQzIDkwLjU1OTY4NzUsMTEuNDUxOTY0MyA4OS43MTk5NTU0LDExLjQ1MTk2NDMgQzg4Ljg4MTAyNjgsMTEuNDUxOTY0MyA4OC4wNDI1LDExLjQ1MTk2NDMgODcuMjAzNTcxNCwxMS40NTE5NjQzIEM4Ni4zNjM4MzkzLDExLjQ1MTk2NDMgODUuNTI0MjQxMSwxMS40NTE5NjQzIDg0LjY4NTg0ODIsMTEuNDUxOTY0MyBDODQuNjg1ODQ4MiwxMC42MTQyNDExIDg0LjY4NTg0ODIsOS43NzMzMDM1NyA4NC42ODU4NDgyLDguOTM0MTA3MTQgQzg0LjY4NTg0ODIsOC4wOTUzMTI1IDg0LjY4NTg0ODIsNy4yNTYxMTYwNyA4NC42ODU4NDgyLDYuNDE2NjUxNzkgQzg0LjY4NTg0ODIsNS41Nzk1OTgyMSA4NC42ODU4NDgyLDQuNzQwNTM1NzEgODQuNjg1ODQ4MiwzLjkwMDgwMzU3IEM4NS41MjQyNDExLDMuOTAwODAzNTcgODYuMzYzODM5MywzLjkwMDgwMzU3IDg3LjIwMzU3MTQsMy45MDA4MDM1NyBDODguMDQyNSwzLjkwMDgwMzU3IDg4Ljg4MTAyNjgsMy45MDA4MDM1NyA4OS43MTk5NTU0LDMuOTAwODAzNTcgQzkwLjU1OTY4NzUsMy45MDA4MDM1NyA5MS4zOTgwODA0LDMuODk0MTA3MTQgOTIuMjM3MDA4OSwzLjg5NDEwNzE0IEM5Mi4yMzcwMDg5LDQuNzMzMDM1NzEgOTIuMjQwODkyOSw1LjM1NDA2MjUgOTIuMjQwODkyOSw2LjE5MzI1ODkzIEM5Mi4yNDA4OTI5LDcuMDMyMDUzNTcgOTIuMjM3MDA4OSw4LjExNzE0Mjg2IDkyLjIzNzAwODksOC45NDc1IEM5Mi4yMzcwMDg5LDkuNzg2OTY0MjkgOTIuMjM3MDA4OSwxMC42MTQyNDExIDkyLjIzNzAwODksMTEuNDUxOTY0MyIgaWQ9IkZpbGwtMjAiIGZpbGw9IiM2NjY3NjYiPjwvcGF0aD4KICAgICAgICAgICAgICAgIDxwYXRoIGQ9Ik0yMDAuNjY3ODU3LDIuNTMwMDQ0NjQgTDIwMC40NjAyNjgsMi41MzAwNDQ2NCBMMjAwLjQ2MDI2OCwyLjEwNjQyODU3IEwyMDAuNzI1NDQ2LDIuMTA2NDI4NTcgQzIwMC43NjAyNjgsMi4xMDY0Mjg1NyAyMDAuNzk1MDg5LDIuMTA4NDM3NSAyMDAuODI5OTExLDIuMTEyNDU1MzYgQzIwMC44NjMzOTMsMi4xMTY0NzMyMSAyMDAuODk1NTM2LDIuMTI1NTgwMzYgMjAwLjkyMzY2MSwyLjEzOTY0Mjg2IEMyMDAuOTUxNzg2LDIuMTUzODM5MjkgMjAwLjk3NTg5MywyLjE3NDQ2NDI5IDIwMC45OTMzMDQsMi4yMDE2NTE3OSBDMjAxLjAxMjA1NCwyLjIyODk3MzIxIDIwMS4wMjE0MjksMi4yNjQ3MzIxNCAyMDEuMDIxNDI5LDIuMzA5MTk2NDMgQzIwMS4wMjE0MjksMi4zNjE2OTY0MyAyMDEuMDEwNzE0LDIuNDAyOTQ2NDMgMjAwLjk5MDYyNSwyLjQzMzIxNDI5IEMyMDAuOTcwNTM2LDIuNDYzNDgyMTQgMjAwLjk0Mzc1LDIuNDg1NzE0MjkgMjAwLjkxMTYwNywyLjQ5OTc3Njc5IEMyMDAuODc5NDY0LDIuNTEzOTczMjEgMjAwLjg0MTk2NCwyLjUyMjU0NDY0IDIwMC43OTkxMDcsMi41MjU0OTEwNyBDMjAwLjc1NjI1LDIuNTI4NTcxNDMgMjAwLjcxMjA1NCwyLjUzMDA0NDY0IDIwMC42Njc4NTcsMi41MzAwNDQ2NCBaIE0yMDEuMTIxODc1LDIuNTggQzIwMS4xODg4MzksMi41MjQ0MTk2NCAyMDEuMjIyMzIxLDIuNDM3MjMyMTQgMjAxLjIyMjMyMSwyLjMxODE2OTY0IEMyMDEuMjIyMzIxLDIuMDY4MTI1IDIwMS4wNjgzMDQsMS45NDMwMzU3MSAyMDAuNzU4OTI5LDEuOTQzMDM1NzEgTDIwMC4yNTkzNzUsMS45NDMwMzU3MSBMMjAwLjI1OTM3NSwzLjI1OTI4NTcxIEwyMDAuNDYwMjY4LDMuMjU5Mjg1NzEgTDIwMC40NjAyNjgsMi42OTM0Mzc1IEwyMDAuNjc3MjMyLDIuNjkzNDM3NSBMMjAxLjAzNjE2MSwzLjI1OTI4NTcxIEwyMDEuMjUzMTI1LDMuMjU5Mjg1NzEgTDIwMC44NzE0MjksMi42NzgzMDM1NyBDMjAwLjk3MTg3NSwyLjY2ODI1ODkzIDIwMS4wNTQ5MTEsMi42MzU0NDY0MyAyMDEuMTIxODc1LDIuNTggWiBNMjAxLjU1MDQ0NiwyLjk4MzkyODU3IEMyMDEuNTAyMjMyLDMuMTAwOTgyMTQgMjAxLjQzNTI2OCwzLjIwMjIzMjE0IDIwMS4zNTA4OTMsMy4yODc5NDY0MyBDMjAxLjI2NjUxOCwzLjM3Mzc5NDY0IDIwMS4xNjg3NSwzLjQ0MTI5NDY0IDIwMS4wNTYyNSwzLjQ5MDcxNDI5IEMyMDAuOTQzNzUsMy41NDAxMzM5MyAyMDAuODIxODc1LDMuNTY0OTEwNzEgMjAwLjY5MTk2NCwzLjU2NDkxMDcxIEMyMDAuNTYyMDU0LDMuNTY0OTEwNzEgMjAwLjQ0MDE3OSwzLjU0MDEzMzkzIDIwMC4zMjc2NzksMy40OTA3MTQyOSBDMjAwLjIxMzgzOSwzLjQ0MTI5NDY0IDIwMC4xMTQ3MzIsMy4zNzM3OTQ2NCAyMDAuMDMxNjk2LDMuMjg3OTQ2NDMgQzE5OS45NDg2NjEsMy4yMDIyMzIxNCAxOTkuODgzMDM2LDMuMTAwOTgyMTQgMTk5LjgzMzQ4MiwyLjk4MzkyODU3IEMxOTkuNzg1MjY4LDIuODY3MDA4OTMgMTk5Ljc2MTE2MSwyLjczOTc3Njc5IDE5OS43NjExNjEsMi42MDI2MzM5MyBDMTk5Ljc2MTE2MSwyLjQ2NzYzMzkzIDE5OS43ODUyNjgsMi4zNDA5Mzc1IDE5OS44MzM0ODIsMi4yMjI5NDY0MyBDMTk5Ljg4MzAzNiwyLjEwNDgyMTQzIDE5OS45NDg2NjEsMi4wMDMwMzU3MSAyMDAuMDMxNjk2LDEuOTE3MzIxNDMgQzIwMC4xMTQ3MzIsMS44MzE2MDcxNCAyMDAuMjEzODM5LDEuNzYzOTczMjEgMjAwLjMyNzY3OSwxLjcxNDU1MzU3IEMyMDAuNDQwMTc5LDEuNjY1MTMzOTMgMjAwLjU2MjA1NCwxLjY0MDM1NzE0IDIwMC42OTE5NjQsMS42NDAzNTcxNCBDMjAwLjgyMTg3NSwxLjY0MDM1NzE0IDIwMC45NDM3NSwxLjY2NTEzMzkzIDIwMS4wNTYyNSwxLjcxNDU1MzU3IEMyMDEuMTY4NzUsMS43NjM5NzMyMSAyMDEuMjY2NTE4LDEuODMxNjA3MTQgMjAxLjM1MDg5MywxLjkxNzMyMTQzIEMyMDEuNDM1MjY4LDIuMDAzMDM1NzEgMjAxLjUwMjIzMiwyLjEwNDgyMTQzIDIwMS41NTA0NDYsMi4yMjI5NDY0MyBDMjAxLjYsMi4zNDA5Mzc1IDIwMS42MjQxMDcsMi40Njc2MzM5MyAyMDEuNjI0MTA3LDIuNjAyNjMzOTMgQzIwMS42MjQxMDcsMi43Mzk3NzY3OSAyMDEuNiwyLjg2NzAwODkzIDIwMS41NTA0NDYsMi45ODM5Mjg1NyBaIE0yMDEuNzM1MjY4LDIuMTU2MjUgQzIwMS42NzUsMi4wMTgxNjk2NCAyMDEuNTkzMzA0LDEuODk5MTA3MTQgMjAxLjQ5MDE3OSwxLjc5OTE5NjQzIEMyMDEuMzg3MDU0LDEuNjk5NDE5NjQgMjAxLjI2NjUxOCwxLjYyMDgwMzU3IDIwMS4xMjg1NzEsMS41NjMzNDgyMSBDMjAwLjk5MTk2NCwxLjUwNTc1ODkzIDIwMC44NDU5ODIsMS40NzY5NjQyOSAyMDAuNjkxOTY0LDEuNDc2OTY0MjkgQzIwMC41Mzc5NDYsMS40NzY5NjQyOSAyMDAuMzkxOTY0LDEuNTA1NzU4OTMgMjAwLjI1NTM1NywxLjU2MzM0ODIxIEMyMDAuMTE4NzUsMS42MjA4MDM1NyAxOTkuOTk4MjE0LDEuNjk5NDE5NjQgMTk5Ljg5NTA4OSwxLjc5OTE5NjQzIEMxOTkuNzkwNjI1LDEuODk5MTA3MTQgMTk5LjcwODkyOSwyLjAxODE2OTY0IDE5OS42NSwyLjE1NjI1IEMxOTkuNTg5NzMyLDIuMjk0NTk4MjEgMTk5LjU2MDI2OCwyLjQ0MzM5Mjg2IDE5OS41NjAyNjgsMi42MDI2MzM5MyBDMTk5LjU2MDI2OCwyLjc2NDE1MTc5IDE5OS41ODk3MzIsMi45MTMzNDgyMSAxOTkuNjUsMy4wNTA0OTEwNyBDMTk5LjcwODkyOSwzLjE4Nzc2Nzg2IDE5OS43OTA2MjUsMy4zMDYxNjA3MSAxOTkuODk1MDg5LDMuNDA2MDcxNDMgQzE5OS45OTgyMTQsMy41MDU4NDgyMSAyMDAuMTE4NzUsMy41ODQ1OTgyMSAyMDAuMjU1MzU3LDMuNjQyMDUzNTcgQzIwMC4zOTE5NjQsMy42OTk1MDg5MyAyMDAuNTM3OTQ2LDMuNzI4MzAzNTcgMjAwLjY5MTk2NCwzLjcyODMwMzU3IEMyMDAuODQ1OTgyLDMuNzI4MzAzNTcgMjAwLjk5MTk2NCwzLjY5OTUwODkzIDIwMS4xMjg1NzEsMy42NDIwNTM1NyBDMjAxLjI2NjUxOCwzLjU4NDU5ODIxIDIwMS4zODcwNTQsMy41MDU4NDgyMSAyMDEuNDkwMTc5LDMuNDA2MDcxNDMgQzIwMS41OTMzMDQsMy4zMDYxNjA3MSAyMDEuNjc1LDMuMTg3NzY3ODYgMjAxLjczNTI2OCwzLjA1MDQ5MTA3IEMyMDEuNzk1NTM2LDIuOTEzMzQ4MjEgMjAxLjgyNSwyLjc2NDE1MTc5IDIwMS44MjUsMi42MDI2MzM5MyBDMjAxLjgyNSwyLjQ0MzM5Mjg2IDIwMS43OTU1MzYsMi4yOTQ1OTgyMSAyMDEuNzM1MjY4LDIuMTU2MjUgTDIwMS43MzUyNjgsMi4xNTYyNSBaIiBpZD0iRmlsbC0yMSIgZmlsbD0iIzk5OTk5OSI+PC9wYXRoPgogICAgICAgICAgICAgICAgPHBvbHlnb24gaWQ9IkZpbGwtMjIiIGZpbGw9IiMwMDg4Q0MiIHBvaW50cz0iODQuNjgzODM5MyAxMS40NjY4MzA0IDkyLjI0MzAzNTcgMTEuNDY2ODMwNCA5Mi4yNDMwMzU3IDMuODg1ODAzNTcgODQuNjgzODM5MyAzLjg4NTgwMzU3Ij48L3BvbHlnb24+CiAgICAgICAgICAgIDwvZz4KICAgICAgICA8L2c+CiAgICA8L2c+Cjwvc3ZnPg==" alt="Powered by Symplicity"></a></div><div><div class="footer-links ng-star-inserted"><a href=http://www.symplicity.com/privacy_policy target=_blank translate ng-reflect-translate>Privacy Policy</a> | <a href=http://www.symplicity.com/terms_of_use target=_blank translate ng-reflect-translate>Terms of Use</a></div></div></div></footer></div></div></div></main-layout><size-detector ng-reflect-id=main-layout><div class="display-mobile-block hide is-0 ng-star-inserted sf-hidden"></div><div class="display-sm-block hide is-1 ng-star-inserted sf-hidden"></div><div class="display-md-block hide is-2 ng-star-inserted sf-hidden"></div><div class="display-lg-block hide is-3 ng-star-inserted sf-hidden"></div><div class="display-desktop-block hide is-4 ng-star-inserted"></div></size-detector></my-app><div id=teal-job-tracker-root-stable style=z-index:2147483647><iframe id=teal-job-tracker-iframe-stable style="background:rgb(255,255,255)!important;width:380px!important;height:100vh!important;position:fixed!important;inset:0px -380px auto auto!important;z-index:2147483647!important;border:0px!important;box-shadow:rgba(0,0,0,0.25) 0px 0px 16px -3px!important;transition:right 0.25s ease-in-out!important;transform:none!important" sandbox="allow-popups allow-top-navigation allow-top-navigation-by-user-activation" srcdoc="<!DOCTYPE html> <html><meta charset=utf-8><style data-styled=active data-styled-version=5.3.1>.jkanGt{display:flex;padding:16px 0px 8px;-webkit-box-pack:justify;justify-content:space-between;-webkit-box-align:center;align-items:center;font-size:12px}.jkanGt span{font-feature-settings:"tnum";font-size:12px;float:left;opacity:0.5}.jkanGt a{color:white;opacity:0.5;transition:opacity 0.3s;text-decoration:underline;font-size:12px}.jkanGt a:hover{text-decoration:underline;opacity:1;color:white!important}.tMHxk{display:block;position:absolute;z-index:1000;top:60px;right:-16px;left:-16px;background:var(--dark-teal);text-align:right;color:white;padding:0px;height:0px;overflow:hidden;transform-origin:center top;transition:transform 0.18s ease-in-out;transform:scaleY(0)}.tMHxk ul{margin:0px;padding:0px;list-style:none}.tMHxk ul li{position:relative;font-size:14px;line-height:32px;display:flex;-webkit-box-pack:end;justify-content:flex-end;-webkit-box-align:center;align-items:center;width:auto}.tMHxk ul li.link-item a{pointer-events:all;display:flex;flex-direction:row;text-decoration:none;color:rgb(224,173,110);-webkit-box-pack:end;justify-content:flex-end;-webkit-box-align:center;align-items:center}.tMHxk ul li.link-item a:hover{color:rgb(244,195,138)}.jWBOYC{position:relative;display:block;width:22px;height:22px;border:2px solid;transform:scale(0.66);border-radius:20px}.jWBOYC::after,.jWBOYC::before{content:"";display:block;box-sizing:border-box;position:absolute;right:4px}.jWBOYC::after{width:6px;height:6px;border-top:2px solid;border-right:2px solid;transform:rotate(45deg);bottom:6px}.jWBOYC::before{width:10px;height:2px;bottom:8px;background:currentcolor}</style><style>@media (min-width:1200px){}@media (min-width:992px){}@media (max-width:991px){}@media (max-width:767px){}@media (max-width:991px){}@media (max-width:767px){}/*!* Quill Editor v1.3.7* https://quilljs.com/* Copyright (c) 2014, Jason Chen* Copyright (c) 2013, salesforce.com*/@media (pointer:coarse){}:root{--content-margin-x:.75rem;--content-margin-y:.75rem}@media (min-width:992px){:root{--content-margin-x:1rem;--content-margin-y:1rem}}@keyframes _rotation_hvjtq_1{0%{transform:rotate(0)}to{transform:rotate(360deg)}}@media (prefers-contrast:more){}@supports (width:min(var(--min),100%)){._grid_1prkj_1{grid-template-columns:repeat(auto-fit,minmax(min(var(--min),100%),1fr))}}:root{--color-danger-900:#771f0d}@font-face{font-weight:400;font-family:Poppins;font-style:normal;src:url(fonts/Poppins-Regular.woff)format("woff");unicode-range:U+0000-00FF,U+0131,U+0152-0153,U+02BB-02BC,U+02C6,U+02DA,U+02DC,U+0304,U+0308,U+0329,U+2000-206F,U+2074,U+20AC,U+2122,U+2191,U+2193,U+2212,U+2215,U+FEFF,U+FFFD}@font-face{font-weight:400;font-family:Poppins;font-style:italic;src:url(/fonts/Poppins-RegularItalic.woff)format("woff");unicode-range:U+0000-00FF,U+0131,U+0152-0153,U+02BB-02BC,U+02C6,U+02DA,U+02DC,U+0304,U+0308,U+0329,U+2000-206F,U+2074,U+20AC,U+2122,U+2191,U+2193,U+2212,U+2215,U+FEFF,U+FFFD}@font-face{font-weight:500;font-family:Poppins;font-style:normal;src:url(/fonts/Poppins-Medium.woff)format("woff");unicode-range:U+0000-00FF,U+0131,U+0152-0153,U+02BB-02BC,U+02C6,U+02DA,U+02DC,U+0304,U+0308,U+0329,U+2000-206F,U+2074,U+20AC,U+2122,U+2191,U+2193,U+2212,U+2215,U+FEFF,U+FFFD}@font-face{font-weight:500;font-family:Poppins;font-style:italic;src:url(/fonts/Poppins-MediumItalic.woff)format("woff");unicode-range:U+0000-00FF,U+0131,U+0152-0153,U+02BB-02BC,U+02C6,U+02DA,U+02DC,U+0304,U+0308,U+0329,U+2000-206F,U+2074,U+20AC,U+2122,U+2191,U+2193,U+2212,U+2215,U+FEFF,U+FFFD}@font-face{font-weight:600;font-family:Poppins;font-style:normal;src:url(/fonts/Poppins-SemiBold.woff)format("woff");unicode-range:U+0000-00FF,U+0131,U+0152-0153,U+02BB-02BC,U+02C6,U+02DA,U+02DC,U+0304,U+0308,U+0329,U+2000-206F,U+2074,U+20AC,U+2122,U+2191,U+2193,U+2212,U+2215,U+FEFF,U+FFFD}@font-face{font-weight:600;font-family:Poppins;font-style:italic;src:url(/fonts/Poppins-SemiBoldItalic.woff)format("woff");unicode-range:U+0000-00FF,U+0131,U+0152-0153,U+02BB-02BC,U+02C6,U+02DA,U+02DC,U+0304,U+0308,U+0329,U+2000-206F,U+2074,U+20AC,U+2122,U+2191,U+2193,U+2212,U+2215,U+FEFF,U+FFFD}:root{--teal:#244747;--dark-teal:#11292a;--faded-teal:#e9f3f3;--gold:#be9369;--sand:#f4c38a;--medium-gold:#e0ad6e;--dark-gold:#c18b49;--light-gray:#dcdcdc;--beige:#f4efea;--light-beige:#f9f7f5;--dark-beige:#cbb7a4;--gray:#cbcbcb;--success-light:#d6f3e2;--success:#008556;--success-dark:#086343}/*! * * antd v4.24.8 * * Copyright 2015-present, Alipay, Inc. * All rights reserved. * */body,html{width:100%}*,:after,:before{box-sizing:border-box}html{font-family:sans-serif;line-height:1.15;-webkit-text-size-adjust:100%;-ms-text-size-adjust:100%;-ms-overflow-style:scrollbar;-webkit-tap-highlight-color:rgba(0,0,0,0)}@-ms-viewport{width:device-width}body{font-size:14px;font-variant:tabular-nums;font-feature-settings:"tnum"}ul{margin-top:0;margin-bottom:1em}a{background-color:transparent;outline:none;cursor:pointer;transition:color .3s;-webkit-text-decoration-skip:objects}a:hover{color:#40a9ff}a:active{color:#096dd9}a:active,a:focus,a:hover{text-decoration:none;outline:0}img{vertical-align:middle;border-style:none}a{touch-action:manipulation}button{margin:0;font-family:inherit}button{overflow:visible}button{text-transform:none}html [type=button]{-webkit-appearance:button}::-webkit-file-upload-button{font:inherit;-webkit-appearance:button}::selection{color:#fff;background:#1890ff}@keyframes antFadeIn{0%{opacity:0}to{opacity:1}}@keyframes antFadeOut{0%{opacity:1}to{opacity:0}}@keyframes antMoveDownIn{0%{transform:translateY(100%);transform-origin:0 0;opacity:0}to{transform:translateY(0);transform-origin:0 0;opacity:1}}@keyframes antMoveDownOut{0%{transform:translateY(0);transform-origin:0 0;opacity:1}to{transform:translateY(100%);transform-origin:0 0;opacity:0}}@keyframes antMoveLeftIn{0%{transform:translate(-100%);transform-origin:0 0;opacity:0}to{transform:translate(0);transform-origin:0 0;opacity:1}}@keyframes antMoveLeftOut{0%{transform:translate(0);transform-origin:0 0;opacity:1}to{transform:translate(-100%);transform-origin:0 0;opacity:0}}@keyframes antMoveRightIn{0%{transform:translate(100%);transform-origin:0 0;opacity:0}to{transform:translate(0);transform-origin:0 0;opacity:1}}@keyframes antMoveRightOut{0%{transform:translate(0);transform-origin:0 0;opacity:1}to{transform:translate(100%);transform-origin:0 0;opacity:0}}@keyframes antMoveUpIn{0%{transform:translateY(-100%);transform-origin:0 0;opacity:0}to{transform:translateY(0);transform-origin:0 0;opacity:1}}@keyframes antMoveUpOut{0%{transform:translateY(0);transform-origin:0 0;opacity:1}to{transform:translateY(-100%);transform-origin:0 0;opacity:0}}@keyframes loadingCircle{to{transform:rotate(1turn)}}html{--antd-wave-shadow-color:#1890ff;--scroll-bar:0}@keyframes waveEffect{to{box-shadow:0 0#1890ff;box-shadow:0 0 0 6px var(--antd-wave-shadow-color)}}@keyframes fadeEffect{to{opacity:0}}@keyframes antSlideUpIn{0%{transform:scaleY(.8);transform-origin:0 0;opacity:0}to{transform:scaleY(1);transform-origin:0 0;opacity:1}}@keyframes antSlideUpOut{0%{transform:scaleY(1);transform-origin:0 0;opacity:1}to{transform:scaleY(.8);transform-origin:0 0;opacity:0}}@keyframes antSlideDownIn{0%{transform:scaleY(.8);transform-origin:100% 100%;opacity:0}to{transform:scaleY(1);transform-origin:100% 100%;opacity:1}}@keyframes antSlideDownOut{0%{transform:scaleY(1);transform-origin:100% 100%;opacity:1}to{transform:scaleY(.8);transform-origin:100% 100%;opacity:0}}@keyframes antSlideLeftIn{0%{transform:scaleX(.8);transform-origin:0 0;opacity:0}to{transform:scaleX(1);transform-origin:0 0;opacity:1}}@keyframes antSlideLeftOut{0%{transform:scaleX(1);transform-origin:0 0;opacity:1}to{transform:scaleX(.8);transform-origin:0 0;opacity:0}}@keyframes antSlideRightIn{0%{transform:scaleX(.8);transform-origin:100%0;opacity:0}to{transform:scaleX(1);transform-origin:100%0;opacity:1}}@keyframes antSlideRightOut{0%{transform:scaleX(1);transform-origin:100%0;opacity:1}to{transform:scaleX(.8);transform-origin:100%0;opacity:0}}@keyframes antZoomIn{0%{transform:scale(.2);opacity:0}to{transform:scale(1);opacity:1}}@keyframes antZoomOut{0%{transform:scale(1)}to{transform:scale(.2);opacity:0}}@keyframes antZoomBigIn{0%{transform:scale(.8);opacity:0}to{transform:scale(1);opacity:1}}@keyframes antZoomBigOut{0%{transform:scale(1)}to{transform:scale(.8);opacity:0}}@keyframes antZoomUpIn{0%{transform:scale(.8);transform-origin:50%0;opacity:0}to{transform:scale(1);transform-origin:50%0}}@keyframes antZoomUpOut{0%{transform:scale(1);transform-origin:50%0}to{transform:scale(.8);transform-origin:50%0;opacity:0}}@keyframes antZoomLeftIn{0%{transform:scale(.8);transform-origin:0 50%;opacity:0}to{transform:scale(1);transform-origin:0 50%}}@keyframes antZoomLeftOut{0%{transform:scale(1);transform-origin:0 50%}to{transform:scale(.8);transform-origin:0 50%;opacity:0}}@keyframes antZoomRightIn{0%{transform:scale(.8);transform-origin:100% 50%;opacity:0}to{transform:scale(1);transform-origin:100% 50%}}@keyframes antZoomRightOut{0%{transform:scale(1);transform-origin:100% 50%}to{transform:scale(.8);transform-origin:100% 50%;opacity:0}}@keyframes antZoomDownIn{0%{transform:scale(.8);transform-origin:50% 100%;opacity:0}to{transform:scale(1);transform-origin:50% 100%}}@keyframes antZoomDownOut{0%{transform:scale(1);transform-origin:50% 100%}to{transform:scale(.8);transform-origin:50% 100%;opacity:0}}@media screen and (max-width:768px){}@media screen and (max-width:480px){}@keyframes antStatusProcessing{0%{transform:scale(.8);opacity:.5}to{transform:scale(2.4);opacity:0}}@keyframes antZoomBadgeIn{0%{transform:scale(0) translate(50%,-50%);opacity:0}to{transform:scale(1) translate(50%,-50%)}}@keyframes antZoomBadgeOut{0%{transform:scale(1) translate(50%,-50%)}to{transform:scale(0) translate(50%,-50%);opacity:0}}@keyframes antNoWrapperZoomBadgeIn{0%{transform:scale(0);opacity:0}to{transform:scale(1)}}@keyframes antNoWrapperZoomBadgeOut{0%{transform:scale(1)}to{transform:scale(0);opacity:0}}@keyframes antBadgeLoadingCircle{0%{transform-origin:50%}to{transform:translate(50%,-50%) rotate(1turn);transform-origin:50%}}@keyframes antZoomBadgeInRtl{0%{transform:scale(0) translate(-50%,-50%);opacity:0}to{transform:scale(1) translate(-50%,-50%)}}@keyframes antZoomBadgeOutRtl{0%{transform:scale(1) translate(-50%,-50%)}to{transform:scale(0) translate(-50%,-50%);opacity:0}}.ant-btn{line-height:1.5715;position:relative;display:inline-block;white-space:nowrap;text-align:center;background-image:none;cursor:pointer;transition:all .3s cubic-bezier(.645,.045,.355,1);-webkit-user-select:none;-moz-user-select:none;-ms-user-select:none;user-select:none;touch-action:manipulation}.ant-btn,.ant-btn:active,.ant-btn:focus{outline:0}.ant-btn:not([disabled]):hover{text-decoration:none}.ant-btn:not([disabled]):active{outline:0;box-shadow:none}.ant-btn:focus,.ant-btn:hover{color:#40a9ff;border-color:#40a9ff;background:#fff}.ant-btn:active{color:#096dd9;border-color:#096dd9;background:#fff}.ant-btn:active,.ant-btn:focus,.ant-btn:hover{text-decoration:none;background:#fff}.ant-btn>span{display:inline-block}.ant-btn-primary{background:#1890ff}.ant-btn-primary:focus,.ant-btn-primary:hover{color:#fff;border-color:#40a9ff;background:#40a9ff}.ant-btn-primary:active{color:#fff;border-color:#096dd9;background:#096dd9}.ant-btn-round{border-radius:32px}.ant-btn:before{position:absolute;top:-1px;right:-1px;bottom:-1px;left:-1px;z-index:1;display:none;background:#fff;border-radius:inherit;opacity:.35;transition:opacity .2s;content:"";pointer-events:none}.ant-btn:active>span,.ant-btn:focus>span{position:relative}.ant-btn.ant-btn-block{width:100%}.ant-btn:empty{display:inline-block;width:0;visibility:hidden;content:" "}@media only screen and (max-width:480px){}@media (max-width:575px){}@media (max-width:767px){}@media (max-width:991px){}@media (max-width:1199px){}@media (max-width:1599px){}@keyframes diffZoomIn1{0%{transform:scale(0);opacity:0}to{transform:scale(1);opacity:1}}@keyframes diffZoomIn2{0%{transform:scale(0);opacity:0}to{transform:scale(1);opacity:1}}@keyframes diffZoomIn3{0%{transform:scale(0);opacity:0}to{transform:scale(1);opacity:1}}.ant-row{flex-flow:row wrap;min-width:0}.ant-row,.ant-row:after,.ant-row:before{display:flex}.ant-row-center{justify-content:center}.ant-row-space-between{justify-content:space-between}.ant-row-middle{align-items:center}@media (min-width:576px){}@media (min-width:768px){}@media (min-width:992px){}@media (min-width:1200px){}@media (min-width:1600px){}@media (-ms-high-contrast:none),screen and (-ms-high-contrast:active){}.ant-layout{display:flex;flex:auto;flex-direction:column;min-height:0;background:#f0f2f5}.ant-layout,.ant-layout *{box-sizing:border-box}.ant-layout-header{flex:0 0 auto}.ant-layout-content{flex:auto}@media screen and (max-width:768px){}@media screen and (max-width:576px){}@media screen and (max-width:768px){}@media screen and (max-width:576px){}@keyframes MessageMoveOut{0%{max-height:150px;padding:8px;opacity:1}to{max-height:0;padding:0;opacity:0}}@media (max-width:767px){}@keyframes NotificationFadeIn{0%{left:384px;opacity:0}to{left:0;opacity:1}}@keyframes NotificationFadeOut{0%{max-height:150px;margin-bottom:16px;opacity:1}to{max-height:0;margin-bottom:0;padding-top:0;padding-bottom:0;opacity:0}}@keyframes NotificationTopFadeIn{0%{margin-top:-100%;opacity:0}to{margin-top:0;opacity:1}}@keyframes NotificationBottomFadeIn{0%{margin-bottom:-100%;opacity:0}to{margin-bottom:0;opacity:1}}@keyframes NotificationLeftFadeIn{0%{right:384px;opacity:0}to{right:0;opacity:1}}@media (-ms-high-contrast:none){}@media only screen and (max-width:992px){}@media only screen and (max-width:576px){}@media (-ms-high-contrast:none),screen and (-ms-high-contrast:active){}@keyframes ant-progress-active{0%{transform:translate(-100%) scaleX(0);opacity:.1}20%{transform:translate(-100%) scaleX(0);opacity:.5}to{transform:translate(0) scaleX(1);opacity:0}}@keyframes antRadioEffect{0%{transform:scale(1);opacity:.5}to{transform:scale(1.6);opacity:0}}@media (-ms-high-contrast:none){}@media (-ms-high-contrast:none){}@keyframes ant-skeleton-loading{0%{transform:translate(-37.5%)}to{transform:translate(37.5%)}}@keyframes ant-skeleton-loading-rtl{0%{background-position:0 50%}to{background-position:100% 50%}}@media (-ms-high-contrast:active),(-ms-high-contrast:none){}@keyframes antSpinMove{to{opacity:1}}@keyframes antRotate{to{transform:rotate(1turn)}}@keyframes antRotateRtl{to{transform:rotate(-405deg)}}@media (-ms-high-contrast:none){}@keyframes antCheckboxEffect{0%{transform:scale(1);opacity:.5}to{transform:scale(1.6);opacity:0}}@keyframes ant-tree-node-fx-do-not-use{0%{opacity:0}to{opacity:1}}@keyframes uploadAnimateInlineIn{0%{width:0;height:0;margin:0;padding:0;opacity:0}}@keyframes uploadAnimateInlineOut{to{width:0;height:0;margin:0;padding:0;opacity:0}}:root{--beige:#ece4db;--bright-gold:#f5b501;--dark-beige:#cbb7a4;--dark-gold:#c18b49;--dark-teal:#005149;--dark-silver:#a0a0a0;--deep-gray:#333333;--faded-teal:#e9f3f3;--gold:#c18b49;--gray-beige:#d2cbc4;--gray:#cbcbcb;--light-beige:#fcfaf9;--light-gray:#dcdcdc;--light-teal:#99bfbf;--medium-gold:#e0ad6e;--medium-teal:#5b8080;--pigeon-gray:#959595;--sand:#f4c38a;--success-dark:#086343;--success-light:#d6f3e2;--success:#008556;--teal:#244747}html,body{height:100%!important}body{background-color:transparent;color:#000;font-family:Poppins,Arial,Roboto,-apple-system,sans-serif;font-weight:400;line-height:1.2}a{color:var(--teal);text-decoration:underline}.ant-btn{padding:10px;font-size:14px;font-weight:600;height:auto;border:0;text-shadow:none;box-shadow:none}.ant-btn.ant-btn-primary{background-color:var(--dark-teal);color:#fff}.ant-btn.ant-btn-primary{border-color:#fff;border-width:0}.frame-root,.frame-content{height:100%}.popup{height:100%;overflow:hidden}.popup .ant-layout{box-shadow:0 0 10px #0003}.popup>.ant-layout{height:100%}.popup .ant-layout-content{padding:1.5rem .875rem;background-color:#fff;overflow-y:auto;min-height:188px}.popup .ant-layout-header.nav{background:var(--dark-teal);color:#fff;height:auto;line-height:1;padding:1rem .875rem;position:relative}.popup .ant-layout-header.title{background:var(--light-beige);border-bottom:1px solid var(--beige);color:#000;height:auto;line-height:1;font-size:16px;font-weight:600;padding:.5rem .875rem}.popup .close-arrow{margin-right:12px}.popup .logout-link:hover,.popup .next-link:hover{color:var(--gold)}.popup .mb-1{margin-bottom:1rem}footer{flex-direction:column;gap:.5rem}@keyframes blinker{50%{opacity:0}}@keyframes skeleton-loading{0%{background-color:#c2cfd6}to{background-color:#f0f3f5}}:root{--color-background-surface-primary-default:#ffffff;--color-background-surface-primary-hover:#fafafa;--color-background-surface-primary-pressed:#f5f5f5;--color-background-surface-secondary-default:#f5f5f5;--color-background-surface-secondary-hover:#e5e5e5;--color-background-surface-secondary-pressed:#d4d4d4;--color-background-surface-brand-default:#f0faf8;--color-background-surface-brand-hover:#cdeeeb;--color-background-surface-brand-pressed:#9cded6;--color-background-surface-success-default:#f0faf8;--color-background-surface-success-hover:#cdeeeb;--color-background-surface-success-pressed:#9cded6;--color-background-surface-warning-default:#fffde5;--color-background-surface-warning-hover:#fffac2;--color-background-surface-warning-pressed:#fff185;--color-background-surface-danger-default:#fdf3f1;--color-background-surface-danger-hover:#fdf3f1;--color-background-surface-danger-pressed:#f9d0c8;--color-background-surface-accent-default:#fffde5;--color-background-surface-accent-hover:#fffac2;--color-background-surface-accent-pressed:#fff185;--color-background-fill-bold-brand-default:#005249;--color-background-fill-bold-brand-hover:#1d5851;--color-background-fill-bold-brand-pressed:#267369;--color-background-fill-bold-neutral-default:#ffffff;--color-background-fill-bold-neutral-hover:#fafafa;--color-background-fill-bold-neutral-pressed:#f5f5f5;--color-background-fill-bold-success-default:#005249;--color-background-fill-bold-success-hover:#1d5851;--color-background-fill-bold-success-pressed:#267369;--color-background-fill-bold-warning-default:#f4b301;--color-background-fill-bold-warning-hover:#ffe042;--color-background-fill-bold-warning-pressed:#ffe042;--color-background-fill-bold-danger-default:#e84e30;--color-background-fill-bold-danger-hover:#f08a75;--color-background-fill-bold-danger-pressed:#f5b1a3;--color-background-fill-bold-accent-default:#f4b301;--color-background-fill-bold-accent-hover:#ffe042;--color-background-fill-bold-accent-pressed:#ffe042;--color-background-fill-subtle-brand-default:#f0faf8;--color-background-fill-subtle-brand-hover:#cdeeeb;--color-background-fill-subtle-brand-pressed:#9cded6;--color-background-fill-subtle-neutral-default:#fafafa;--color-background-fill-subtle-neutral-hover:#f5f5f5;--color-background-fill-subtle-neutral-pressed:#e5e5e5;--color-background-fill-subtle-success-default:#f0faf8;--color-background-fill-subtle-success-hover:#cdeeeb;--color-background-fill-subtle-success-pressed:#9cded6;--color-background-fill-subtle-warning-default:#fffde5;--color-background-fill-subtle-warning-hover:#fffac2;--color-background-fill-subtle-warning-pressed:#fff185;--color-background-fill-subtle-danger-default:#fdf3f1;--color-background-fill-subtle-danger-hover:#fdf3f1;--color-background-fill-subtle-danger-pressed:#f9d0c8;--color-background-fill-subtle-accent-default:#fffde5;--color-background-fill-subtle-accent-hover:#fffac2;--color-background-fill-subtle-accent-pressed:#fff185;--color-mono-50:#ffffff;--color-mono-950:#000000;--color-neutral-50:#fafafa;--color-neutral-100:#f5f5f5;--color-neutral-200:#e5e5e5;--color-neutral-300:#d4d4d4;--color-neutral-400:#a3a3a3;--color-neutral-500:#737373;--color-neutral-600:#525252;--color-neutral-700:#404040;--color-neutral-800:#262626;--color-neutral-900:#171717;--color-neutral-950:#0a0a0a;--color-brand-50:#f0faf8;--color-brand-100:#cdeeeb;--color-brand-200:#9cded6;--color-brand-300:#66ccc2;--color-brand-400:#40bfae;--color-brand-500:#3bb09c;--color-brand-600:#2f8e81;--color-brand-700:#267369;--color-brand-800:#1d5851;--color-brand-900:#005249;--color-brand-950:#003d38;--color-accent-50:#fffde5;--color-accent-100:#fffac2;--color-accent-200:#fff185;--color-accent-300:#ffe042;--color-accent-400:#ffe042;--color-accent-500:#f4b301;--color-accent-600:#d18800;--color-accent-700:#a75f02;--color-accent-800:#8a4c0a;--color-accent-900:#753d0f;--color-accent-950:#431e04;--color-success-50:#f0faf8;--color-success-100:#cdeeeb;--color-success-200:#9cded6;--color-success-300:#66ccc2;--color-success-400:#40bfae;--color-success-500:#3bb09c;--color-success-600:#2f8e81;--color-success-700:#267369;--color-success-800:#1d5851;--color-success-900:#005249;--color-success-950:#003d38;--color-warning-50:#fffde5;--color-warning-100:#fffac2;--color-warning-200:#fff185;--color-warning-300:#ffe042;--color-warning-400:#ffe042;--color-warning-500:#f4b301;--color-warning-600:#d18800;--color-warning-700:#a75f02;--color-warning-800:#8a4c0a;--color-warning-900:#753d0f;--color-warning-950:#431e04;--color-danger-50:#fdf3f1;--color-danger-100:#fdf3f1;--color-danger-200:#f9d0c8;--color-danger-300:#f5b1a3;--color-danger-400:#f08a75;--color-danger-500:#e84e30;--color-danger-600:#dc3918;--color-danger-700:#c53316;--color-danger-800:#a12a12;--color-danger-900:#771f0d;--color-danger-950:#491308;--color-border-primary-default:#e5e5e5;--color-border-primary-hover:#d4d4d4;--color-border-primary-pressed:#a3a3a3;--color-border-secondary-default:#f5f5f5;--color-border-secondary-hover:#e5e5e5;--color-border-secondary-pressed:#d4d4d4;--color-border-brand-default:#005249;--color-border-brand-hover:#1d5851;--color-border-brand-pressed:#267369;--color-border-success-default:#005249;--color-border-success-hover:#1d5851;--color-border-success-pressed:#267369;--color-border-warning-default:#753d0f;--color-border-warning-hover:#8a4c0a;--color-border-warning-pressed:#a75f02;--color-border-danger-default:#771f0d;--color-border-danger-hover:#a12a12;--color-border-danger-pressed:#c53316;--color-border-accent-default:#fff185;--color-icon-primary-default:#171717;--color-icon-primary-inverted:#fafafa;--color-icon-secondary-default:#404040;--color-icon-secondary-inverted:#fafafa;--color-icon-brand-default:#005249;--color-icon-brand-inverted:#f0faf8;--color-icon-success-default:#005249;--color-icon-success-inverted:#f0faf8;--color-icon-warning-default:#753d0f;--color-icon-warning-inverted:#fffde5;--color-icon-danger-default:#771f0d;--color-icon-danger-inverted:#fdf3f1;--color-icon-accent-default:#753d0f;--color-icon-accent-inverted:#fffde5;--color-text-primary-default:#171717;--color-text-primary-inverted:#fafafa;--color-text-secondary-default:#737373;--color-text-secondary-inverted:#737373;--color-text-brand-default:#005249;--color-text-brand-inverted:#f0faf8;--color-text-success-default:#005249;--color-text-success-inverted:#f0faf8;--color-text-warning-default:#753d0f;--color-text-warning-inverted:#fffde5;--color-text-danger-default:#771f0d;--color-text-danger-inverted:#fdf3f1;--color-text-accent-default:#753d0f;--color-text-accent-inverted:#fffde5;--font-sans:Poppins,Helvetica Neue,Roboto,arial,sans-serif,Apple Color Emoji;--radius-none:0;--radius-xs:.125rem;--radius-sm:.25rem;--radius-base:.375rem;--radius-lg:.5rem;--radius-xl:.75rem;--radius-2xl:1rem;--radius-3xl:1.5rem;--radius-round:600rem;--shadow-none:none;--shadow-xs:0px 1px 2px 0px rgba(0,0,0,0);--shadow-sm:0px 1px 2px -1px rgba(0,0,0,.1),0px 1px 3px 0px rgba(0,0,0,.1);--shadow-base:0px 2px 4px -2px rgba(0,0,0,.1),0px 4px 6px -1px rgba(0,0,0,.1);--shadow-lg:0px 4px 6px -4px rgba(0,0,0,.1),0px 10px 15px -3px rgba(0,0,0,.1);--shadow-xl:0px 8px 10px -6px rgba(0,0,0,.1),0px 20px 25px -5px rgba(0,0,0,.1);--size-leading-xs:1rem;--size-leading-sm:1.25rem;--size-leading-md:1.5rem;--size-leading-lg:1.75rem;--size-leading-xl:1.75rem;--size-leading-2xl:2rem;--size-leading-3xl:2.25rem;--size-leading-4xl:2.5rem;--size-leading-5xl:3rem;--size-text-xs:.75rem;--size-text-sm:.875rem;--size-text-md:1rem;--size-text-lg:1.125rem;--size-text-xl:1.25rem;--size-text-2xl:1.5rem;--size-text-3xl:1.875rem;--size-text-4xl:2.25rem;--size-text-5xl:3rem;--spacing-3xs:.25rem;--spacing-2xs:.375rem;--spacing-xs:.5rem;--spacing-sm:.75rem;--spacing-base:1rem;--spacing-lg:1.25rem;--spacing-xl:1.5rem;--spacing-2xl:2rem;--spacing-3xl:2.5rem;--spacing-4xl:4rem;--spacing-5xl:5rem;--weight-thin:100;--weight-extralight:200;--weight-light:300;--weight-regular:400;--weight-medium:500;--weight-semibold:600;--weight-bold:700;--weight-extrabold:800;--weight-black:900}body{-webkit-font-smoothing:antialiased;-moz-osx-font-smoothing:grayscale;margin:0}*,*:before,*:after{box-sizing:border-box}html:focus-within{scroll-behavior:smooth}@media (prefers-reduced-motion:reduce){html:focus-within{scroll-behavior:auto}*,*:before,*:after{transition-duration:.01ms!important;animation-duration:.01ms!important;animation-iteration-count:1!important;scroll-behavior:auto!important}}</style><meta name=referrer content=no-referrer><meta http-equiv=content-security-policy content="default-src 'none'; font-src 'self' data:; img-src 'self' data:; style-src 'unsafe-inline'; media-src 'self' data:; script-src 'unsafe-inline' data:; object-src 'self' data:; frame-src 'self' data:;"><style>img[src="data:,"],source[src="data:,"]{display:none!important}</style><body><div class=frame-root><div class=frame-content><div class=popup><section class=ant-layout><header class="ant-layout-header nav"><div class="ant-row ant-row-space-between ant-row-middle"><div class="ant-row ant-row-middle"><a class=close-arrow><img src=data:,></a><img src=data:,></div><div></div></div><div class="sc-hKgJUU tMHxk"><ul><li class=link-item><a class=ext-link rel="noopener noreferrer" href=https://app.tealhq.com/job-tracker target=_blank>Job Tracker <i class="sc-jSgvzq jWBOYC" style=margin-left:4px;margin-right:-5px></i></a><li class=link-item><a rel="noopener noreferrer" href=https://app.tealhq.com/contact-tracker target=_blank>Contact Tracker <i class="sc-jSgvzq jWBOYC" style=margin-left:4px;margin-right:-5px></i></a><li class=link-item><a rel="noopener noreferrer" href=https://app.tealhq.com/company-tracker target=_blank>Company Tracker <i class="sc-jSgvzq jWBOYC" style=margin-left:4px;margin-right:-5px></i></a><li class=link-item><a class=ext-link rel="noopener noreferrer" href=https://app.tealhq.com/resume-builder target=_blank>Resume Builder <i class="sc-jSgvzq jWBOYC" style=margin-left:4px;margin-right:-5px></i></a></ul><footer class="sc-dlfnuX jkanGt"><span>Version&nbsp;3.8.9</span><a class=logout-link>Log Out</a></footer></div></header><header class="ant-layout-header title">Sign in to Teal</header><main class=ant-layout-content><div class="ant-row ant-row-center"><button type=button class="ant-btn ant-btn-round ant-btn-primary ant-btn-block mb-1"><span>Log In</span></button></div></main></section></div></div></div>"></iframe></div><div id=teal-job-tracker-companion-root-stable style=display:block;z-index:2147483646;bottom:58px;right:0px;position:fixed;height:60px;width:auto></div>
The page for the Cerebras position above has 3506 empty lines with only 809 lines dedicated to actual code, and the HTML code on non-empty lines isn’t exactly the most pleasing to read.
…but thanks to everything being stored in tables I can just use Python’s trusty BeautifulSoup4 library on my locally saved HTML pages and get the text in every table data cell in less than 50 lines of code:
import argparsefrom bs4 import BeautifulSoup
def parse_html_file(filepath, verbose=False): with open(filepath, 'r', encoding='utf-8') as file: html_content = file.read()
soup = BeautifulSoup(html_content, 'lxml')
data = {} rows = soup.find_all('tr') ## find all table rows
for row in rows: tds = row.find_all('td') ## find all table data cells
if len(tds) >= 2: label_td = tds[0] label_text = '\n'.join(label_td.stripped_strings)
value_td = tds[1] value_text = '\n'.join(value_td.stripped_strings)
data[label_text] = value_text
if verbose: for key, value in data.items(): print(f"{key}: {value}") else: print("Parsing completed.")
if __name__ == "__main__": parser = argparse.ArgumentParser(description="Parse HTML for 2 column table data.") parser.add_argument("-f", "--filepath", required=True, help="Path to the HTML file to be parsed.") parser.add_argument("-v", "--verbose", action="store_true", help="Print parsed data.")
args = parser.parse_args() parse_html_file(args.filepath, args.verbose)
Trying out the quickly-written parse.py
script on one of the saved HTML pages, and it’s able to get all of the values for all of the table fields with no issues:
python parse.py --verbose --filepath "Job ID 43628....html"
Position Type:: Professional Experience Year Co-op (PEY Co-op: 12-16 months)Job Title:: ML Developer - Software Automation & Developer InfrastructureJob Location:: TorontoJob Location Type:: FlexibleIf working on site, can you provide a copy of your COVID-19 safety protocols?:: NoNumber of Positions:: 1Salary:: $42.00 hourly for 40.0 hours per weekStart Date:: 05/06/2024End Date:: 04/25/2025Job Function:: Information Technology (IT)Job Description:: Cerebras Systems has pioneered a groundbreaking chip and system that revolutionizes deep learning applications. Our system empowers ML researchers to achieve unprecedented speeds in training and inference workloads, propelling AI innovation to new horizons.The Condor Galaxy 1 (CG-1), unveiled in a recent announcement, stands as a testament to Cerebras' commitment to pushing the boundaries of AI computing. With a staggering 4 ExaFLOP processing power, 54 million cores, and 64-node architecture, the CG-1 is the first of nine powerful supercomputers to be built and operated through an exclusive partnership between Cerebras and G42. This strategic collaboration aims to redefine the possibilities of AI by creating a network of interconnected supercomputers that will collectively deliver a mind-boggling 36 ExaFLOPS of AI compute power upon completion in 2024.Cerebras is building a team of exceptional people to work together on big problems. Join us!.About The RoleAs a Machine Developer - Software Automation & Developer Infrastructure Engineer, you will use your knowledge of testing and testability to influence better software design, promote proper engineering practice, bug prevention strategies, testability, scalability, and other advanced quality concepts. The position will play a huge role in the quality of Cerebras software. We are looking for engineers that have a broad set of technical skills and who are ready to tackle the biggest at-scale problems in HW-based deep learning accelerators.ResponsibilitiesWrite scripts to automate testing and create tools to allow easy development of software regression testsHelp identify weak spots and potential customer pain points and drive the software organization towards customer focused quality metricsImplement creative ways to break software and identify potential problemsContribute to developing requirements specifications with a focus on developing verification testsJob Requirements:: RequirementsEnrolled within University of Toronto's PEY program with a degree in Computer Science, Computer Engineering, or any other related disciplineExperience in developing automated tests for compute/machine learning or networking systems within a large-scale enterprise environmentAbility to take responsibility for monitoring product development and usage at all levels with an end goal toward improving product qualityStrong knowledge of software system design, C++ and PythonPreferredStrong software testing experience with a proven track record in scaling highly technical teamsKnowledge of UNIX/Linux and Windows environmentsKnowledge of neural network architecture and ML/AI deep learning principlesPrior experience in designing and developing test automation for HW systems involving ASICs or FPGAsPrior experience working with live hardware systems and debug tools operating in a real time environment such as networking devices or live computing systemsPreferred Disciplines:: Computer EngineeringComputer ScienceEngineering Science (Electrical and Computer)Engineering Science (Infrastructure)Engineering Science (Machine Intelligence)Engineering Science (Robotics)All Co-op programs:: NoTargeted Co-op Programs:: Targeted ProgramsProfessional Experience Year Co-op (12 - 16 months)Application Deadline:: Nov 1, 2023 11:59 PMApplication Receipt Procedure:: Online via systemIf by Website, go to:: https://www.cerebras.net/careers/?gh_jid=5321500003Additional Application Information:: Please apply withboth resume & transcript.Lacking transcript will disqualify you from being considered.Note that applications will be considered on a rolling basis. Apply as early as possible.Note to PEY Co-op applicants: In addition to your application by email/website, please ensure that you select the “I intend to apply for this position” tab on the portal. This will give us a record of your submitted application in the event that you will be invited for interviews.U of T Job Coordinator:: Yasmine AbdelhadyOrganization:: Cerebras SystemsDivision:: Computer ScienceWebsite:: https://cerebras.net/Length of Workterm:: FLEXIBLE PEY Co-op: 12-16 months (range)
Output of the parse.py script for the Cerebras job posting.
Finetuning data extraction
There’s a few nuances to the data extraction that mean this simple script needs just a bit more extending so it can properly parse the entire dataset.
I’m getting the text corresponding to any inline links, but the links themselves aren’t included since they’re within the html <a>
tags, so I need to add handling for those as well. Another nuance is the fact that the formatting for HTML pages has changed3 (several times actually) over the course of the last two semesters.
Since the location of the data has always remained in tables, that’s largely a non-issue. While the job title and company name included in the header above any table on job posting pages are currently missed by the script, that data is also present in the tables below (and extracted by the script properly), so that, too, is a non-issue.
With one exception: job IDs aren’t extracted.
Luckily, I had the foresight to configure SingleFile to include the job ID automatically as part of the filename for each HTML page back when I started the archival process, so I can add some logic to parse that as well. Unluckily, however, there were a few periods of time where that configuration was lost4, so I’m going to have to do some file contents parsing regardless.
A quick manual parsing of the different HTML files shows that there’s only two different locations that job IDs can be located (one for the old design, and the other for the new design), so I can just add another function to try and find the job ID located at either location in all files and I’m now able to extract all the job IDs as well:
import argparsefrom bs4 import BeautifulSoupimport re
def extract_job_id_from_html(soup): ## try to find job ID in a <h1> tag with the specific class
header_tag = soup.find('h1', class_='h3 dashboard-header__profile-information-name mobile--small-font color--font--white margin--b--s') if header_tag: header_text = header_tag.get_text(strip=True) match = re.match(r'^(\d+)', header_text) if match: return match.group(1)
## if not found, try to find an <h1> tag containing the words "Job ID" job_id_tag = soup.find('h1', string=re.compile(r'Job ID', re.IGNORECASE)) if job_id_tag: job_id_text = job_id_tag.get_text(strip=True) match = re.search(r'Job ID\s*:\s*(\d+)', job_id_text, re.IGNORECASE) if match: return match.group(1)
return None
def parse_html_file(filepath, verbose=False): with open(filepath, 'r', encoding='utf-8') as file: html_content = file.read()
36 collapsed lines
soup = BeautifulSoup(html_content, 'lxml')
data = {} job_id = extract_job_id_from_html(soup) if job_id: data['Job ID'] = job_id
rows = soup.find_all('tr') ## find all table rows
for row in rows: tds = row.find_all('td') ## find all table data cells
if len(tds) >= 2: label_td = tds[0] label_text = '\n'.join(label_td.stripped_strings)
value_td = tds[1] value_text = '\n'.join(value_td.stripped_strings)
data[label_text] = value_text
return data
if __name__ == "__main__": parser = argparse.ArgumentParser(description="Parse HTML for 2 column table data.") parser.add_argument("-f", "--filepath", required=True, help="Path to the HTML file to be parsed.") parser.add_argument("-v", "--verbose", action="store_true", help="Print parsed data.")
args = parser.parse_args() data = parse_html_file(args.filepath, args.verbose)
if args.verbose: for key, value in data.items(): print(f"{key}: {value}") else: print("Parsing completed.")
Now with a method of easily extracting all the relevant data from each HTML page, all that’s left is to automate running the parser across all files saved within timestamped subdirectories on my local computer and pipe the data into a SQLite DB.
Storing data in SQLite
Why SQLite?
SQLite sits right there in the sweet middle spot between raw data formats (like CSV, JSON) that are good fits for simple data (e.g. temperature, word lists) but aren’t as great for larger datasets with more complexity (especially when it comes to data analysis) and other larger RDBMS (relational database management system) libraries that might be better designed for scaleability but are really just overkill for the little pet project that I have here at hand.
And the fact that SQLite is a single file on disk means that sharing the finely extracted and processed data is a lot easier too, which is important for me because I want future PEY co-op students to learn what they can from the dataset and better set their expectations for what to expect from program (and I hope the data helps prospective UofT students better make their admission decisions as well).
Most importantly, SQLite is serverless (unlike PostgreSQL or MySQL), which saves me a lot of headache and setup[^especially] for this relatively small-scale project (especially since sqlite3
is built into Python’s standard library!).
Pipelining data from ~2k HTML files to a single SQLite DB
Using SQLite with Python via sqlite3
is simple enough. All I need to do is add some additional code for extracting the job posting date from the parent folder for each job posting’s HTML page and for iterating across every subfolder for PEY job postings on my local computer, as well as draft up a schema for the SQLite DB and write some code for piping data from Python dictionaries to the DB file.
Database Schema
Thankfully, all job postings share largely the same fields, so (for the time being) the schema just ends up being an amalgamation of all the relevant table fields.
One small slight is that Application Receipt Procedure
and Application Method
are often used interchangeably (Application Receipt Procedure
largely before the redesign, and Application Method
after), and when one field is used the other is usually left blank (except for some positions which use both, meaning that I can’t exactly combine them into a single field without losing data; e.g. sometimes Application Method
for a posting states to use the built-in applicant tracking system while Application Receipt Procedure
states to use some company link, which is likely just attributable to job posters sticking with the default options when creating a new post). It’s largely a non-issue, and after some further exploration into the specific overlapping data I should be able to easily combine both into a single field.
I could potentially extend the schema with functional dependencies for repetitive data like company names, although it’s really only company
and maybe a few other fields where values are guaranteed to repeat (Job Location Type
, Salary
, etc. can vary wildly and use combinations of text or integers, so there’s not much we can do to unify them) so it’s not the best representation for a general-purpose database (lossy conversions can always be made for analysis).
Regardless, I can update the previous python file used for parsing to handle the new SQLite schema easily enough:
...def store_data_in_db(data, db_cursor): columns = ', '.join([f'"{key}"' for key in data.keys()]) placeholders = ', '.join(['?' for _ in data.values()]) sql = f'INSERT INTO "JobPosting" ({columns}) VALUES ({placeholders})' try: db_cursor.execute(sql, tuple(data.values())) except sqlite3.IntegrityError: logging.info("Integrity Error: Skipping row") pass
def create_db_schema(db_cursor): db_cursor.execute(''' CREATE TABLE IF NOT EXISTS JobPosting ( id INTEGER, postingDate DATE, title TEXT, company TEXT, companyDivision TEXT, companyWebsite TEXT, location TEXT, locationType TEXT, numPositions INTEGER, salary TEXT, startDate TEXT, endDate TEXT, function TEXT, description TEXT, requirements TEXT, preferredDisciplines TEXT, applicationDeadline TEXT, applicationMethod TEXT, applicationReceiptProcedure TEXT, applicationDetails TEXT, PRIMARY KEY(id, postingDate) ) ''')
if __name__ == "__main__": ...
Parsing to .db
Finally, with some additional code for properly parsing through all subfolders in my local directory and setting the postingDate
value based on the folder name5 for each file, I can transform the entire dataset of >1.8k job postings into a single .db
file under 10MB in size in ~3 min (which might’ve taken even less time if it weren’t running on an old spinning hard drive).
import argparsefrom bs4 import BeautifulSoupimport reimport osimport sqlite3from tqdm import tqdmimport logging
def extract_job_id_from_html(soup): ## Try to find job ID in a <h1> tag with the specific class header_tag = soup.find('h1', class_='h3 dashboard-header__profile-information-name mobile--small-font color--font--white margin--b--s') if header_tag: header_text = header_tag.get_text(strip=True) match = re.match(r'^(\d+)', header_text) if match: return match.group(1)
## If not found, try to find an <h1> tag containing the words "Job ID" job_id_tag = soup.find('h1', string=re.compile(r'Job ID', re.IGNORECASE)) if job_id_tag: job_id_text = job_id_tag.get_text(strip=True) match = re.search(r'Job ID\s*:\s*(\d+)', job_id_text, re.IGNORECASE) if match: return match.group(1)
return None
def parse_html_file(filepath, job_posting_date, verbose=False): with open(filepath, 'r', encoding='utf-8') as file: html_content = file.read()
soup = BeautifulSoup(html_content, 'lxml')
## Extract the year, month, and day from the job_posting_date string posting_date = job_posting_date.split('_')[0] data = {'postingDate': posting_date} job_id = extract_job_id_from_html(soup) if job_id: data['id'] = job_id
rows = soup.find_all('tr') ## find all table rows
for row in rows: tds = row.find_all('td') ## find all table data cells
if len(tds) >= 2: label_td = tds[0] label_text = '\n'.join(label_td.stripped_strings).replace(':', '')
value_td = tds[1] value_text = '\n'.join(value_td.stripped_strings)
links = value_td.find_all('a') for link in links: url = link.get('href') link_text = link.get_text() value_text = value_text.replace(link_text, f'{link_text} ({url})')
## Map label_text to corresponding database column column_mapping = { ## 'Job ID': 'id', ## 'Job Posting Date': 'postingDate', 'Job Title': 'title', 'Organization': 'company', 'Division': 'companyDivision', 'Website': 'companyWebsite', 'Job Location': 'location', 'Job Location Type': 'locationType', 'Number of Positions': 'numPositions', 'Salary': 'salary', 'Start Date': 'startDate', 'End Date': 'endDate', 'Job Function': 'function', 'Job Description': 'description', 'Job Requirements': 'requirements', 'Preferred Disciplines': 'preferredDisciplines', 'Application Deadline': 'applicationDeadline', 'Application Method': 'applicationMethod', 'Application Receipt Procedure': 'applicationReceiptProcedure', 'If by Website, go to': 'applicationReceiptProcedure', 'Additional Application Information': 'applicationDetails', }
## Check if label_text matches any of the predefined columns if label_text in column_mapping: db_column = column_mapping[label_text] ## If key already exists, append the value to it if db_column in data: data[db_column] += f'\n{value_text}' else: data[db_column] = value_text
return data
def store_data_in_db(data, db_cursor): columns = ', '.join([f'"{key}"' for key in data.keys()]) placeholders = ', '.join(['?' for _ in data.values()]) sql = f'INSERT INTO "JobPosting" ({columns}) VALUES ({placeholders})' try: db_cursor.execute(sql, tuple(data.values())) except sqlite3.IntegrityError: logging.info("Integrity Error: Skipping row") pass
def create_db_schema(db_cursor): db_cursor.execute(''' CREATE TABLE IF NOT EXISTS JobPosting ( id INTEGER, postingDate DATE, title TEXT, company TEXT, companyDivision TEXT, companyWebsite TEXT, location TEXT, locationType TEXT, numPositions INTEGER, salary TEXT, startDate TEXT, endDate TEXT, function TEXT, description TEXT, requirements TEXT, preferredDisciplines TEXT, applicationDeadline TEXT, applicationMethod TEXT, applicationReceiptProcedure TEXT, applicationDetails TEXT, PRIMARY KEY(id, postingDate) ) ''')
if __name__ == "__main__": logging.basicConfig(filename='run.log', level=logging.INFO, format='%(asctime)s %(message)s')
parser = argparse.ArgumentParser(description="Parse HTML files in a folder and store data in SQLite DB.") parser.add_argument("-d", "--directory", default=os.getcwd(), help="Path to the directory containing HTML files. Default is the current directory.") parser.add_argument("--db", default=os.path.join(os.getcwd(), "job_postings.db"), help="SQLite database file to store the parsed data. Default is 'job_postings.db' in the directory specified by -d.") parser.add_argument("-v", "--verbose", action="store_true", help="logging.info parsed data.")
args = parser.parse_args()
conn = sqlite3.connect(args.db) cursor = conn.cursor() create_db_schema(cursor)
## Get the list of files files = [os.path.join(dirpath, file) for dirpath, _, files in os.walk(args.directory) for file in files if file.endswith('.html') or file.endswith('.htm')]
## Create a progress bar with tqdm(total=len(files)) as pbar: for subdir, _, files in os.walk(args.directory): job_posting_date = os.path.basename(subdir) for file in files: if file.endswith('.html') or file.endswith('.htm'): filepath = os.path.join(subdir, file) logging.info(filepath) data = parse_html_file(filepath, job_posting_date, args.verbose) store_data_in_db(data, cursor) ## Update the progress bar pbar.update(1)
conn.commit() conn.close() logging.info("Parsing and storing completed.")
Viewing the data
And so we have it: a single database file storing every single job posted on the UofT PEY Co-op job board for 2T5s6 (from 2023 to 2024). You can take a look at some of the results from querying the data below7, or download the SQLite .db
file from the latest release at github.com/sadmanca/uoft-pey-coop-job-postings.
Sample Queries
Which companies posted the most number of jobs?
SELECT company, COUNT(*) as num_postingsFROM JobPostingGROUP BY companyORDER BY num_postings DESC;
Company | Num Postings |
---|---|
Sanofi Canada | 92 |
Celestica Inc. | 74 |
Hydro One | 62 |
Huawei Technologies Canada Co.,Ltd. | 47 |
Pulsenics Inc | 35 |
Airbus | 31 |
RAISE (Ian Martin Group) on behalf of Enbridge | 30 |
Geotab | 30 |
The Independent Electricity System Operator (IESO) | 26 |
SOTI Inc. | 25 |
Ericsson | 23 |
STEMCELL Technologies | 22 |
Isowater Corporation | 22 |
Toronto Hydro | 20 |
Veoneer Canada Inc. (Formerly Autoliv) | 18 |
AMD (Advanced Micro Devices, Inc.) | 18 |
The Six Semiconductor | 17 |
Cenovus | 17 |
Qualcomm Canada Inc. | 15 |
Prep Doctors | 14 |
Kepler Communications | 13 |
Evertz Microsystems Ltd | 13 |
Agnico Eagle Mines Ltd. | 13 |
Xero | 12 |
Untether AI | 12 |
National Research Council Canada | 12 |
Bombardier Aerospace | 12 |
AlphaWave SEMI | 12 |
Vale Canada | 11 |
Questrade | 11 |
Paradigm Electronics Inc. | 11 |
Lincoln Electric Company of Canada | 11 |
IBI Group | 11 |
FGF Brands | 11 |
Environment and Climate Change Canada | 11 |
City of Toronto | 11 |
Atlantic Packaging Products Ltd. | 11 |
Acciona Infrastructure Canada Inc. | 11 |
ecobee | 10 |
SAFRAN Landing Systems (Messier-Dowty Inc.) | 10 |
IKO Industries | 10 |
Ceridian | 10 |
Synopsys | 9 |
NETINT Technologies Inc. | 9 |
MHIRJ Aviation Group | 9 |
Litens Automotive Group | 9 |
Kinectrics Inc. | 9 |
Collins Aerospace | 9 |
Cerebras Systems | 9 |
Canadian Natural Resources Limited | 9 |
Brigham & Women’s Hospital, Harvard Medical School | 9 |
York Region | 8 |
Toronto Transit Commission (TTC) | 8 |
Tenstorrent Inc | 8 |
Honda of Canada Mfg | 8 |
Stackpole International | 7 |
EllisDon | 7 |
Aecon Group Inc. | 7 |
eCAMION | 6 |
Thermo Fisher Scientific | 6 |
Royal Canadian Mounted Police (RCMP) | 6 |
Powersmiths International Corp. | 6 |
Ontario Clean Water Agency (OCWA) | 6 |
Northbridge Financial | 6 |
NVIDIA | 6 |
NOVA Chemicals | 6 |
Mondelez International | 6 |
Ministry of Transportation | 6 |
Mattamy Homes Ltd. | 6 |
Marvell Technology Group (US) | 6 |
Martinrea International Inc | 6 |
Ledcor | 6 |
Kingston Midstream | 6 |
Global Affairs Canada | 6 |
Genesys Canada Laboratories Inc. (Genesys) | 6 |
ExxonMobil Canada | 6 |
Amazon | 6 |
Welbilt | 5 |
WSP Canada Inc. | 5 |
Suncor Energy | 5 |
Smith + Andersen | 5 |
Purpose Building Inc. | 5 |
Ontario Teachers Pension Plan (OTPP) | 5 |
Mold-Masters Limited | 5 |
Mission Control Space Services | 5 |
Ministry of the Environment, Conservation and Parks | 5 |
Microchip Technology | 5 |
Jaguar Land Rover Canada | 5 |
Husky Injection Molding Systems Ltd. | 5 |
Colortech Inc. | 5 |
BBA | 5 |
Astera Labs | 5 |
Arxtron Technologies | 5 |
Air Canada | 5 |
eShipper | 4 |
e-Zinc Inc | 4 |
Zynga | 4 |
WOLF Advanced Technology | 4 |
VPC Group Inc. | 4 |
University of Toronto - Facilities & Services | 4 |
The Travel Corporation | 4 |
Tesla Motors | 4 |
Synaptive Medical Inc. | 4 |
Solvay Inc | 4 |
Scotiabank | 4 |
Purolator Inc. | 4 |
Petro Canada Lubricants (Hollyfrontier) | 4 |
Peel Plastic Products Limited | 4 |
PICCO Group of Companies | 4 |
Ministry of Children, Youth, and Social Services | 4 |
KAPP Infrastructure Inc. | 4 |
Intersect Power | 4 |
Intel Canada | 4 |
Grounded Engineering | 4 |
GHD | 4 |
CGI Group Inc. | 4 |
Bronte Construction | 4 |
Bayer Healthcare | 4 |
Alectra Utilities | 4 |
Zebra Technologies | 3 |
Viavi Solutions | 3 |
Umicore Autocat Canada Corp | 3 |
Uken Games | 3 |
Tencent Technology Company | 3 |
TYLin | 3 |
Seismic Software, Inc. | 3 |
Region of Peel | 3 |
Region of Durham | 3 |
Pulsenics | 3 |
Perpetua | 3 |
Nfinite Nanotechnology | 3 |
Nanoleaf | 3 |
Movellus | 3 |
Mother Parker’s Tea and Coffee | 3 |
Ministry of Health and Long-Term Care | 3 |
Magna International | 3 |
MDA | 3 |
Lumentum Inc. | 3 |
LEA Consulting Ltd. | 3 |
Kernal Biologics, Inc. | 3 |
InteraXon Inc | 3 |
Halton Region | 3 |
Green Infrastructure Partners Inc | 3 |
GGY a Moodys Analytics Company | 3 |
FedEx Ground | 3 |
FedEx Express Canada | 3 |
Eastern Power Limited | 3 |
Dynasty Power | 3 |
Deighton Associates Ltd. | 3 |
Defence Research and Development Canada | 3 |
Defence R&D Canada (DRDC) - Ottawa | 3 |
Circle Cardiovascular Imaging Inc. | 3 |
Ciena | 3 |
Cadence Design Systems Inc | 3 |
CES Transformers | 3 |
C. F. Crozier & Associates | 3 |
Boston Scientific | 3 |
Boeing | 3 |
Arctic Wolf Networks | 3 |
Aercoustics Engineering Limited | 3 |
AYOS Designs | 3 |
AGS Automotive Systems | 3 |
Zaber Technologies | 2 |
Xpan Inc. | 2 |
WalterFedy | 2 |
Volta Energy | 2 |
Veeva Systems | 2 |
Varicent | 2 |
Vale | 2 |
Urbantech Consulting | 2 |
University of Toronto - ITS Enterprise Applications and Solutions Integration | 2 |
University of Toronto | 2 |
Turnkey Modular Systems Inc | 2 |
Thornhill Medical | 2 |
Thales Canada | 2 |
Terrestrial Energy | 2 |
TC Energy (formerly TransCanada) | 2 |
Synergy Partners | 2 |
Stryker | 2 |
Structura Biotechnology Inc. | 2 |
StratumAI | 2 |
StoneX Group Inc. | 2 |
Square | 2 |
Spring Air Systems Inc. | 2 |
SparkLease Inc | 2 |
Snowflake | 2 |
Smarter Alloys | 2 |
SmartTrade Technologies | 2 |
SickKids | 2 |
Shell Canada Limited | 2 |
Safuture Inc. | 2 |
Royal Bank of Canada (RBC) | 2 |
Read Jones Christoffersen (RJC Engineers) | 2 |
Rapyuta Robotics Co., Ltd. (SWITZERLAND) | 2 |
Rakuten Kobo Inc. | 2 |
RBC Capital Markets | 2 |
Quantum Lifecycle Partners LP | 2 |
Quanta Technology | 2 |
Qualitrol Corp | 2 |
Overbond | 2 |
Nuclear Waste Management Organization | 2 |
Next Hydrogen Corporation (NHC) | 2 |
Mokal Industries | 2 |
ModiFace | 2 |
Modern Niagara Group Inc. | 2 |
Magna | 2 |
Lobo Consulting Services Inc. | 2 |
Liburdi Turbine Services | 2 |
Lanxess | 2 |
Lake Harbour Co Ltd | 2 |
Kijiji | 2 |
KGHM International LTD. | 2 |
Jamieson Wellness Inc. | 2 |
Intel Corporation | 2 |
Inoki | 2 |
Indigenous Services Canada | 2 |
Indie Semiconductor | 2 |
Hungerford Consulting Ltd. | 2 |
Health Canada | 2 |
Hatch Ltd. | 2 |
Harmonic Fund Services | 2 |
Hanon Systems EFP Canada Ltd. (Formerly Magna) | 2 |
HBNG Holborn Group | 2 |
Groq Inc. | 2 |
Griffith Foods Ltd. | 2 |
Grascan Construction Ltd. | 2 |
G&W Canada Corporation | 2 |
Faire | 2 |
Evoco Ltd | 2 |
Empire Life | 2 |
Electrovaya Corp. | 2 |
EHV Power | 2 |
Dragados Canada | 2 |
Deep Genomics | 2 |
Continuous Colour Coat Limited | 2 |
Coltene SciCan | 2 |
City of Mississauga | 2 |
Chatsimple | 2 |
ChargeLab Inc | 2 |
CentML Inc | 2 |
Canadian Tire Corporation | 2 |
Canadian Nuclear Safety Commission | 2 |
Canadensys Aerospace | 2 |
Cadillac Fairview | 2 |
CIMCO Refrigeration | 2 |
CERT Systems Inc. | 2 |
British Columbia Investment Management Corp | 2 |
BlueRock Theraputics | 2 |
Bloom AI | 2 |
Berg Chilling Systems | 2 |
Bank of Montreal (BMO) | 2 |
Bank of America Merrill Lynch | 2 |
BMO Financial Group | 2 |
Astro Shoring | 2 |
Arup | 2 |
Arctic Canadian Diamond Company Ltd. | 2 |
Aqusense | 2 |
Alta Planning + Design Canada, Inc. | 2 |
ACS Powersports | 2 |
iManage | 1 |
ePIC Blockchain Technologies | 1 |
Zomp Inc. | 1 |
ZS Associates | 1 |
YScope Inc | 1 |
Wolfe Heavy Equipment | 1 |
Walmart Canada | 1 |
Wakefield Canada - Castrol | 1 |
Volvo Cars | 1 |
Vivid Machines | 1 |
Vexos | 1 |
Veolia Water Technologies & Solutions | 1 |
Upstream Works Software | 1 |
Upper Canada Consultant | 1 |
University of Toronto Scarborough Campus | 1 |
UT Elevator (NA) Inc. | 1 |
UHN- (Princess Margaret, Toronto General, Toronto Western, Toronto Rehab) | 1 |
Tridel | 1 |
Trexo Robotics | 1 |
Trend Micro Canada Technologies Inc. | 1 |
Tractel Ltd | 1 |
Toyota | 1 |
Town of Oakville | 1 |
Thomson Reuters | 1 |
Third Rails Ltd | 1 |
The Poirier Group | 1 |
The Napoleon Group of Companies | 1 |
The Miller Group | 1 |
The Master Group | 1 |
Tetra Trust | 1 |
Tetra Tech | 1 |
Tersa Earth Innovations | 1 |
Tenaris Shawcor | 1 |
Teck Resources Ltd. | 1 |
Technical University of Munich (TUM) | 1 |
TD Securities | 1 |
Synopsys Inc | 1 |
Sun Life Financial | 1 |
Stelco | 1 |
Stantec Consulting Ltd. | 1 |
Sowingo | 1 |
SnapWrite | 1 |
Slate Technologies Canada Limited | 1 |
Simpplr | 1 |
Signal 1 | 1 |
Siemens Canada Limited | 1 |
Semtech | 1 |
Select Equity Group, L.P. | 1 |
Salytics | 1 |
Safety Power Inc | 1 |
SRK Consulting (Canada) Inc. | 1 |
SNC-Lavalin | 1 |
SLR Consulting (Canada) Ltd. | 1 |
SGS Canada Inc | 1 |
S&C Electric Canada Ltd. | 1 |
RxFood | 1 |
Rockwool | 1 |
RocMar Engineering Inc. | 1 |
Ripple Therapeutics | 1 |
Reinsurance Management Associates | 1 |
Regional Municipality of York (York Region) | 1 |
QMC Metering Solutions | 1 |
Public Services and Procurement Canada | 1 |
Providence Therapeutics | 1 |
Procter & Gamble (P&G) | 1 |
ProAutomated | 1 |
Princess Margaret Cancer Centre, University Health Network | 1 |
Pratt & Whitney Canada Inc. | 1 |
Powertech Labs Inc. | 1 |
Power Advisory | 1 |
Posterity Group | 1 |
Pomerleau, Inc | 1 |
PointClickCare | 1 |
Pinchin Ltd. | 1 |
PepsiCo | 1 |
Pelicargo | 1 |
PartnerRe Life Reinsurance Company of Canada | 1 |
Parsons Inc. | 1 |
Parametric Research Labs Inc. | 1 |
Pani Energy | 1 |
Ovintiv | 1 |
Ontario Transit Group | 1 |
Ontario Public Service | 1 |
Ontario Ministry of Transportation | 1 |
OPTrust | 1 |
ONIT Energy Ltd | 1 |
Nutrien | 1 |
Nuclear Promise X Inc. | 1 |
Nojumi Solutions Inc. | 1 |
New School Foods Inc. | 1 |
NAPCO, Royal Pipe & Fittings | 1 |
Modern Niagara | 1 |
Micrometric Jig Boring & Jig Grinding Ltd. | 1 |
Metro Inc. | 1 |
Metric Contracting Service Corporation | 1 |
Mesosil | 1 |
McCain Foods | 1 |
Mars Canada Inc. | 1 |
Manulife Financial | 1 |
Manulife | 1 |
Mancor Industries | 1 |
Lumerate | 1 |
Loblaw Companies Limited | 1 |
Lactalis Canada | 1 |
Konrad Group | 1 |
Kinross Gold Corporation | 1 |
Kiewit | 1 |
Where are most jobs located?
SELECT location, COUNT(*) as num_postingsFROM JobPostingGROUP BY locationORDER BY num_postings DESC;
Location | Num Postings |
---|---|
Toronto | 295 |
Toronto, ON | 168 |
Mississauga, ON | 73 |
Mississauga | 55 |
Markham, Ontario | 52 |
Oakville, ON | 38 |
Ottawa, ON | 35 |
Mirabel, Quebec | 31 |
Markham | 27 |
Vancouver, BC | 25 |
North York, ON | 20 |
Toronto, ON, CA | 18 |
Owen Sound | 18 |
Ottawa | 18 |
Oakville | 18 |
7455 Birchmount Rd, Markham, ON L3R 5C2, Canada | 18 |
Markham, ON | 17 |
Main Office (Markham, ON) | 17 |
Connaught Campus, 1755 Steeles Avenue West, Toronto, ON, Canada, M2R 3T4 | 17 |
Brampton | 17 |
Toronto, Ontario | 15 |
Mississauga, Ontario | 15 |
Vaughan | 14 |
Brampton, ON | 13 |
Burlington | 12 |
Etobicoke | 11 |
1755 Steeles Avenue West, Toronto, ON, Canada, M2R 3T4 | 11 |
Markham, Ontario, Canada | 10 |
Calgary | 10 |
Scarborough | 9 |
Whitby | 8 |
Remote | 8 |
Etobicoke, Ontario | 8 |
Concord, Ontario | 8 |
Canada | 8 |
Rankin Inlet, Nunavut | 7 |
Newmarket | 7 |
7 | |
Woodbridge | 6 |
Remote / Toronto | 6 |
North York | 6 |
Newmarket, Ontario | 6 |
National Capital Region, Ottawa | 6 |
Estevan, SK | 6 |
Concord, ON | 6 |
Calgary, Alberta | 6 |
Calgary, AB - Head Office or Fort McMurray, AB ? Horizon and Albian Mine Site | 6 |
Alliston, Ontario | 6 |
Ajax, ON | 6 |
1755 Steeles Avenue West, Toronto, ON | 6 |
Ottawa, Ontario | 5 |
Ontario | 5 |
Lloydminster, SK | 5 |
Joffre, AB | 5 |
Cambridge, Massachusetts, USA | 5 |
Calgary, AB | 5 |
CA-AB-Calgary | 5 |
240 Richmond Street West Toronto, ON, Canada, M5V 1V6 | 5 |
Waterloo | 4 |
Toronto, Canada | 4 |
Newmarket, ON | 4 |
Mississauga, ON, CA | 4 |
Markham, Canada | 4 |
Collingwood | 4 |
Bolton, ON | 4 |
AI CENTRE of EXCELLENCE (Digital Data Hub), 240 Richmond Street West Toronto, ON, Canada, M5V 1V6 | 4 |
49 Rutherford Rd, Brampton | 4 |
3966 JinDu Rd., Shanghai | 4 |
2430 Royal Windsor Drive, Mississauga, Ontario | 4 |
196 Spadina Ave, Toronto ON | 4 |
1890 Alstep Dr, Mississauga, ON L5S 1W1 | 4 |
175 Mostar Street, Suite 200, Stouffville, Ontario | 4 |
175 Galaxy Blvd., Toronto, ON | 4 |
1 Commerce Valley Dr E, Thornhill, ON L3T 7X6 | 4 |
Winnipeg, MB | 3 |
Whitby/Scarborough | 3 |
Vaughan, ON | 3 |
Vancouver | 3 |
Toronto, Ontario, CA | 3 |
Toronto, ON, Canada | 3 |
Toronto or Thornhill | 3 |
Sudbury | 3 |
St. George (downtown Toronto) | 3 |
Sarnia, Ontario | 3 |
Mississauga, 2100 Meadowvale Blvd. (Hwy 401/Mississauga Rd) | 3 |
Fargo, North Dakota | 3 |
Downtown Toronto | 3 |
Chatham, ON | 3 |
Cambridge, MA (USA) | 3 |
Burlington, On | 3 |
Burlington, ON | 3 |
Brampton, ON (87 Orenda Road) | 3 |
Beijing, China | 3 |
Barrie, ON | 3 |
Baker Lake or Rankin Inlet, Nunavut | 3 |
Alberta | 3 |
Aercoustics Office - 1004 Middlegate Road, Mississauga | 3 |
90 Bales Drive East, East Gwillimbury | 3 |
71 Rexdale Boulevard | 3 |
500 Commissioners Street | 3 |
33 Kern road | 3 |
2400 Royal Windsor Drive, Mississauga, Ontario | 3 |
150 Toro Road | 3 |
Yellowknife, NT | 2 |
Woodbridge, Ontario | 2 |
Waterloo, ON | 2 |
Vaughan, Ontario | 2 |
Various in Canada | 2 |
Vancouver, British Columbia | 2 |
Toronto, Hybrid | 2 |
Toronto or Ottawa | 2 |
Toronto Office: 317 Adelaide Street West, Toronto | 2 |
Toronto (HQ) - Hybrid | 2 |
Toronto (Gardiner Expressway at Exhibition Station) | 2 |
Toronto & Vancouver | 2 |
Tiverton, Ontario | 2 |
Throughout Toronto | 2 |
TOKYO, JAPAN | 2 |
Stouffville | 2 |
SickKids Research Institute - 686 Bay St. Toronto, ON M5G 0A4 | 2 |
See Notes | 2 |
Santa Clara, California, USA | 2 |
Santa Clara, CA (USA) | 2 |
ST. George Campus | 2 |
Richmond Hill | 2 |
Remote in Canada | 2 |
Remote (HQ located in Montreal) | 2 |
Rankin Inlet or Baker Lake, Nunavut | 2 |
Ottawa/Gatineau | 2 |
Orillia | 2 |
Ontario, Canada | 2 |
One of 71 Rexdale Boulevard OR 715 Milner Avenue | 2 |
One of 500 Commissioners St, 71 Rexdale Blvd, 715 Milner Ave | 2 |
On-site | 2 |
Oakville, Ontario | 2 |
North York, Ontario | 2 |
North York, ON or Chatham, ON | 2 |
Newmarket, ON, CA | 2 |
Multiple locations | 2 |
Mont-Saint-Hilaire, QC | 2 |
Metro Hall, 55 John Street, Toronto, ON | 2 |
Markham, ON, CA | 2 |
Kitchener | 2 |
Hybrid Work Environment - Virtual and/or East Gwillimbury, Ontario | 2 |
Greater Toronto Area | 2 |
Georgetown, ON, Canada | 2 |
Georgetown, ON | 2 |
GTA, London/Guelph, Ottawa | 2 |
GTA | 2 |
Fort Saskatchewan, AB | 2 |
Fort McMurray, Alberta | 2 |
Etobicoke, ON | 2 |
Downtown Toronto or Downtown Ottawa | 2 |
DRDC Ottawa, Shirley’s Bay Campus, 3701 Carling Avenue, Ottawa, ON K1A 0Z4 | 2 |
Collingwood ON | 2 |
Cambridge, ON | 2 |
Cambridge | 2 |
Calgary and Various Locations | 2 |
Burnaby, BC | 2 |
Boston, MA, USA | 2 |
Boston, MA | 2 |
Bolton, Ontario | 2 |
Bolton | 2 |
Boisbriand/Mississauga | 2 |
Barrie, ON, CA | 2 |
Barrie | 2 |
Alliston | 2 |
Ajax, Ontario | 2 |
Ajax | 2 |
95 Arrow Road, M9M 2L4 | 2 |
901 Simcoe Street South Oshawa | 2 |
843 Eastern Ave & Union Station | 2 |
8027 Dixie Rd. Brampton | 2 |
7455 Birchmount Road, Markham, ON | 2 |
715 Milner Avenue | 2 |
71 Rexdale Blvd | 2 |
6688 Kitimat Rd., Mississauga, ON, L5N 1P8 | 2 |
60 Wingold Ave, Toronto, Ontario M6B 1P5 | 2 |
501 Alliance Ave, Suite 406, Toronto, ON | 2 |
400 Highway 6 North, DUNDAS, ON L9H 7K4 | 2 |
40 Bertrand Ave, Scarborough, ON M1L 2P6, Canada. | 2 |
393 University Avenue | 2 |
3745 Barnes Lake Road - Ashcroft, BC | 2 |
3484 Semenyk Ct, Mississauga | 2 |
300 - 3200 Dufferin St | 2 |
3 Paisley Ln, Uxbridge, ON L9P 0G5 | 2 |
277 Gladstone Ave. Toronto, Ontario, M6J 3L9 | 2 |
250 University Avenue Suite 400, Toronto | 2 |
250 Ferrand Drive | 2 |
2440 Winston Park Dr, Oakville, ON L6H 7V2 | 2 |
2425 Matheson Blvd E #200, Mississauga, ON L4W 5K4, Canada | 2 |
24 Buckingham St, Etobicoke M8Y 2W2 | 2 |
20 carlson court | 2 |
1755 Steeles Avenue West, Toronto, ON | 2 |
1138 Bathurst Street (Bathurst & Dupont) | 2 |
111 Progress Ave and 333 progress Ave, Scarborough | 2 |
1 York Street Suite 1010 | 2 |
toronto, ontario | 1 |
Woodbridge, ON | 1 |
West Hill, ON, CA, M1E 2K3 | 1 |
Waterloo/Remote | 1 |
Waterloo, Ontario | 1 |
Wakefield Canada | 1 |
Virtual | 1 |
Victoria, BC | 1 |
Various location in Canada | 1 |
Various in Ontario | 1 |
Various Construction Field Offices within the Metropolitan Toronto area | 1 |
Town of Oakville Town Hall: 1225 Trafalgar Road, Oakville; and Work From Home | 1 |
Toronto/Thornhill | 1 |
Toronto/Barrie, ON | 1 |
Toronto, Ontario OR Montreal, Quebec | 1 |
Toronto, Ontario (Hybrid) | 1 |
Toronto, ON or Calgary, AB | 1 |
Toronto, ON (various locations) | 1 |
Toronto, CA | 1 |
Toronto and Kitchener | 1 |
Toronto OR Gatineau | 1 |
Toronto ON | 1 |
Toronto (Hybrid) | 1 |
Toronto (HQ) - Hybrid - In office once a week | 1 |
Tiverton | 1 |
Thunder Bay, ON | 1 |
Thornhill, ON | 1 |
TORONTO | 1 |
TD Centre, 66 Wellington St W, Toronto | 1 |
Surrey, BC | 1 |
Suite 440, 20 Toronto Street, Toronto, ON M5C 2B8 | 1 |
Sudbury, Ontario | 1 |
Sudbury, ON, CAN | 1 |
Sudbury, ON Canada | 1 |
Strathroy, ON | 1 |
St. Clair River / Toronto | 1 |
St. Catharines, ON | 1 |
Scarborough, Ontario | 1 |
Richmond hill | 1 |
Rexdale, ON, CA | 1 |
Regina | 1 |
Rainbow Lake, AB | 1 |
Princess Margaret Cancer Centre | 1 |
Pickering, ON | 1 |
Peterborough, Ontario | 1 |
Ottawa, Toronto, North Bay | 1 |
Ottawa, On | 1 |
Ottawa (preferred) or remote | 1 |
Orillia, ON, CA | 1 |
Oakville, ON or Toronto, ON | 1 |
OPS office locations in Ontario (Toronto, Oshawa, Guelph, St. Catherines, North Bay, Kingston, Sudbury, London, Ottawa, Newmarket, Hamilton, Thunder Bay, Sault Ste Marie, and Peterborough) and/or remotely following a hybrid model | 1 |
North York, Toronto OR Calgary, Alberta | 1 |
North York, ON or Toronto, ON | 1 |
North Bay | 1 |
Nepean | 1 |
Nanticoke, ON, CA | 1 |
NEWMARKET, ON | 1 |
Munich, Germany (Preferred) or Toronto, Canada | 1 |
Multiple | 1 |
Mountain View, CA | 1 |
Mooretown, ON | 1 |
Montreal, Toronto | 1 |
Montreal, Quebec | 1 |
Mississuaga, ON | 1 |
Mississauga,ON | 1 |
Mississauga, Ontarip | 1 |
Mississauga, On | 1 |
Mississauga, Canada | 1 |
Markhan | 1 |
Markham, ON Canada | 1 |
Markham, Mississauga, OR Ottawa, ON | 1 |
Markham or Oakville | 1 |
Markham or Mississauga, ON | 1 |
Manhattan, NY | 1 |
MaRS Centre, 101 College Street, Toronto | 1 |
London, UK | 1 |
London | 1 |
Lloydminster, AB; Lloydminster, SK | 1 |
Laval, Quebec | 1 |
Lakefield, ON | 1 |
Lake Charles, Louisiana | 1 |
Kingston, ON | 1 |
Kingston (294 King Street East) and/or Toronto (4900 Yonge Street) | 1 |
Kincardine, ON, CA | 1 |
Kincardine | 1 |
Impala Canada | 1 |
Hyrbid, Toronto | 1 |
Hybrid, Toronto | 1 |
Hamilton, Ontario | 1 |
Hamilton and/or Vaughan offices | 1 |
Guelph Office | 1 |
Greater Sudbury, ON | 1 |
GTA, or outskirts of Ontario | 1 |
GTA, London/Guelph, | 1 |
For McMurray | 1 |
Flexible | 1 |
Etobicoke, Ontario/ Remote | 1 |
Edmonton, AB, CA | 1 |
Edmonton or Calgary, AB | 1 |
Dresden, ON | 1 |
Downtown Toronto, Richmond Hill, Vancouver / Virtual | 1 |
Downtown Toronto (remote) | 1 |
Downsview, 123 Garratt Blvd, North York, ON | 1 |
Dorval, Quebec | 1 |
Delta, Canada | 1 |
Copper Cliff, ON | 1 |
Connaught Campus,1755 Steeles Avenue West, Toronto, ON, Canada, M2R 3T4 | 1 |
Concord | 1 |
How many job postings are there for mechatronics positions?
SELECT COUNT(*) AS num_mech_postingsFROM JobPostingWHERE title LIKE '%mechatronic%' OR company LIKE '%mechatronic%' OR companyDivision LIKE '%mechatronic%' OR function LIKE '%mechatronic%' OR description LIKE '%mechatronic%' OR requirements LIKE '%mechatronic%' OR preferredDisciplines LIKE '%mechatronic%';
┌─────────────────────┐│ num_mech_postings ││─────────────────────││ 87 │└─────────────────────┘
What does a job for a mechatronics position look like?
SELECT *FROM JobPostingWHERE title LIKE '%mechatronic%' OR company LIKE '%mechatronic%' OR companyDivision LIKE '%mechatronic%' OR function LIKE '%mechatronic%' OR description LIKE '%mechatronic%' OR requirements LIKE '%mechatronic%' OR preferredDisciplines LIKE '%mechatronic%'LIMIT 1;
id | postingDate | title | company | companyDivision | companyWebsite | location | locationType | numPositions | salary | startDate | endDate | function |
---|---|---|---|---|---|---|---|---|---|---|---|---|
43873 | 2023-09-18 | Operational Technology Intern | Honda of Canada Mfg | Recruitment | https://www.hondacanadamfg.ca/ | Alliston, Ontario | On-Site | 45 | $26.17 hourly for 40.0 hours per week | 05/06/2024 | 08/25/2025 | Engineering |
Next Steps
Edit (2024-11-11): part 2 of this post series was intended to be about cleaning the data. Unfortunately, I’ve been so preoccupied with other things (including a better dashboard for 2024-2025 co-op job postings) that I haven’t had the time to do any of that 😅, so part 2 ended up being about the dashboard I built to display all this job info on my website. As it is, I’ll leave the data cleaning for another day, but I hope you found the initial insights interesting!
You’ll notice that the data still needs some cleaning8, which is fine, should be fairly simple to do by manually parsing through the dataset and aggregating similar values.
But after some basic data cleaning is the fun part: analyzing the data to generate some insights on posted jobs, including…
- How many jobs are posted for chemical/mechanical/mineral/… engineers?
- During what periods are the bulk of jobs posted?
- When do specific companies post most/all their jobs?
- How many jobs are reposted9, and which ones?
- …and more!
Footnotes
-
Chrome and virtually all other browsers have a slightly more complicated setup for saving pages which makes organizing files for saved pages slightly less elegant compared to dealing with just a single file via SingleFile: when you press Ctrl + S on a page, it doesn’t just save that page’s HTML file but also a folder containing all of the media from the page (which, given that none of the job postings contain images, is just one more thing to have to delete). ↩
-
Despite a large portion of the saved HTML files consisting of useless space in lines of JavaScript, CSS, and HTML that have nothing to do with the data that counts, it’s just not worth the time investment for me to parse through every file and remove the clutter since all the saved job postings for a year only take up a couple hundred MB. ↩
-
At times purposefully by myself (e.g. adding Job ID and later Job Title to filenames for saved HTML files for easier file browsing and duplication checking), and at times by the university (i.e. there was a big redesign of the job board that took place in the latter half of the fall semester, which made it so the PEY job board uses the same frontend design as job boards on CLNx, whereas prior to that it looked a bit different despite functionally working the same). ↩
-
At one point, all of my extensions were uninstalled somehow (probably due to a Chrome update gone wrong), and so I had forgotten to reconfigure SingleFile to include job IDs in the saved filenames for many ways until I got some free time and was able to pore over my notes (and reconfigure everything back to the way it was). ↩
-
e.g.
📁2023-09-17_20-14-10
; easily achievable thanks to github.com/sadmanca/ahk-scripts/blob/master/keys.ahk ↩ -
UofT Engineering students and alumni often include their graduation year after their name to denote their class. If you start first year in 2021, you will be part of the class of 2025, denoted as “2T5” (pronounced “two-tee-five”). ↩
-
I’ve built-in a SQL view called
JobPostings
(the table with the actual data is calledJobPosting
, without the “s”) that excludes some of the columns with very long values (e.g.description
,requirements
,preferredDisciplines
,applicationDetails
) to make table formatting look a bit better if you’re runningSELECT *
queries. To run queries with the excluded columns, use theJobPosting
table instead. ↩ -
e.g.
Toronto
andToronto, ON
are present as two distinct locations, when really they should just be one. ↩ -
Something the Engineering Career Centre (ECC) people who run the PEY Co-op job board don’t inform you about ahead of time, employers can “repost” a job with the same job id (usually several weeks) after the original which makes it’ll show up under
New Posting Since Last Login
even if you’ve already viewed (and even though it’s technically still the same single job posting). It’s not commonplace but it did occur for a few dozen jobs (I imagine for those where the position was left unfilled after some initial batch of students applied and it was reposted to reinvigorate interest). ↩